Unsupervised domain adaptation (UDA) for person re-ID.
UDA methods have attracted much attention because their capability of saving the cost of manual annotations. There are three main categories of methods.
UDA方法由于节省了手工标注的花费而吸引了许多关注,这里主要有三种方法:
The first category of clustering-based methods maintains state-of-the-art performance to date
第一类集群时至今日也保持着最优的性能
- (Fan et al., 2018) proposed to alternatively assign labels for unlabeled training samples and optimize the network with the generated targets.
原文链接:https://arxiv.org/pdf/1705.10444.pdf
提出为未标记的训练样本交替分配标签(fine-tune与clustering交替的过程),并且用生成的目标优化网络。
1.解决的问题:
(1)用无标签数据(或 其他域有标签数据及无标签数据)训练模型。
(2)把K-means和CNN结合,减轻了cluster太过noisy的问题。
(3)用自步学习更加流畅地完成模型训练。
2.训练步骤:
step1: ImageNet上预训练的ResNet
step2: 用不相关的有标签数据对网络进行初始化
step3: 将无标签的数据喂给网络,进行聚类。(聚类到底是怎样的,如何初始化聚类中心)(觉得这个初始点的选择应该很重要吧)
step4: 选择离中心最近的几个点,加入“可靠训练集”。训练集的标签即为k-means的k。
step5: 将“可靠训练集”的数据喂给网络再次训练。因为模型会越来越好,所以接近聚类中心的样本会随着epoch增加,即本文的卖点之一。(为避免陷入局部最优,先从“可靠度”最高的样本训练起)
step6: 以上3,4,5环节周而复始。直到每次选择的个数稳定。
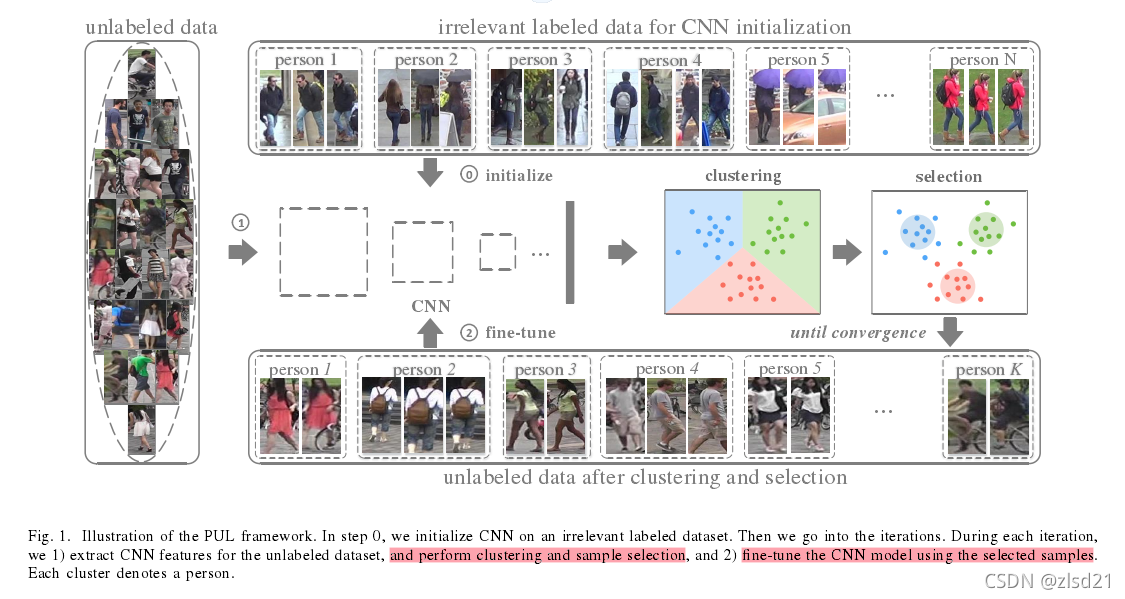 本文提出了一个关于self-paced learning(自步学习)方法,我查了一下,觉得这篇博客较为清晰,供大家参考 https://blog.csdn.net/weixin_37805505/article/details/79144854
- (Lin et al., 2019) proposed a bottom up clustering framework with a repelled loss.
