Table of Contents
初始
初识机器学习
python机器学习的生态圈
第一个机器学习项目
import numpy as np
import matplotlib.pyplot as plt
from pandas.plotting import scatter_matrix
import pandas as pd
机器学习中的hello world项目
(1)导入数据 (2)概述数据 (3)数据可视化 (4)评估算法 (5)实施预测
from sklearn.model_selection import train_test_split
from sklearn.model_selection import KFold
from sklearn.model_selection import cross_val_score
from sklearn.metrics import classification_report
from sklearn.metrics import confusion_matrix
from sklearn.metrics import accuracy_score
from sklearn.linear_model import LogisticRegression
from sklearn.tree import DecisionTreeClassifier
from sklearn.discriminant_analysis import LinearDiscriminantAnalysis
from sklearn.neighbors import KNeighborsClassifier
from sklearn.naive_bayes import GaussianNB
from sklearn.svm import SVC
导入数据
filename=r'iris.data'
names=['separ-length','separ-width','petal-length','petal-width','class']
dataset=pd.read_table(filename,names=names,sep=',')
dataset
| separ-length | separ-width | petal-length | petal-width | class |
---|
0 | 5.1 | 3.5 | 1.4 | 0.2 | Iris-setosa |
---|
1 | 4.9 | 3.0 | 1.4 | 0.2 | Iris-setosa |
---|
2 | 4.7 | 3.2 | 1.3 | 0.2 | Iris-setosa |
---|
3 | 4.6 | 3.1 | 1.5 | 0.2 | Iris-setosa |
---|
4 | 5.0 | 3.6 | 1.4 | 0.2 | Iris-setosa |
---|
... | ... | ... | ... | ... | ... |
---|
145 | 6.7 | 3.0 | 5.2 | 2.3 | Iris-virginica |
---|
146 | 6.3 | 2.5 | 5.0 | 1.9 | Iris-virginica |
---|
147 | 6.5 | 3.0 | 5.2 | 2.0 | Iris-virginica |
---|
148 | 6.2 | 3.4 | 5.4 | 2.3 | Iris-virginica |
---|
149 | 5.9 | 3.0 | 5.1 | 1.8 | Iris-virginica |
---|
150 rows × 5 columns
概述数据
dataset.skew()
separ-length 0.314911
separ-width 0.334053
petal-length -0.274464
petal-width -0.104997
dtype: float64
dataset.hist()
array([[<AxesSubplot:title={'center':'separ-length'}>,
<AxesSubplot:title={'center':'separ-width'}>],
[<AxesSubplot:title={'center':'petal-length'}>,
<AxesSubplot:title={'center':'petal-width'}>]], dtype=object)
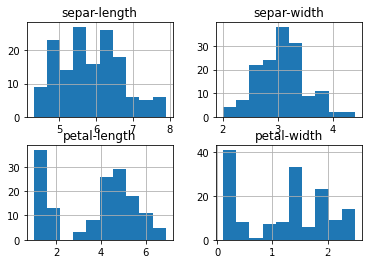
dataset.plot(kind='density',subplots=True,layout=(2,2))
array([[<AxesSubplot:ylabel='Density'>, <AxesSubplot:ylabel='Density'>],
[<AxesSubplot:ylabel='Density'>, <AxesSubplot:ylabel='Density'>]],
dtype=object)
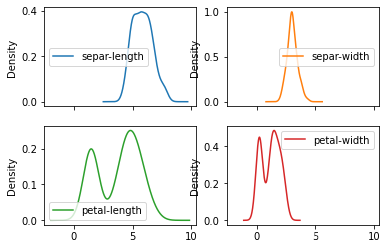
dataset.shape
(150, 5)
dataset.head(10)
| separ-length | separ-width | petal-length | petal-width | class |
---|
0 | 5.1 | 3.5 | 1.4 | 0.2 | Iris-setosa |
---|
1 | 4.9 | 3.0 | 1.4 | 0.2 | Iris-setosa |
---|
2 | 4.7 | 3.2 | 1.3 | 0.2 | Iris-setosa |
---|
3 | 4.6 | 3.1 | 1.5 | 0.2 | Iris-setosa |
---|
4 | 5.0 | 3.6 | 1.4 | 0.2 | Iris-setosa |
---|
5 | 5.4 | 3.9 | 1.7 | 0.4 | Iris-setosa |
---|
6 | 4.6 | 3.4 | 1.4 | 0.3 | Iris-setosa |
---|
7 | 5.0 | 3.4 | 1.5 | 0.2 | Iris-setosa |
---|
8 | 4.4 | 2.9 | 1.4 | 0.2 | Iris-setosa |
---|
9 | 4.9 | 3.1 | 1.5 | 0.1 | Iris-setosa |
---|
dataset.describe()
| separ-length | separ-width | petal-length | petal-width |
---|
count | 150.000000 | 150.000000 | 150.000000 | 150.000000 |
---|
mean | 5.843333 | 3.054000 | 3.758667 | 1.198667 |
---|
std | 0.828066 | 0.433594 | 1.764420 | 0.763161 |
---|
min | 4.300000 | 2.000000 | 1.000000 | 0.100000 |
---|
25% | 5.100000 | 2.800000 | 1.600000 | 0.300000 |
---|
50% | 5.800000 | 3.000000 | 4.350000 | 1.300000 |
---|
75% | 6.400000 | 3.300000 | 5.100000 | 1.800000 |
---|
max | 7.900000 | 4.400000 | 6.900000 | 2.500000 |
---|
dataset.groupby('class').count()
| separ-length | separ-width | petal-length | petal-width |
---|
class | | | | |
---|
Iris-setosa | 50 | 50 | 50 | 50 |
---|
Iris-versicolor | 50 | 50 | 50 | 50 |
---|
Iris-virginica | 50 | 50 | 50 | 50 |
---|
数据可视化
plt.style.use('seaborn-notebook')
dataset.plot(kind='box',subplots=True,layout=(2,2),sharex=False,sharey=False)
separ-length AxesSubplot(0.125,0.536818;0.352273x0.343182)
separ-width AxesSubplot(0.547727,0.536818;0.352273x0.343182)
petal-length AxesSubplot(0.125,0.125;0.352273x0.343182)
petal-width AxesSubplot(0.547727,0.125;0.352273x0.343182)
dtype: object
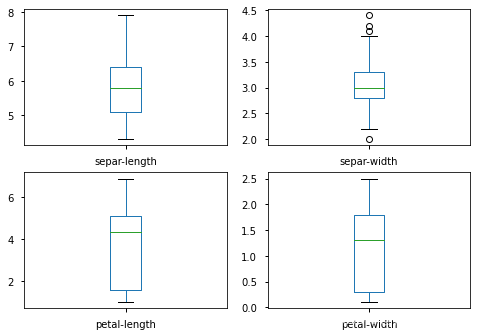
dataset.hist()
array([[<AxesSubplot:title={'center':'separ-length'}>,
<AxesSubplot:title={'center':'separ-width'}>],
[<AxesSubplot:title={'center':'petal-length'}>,
<AxesSubplot:title={'center':'petal-width'}>]], dtype=object)
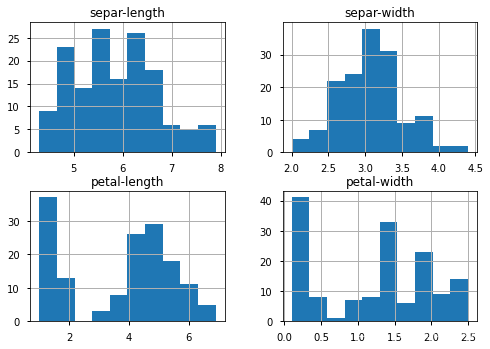
pd.plotting.scatter_matrix(dataset)
array([[<AxesSubplot:xlabel='separ-length', ylabel='separ-length'>,
<AxesSubplot:xlabel='separ-width', ylabel='separ-length'>,
<AxesSubplot:xlabel='petal-length', ylabel='separ-length'>,
<AxesSubplot:xlabel='petal-width', ylabel='separ-length'>],
[<AxesSubplot:xlabel='separ-length', ylabel='separ-width'>,
<AxesSubplot:xlabel='separ-width', ylabel='separ-width'>,
<AxesSubplot:xlabel='petal-length', ylabel='separ-width'>,
<AxesSubplot:xlabel='petal-width', ylabel='separ-width'>],
[<AxesSubplot:xlabel='separ-length', ylabel='petal-length'>,
<AxesSubplot:xlabel='separ-width', ylabel='petal-length'>,
<AxesSubplot:xlabel='petal-length', ylabel='petal-length'>,
<AxesSubplot:xlabel='petal-width', ylabel='petal-length'>],
[<AxesSubplot:xlabel='separ-length', ylabel='petal-width'>,
<AxesSubplot:xlabel='separ-width', ylabel='petal-width'>,
<AxesSubplot:xlabel='petal-length', ylabel='petal-width'>,
<AxesSubplot:xlabel='petal-width', ylabel='petal-width'>]],
dtype=object)
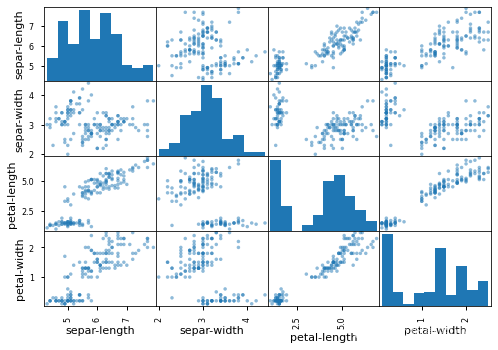
评估算法
(1)分离出评估数据集 (2)采用10折交叉验证来评估算法模型 (3)生成6个不同的模型来预测新数据 (4)选择最优模型
分离评估数据集
X=np.array(dataset.iloc[:,0:4])
Y=np.array(dataset.iloc[:,4])
validation_size=0.2
seed=7
X_train,X_test,Y_train,Y_test=train_test_split(X,Y,test_size=validation_size,random_state=seed)
创建模型
models={}
models['LR']=LogisticRegression(max_iter=1000)
models['LDA']=LinearDiscriminantAnalysis()
models['KNN']=KNeighborsClassifier()
models['CART']=DecisionTreeClassifier()
models['NB']=GaussianNB()
models['SVM']=SVC()
results=[]
for key in models:
kfold=KFold(n_splits=10,random_state=seed,shuffle=True)
cv_results=cross_val_score(models[key],X_train,Y_train,cv=kfold,scoring='accuracy')
results.append(cv_results)
print('%s:%f(%f)' %(key,cv_results.mean(),cv_results.std()))
LR:0.983333(0.033333)
LDA:0.975000(0.038188)
KNN:0.983333(0.033333)
CART:0.958333(0.076830)
NB:0.966667(0.040825)
SVM:0.983333(0.033333)
选择最优模型
plt.boxplot(results)
plt.xticks([i+1 for i in range(6)],models.keys())
([<matplotlib.axis.XTick at 0x17d53183250>,
<matplotlib.axis.XTick at 0x17d53183f40>,
<matplotlib.axis.XTick at 0x17d531aad00>,
<matplotlib.axis.XTick at 0x17d53644b80>,
<matplotlib.axis.XTick at 0x17d5364e0d0>,
<matplotlib.axis.XTick at 0x17d536448e0>],
[Text(1, 0, 'LR'),
Text(2, 0, 'LDA'),
Text(3, 0, 'KNN'),
Text(4, 0, 'CART'),