提出了一种具有排斥损失的自顶向下的聚类框架
文章思想:作者的方法考虑到了行人再识别任务的两个基本的事实:不同人间的diversity和同一个人间的similarity。作者的算法最开始把每个人作为单独的一类,来最大化每类的diversity,然后逐渐的把相似的类合并为同一类,来提升每类的similarity。作者在自底向上的聚类过程中利用了一个多样性正则项来平和每个cluster的数据量,最终,作者的模型在diversity和similarity之间达到了很好的平衡。
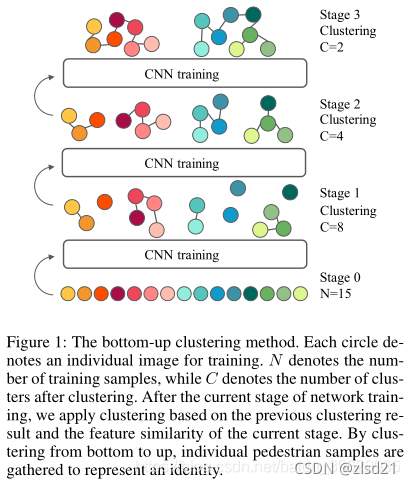 3. (Yang et al., 2019) introduced to assign hard pseudo labels for both global and local features. However, the training of the neural network was substantially hindered by the noise of the hard pseudo labels generated by clustering algorithms, which was mostly ignored by existing methods.
提出用于为全局和局部特征分配的硬伪标签,然而,现有的聚类算法大多忽略硬伪标签的噪声,严重阻碍了神经网络的训练。
文章思想:两个数据库之间,图像风格迥异。如果将图像进行分割,那么每一块之间的风格差异或许会小一点。这样,也就学到了鲁棒性更强的特征。 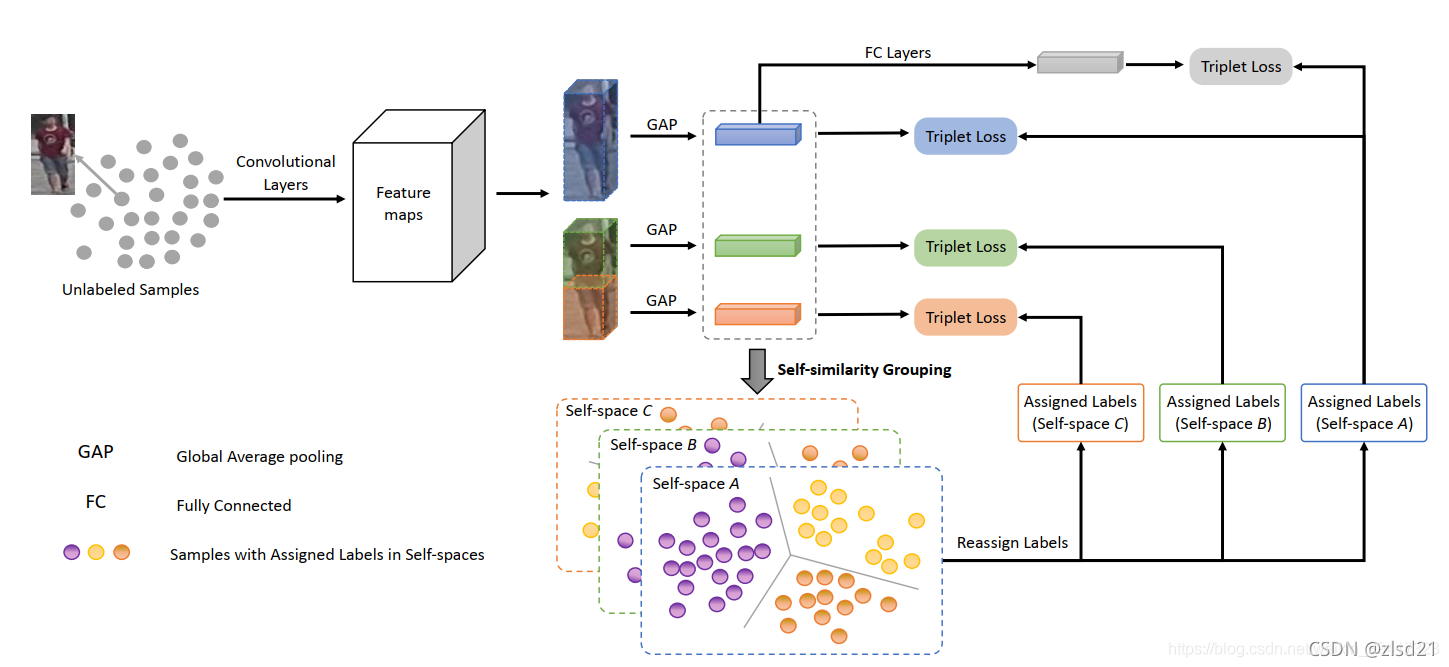 蓝色、绿色、红色分别代表了整个图像,上半图像和下半图像。分别提取出各自的特征向量。灰色的是将三者连接在一起。
对蓝色、绿色、红色三类特征向量分别进行聚类,每一个特征向量就获得了对应的标签。灰色的标签直接和蓝色的保持一致。所以,一个人的上半身、下半身、全身对应的标签可能是不一样的。这就与开头的思想相对应,学习的过程中不会拘泥于整个图像的标签,更具有灵活性。
有了标签之后,对每一类特征向量分别使用最难三元组损失对模型进行训练。
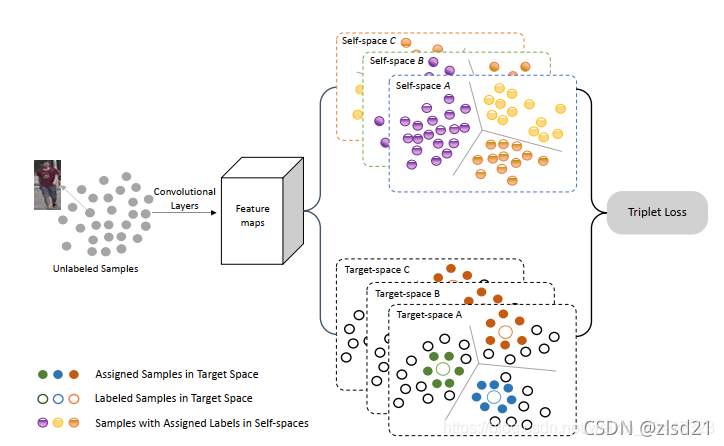 对整张图像的特征向量聚类结束后,总共分为了N类。从每一类中随机挑选一张图像,共N张,分别提取该图像的全局、上半部分、下半部分的特征向量,作为字典。在采样的过程中,对被采到的图像提取三类特征向量,每一类分别和上述提到的N张图像对应类别的特征向量比较,距离最小的,就和其保持相同的标签。在此基础上,再进行最难三元组损失。
原文链接:https://blog.csdn.net/weixin_39417323/article/details/103474275
The second category of methods learns domain-invariant features from style-transferred source-domain images.
第二部分是从style-transferred的源图像学习域不变特征
5. SPGAN (Deng et al., 2018) and PTGAN (Wei et al., 2018) transformed source-domain images to match the image styles of the target domain while maintaining the original person identities. The style-transferred images and their identity labels were then used to fine-tune the model.