Text(5, 0, 'NB'),
Text(6, 0, 'SVM')])
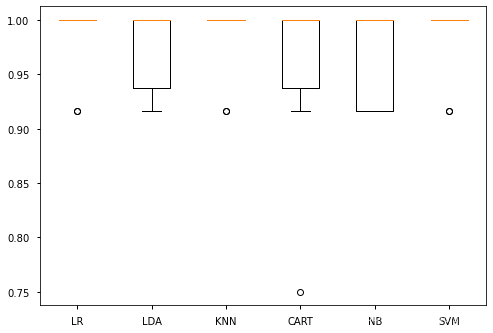
实施预测
svm=SVC()
svm.fit(X=X_train,y=Y_train)
pred=svm.predict(X_test)
accuracy_score(Y_test,pred)
0.8666666666666667
confusion_matrix(Y_test,pred)
array([[ 7, 0, 0],
[ 0, 10, 2],
[ 0, 2, 9]], dtype=int64)
print(classification_report(Y_test,pred))
precision recall f1-score support
Iris-setosa 1.00 1.00 1.00 7
Iris-versicolor 0.83 0.83 0.83 12
Iris-virginica 0.82 0.82 0.82 11
accuracy 0.87 30
macro avg 0.88 0.88 0.88 30
weighted avg 0.87 0.87 0.87 30
数据准备
数据预处理
调整数据尺度
from sklearn import datasets
iris=datasets.load_iris()
from sklearn.preprocessing import MinMaxScaler
transformer=MinMaxScaler(feature_range=(0,1))
newX=transformer.fit_transform(iris.data)
newX
array([[0.22222222, 0.625 , 0.06779661, 0.04166667],
[0.16666667, 0.41666667, 0.06779661, 0.04166667],
[0.11111111, 0.5 , 0.05084746, 0.04166667],
[0.08333333, 0.45833333, 0.08474576, 0.04166667],
[0.19444444, 0.66666667, 0.06779661, 0.04166667],
[0.30555556, 0.79166667, 0.11864407, 0.125 ],
[0.08333333, 0.58333333, 0.06779661, 0.08333333],
[0.19444444, 0.58333333, 0.08474576, 0.04166667],
[0.02777778, 0.375 , 0.06779661, 0.04166667],
[0.16666667, 0.45833333, 0.08474576, 0. ],
[0.30555556, 0.70833333, 0.08474576, 0.04166667],
[0.13888889, 0.58333333, 0.10169492, 0.04166667],
[0.13888889, 0.41666667, 0.06779661, 0. ],
[0. , 0.41666667, 0.01694915, 0. ],
[0.41666667, 0.83333333, 0.03389831, 0.04166667],
[0.38888889, 1. , 0.08474576, 0.125 ],
[0.30555556, 0.79166667, 0.05084746, 0.125 ],
[0.22222222, 0.625 , 0.06779661, 0.08333333],
[0.38888889, 0.75 , 0.11864407, 0.08333333],
[0.22222222, 0.75 , 0.08474576, 0.08333333],
[0.30555556, 0.58333333, 0.11864407, 0.04166667],
[0.22222222, 0.70833333, 0.08474576, 0.125 ],
[0.08333333, 0.66666667, 0. , 0.04166667],
[0.22222222, 0.54166667, 0.11864407, 0.16666667],
[0.13888889, 0.58333333, 0.15254237, 0.04166667],
[0.19444444, 0.41666667, 0.10169492, 0.04166667],
[0.19444444, 0.58333333, 0.10169492, 0.125 ],
[0.25 , 0.625 , 0.08474576, 0.04166667],
[0.25 , 0.58333333, 0.06779661, 0.04166667],
[0.11111111, 0.5 , 0.10169492, 0.04166667],
[0.13888889, 0.45833333, 0.10169492, 0.04166667],
[0.30555556, 0.58333333, 0.08474576, 0.125 ],
[0.25 , 0.875 , 0.08474576, 0. ],
[0.33333333, 0.91666667, 0.06779661, 0.04166667],
[0.16666667, 0.45833333, 0.08474576, 0.04166667],
[0.19444444, 0.5 , 0.03389831, 0.04166667],
[0.33333333, 0.625 , 0.05084746, 0.04166667],
[0.16666667, 0.66666667, 0.06779661, 0. ],
[0.02777778, 0.41666667, 0.05084746, 0.04166667],
[0.22222222, 0.58333333, 0.08474576, 0.04166667],
[0.19444444, 0.625 , 0.05084746, 0.08333333],
[0.05555556, 0.125 , 0.05084746, 0.08333333],
[0.02777778, 0.5 , 0.05084746, 0.04166667],
[0.19444444, 0.625 , 0.10169492, 0.20833333],
[0.22222222, 0.75 , 0.15254237, 0.125 ],
[0.13888889, 0.41666667, 0.06779661, 0.08333333],
[0.22222222, 0.75 , 0.10169492, 0.04166667],
[0.08333333, 0.5 , 0.06779661, 0.04166667],
[0.27777778, 0.70833333, 0.08474576, 0.04166667],
[0.19444444, 0.54166667, 0.06779661, 0.04166667],
[0.75 , 0.5 , 0.62711864, 0.54166667],
[0.58333333, 0.5 , 0.59322034, 0.58333333],
[0.72222222, 0.45833333, 0.66101695, 0.58333333],
[0.33333333, 0.125 , 0.50847458, 0.5 ],
[0.61111111, 0.33333333, 0.61016949, 0.58333333],
[0.38888889, 0.33333333, 0.59322034, 0.5 ],
[0.55555556, 0.54166667, 0.62711864, 0.625 ],
[0.16666667, 0.16666667, 0.38983051, 0.375 ],
[0.63888889, 0.375 , 0.61016949, 0.5 ],
[0.25 , 0.29166667, 0.49152542, 0.54166667],
[0.19444444, 0. , 0.42372881, 0.375 ],
[0.44444444, 0.41666667, 0.54237288, 0.58333333],
[0.47222222, 0.08333333, 0.50847458, 0.375 ],
[0.5 , 0.375 , 0.62711864, 0.54166667],
[0.36111111, 0.375 , 0.44067797, 0.5 ],
[0.66666667, 0.45833333, 0.57627119, 0.54166667],
[0.36111111, 0.41666667, 0.59322034, 0.58333333],
[0.41666667, 0.29166667, 0.52542373, 0.375 ],
[0.52777778, 0.08333333, 0.59322034, 0.58333333],
[0.36111111, 0.20833333, 0.49152542, 0.41666667],
[0.44444444, 0.5 , 0.6440678 , 0.70833333],
[0.5 , 0.33333333, 0.50847458, 0.5 ],
[0.55555556, 0.20833333, 0.66101695, 0.58333333],
[0.5 , 0.33333333, 0.62711864, 0.45833333],
[0.58333333, 0.375 , 0.55932203, 0.5 ],
[0.63888889, 0.41666667, 0.57627119, 0.54166667],
[0.69444444, 0.33333333, 0.6440678 , 0.54166667],
[0.66666667, 0.41666667, 0.6779661 , 0.66666667],
[0.47222222, 0.375 , 0.59322034, 0.58333333],
[0.38888889, 0.25 , 0.42372881, 0.375 ],
[0.33333333, 0.16666667, 0.47457627, 0.41666667],
[0.33333333, 0.16666667, 0.45762712, 0.375 ],
[0.41666667, 0.29166667, 0.49152542, 0.45833333],
[0.47222222, 0.29166667, 0.69491525, 0.625 ],
[0.30555556, 0.41666667, 0.59322034, 0.58333333],
[0.47222222, 0.58333333, 0.59322034, 0.625 ],
[0.66666667, 0.45833333, 0.62711864, 0.58333333],
[0.55555556, 0.125 , 0.57627119, 0.5 ],
[0.36111111, 0.41666667, 0.52542373, 0.5 ],
[0.33333333, 0.20833333, 0.50847458, 0.5 ],
[0.33333333, 0.25 , 0.57627119, 0.45833333],
[0.5 , 0.41666667, 0.61016949, 0.54166667],
[0.41666667, 0.25 , 0.50847458, 0.45833333],
[0.19444444, 0.125 , 0.38983051, 0.375 ],
[0.36111111, 0.29166667, 0.54237288, 0.5 ],
[0.38888889, 0.41666667, 0.54237288, 0.45833333],
[0.38888889, 0.375 , 0.54237288, 0.5 ],
[0.52777778, 0.375 , 0.55932203, 0.5 ],
[0.22222222, 0.20833333, 0.33898305, 0.41666667],
[0.38888889, 0.33333333, 0.52542373, 0.5 ],
[0.55555556, 0.54166667, 0.84745763, 1. ],
[0.41666667, 0.29166667, 0.69491525, 0.75 ],
[0.77777778, 0.41666667, 0.83050847, 0.83333333],
[0.55555556, 0.375 , 0.77966102, 0.70833333],
[0.61111111, 0.41666667, 0.81355932, 0.875 ],
[0.91666667, 0.41666667, 0.94915254, 0.83333333],
[0.16666667, 0.20833333, 0.59322034, 0.66666667],
[0.83333333, 0.375 , 0.89830508, 0.70833333],
[0.66666667, 0.20833333, 0.81355932, 0.70833333],
[0.80555556, 0.66666667, 0.86440678, 1. ],
[0.61111111, 0.5 , 0.69491525, 0.79166667],
[0.58333333, 0.29166667, 0.72881356, 0.75 ],
[0.69444444, 0.41666667, 0.76271186, 0.83333333],
[0.38888889, 0.20833333, 0.6779661 , 0.79166667],
[0.41666667, 0.33333333, 0.69491525, 0.95833333],
[0.58333333, 0.5 , 0.72881356, 0.91666667],
[0.61111111, 0.41666667, 0.76271186, 0.70833333],
[0.94444444, 0.75 , 0.96610169, 0.875 ],
[0.94444444, 0.25 , 1. , 0.91666667],
[0.47222222, 0.08333333, 0.6779661 , 0.58333333],
[0.72222222, 0.5 , 0.79661017, 0.91666667],
[0.36111111, 0.33333333, 0.66101695, 0.79166667],
[0.94444444, 0.33333333, 0.96610169, 0.79166667],
[0.55555556, 0.29166667, 0.66101695, 0.70833333],
[0.66666667, 0.54166667, 0.79661017, 0.83333333],
[0.80555556, 0.5 , 0.84745763, 0.70833333],
[0.52777778, 0.33333333, 0.6440678 , 0.70833333],
[0.5 , 0.41666667, 0.66101695, 0.70833333],
[0.58333333, 0.33333333, 0.77966102, 0.83333333],
[0.80555556, 0.41666667, 0.81355932, 0.625 ],
[0.86111111, 0.33333333, 0.86440678, 0.75 ],
[1. , 0.75 , 0.91525424, 0.79166667],
[0.58333333, 0.33333333, 0.77966102, 0.875 ],
[0.55555556, 0.33333333, 0.69491525, 0.58333333],
[0.5 , 0.25 , 0.77966102, 0.54166667],
[0.94444444, 0.41666667, 0.86440678, 0.91666667],
[0.55555556, 0.58333333, 0.77966102, 0.95833333],
[0.58333333, 0.45833333, 0.76271186, 0.70833333],
[0.47222222, 0.41666667, 0.6440678 , 0.70833333],
[0.72222222, 0.45833333, 0.74576271, 0.83333333],
[0.66666667, 0.45833333, 0.77966102, 0.95833333],
[0.72222222, 0.45833333, 0.69491525, 0.91666667],
[0.41666667, 0.29166667, 0.69491525, 0.75 ],
[0.69444444, 0.5 , 0.83050847, 0.91666667],
[0.66666667, 0.54166667, 0.79661017, 1. ],
[0.66666667, 0.41666667, 0.71186441, 0.91666667],
[0.55555556, 0.20833333, 0.6779661 , 0.75 ],
[0.61111111, 0.41666667, 0.71186441, 0.79166667],
[0.52777778, 0.58333333, 0.74576271, 0.91666667],
[0.44444444, 0.41666667, 0.69491525, 0.70833333]])
正态化数据
from sklearn.preprocessing import StandardScaler
transformer=StandardScaler()
newX=transformer.fit_transform(iris.data)
newX
array([[-9.00681170e-01, 1.01900435e+00, -1.34022653e+00,
-1.31544430e+00],
[-1.14301691e+00, -1.31979479e-01, -1.34022653e+00,
-1.31544430e+00],
[-1.38535265e+00, 3.28414053e-01, -1.39706395e+00,
-1.31544430e+00],
[-1.50652052e+00, 9.82172869e-02, -1.28338910e+00,
-1.31544430e+00],
[-1.02184904e+00, 1.24920112e+00, -1.34022653e+00,
-1.31544430e+00],
[-5.37177559e-01, 1.93979142e+00, -1.16971425e+00,
-1.05217993e+00],
[-1.50652052e+00, 7.88807586e-01, -1.34022653e+00,
-1.18381211e+00],
[-1.02184904e+00, 7.88807586e-01, -1.28338910e+00,
-1.31544430e+00],
[-1.74885626e+00, -3.62176246e-01, -1.34022653e+00,
-1.31544430e+00],
[-1.14301691e+00, 9.82172869e-02, -1.28338910e+00,
-1.44707648e+00],
[-5.37177559e-01, 1.47939788e+00, -1.28338910e+00,
-1.31544430e+00],
[-1.26418478e+00, 7.88807586e-01, -1.22655167e+00,
-1.31544430e+00],
[-1.26418478e+00, -1.31979479e-01, -1.34022653e+00,
-1.44707648e+00],
[-1.87002413e+00, -1.31979479e-01, -1.51073881e+00,
-1.44707648e+00],
[-5.25060772e-02, 2.16998818e+00, -1.45390138e+00,
-1.31544430e+00],
[-1.73673948e-01, 3.09077525e+00, -1.28338910e+00,
-1.05217993e+00],
[-5.37177559e-01, 1.93979142e+00, -1.39706395e+00,
-1.05217993e+00],
[-9.00681170e-01, 1.01900435e+00, -1.34022653e+00,
-1.18381211e+00],
[-1.73673948e-01, 1.70959465e+00, -1.16971425e+00,
-1.18381211e+00],
[-9.00681170e-01, 1.70959465e+00, -1.28338910e+00,
-1.18381211e+00],
[-5.37177559e-01, 7.88807586e-01, -1.16971425e+00,
-1.31544430e+00],
[-9.00681170e-01, 1.47939788e+00, -1.28338910e+00,
-1.05217993e+00],
[-1.50652052e+00, 1.24920112e+00, -1.56757623e+00,
-1.31544430e+00],
[-9.00681170e-01, 5.58610819e-01, -1.16971425e+00,
-9.20547742e-01],
[-1.26418478e+00, 7.88807586e-01, -1.05603939e+00,
-1.31544430e+00],
[-1.02184904e+00, -1.31979479e-01, -1.22655167e+00,
-1.31544430e+00],
[-1.02184904e+00, 7.88807586e-01, -1.22655167e+00,
-1.05217993e+00],
[-7.79513300e-01, 1.01900435e+00, -1.28338910e+00,
-1.31544430e+00],
[-7.79513300e-01, 7.88807586e-01, -1.34022653e+00,
-1.31544430e+00],
[-1.38535265e+00, 3.28414053e-01, -1.22655167e+00,
-1.31544430e+00],
[-1.26418478e+00, 9.82172869e-02, -1.22655167e+00,
-1.31544430e+00],
[-5.37177559e-01, 7.88807586e-01, -1.28338910e+00,
-1.05217993e+00],
[-7.79513300e-01, 2.40018495e+00, -1.28338910e+00,
-1.44707648e+00],
[-4.16009689e-01, 2.63038172e+00, -1.34022653e+00,
-1.31544430e+00],
[-1.14301691e+00, 9.82172869e-02, -1.28338910e+00,
-1.31544430e+00],
[-1.02184904e+00, 3.28414053e-01, -1.45390138e+00,
-1.31544430e+00],
[-4.16009689e-01, 1.01900435e+00, -1.39706395e+00,
-1.31544430e+00],
[-1.14301691e+00, 1.24920112e+00, -1.34022653e+00,
-1.44707648e+00],
[-1.74885626e+00, -1.31979479e-01, -1.39706395e+00,
-1.31544430e+00],
[-9.00681170e-01, 7.88807586e-01, -1.28338910e+00,
-1.31544430e+00],
[-1.02184904e+00, 1.01900435e+00, -1.39706395e+00,
-1.18381211e+00],
[-1.62768839e+00, -1.74335684e+00, -1.39706395e+00,
-1.18381211e+00],
[-1.74885626e+00, 3.28414053e-01, -1.39706395e+00,
-1.31544430e+00],
[-1.02184904e+00, 1.01900435e+00, -1.22655167e+00,
-7.88915558e-01],
[-9.00681170e-01, 1.70959465e+00, -1.05603939e+00,
-1.05217993e+00],
[-1.26418478e+00, -1.31979479e-01, -1.34022653e+00,
-1.18381211e+00],
[-9.00681170e-01, 1.70959465e+00, -1.22655167e+00,
-1.31544430e+00],
[-1.50652052e+00, 3.28414053e-01, -1.34022653e+00,
-1.31544430e+00],
[-6.58345429e-01, 1.47939788e+00, -1.28338910e+00,
-1.31544430e+00],
[-1.02184904e+00, 5.58610819e-01, -1.34022653e+00,
-1.31544430e+00],
[ 1.40150837e+00, 3.28414053e-01, 5.35408562e-01,
2.64141916e-01],
[ 6.74501145e-01, 3.28414053e-01, 4.21733708e-01,
3.95774101e-01],
[ 1.28034050e+00, 9.82172869e-02, 6.49083415e-01,
3.95774101e-01],
[-4.16009689e-01, -1.74335684e+00, 1.37546573e-01,
1.32509732e-01],
[ 7.95669016e-01, -5.92373012e-01, 4.78571135e-01,
3.95774101e-01],
[-1.73673948e-01, -5.92373012e-01, 4.21733708e-01,
1.32509732e-01],
[ 5.53333275e-01, 5.58610819e-01, 5.35408562e-01,
5.27406285e-01],
[-1.14301691e+00, -1.51316008e+00, -2.60315415e-01,
-2.62386821e-01],
[ 9.16836886e-01, -3.62176246e-01, 4.78571135e-01,
1.32509732e-01],
[-7.79513300e-01, -8.22569778e-01, 8.07091462e-02,
2.64141916e-01],
[-1.02184904e+00, -2.43394714e+00, -1.46640561e-01,
-2.62386821e-01],
[ 6.86617933e-02, -1.31979479e-01, 2.51221427e-01,
3.95774101e-01],
[ 1.89829664e-01, -1.97355361e+00, 1.37546573e-01,
-2.62386821e-01],
[ 3.10997534e-01, -3.62176246e-01, 5.35408562e-01,
2.64141916e-01],
[-2.94841818e-01, -3.62176246e-01, -8.98031345e-02,
1.32509732e-01],
[ 1.03800476e+00, 9.82172869e-02, 3.64896281e-01,
2.64141916e-01],
[-2.94841818e-01, -1.31979479e-01, 4.21733708e-01,
3.95774101e-01],
[-5.25060772e-02, -8.22569778e-01, 1.94384000e-01,
-2.62386821e-01],
[ 4.32165405e-01, -1.97355361e+00, 4.21733708e-01,
3.95774101e-01],
[-2.94841818e-01, -1.28296331e+00, 8.07091462e-02,
-1.30754636e-01],
[ 6.86617933e-02, 3.28414053e-01, 5.92245988e-01,
7.90670654e-01],
[ 3.10997534e-01, -5.92373012e-01, 1.37546573e-01,
1.32509732e-01],
[ 5.53333275e-01, -1.28296331e+00, 6.49083415e-01,
3.95774101e-01],
[ 3.10997534e-01, -5.92373012e-01, 5.35408562e-01,
8.77547895e-04],
[ 6.74501145e-01, -3.62176246e-01, 3.08058854e-01,
1.32509732e-01],
[ 9.16836886e-01, -1.31979479e-01, 3.64896281e-01,
2.64141916e-01],
[ 1.15917263e+00, -5.92373012e-01, 5.92245988e-01,
2.64141916e-01],
[ 1.03800476e+00, -1.31979479e-01, 7.05920842e-01,
6.59038469e-01],
[ 1.89829664e-01, -3.62176246e-01, 4.21733708e-01,