转换源域图像以匹配目标域的图像样式,同时保持原始的人的身份。然后使用样式转换的图像及其身份标签对模型进行微调。
文章思想:
1.解决domain gap 问题
该文方法是提出一个“learning via translation”framework,使用GAN把source domain的图片转换到target domain中,并使用这些translated images训练ReID model。流程如下图Figure2。 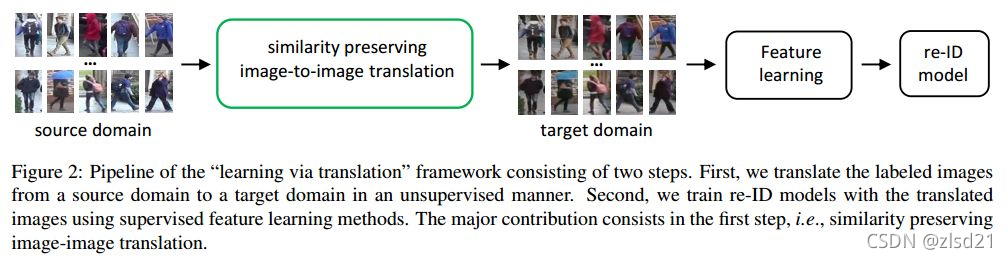 2.解决unsupervised image-image translation过程中,source-domain labels 信息会丢失
(1)对于每张图片,ID信息对于识别有重要意义,需要保留 --> self-similarity。可见下图Figure1,转换前后的图片要尽量相似。
(2)source 和 target domain中包含的人员是没有overlap的,因此,转换得到的图片应该要和target domain的任何一张图片都不相似–> domain-dissimilarity。
因此,作者提出Similarity Preserving GAN (SPGAN)来实现他的两个motivation。 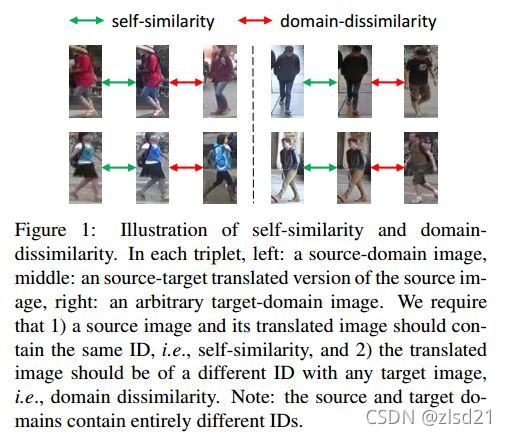
6. HHL (Zhong et al., 2018) learned camera-invariant features with camera style transferred images. However, the retrieval performances of these methods deeply relied on the image generation quality, and they did not explore the complex relations between different samples in the target domain.
HHL (Zhong et al., 2018)学习了相机不变特征相机风格转移的图像。然而,这些方法的检索性能很大程度上依赖于图像生成质量,没有探索目标域中不同样本之间的复杂关系。
引入了一种Hetero(异质)-Homogeneous(同质) Learning (HHL) 学习方法,考虑两个属性:相机不变性,即一张图像迁移到其他相机后ID不变;源域和目标域ID不重叠,因此源域和目标域各选一张图像组成pair必定是负对。前者在目标域操作,为同质;而后者在源域和目标域操作,为异质。 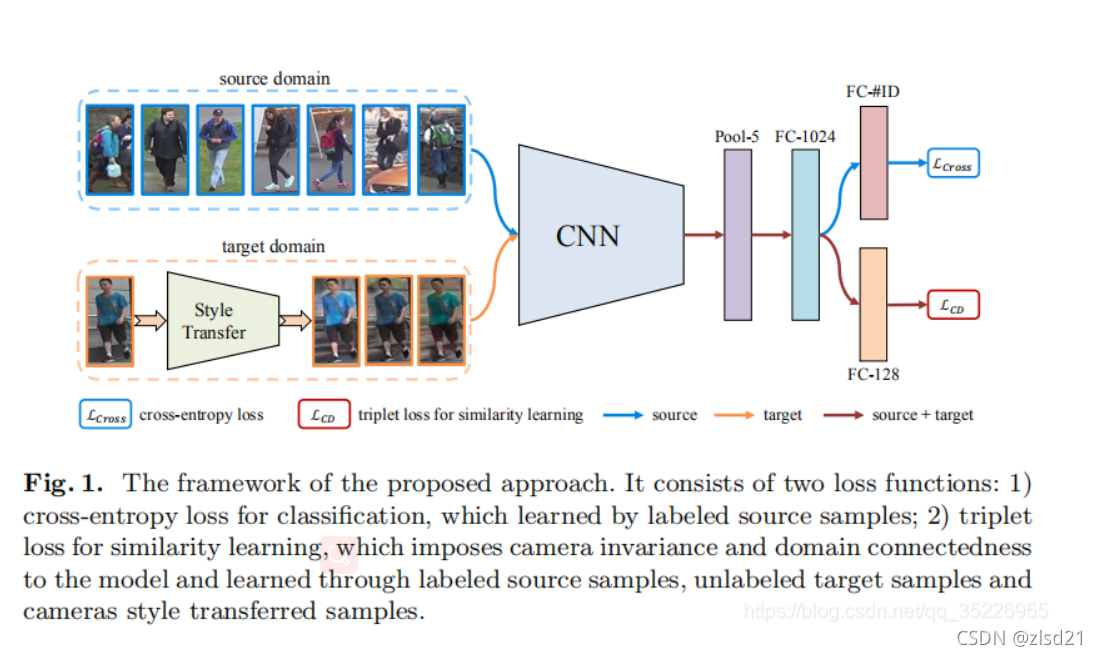 基本思路:利用source domain和target domain进行混合训练,以domain adaption a) Target domain利用camStyle进行各个摄像头的数据增强 b) Source和target经过ResNet50的baseline c) 输出有两个branches:
一个是cross-entropy loss for classification
一个是triplet loss for similarity learning:source的anchor image,利用source label构建正样本对,然后再选择target image形成负样本对。
文章的Hetero-Homogeneous Learning 在训练阶段,每个batch包含了labeled source images,unlabeled real target images以及对应的camStyle生成的fake target images。这样,前二者是学习两个domain之间的关系,后两者学习到了target domain中的camera invariances。
The third category of methods attempts on optimizing the neural networks with soft labels for target-domain samples by computing the similarities with reference images or features.
第三类方法尝试通过计算与参考图像或特征的相似性来优化目标域样本的软标签神经网络** (开始提出soft labels的思想)
7. ENC (Zhong et al., 2019) assigned soft labels by saving averaged features with an exemplar memory module. 以存储器的方式保存平均特征从而获得软标签(这篇文章我会单独写一篇总结) 文章名字:Exemplar Memory for Domain Adaptive Person Re-identification 论文连接https://arxiv.org/abs/1904.01990 代码:https://github.com/zhunzhong07/ECN