3.95774101e-01],
[-1.73673948e-01, -1.05276654e+00, -1.46640561e-01,
-2.62386821e-01],
[-4.16009689e-01, -1.51316008e+00, 2.38717193e-02,
-1.30754636e-01],
[-4.16009689e-01, -1.51316008e+00, -3.29657076e-02,
-2.62386821e-01],
[-5.25060772e-02, -8.22569778e-01, 8.07091462e-02,
8.77547895e-04],
[ 1.89829664e-01, -8.22569778e-01, 7.62758269e-01,
5.27406285e-01],
[-5.37177559e-01, -1.31979479e-01, 4.21733708e-01,
3.95774101e-01],
[ 1.89829664e-01, 7.88807586e-01, 4.21733708e-01,
5.27406285e-01],
[ 1.03800476e+00, 9.82172869e-02, 5.35408562e-01,
3.95774101e-01],
[ 5.53333275e-01, -1.74335684e+00, 3.64896281e-01,
1.32509732e-01],
[-2.94841818e-01, -1.31979479e-01, 1.94384000e-01,
1.32509732e-01],
[-4.16009689e-01, -1.28296331e+00, 1.37546573e-01,
1.32509732e-01],
[-4.16009689e-01, -1.05276654e+00, 3.64896281e-01,
8.77547895e-04],
[ 3.10997534e-01, -1.31979479e-01, 4.78571135e-01,
2.64141916e-01],
[-5.25060772e-02, -1.05276654e+00, 1.37546573e-01,
8.77547895e-04],
[-1.02184904e+00, -1.74335684e+00, -2.60315415e-01,
-2.62386821e-01],
[-2.94841818e-01, -8.22569778e-01, 2.51221427e-01,
1.32509732e-01],
[-1.73673948e-01, -1.31979479e-01, 2.51221427e-01,
8.77547895e-04],
[-1.73673948e-01, -3.62176246e-01, 2.51221427e-01,
1.32509732e-01],
[ 4.32165405e-01, -3.62176246e-01, 3.08058854e-01,
1.32509732e-01],
[-9.00681170e-01, -1.28296331e+00, -4.30827696e-01,
-1.30754636e-01],
[-1.73673948e-01, -5.92373012e-01, 1.94384000e-01,
1.32509732e-01],
[ 5.53333275e-01, 5.58610819e-01, 1.27429511e+00,
1.71209594e+00],
[-5.25060772e-02, -8.22569778e-01, 7.62758269e-01,
9.22302838e-01],
[ 1.52267624e+00, -1.31979479e-01, 1.21745768e+00,
1.18556721e+00],
[ 5.53333275e-01, -3.62176246e-01, 1.04694540e+00,
7.90670654e-01],
[ 7.95669016e-01, -1.31979479e-01, 1.16062026e+00,
1.31719939e+00],
[ 2.12851559e+00, -1.31979479e-01, 1.61531967e+00,
1.18556721e+00],
[-1.14301691e+00, -1.28296331e+00, 4.21733708e-01,
6.59038469e-01],
[ 1.76501198e+00, -3.62176246e-01, 1.44480739e+00,
7.90670654e-01],
[ 1.03800476e+00, -1.28296331e+00, 1.16062026e+00,
7.90670654e-01],
[ 1.64384411e+00, 1.24920112e+00, 1.33113254e+00,
1.71209594e+00],
[ 7.95669016e-01, 3.28414053e-01, 7.62758269e-01,
1.05393502e+00],
[ 6.74501145e-01, -8.22569778e-01, 8.76433123e-01,
9.22302838e-01],
[ 1.15917263e+00, -1.31979479e-01, 9.90107977e-01,
1.18556721e+00],
[-1.73673948e-01, -1.28296331e+00, 7.05920842e-01,
1.05393502e+00],
[-5.25060772e-02, -5.92373012e-01, 7.62758269e-01,
1.58046376e+00],
[ 6.74501145e-01, 3.28414053e-01, 8.76433123e-01,
1.44883158e+00],
[ 7.95669016e-01, -1.31979479e-01, 9.90107977e-01,
7.90670654e-01],
[ 2.24968346e+00, 1.70959465e+00, 1.67215710e+00,
1.31719939e+00],
[ 2.24968346e+00, -1.05276654e+00, 1.78583195e+00,
1.44883158e+00],
[ 1.89829664e-01, -1.97355361e+00, 7.05920842e-01,
3.95774101e-01],
[ 1.28034050e+00, 3.28414053e-01, 1.10378283e+00,
1.44883158e+00],
[-2.94841818e-01, -5.92373012e-01, 6.49083415e-01,
1.05393502e+00],
[ 2.24968346e+00, -5.92373012e-01, 1.67215710e+00,
1.05393502e+00],
[ 5.53333275e-01, -8.22569778e-01, 6.49083415e-01,
7.90670654e-01],
[ 1.03800476e+00, 5.58610819e-01, 1.10378283e+00,
1.18556721e+00],
[ 1.64384411e+00, 3.28414053e-01, 1.27429511e+00,
7.90670654e-01],
[ 4.32165405e-01, -5.92373012e-01, 5.92245988e-01,
7.90670654e-01],
[ 3.10997534e-01, -1.31979479e-01, 6.49083415e-01,
7.90670654e-01],
[ 6.74501145e-01, -5.92373012e-01, 1.04694540e+00,
1.18556721e+00],
[ 1.64384411e+00, -1.31979479e-01, 1.16062026e+00,
5.27406285e-01],
[ 1.88617985e+00, -5.92373012e-01, 1.33113254e+00,
9.22302838e-01],
[ 2.49201920e+00, 1.70959465e+00, 1.50164482e+00,
1.05393502e+00],
[ 6.74501145e-01, -5.92373012e-01, 1.04694540e+00,
1.31719939e+00],
[ 5.53333275e-01, -5.92373012e-01, 7.62758269e-01,
3.95774101e-01],
[ 3.10997534e-01, -1.05276654e+00, 1.04694540e+00,
2.64141916e-01],
[ 2.24968346e+00, -1.31979479e-01, 1.33113254e+00,
1.44883158e+00],
[ 5.53333275e-01, 7.88807586e-01, 1.04694540e+00,
1.58046376e+00],
[ 6.74501145e-01, 9.82172869e-02, 9.90107977e-01,
7.90670654e-01],
[ 1.89829664e-01, -1.31979479e-01, 5.92245988e-01,
7.90670654e-01],
[ 1.28034050e+00, 9.82172869e-02, 9.33270550e-01,
1.18556721e+00],
[ 1.03800476e+00, 9.82172869e-02, 1.04694540e+00,
1.58046376e+00],
[ 1.28034050e+00, 9.82172869e-02, 7.62758269e-01,
1.44883158e+00],
[-5.25060772e-02, -8.22569778e-01, 7.62758269e-01,
9.22302838e-01],
[ 1.15917263e+00, 3.28414053e-01, 1.21745768e+00,
1.44883158e+00],
[ 1.03800476e+00, 5.58610819e-01, 1.10378283e+00,
1.71209594e+00],
[ 1.03800476e+00, -1.31979479e-01, 8.19595696e-01,
1.44883158e+00],
[ 5.53333275e-01, -1.28296331e+00, 7.05920842e-01,
9.22302838e-01],
[ 7.95669016e-01, -1.31979479e-01, 8.19595696e-01,
1.05393502e+00],
[ 4.32165405e-01, 7.88807586e-01, 9.33270550e-01,
1.44883158e+00],
[ 6.86617933e-02, -1.31979479e-01, 7.62758269e-01,
7.90670654e-01]])
标准化数据
from sklearn.preprocessing import Normalizer
transformer=Normalizer()
newX=transformer.fit_transform(iris.data)
newX
array([[0.80377277, 0.55160877, 0.22064351, 0.0315205 ],
[0.82813287, 0.50702013, 0.23660939, 0.03380134],
[0.80533308, 0.54831188, 0.2227517 , 0.03426949],
[0.80003025, 0.53915082, 0.26087943, 0.03478392],
[0.790965 , 0.5694948 , 0.2214702 , 0.0316386 ],
[0.78417499, 0.5663486 , 0.2468699 , 0.05808704],
[0.78010936, 0.57660257, 0.23742459, 0.0508767 ],
[0.80218492, 0.54548574, 0.24065548, 0.0320874 ],
[0.80642366, 0.5315065 , 0.25658935, 0.03665562],
[0.81803119, 0.51752994, 0.25041771, 0.01669451],
[0.80373519, 0.55070744, 0.22325977, 0.02976797],
[0.786991 , 0.55745196, 0.26233033, 0.03279129],
[0.82307218, 0.51442011, 0.24006272, 0.01714734],
[0.8025126 , 0.55989251, 0.20529392, 0.01866308],
[0.81120865, 0.55945424, 0.16783627, 0.02797271],
[0.77381111, 0.59732787, 0.2036345 , 0.05430253],
[0.79428944, 0.57365349, 0.19121783, 0.05883625],
[0.80327412, 0.55126656, 0.22050662, 0.04725142],
[0.8068282 , 0.53788547, 0.24063297, 0.04246464],
[0.77964883, 0.58091482, 0.22930848, 0.0458617 ],
[0.8173379 , 0.51462016, 0.25731008, 0.03027177],
[0.78591858, 0.57017622, 0.23115252, 0.06164067],
[0.77577075, 0.60712493, 0.16864581, 0.03372916],
[0.80597792, 0.52151512, 0.26865931, 0.07901744],
[0.776114 , 0.54974742, 0.30721179, 0.03233808],
[0.82647451, 0.4958847 , 0.26447184, 0.03305898],
[0.79778206, 0.5424918 , 0.25529026, 0.06382256],
[0.80641965, 0.54278246, 0.23262105, 0.03101614],
[0.81609427, 0.5336001 , 0.21971769, 0.03138824],
[0.79524064, 0.54144043, 0.27072022, 0.03384003],
[0.80846584, 0.52213419, 0.26948861, 0.03368608],
[0.82225028, 0.51771314, 0.22840286, 0.06090743],
[0.76578311, 0.60379053, 0.22089897, 0.0147266 ],
[0.77867447, 0.59462414, 0.19820805, 0.02831544],
[0.81768942, 0.51731371, 0.25031309, 0.03337508],
[0.82512295, 0.52807869, 0.19802951, 0.03300492],
[0.82699754, 0.52627116, 0.19547215, 0.03007264],
[0.78523221, 0.5769053 , 0.22435206, 0.01602515],
[0.80212413, 0.54690282, 0.23699122, 0.03646019],
[0.80779568, 0.53853046, 0.23758697, 0.03167826],
[0.80033301, 0.56023311, 0.20808658, 0.04801998],
[0.86093857, 0.44003527, 0.24871559, 0.0573959 ],
[0.78609038, 0.57170209, 0.23225397, 0.03573138],
[0.78889479, 0.55222635, 0.25244633, 0.09466737],
[0.76693897, 0.57144472, 0.28572236, 0.06015208],
[0.82210585, 0.51381615, 0.23978087, 0.05138162],
[0.77729093, 0.57915795, 0.24385598, 0.030482 ],
[0.79594782, 0.55370283, 0.24224499, 0.03460643],
[0.79837025, 0.55735281, 0.22595384, 0.03012718],
[0.81228363, 0.5361072 , 0.22743942, 0.03249135],
[0.76701103, 0.35063361, 0.51499312, 0.15340221],
[0.74549757, 0.37274878, 0.52417798, 0.17472599],
[0.75519285, 0.33928954, 0.53629637, 0.16417236],
[0.75384916, 0.31524601, 0.54825394, 0.17818253],
[0.7581754 , 0.32659863, 0.5365549 , 0.17496355],
[0.72232962, 0.35482858, 0.57026022, 0.16474184],
[0.72634846, 0.38046824, 0.54187901, 0.18446945],
[0.75916547, 0.37183615, 0.51127471, 0.15493173],
[0.76301853, 0.33526572, 0.53180079, 0.15029153],
[0.72460233, 0.37623583, 0.54345175, 0.19508524],
[0.76923077, 0.30769231, 0.53846154, 0.15384615],
[0.73923462, 0.37588201, 0.52623481, 0.187941 ],
[0.78892752, 0.28927343, 0.52595168, 0.13148792],
[0.73081412, 0.34743622, 0.56308629, 0.16772783],
[0.75911707, 0.3931142 , 0.48800383, 0.17622361],
[0.76945444, 0.35601624, 0.50531337, 0.16078153],
[0.70631892, 0.37838513, 0.5675777 , 0.18919257],
[0.75676497, 0.35228714, 0.53495455, 0.13047672],
[0.76444238, 0.27125375, 0.55483721, 0.18494574],
[0.76185188, 0.34011245, 0.53057542, 0.14964948],
[0.6985796 , 0.37889063, 0.56833595, 0.21312598],
[0.77011854, 0.35349703, 0.50499576, 0.16412362],
[0.74143307, 0.29421947, 0.57667016, 0.17653168],
[0.73659895, 0.33811099, 0.56754345, 0.14490471],
[0.76741698, 0.34773582, 0.51560829, 0.15588157],
[0.76785726, 0.34902603, 0.51190484, 0.16287881],
[0.76467269, 0.31486523, 0.53976896, 0.15743261],
[0.74088576, 0.33173989, 0.55289982, 0.18798594],
[0.73350949, 0.35452959, 0.55013212, 0.18337737],
[0.78667474, 0.35883409, 0.48304589, 0.13801311],
[0.76521855, 0.33391355, 0.52869645, 0.15304371],
[0.77242925, 0.33706004, 0.51963422, 0.14044168],
[0.76434981, 0.35581802, 0.51395936, 0.15814134],
[0.70779525, 0.31850786, 0.60162596, 0.1887454 ],
[0.69333409, 0.38518561, 0.57777841, 0.1925928 ],
[0.71524936, 0.40530797, 0.53643702, 0.19073316],
[0.75457341, 0.34913098, 0.52932761, 0.16893434],
[0.77530021, 0.28304611, 0.54147951, 0.15998258],
[0.72992443, 0.39103094, 0.53440896, 0.16944674],
[0.74714194, 0.33960997, 0.54337595, 0.17659719],
[0.72337118, 0.34195729, 0.57869695, 0.15782644],
[0.73260391, 0.36029701, 0.55245541, 0.1681386 ],
[0.76262994, 0.34186859, 0.52595168, 0.1577855 ],
[0.76986879, 0.35413965, 0.5081134 , 0.15397376],
[0.73544284, 0.35458851, 0.55158213, 0.1707278 ],
[0.73239618, 0.38547167, 0.53966034, 0.15418867],
[0.73446047, 0.37367287, 0.5411814 , 0.16750853],
[0.75728103, 0.3542121 , 0.52521104, 0.15878473],
[0.78258054, 0.38361791, 0.4603415 , 0.16879188],
[0.7431482 , 0.36505526, 0.5345452 , 0.16948994],
[0.65387747, 0.34250725, 0.62274045, 0.25947519],
[0.69052512, 0.32145135, 0.60718588, 0.22620651],
[0.71491405, 0.30207636, 0.59408351, 0.21145345],
[0.69276796, 0.31889319, 0.61579374, 0.1979337 ],
[0.68619022, 0.31670318, 0.61229281, 0.232249 ],
[0.70953708, 0.28008043, 0.61617694, 0.1960563 ],
[0.67054118, 0.34211284, 0.61580312, 0.23263673],
[0.71366557, 0.28351098, 0.61590317, 0.17597233],
[0.71414125, 0.26647062, 0.61821183, 0.19185884],
[0.69198788, 0.34599394, 0.58626751, 0.24027357],
[0.71562645, 0.3523084 , 0.56149152, 0.22019275],
[0.71576546, 0.30196356, 0.59274328, 0.21249287],
[0.71718148, 0.31640359, 0.58007326, 0.22148252],
[0.6925518 , 0.30375079, 0.60750157, 0.24300063],
[0.67767924, 0.32715549, 0.59589036, 0.28041899],
[0.69589887, 0.34794944, 0.57629125, 0.25008866],
[0.70610474, 0.3258945 , 0.59747324, 0.1955367 ],
[0.69299099, 0.34199555, 0.60299216, 0.19799743],
[0.70600618, 0.2383917 , 0.63265489, 0.21088496],
[0.72712585, 0.26661281, 0.60593821, 0.18178146],
[0.70558934, 0.32722984, 0.58287815, 0.23519645],
[0.68307923, 0.34153961, 0.59769433, 0.24395687],
[0.71486543, 0.25995106, 0.62202576, 0.18567933],
[0.73122464, 0.31338199, 0.56873028, 0.20892133],
[0.69595601, 0.3427843 , 0.59208198, 0.21813547],
[0.71529453, 0.31790868, 0.59607878, 0.17882363],
[0.72785195, 0.32870733, 0.56349829, 0.21131186],
[0.71171214, 0.35002236, 0.57170319, 0.21001342],
[0.69594002, 0.30447376, 0.60894751, 0.22835532],
[0.73089855, 0.30454106, 0.58877939, 0.1624219 ],
[0.72766159, 0.27533141, 0.59982915, 0.18683203],
[0.71578999, 0.34430405, 0.5798805 , 0.18121266],
[0.69417747, 0.30370264, 0.60740528, 0.2386235 ],
[0.72366005, 0.32162669, 0.58582004, 0.17230001],
[0.69385414, 0.29574111, 0.63698085, 0.15924521],
[0.73154399, 0.28501714, 0.57953485, 0.21851314],
[0.67017484, 0.36168166, 0.59571097, 0.2553047 ],
[0.69804799, 0.338117 , 0.59988499, 0.196326 ],
[0.71066905, 0.35533453, 0.56853524, 0.21320072],
[0.72415258, 0.32534391, 0.56672811, 0.22039426],
[0.69997037, 0.32386689, 0.58504986, 0.25073566],
[0.73337886, 0.32948905, 0.54206264, 0.24445962],
[0.69052512, 0.32145135, 0.60718588, 0.22620651],
[0.69193502, 0.32561648, 0.60035539, 0.23403685],
[0.68914871, 0.33943145, 0.58629069, 0.25714504],
[0.72155725, 0.32308533, 0.56001458, 0.24769876],
[0.72965359, 0.28954508, 0.57909015, 0.22005426],
[0.71653899, 0.3307103 , 0.57323119, 0.22047353],
[0.67467072, 0.36998072, 0.58761643, 0.25028107],
[0.69025916, 0.35097923, 0.5966647 , 0.21058754]])
二值数据
from sklearn.preprocessing import Binarizer
transformer=Binarizer(threshold=0.25)
newX=transformer.fit_transform(iris.data)
newX
array([[1., 1., 1., 0.],
[1., 1., 1., 0.],
[1., 1., 1., 0.],
[1., 1., 1., 0.],
[1., 1., 1., 0.],
[1., 1., 1., 1.],
[1., 1., 1., 1.],
[1., 1., 1., 0.],
[1., 1., 1., 0.],
[1., 1., 1., 0.],
[1., 1., 1., 0.],
[1., 1., 1., 0.],
[1., 1., 1., 0.],
[1., 1., 1., 0.],
[1., 1., 1., 0.],
[1., 1., 1., 1.],
[1., 1., 1., 1.],
[1., 1., 1., 1.],
[1., 1., 1., 1.],
[1., 1., 1., 1.],
[1., 1., 1., 0.],
[1., 1., 1., 1.],
[1., 1., 1., 0.],
[1., 1., 1., 1.],
[1., 1., 1., 0.],
[1., 1., 1., 0.],
[1., 1., 1., 1.],
[1., 1., 1., 0.],
[1., 1., 1., 0.],
[1., 1., 1., 0.],
[1., 1., 1., 0.],
[1., 1., 1., 1.],
[1., 1., 1., 0.],
[1., 1., 1., 0.],