8. MAR (Yu et al., 2019) conducted multiple soft-label learning by comparing with a set of reference persons. However, the reference images and features might not be representative enough to generate accurate labels for achieving advanced performances. 进行多次软标签学习与一组参照人比较。然而,参考图像和特征可能没有足够的代表性来生成精确的标签来实现高级性能。(这篇文章我会单独写一篇总结) 文章名字:Unsupervised Person Re-identification by Soft Multilabel Learning 原文链接:https://arxiv.org/abs/1903.06325
Generic domain adaptation methods for close-set recognition.
Generic domain adaptation methods learn features that can minimize the differences between data distributions of source and target domains.
- Adversarial learning based methods (Zhang et al., 2018a; Tzeng et al., 2017; Ghifary et al., 2016; Bousmalis et al., 2016; Tzeng et al., 2015) adopted a domain classifier to dispel the discriminative domain information from the learned features in order to reduce the domain gap.
- There also exist methods (Tzeng et al., 2014; Long et al., 2015; Yan et al., 2017; Saito et al., 2018; Ghifary et al., 2016) that minimize the Maximum Mean Discrepancy (MMD) loss between source- and target-domain distributions. However, these methods assume that the classes on different domains are shared, which is not suitable for unsupervised domain adaptation on person re-ID.
Teacher-student models
Teacher-student models have been widely studied in semi-supervised learning methods and knowledge/model distillation methods. The key idea of teacher-student models is to create consistent training supervisions for labeled/unlabeled data via different models’ predictions.
- Temporal ensembling (Laine & Aila, 2016) maintained an exponential moving average prediction for each sample as the supervisions of the unlabeled samples, while the mean-teacher model (Tarvainen & Valpola,2017) averaged model weights at different training iterations to create the supervisions for unlabeled samples.
- Deep mutual learning (Zhang et al., 2018b) adopted a pool of student models instead of the teacher models by training them with supervisions from each other. However, existing methods with teacher-student mechanisms are mostly designed for close-set recognition problems, where both labeled and unlabeled data share the same set of class labels and could not be directly utilized on unsupervised domain adaptation tasks of person re-ID.
|