[1., 1., 1., 0.],
[1., 1., 1., 0.],
[1., 1., 1., 0.],
[1., 1., 1., 0.],
[1., 1., 1., 0.],
[1., 1., 1., 0.],
[1., 1., 1., 1.],
[1., 1., 1., 1.],
[1., 1., 1., 0.],
[1., 1., 1., 1.],
[1., 1., 1., 1.],
[1., 1., 1., 1.],
[1., 1., 1., 0.],
[1., 1., 1., 0.],
[1., 1., 1., 0.],
[1., 1., 1., 0.],
[1., 1., 1., 1.],
[1., 1., 1., 1.],
[1., 1., 1., 1.],
[1., 1., 1., 1.],
[1., 1., 1., 1.],
[1., 1., 1., 1.],
[1., 1., 1., 1.],
[1., 1., 1., 1.],
[1., 1., 1., 1.],
[1., 1., 1., 1.],
[1., 1., 1., 1.],
[1., 1., 1., 1.],
[1., 1., 1., 1.],
[1., 1., 1., 1.],
[1., 1., 1., 1.],
[1., 1., 1., 1.],
[1., 1., 1., 1.],
[1., 1., 1., 1.],
[1., 1., 1., 1.],
[1., 1., 1., 1.],
[1., 1., 1., 1.],
[1., 1., 1., 1.],
[1., 1., 1., 1.],
[1., 1., 1., 1.],
[1., 1., 1., 1.],
[1., 1., 1., 1.],
[1., 1., 1., 1.],
[1., 1., 1., 1.],
[1., 1., 1., 1.],
[1., 1., 1., 1.],
[1., 1., 1., 1.],
[1., 1., 1., 1.],
[1., 1., 1., 1.],
[1., 1., 1., 1.],
[1., 1., 1., 1.],
[1., 1., 1., 1.],
[1., 1., 1., 1.],
[1., 1., 1., 1.],
[1., 1., 1., 1.],
[1., 1., 1., 1.],
[1., 1., 1., 1.],
[1., 1., 1., 1.],
[1., 1., 1., 1.],
[1., 1., 1., 1.],
[1., 1., 1., 1.],
[1., 1., 1., 1.],
[1., 1., 1., 1.],
[1., 1., 1., 1.],
[1., 1., 1., 1.],
[1., 1., 1., 1.],
[1., 1., 1., 1.],
[1., 1., 1., 1.],
[1., 1., 1., 1.],
[1., 1., 1., 1.],
[1., 1., 1., 1.],
[1., 1., 1., 1.],
[1., 1., 1., 1.],
[1., 1., 1., 1.],
[1., 1., 1., 1.],
[1., 1., 1., 1.],
[1., 1., 1., 1.],
[1., 1., 1., 1.],
[1., 1., 1., 1.],
[1., 1., 1., 1.],
[1., 1., 1., 1.],
[1., 1., 1., 1.],
[1., 1., 1., 1.],
[1., 1., 1., 1.],
[1., 1., 1., 1.],
[1., 1., 1., 1.],
[1., 1., 1., 1.],
[1., 1., 1., 1.],
[1., 1., 1., 1.],
[1., 1., 1., 1.],
[1., 1., 1., 1.],
[1., 1., 1., 1.],
[1., 1., 1., 1.],
[1., 1., 1., 1.],
[1., 1., 1., 1.],
[1., 1., 1., 1.],
[1., 1., 1., 1.],
[1., 1., 1., 1.],
[1., 1., 1., 1.],
[1., 1., 1., 1.],
[1., 1., 1., 1.],
[1., 1., 1., 1.],
[1., 1., 1., 1.],
[1., 1., 1., 1.],
[1., 1., 1., 1.],
[1., 1., 1., 1.],
[1., 1., 1., 1.],
[1., 1., 1., 1.],
[1., 1., 1., 1.],
[1., 1., 1., 1.],
[1., 1., 1., 1.],
[1., 1., 1., 1.],
[1., 1., 1., 1.],
[1., 1., 1., 1.],
[1., 1., 1., 1.],
[1., 1., 1., 1.]])
数据特征选定
单变量特征选定
from sklearn.feature_selection import SelectKBest
from sklearn.feature_selection import chi2
test=SelectKBest(score_func=chi2,k=3)
fit=test.fit(iris.data,iris.target)
print(test.scores_)
features=fit.transform(X)
features
[ 10.81782088 3.7107283 116.31261309 67.0483602 ]
array([[5.1, 1.4, 0.2],
[4.9, 1.4, 0.2],
[4.7, 1.3, 0.2],
[4.6, 1.5, 0.2],
[5. , 1.4, 0.2],
[5.4, 1.7, 0.4],
[4.6, 1.4, 0.3],
[5. , 1.5, 0.2],
[4.4, 1.4, 0.2],
[4.9, 1.5, 0.1],
[5.4, 1.5, 0.2],
[4.8, 1.6, 0.2],
[4.8, 1.4, 0.1],
[4.3, 1.1, 0.1],
[5.8, 1.2, 0.2],
[5.7, 1.5, 0.4],
[5.4, 1.3, 0.4],
[5.1, 1.4, 0.3],
[5.7, 1.7, 0.3],
[5.1, 1.5, 0.3],
[5.4, 1.7, 0.2],
[5.1, 1.5, 0.4],
[4.6, 1. , 0.2],
[5.1, 1.7, 0.5],
[4.8, 1.9, 0.2],
[5. , 1.6, 0.2],
[5. , 1.6, 0.4],
[5.2, 1.5, 0.2],
[5.2, 1.4, 0.2],
[4.7, 1.6, 0.2],
[4.8, 1.6, 0.2],
[5.4, 1.5, 0.4],
[5.2, 1.5, 0.1],
[5.5, 1.4, 0.2],
[4.9, 1.5, 0.1],
[5. , 1.2, 0.2],
[5.5, 1.3, 0.2],
[4.9, 1.5, 0.1],
[4.4, 1.3, 0.2],
[5.1, 1.5, 0.2],
[5. , 1.3, 0.3],
[4.5, 1.3, 0.3],
[4.4, 1.3, 0.2],
[5. , 1.6, 0.6],
[5.1, 1.9, 0.4],
[4.8, 1.4, 0.3],
[5.1, 1.6, 0.2],
[4.6, 1.4, 0.2],
[5.3, 1.5, 0.2],
[5. , 1.4, 0.2],
[7. , 4.7, 1.4],
[6.4, 4.5, 1.5],
[6.9, 4.9, 1.5],
[5.5, 4. , 1.3],
[6.5, 4.6, 1.5],
[5.7, 4.5, 1.3],
[6.3, 4.7, 1.6],
[4.9, 3.3, 1. ],
[6.6, 4.6, 1.3],
[5.2, 3.9, 1.4],
[5. , 3.5, 1. ],
[5.9, 4.2, 1.5],
[6. , 4. , 1. ],
[6.1, 4.7, 1.4],
[5.6, 3.6, 1.3],
[6.7, 4.4, 1.4],
[5.6, 4.5, 1.5],
[5.8, 4.1, 1. ],
[6.2, 4.5, 1.5],
[5.6, 3.9, 1.1],
[5.9, 4.8, 1.8],
[6.1, 4. , 1.3],
[6.3, 4.9, 1.5],
[6.1, 4.7, 1.2],
[6.4, 4.3, 1.3],
[6.6, 4.4, 1.4],
[6.8, 4.8, 1.4],
[6.7, 5. , 1.7],
[6. , 4.5, 1.5],
[5.7, 3.5, 1. ],
[5.5, 3.8, 1.1],
[5.5, 3.7, 1. ],
[5.8, 3.9, 1.2],
[6. , 5.1, 1.6],
[5.4, 4.5, 1.5],
[6. , 4.5, 1.6],
[6.7, 4.7, 1.5],
[6.3, 4.4, 1.3],
[5.6, 4.1, 1.3],
[5.5, 4. , 1.3],
[5.5, 4.4, 1.2],
[6.1, 4.6, 1.4],
[5.8, 4. , 1.2],
[5. , 3.3, 1. ],
[5.6, 4.2, 1.3],
[5.7, 4.2, 1.2],
[5.7, 4.2, 1.3],
[6.2, 4.3, 1.3],
[5.1, 3. , 1.1],
[5.7, 4.1, 1.3],
[6.3, 6. , 2.5],
[5.8, 5.1, 1.9],
[7.1, 5.9, 2.1],
[6.3, 5.6, 1.8],
[6.5, 5.8, 2.2],
[7.6, 6.6, 2.1],
[4.9, 4.5, 1.7],
[7.3, 6.3, 1.8],
[6.7, 5.8, 1.8],
[7.2, 6.1, 2.5],
[6.5, 5.1, 2. ],
[6.4, 5.3, 1.9],
[6.8, 5.5, 2.1],
[5.7, 5. , 2. ],
[5.8, 5.1, 2.4],
[6.4, 5.3, 2.3],
[6.5, 5.5, 1.8],
[7.7, 6.7, 2.2],
[7.7, 6.9, 2.3],
[6. , 5. , 1.5],
[6.9, 5.7, 2.3],
[5.6, 4.9, 2. ],
[7.7, 6.7, 2. ],
[6.3, 4.9, 1.8],
[6.7, 5.7, 2.1],
[7.2, 6. , 1.8],
[6.2, 4.8, 1.8],
[6.1, 4.9, 1.8],
[6.4, 5.6, 2.1],
[7.2, 5.8, 1.6],
[7.4, 6.1, 1.9],
[7.9, 6.4, 2. ],
[6.4, 5.6, 2.2],
[6.3, 5.1, 1.5],
[6.1, 5.6, 1.4],
[7.7, 6.1, 2.3],
[6.3, 5.6, 2.4],
[6.4, 5.5, 1.8],
[6. , 4.8, 1.8],
[6.9, 5.4, 2.1],
[6.7, 5.6, 2.4],
[6.9, 5.1, 2.3],
[5.8, 5.1, 1.9],
[6.8, 5.9, 2.3],
[6.7, 5.7, 2.5],
[6.7, 5.2, 2.3],
[6.3, 5. , 1.9],
[6.5, 5.2, 2. ],
[6.2, 5.4, 2.3],
[5.9, 5.1, 1.8]])
递归特征消除
from sklearn.linear_model import LogisticRegression
from sklearn.feature_selection import RFE
mode=LogisticRegression(max_iter=1000)
rfe=RFE(mode,n_features_to_select=3)
fit=rfe.fit(iris.data,iris.target)
print('特征个数:',fit.n_features_)
print('被选定的特征:',fit.support_)
print('特征排名:',fit.ranking_)
特征个数: 3
被选定的特征: [False True True True]
特征排名: [2 1 1 1]
主要成分分析
from sklearn.decomposition import PCA
pca=PCA(n_components=3)
fit=pca.fit(iris.data)
print('解释方差:%s' %fit.explained_variance_ratio_)
print(fit.components_)
解释方差:[0.92461872 0.05306648 0.01710261]
[[ 0.36138659 -0.08452251 0.85667061 0.3582892 ]
[ 0.65658877 0.73016143 -0.17337266 -0.07548102]
[-0.58202985 0.59791083 0.07623608 0.54583143]]
特征重要性
from sklearn.ensemble import ExtraTreesClassifier
model=ExtraTreesClassifier()
fit=model.fit(iris.data,iris.target)
print(fit.feature_importances_)
[0.10698562 0.06329292 0.42825402 0.40146743]
选择模型
评估算法
分离训练数据集和评估数据集
K折交叉验证分离
弃一交叉验证分离
重复随机评估、训练数据集分离
分离训练数据集和评估数据集
from sklearn.linear_model import LogisticRegression
from sklearn.model_selection import train_test_split
X_train,X_test,Y_train,Y_test=train_test_split(iris.data,iris.target,test_size=0.33,random_state=4)
model=LogisticRegression()
model.fit(X_train,Y_train)
model.score(X_test,Y_test)
0.98
K折交叉验证分离
from sklearn.model_selection import KFold
from sklearn.model_selection import cross_val_score
from sklearn.linear_model import LogisticRegression
kfold=KFold(n_splits=10,random_state=7,shuffle=True)
results=cross_val_score(LogisticRegression(solver='lbfgs',max_iter=1000),iris.data,iris.target,cv=kfold)
print(results)
print(results.mean())
print(results.std())
[0.86666667 0.86666667 1. 1. 1. 1.
1. 0.93333333 1. 1. ]
0.9666666666666668
0.053748384988656986
弃一交叉验证分离
from sklearn.model_selection import LeaveOneOut
from sklearn.model_selection import cross_val_score
from sklearn.linear_model import LogisticRegression
model=LogisticRegression(solver='lbfgs',max_iter=1000)
loocv=LeaveOneOut()
results=cross_val_score(model,iris.data,iris.target,cv=loocv)
print(results)
print(results.mean())
print(results.std())
[1. 1. 1. 1. 1. 1. 1. 1. 1. 1. 1. 1. 1. 1. 1. 1. 1. 1. 1. 1. 1. 1. 1. 1.
1. 1. 1. 1. 1. 1. 1. 1. 1. 1. 1. 1. 1. 1. 1. 1. 1. 1. 1. 1. 1. 1. 1. 1.
1. 1. 1. 1. 1. 1. 1. 1. 1. 1. 1. 1. 1. 1. 1. 1. 1. 1. 1. 1. 1. 1. 0. 1.
1. 1. 1. 1. 1. 0. 1. 1. 1. 1. 1. 0. 1. 1. 1. 1. 1. 1. 1. 1. 1. 1. 1. 1.
1. 1. 1. 1. 1. 1. 1. 1. 1. 1. 0. 1. 1. 1. 1. 1. 1. 1. 1. 1. 1. 1. 1. 0.
1. 1. 1. 1. 1. 1. 1. 1. 1. 1. 1. 1. 1. 1. 1. 1. 1. 1. 1. 1. 1. 1. 1. 1.
1. 1. 1. 1. 1. 1.]
0.9666666666666667
0.17950549357115014
重复分离评估数据集与训练数据集
from sklearn.linear_model import LogisticRegression
from sklearn.model_selection import ShuffleSplit
from sklearn.model_selection import cross_val_score
kfold=ShuffleSplit(n_splits=10,test_size=0.33,random_state=7)
results=cross_val_score(LogisticRegression(solver='lbfgs',max_iter=1000),iris.data,iris.target,cv=kfold)
print(results)
print(results.mean())
print(results.std())
[0.92 0.94 0.94 0.9 0.92 1. 0.98 0.98 0.96 0.98]
0.952
0.031240998703626604
算法评估矩阵
分类算法评估矩阵
分类准确度 对数损失函数 AUC图 混淆矩阵 分类报告
分类准确度
from sklearn.linear_model import LogisticRegression
from sklearn.model_selection import ShuffleSplit
from sklearn.model_selection import cross_val_score
kfold=ShuffleSplit(n_splits=10,test_size=0.33,random_state=7)
results=cross_val_score(LogisticRegression(solver='lbfgs',max_iter=1000),iris.data,iris.target,cv=kfold)
print(results)
print(results.mean())
print(results.std())
[0.92 0.94 0.94 0.9 0.92 1. 0.98 0.98 0.96 0.98]
0.952
0.031240998703626604
对数损失函数
from sklearn.linear_model import LogisticRegression
from sklearn.model_selection import ShuffleSplit
from sklearn.model_selection import cross_val_score
kfold=ShuffleSplit(n_splits=10,test_size=0.33,random_state=7)
results=cross_val_score(LogisticRegression(solver='lbfgs',max_iter=1000),iris.data,iris.target,cv=kfold,scoring='neg_log_loss')
print(results)
print(results.mean())
print(results.std())
[-0.20996844 -0.17826908 -0.17633721 -0.18893534 -0.16890273 -0.11502008
-0.11949119 -0.13442667 -0.15348432 -0.13497036]
-0.1579805422237223
0.02993380620566406
AUC图
from sklearn.linear_model import LogisticRegression
from sklearn.model_selection import KFold
from sklearn.model_selection import cross_val_score
kfold=KFold(n_splits=10,random_state=7,shuffle=True)
results=cross_val_score(LogisticRegression(solver='lbfgs',max_iter=1000),iris.data,iris.target,cv=kfold)
print(results)
print(results.mean())
print(results.std())
[0.86666667 0.86666667 1. 1. 1. 1.
1. 0.93333333 1. 1. ]
0.9666666666666668
0.053748384988656986
混淆矩阵
from sklearn.linear_model import LogisticRegression
from sklearn.metrics import confusion_matrix
from sklearn.model_selection import train_test_split
X_train,X_test,Y_train,Y_test=train_test_split(iris.data,iris.target,test_size=0.33,random_state=4)
model=LogisticRegression(solver='lbfgs',max_iter=1000)
model.fit(X_train,Y_train)
matrix=confusion_matrix(Y_test,y_pred=model.predict(X_test))
columns=['0','1','2']
import pandas as pd
dataframe=pd.DataFrame(matrix,columns=columns)
dataframe
分类报告
from sklearn.linear_model import LogisticRegression
from sklearn.metrics import classification_report
from sklearn.model_selection import train_test_split
X_train,X_test,Y_train,Y_test=train_test_split(iris.data,iris.target,test_size=0.33,random_state=4)
model=LogisticRegression(solver='lbfgs',max_iter=1000)
model.fit(X_train,Y_train)
report=classification_report(y_true=Y_train,y_pred=model.predict(X_train))
print(report)
precision recall f1-score support
0 1.00 1.00 1.00 27
1 1.00 0.95 0.97 38
2 0.95 1.00 0.97 35
accuracy 0.98 100
macro avg 0.98 0.98 0.98 100
weighted avg 0.98 0.98 0.98 100
回归算法矩阵
平均绝对误差MAE
均方误差MSE
决定系数
R
2
R^2
R2
平均绝对误差
from sklearn.linear_model import LogisticRegression
from sklearn.model_selection import cross_val_score
from sklearn.model_selection import KFold
kfold=KFold(n_splits=10,random_state=7,shuffle=True)
model=LogisticRegression(solver='lbfgs',max_iter=1000)
results=cross_val_score(model,iris.data,iris.target,cv=kfold,scoring='neg_mean_absolute_error')
print(results)
print(results.mean())
print(results.std())
[-0.13333333 -0.13333333 -0. -0. -0. -0.
-0. -0.06666667 -0. -0. ]
-0.03333333333333333
0.05374838498865701
均方误差
from sklearn.linear_model import LogisticRegression
from sklearn.model_selection import cross_val_score
from sklearn.model_selection import KFold
kfold=KFold(n_splits=10,random_state=7,shuffle=True)
model=LogisticRegression(solver='lbfgs',max_iter=1000)
results=cross_val_score(model,iris.data,iris.target,cv=kfold,scoring='neg_mean_squared_error')
print(results)
print(results.mean())
print(results.std())
[-0.13333333 -0.13333333 -0. -0. -0. -0.
-0. -0.06666667 -0. -0. ]
-0.03333333333333333
0.05374838498865701
决定系数
R
2
R^2
R2
from sklearn.linear_model import LogisticRegression
from sklearn.model_selection import cross_val_score
from sklearn.model_selection import KFold
kfold=KFold(n_splits=10,random_state=7,shuffle=True)
model=LogisticRegression(solver='lbfgs',max_iter=1000)
results=cross_val_score(model,iris.data,iris.target,cv=kfold,scoring='r2')
print(results)
print(results.mean())
print(results.std())
[0.74137931 0.73684211 1. 1. 1. 1.
1. 0.9 1. 1. ]
0.9378221415607986
0.10367057339437748
审查分类算法
线性算法
逻辑回归 线性判别分析
非线性算法
K近邻 贝特斯分类器 分类与回归树 支持向量机
线性算法
逻辑回归
from sklearn.model_selection import KFold
from sklearn.model_selection import cross_val_score
from sklearn.linear_model import LogisticRegression
results=cross_val_score(LogisticRegression(max_iter=1000),iris.data,iris.target,cv=KFold(n_splits=10,random_state=7,shuffle=True))
results.mean()
0.9666666666666668
线性判别分析
from sklearn.model_selection import KFold
from sklearn.model_selection import cross_val_score
from sklearn.discriminant_analysis import LinearDiscriminantAnalysis
results=cross_val_score(LinearDiscriminantAnalysis(),iris.data,iris.target,cv=KFold(n_splits=10,random_state=7,shuffle=True))
results.mean()
0.9800000000000001
非线性算法
K近邻算法
from sklearn.model_selection import KFold
from sklearn.model_selection import cross_val_score
from sklearn.neighbors import KNeighborsClassifier
results=cross_val_score(KNeighborsClassifier(),iris.data,iris.target,cv=KFold(n_splits=10,random_state=7,shuffle=True))
results.mean()
0.9533333333333334
贝叶斯分类器
from sklearn.model_selection import KFold
from sklearn.model_selection import cross_val_score
from sklearn.naive_bayes import GaussianNB
results=cross_val_score(GaussianNB(),iris.data,iris.target,cv=KFold(n_splits=10,random_state=7,shuffle=True))
results.mean()
0.9533333333333334
分类与回归树
from sklearn.model_selection import KFold
from sklearn.model_selection import cross_val_score
from sklearn.tree import DecisionTreeClassifier
results=cross_val_score(DecisionTreeClassifier(),iris.data,iris.target,cv=KFold(n_splits=10,random_state=7,shuffle=True))
results.mean()
0.96
支持向量机
from sklearn.model_selection import KFold
from sklearn.model_selection import cross_val_score
from sklearn.svm import SVC
results=cross_val_score(SVC(),iris.data,iris.target,cv=KFold(n_splits=10,random_state=7,shuffle=True))
results.mean()
0.9600000000000002
审查回归算法
线性算法
线性回归算法 岭回归算法 套索回归算法 弹性网络回归算法
非线性算法
K近邻算法(KNN) 分类与回归树算法 支持向量机(SVM)
线性算法
线性回归算法
from sklearn.model_selection import KFold
from sklearn.model_selection import cross_val_score
from sklearn.linear_model import LinearRegression
results=cross_val_score(LinearRegression(),iris.data,iris.target,cv=KFold(n_splits=10,random_state=7,shuffle=True))
results.mean()
0.9146928063470222
岭回归算法
from sklearn.model_selection import KFold
from sklearn.model_selection import cross_val_score
from sklearn.linear_model import Ridge
results=cross_val_score(Ridge(),iris.data,iris.target,cv=KFold(n_splits=10,random_state=7,shuffle=True))
results.mean()
0.9151100717792608
套索回归算法
from sklearn.model_selection import KFold
from sklearn.model_selection import cross_val_score
from sklearn.linear_model import Lasso
results=cross_val_score(Lasso(),iris.data,iris.target,cv=KFold(n_splits=10,random_state=7,shuffle=True))
results.mean()
0.3710759235590891
弹性网络回归算法
from sklearn.model_selection import KFold
from sklearn.model_selection import cross_val_score
from sklearn.linear_model import ElasticNet
results=cross_val_score(ElasticNet(),iris.data,iris.target,cv=KFold(n_splits=10,random_state=7,shuffle=True))
results.mean()
0.6892616691679934
非线性算法
K近邻算法
from sklearn.model_selection import KFold
from sklearn.model_selection import cross_val_score
from sklearn.neighbors import KNeighborsRegressor
results=cross_val_score(KNeighborsRegressor(),iris.data,iris.target,cv=KFold(n_splits=10,random_state=7,shuffle=True))
results.mean()
0.9458788291858257
分类与回归树
from sklearn.model_selection import KFold
from sklearn.model_selection import cross_val_score
from sklearn.tree import DecisionTreeRegressor
results=cross_val_score(DecisionTreeRegressor(),iris.data,iris.target,cv=KFold(n_splits=10,random_state=7,shuffle=True))
results.mean()
0.9117332123411979
支持向量机
from sklearn.model_selection import KFold
from sklearn.model_selection import cross_val_score
from sklearn.svm import SVR
results=cross_val_score(SVR(),iris.data,iris.target,cv=KFold(n_splits=10,random_state=7,shuffle=True))
results.mean()
0.9351772150972707
算法比较
models={}
models['LR']=LogisticRegression(max_iter=1000)
models['LDA']=LinearDiscriminantAnalysis()
models['KNN']=KNeighborsClassifier()
models['CART']=DecisionTreeClassifier()
models['NB']=GaussianNB()
models['SVM']=SVC()
results=[]
for key in models:
result=cross_val_score(models[key],iris.data,iris.target,cv=KFold(n_splits=10,random_state=7,shuffle=True))
results.append(result)
msg='%s:%.3f(%.3f)'%(key,result.mean(),result.std())
print(msg)
from matplotlib import pyplot
fig=pyplot.figure()
fig.suptitle('comparison')
ax=fig.add_subplot(111)
pyplot.boxplot(results)
ax.set_xticklabels(models.keys())
LR:0.967(0.054)
LDA:0.980(0.031)
KNN:0.953(0.052)
CART:0.947(0.065)
NB:0.953(0.067)
SVM:0.960(0.053)
[Text(1, 0, 'LR'),
Text(2, 0, 'LDA'),
Text(3, 0, 'KNN'),
Text(4, 0, 'CART'),
Text(5, 0, 'NB'),
Text(6, 0, 'SVM')]
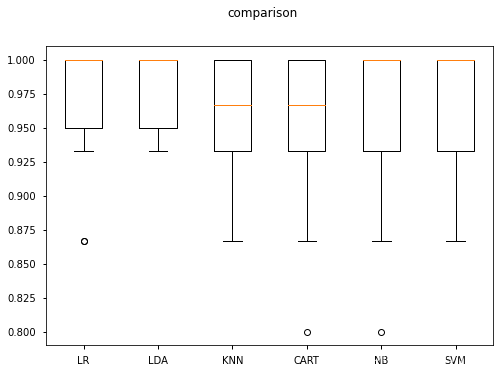
自动流程
数据准备和生成模型的pipeline
from sklearn.model_selection import KFold
from sklearn.model_selection import cross_val_score
from sklearn.preprocessing import StandardScaler
from sklearn.pipeline import Pipeline
from sklearn.discriminant_analysis import LinearDiscriminantAnalysis
model=Pipeline([('std',StandardScaler()),('lin',LinearDiscriminantAnalysis())])
results=cross_val_score(model,iris.data,iris.target,cv=KFold(n_splits=10,random_state=7,shuffle=True))
results.mean()
0.9800000000000001
特征选择和生成模型的pipeline
from sklearn.model_selection import KFold
from sklearn.model_selection import cross_val_score
from sklearn.linear_model import LogisticRegression
from sklearn.pipeline import FeatureUnion
from sklearn.pipeline import Pipeline
from sklearn.decomposition import PCA
from sklearn.feature_selection import SelectKBest
from sklearn.pipeline import Pipeline
from sklearn.discriminant_analysis import LinearDiscriminantAnalysis
fea=[('pca',PCA()),('select',SelectKBest(k=3))]
model=Pipeline([('fea',FeatureUnion(fea)),('log',LogisticRegression(max_iter=1000))])
results=cross_val_score(model,iris.data,iris.target,cv=KFold(n_splits=10,random_state=7,shuffle=True))
results.mean()
0.96
优化模型
集成算法
袋装算法
袋装决策树
from sklearn.model_selection import KFold
from sklearn.model_selection import cross_val_score
from sklearn.ensemble import BaggingClassifier
from sklearn.tree import DecisionTreeClassifier
model=BaggingClassifier(base_estimator=DecisionTreeClassifier(),n_estimators=100,random_state=7)
result=cross_val_score(model,iris.data,iris.target,cv=KFold(n_splits=10,random_state=7,shuffle=True))
print(result)
result.mean()
[0.86666667 0.86666667 1. 1. 1. 1.
1. 0.93333333 0.93333333 1. ]
0.96
随机森林
from sklearn.model_selection import KFold
from sklearn.model_selection import cross_val_score
from sklearn.ensemble import RandomForestClassifier
model=RandomForestClassifier(n_estimators=100,random_state=7,max_features=2)
result=cross_val_score(model,iris.data,iris.target,cv=KFold(n_splits=10,random_state=7,shuffle=True))
print(result)
result.mean()
[0.86666667 0.86666667 1. 1. 0.93333333 1.
1. 0.93333333 0.93333333 1. ]
0.9533333333333334
极端森林
from sklearn.model_selection import KFold
from sklearn.model_selection import cross_val_score
from sklearn.ensemble import ExtraTreesClassifier
model=ExtraTreesClassifier(n_estimators=100,random_state=7,max_features=2)
result=cross_val_score(model,iris.data,iris.target,cv=KFold(n_splits=10,random_state=7,shuffle=True))
print(result)
result.mean()
[0.86666667 0.86666667 1. 1. 0.93333333 1.
1. 0.93333333 0.93333333 0.93333333]
0.9466666666666667
提升算法
AdaBoost
from sklearn.model_selection import KFold
from sklearn.model_selection import cross_val_score
from sklearn.ensemble import AdaBoostClassifier
model=AdaBoostClassifier(n_estimators=100,random_state=7)
result=cross_val_score(model,iris.data,iris.target,cv=KFold(n_splits=10,random_state=7,shuffle=True))
print(result)
result.mean()
[0.93333333 0.86666667 1. 1. 0.93333333 1.
1. 0.93333333 1. 1. ]
0.9666666666666666
随机梯度提升
from sklearn.model_selection import KFold
from sklearn.model_selection import cross_val_score
from sklearn.ensemble import GradientBoostingClassifier
model=GradientBoostingClassifier(n_estimators=100,random_state=7)
result=cross_val_score(model,iris.data,iris.target,cv=KFold(n_splits=10,random_state=7,shuffle=True))
print(result)
result.mean()
[0.93333333 0.8 1. 1. 1. 1.
1. 0.93333333 0.93333333 1. ]
0.96
投票算法
from sklearn.model_selection import KFold
from sklearn.model_selection import cross_val_score
from sklearn.ensemble import VotingClassifier
from sklearn.tree import DecisionTreeClassifier
from sklearn.svm import SVC
from sklearn.linear_model import LogisticRegression
model=VotingClassifier(estimators=[('cart',DecisionTreeClassifier()),('logistic',LogisticRegression(max_iter=1000)),('svm',SVC())])
result=cross_val_score(model,iris.data,iris.target,cv=KFold(n_splits=10,random_state=7,shuffle=True))
print(result)
result.mean()
[0.86666667 0.86666667 1. 1. 1. 1.
1. 0.93333333 1. 1. ]
0.9666666666666668
算法调参
网格搜索优化参数
from sklearn.linear_model import Ridge
from sklearn.model_selection import GridSearchCV
model=Ridge()
param_grid={'alpha':[1,0.1,0.01,0.001,0]}
grid=GridSearchCV(estimator=model,param_grid=param_grid)
grid.fit(iris.data,iris.target)
print(grid.best_score_)
print(grid.best_estimator_.alpha)
0.3225607248900085
0
随机搜索优化参数
from sklearn.linear_model import Ridge
from sklearn.model_selection import RandomizedSearchCV
from scipy.stats import uniform
model=Ridge()
param_grid={'alpha':uniform}
grid=RandomizedSearchCV(estimator=model,param_distributions=param_grid,n_iter=100,random_state=7)
grid.fit(iris.data,iris.target)
print(grid.best_score_)
print(grid.best_estimator_.alpha)
0.32255899144910904
0.0014268805627581926
结果部署
持久化加载模型
通过pickle序列化和反序列化机器学习的模型
from sklearn.model_selection import train_test_split
from sklearn.linear_model import LogisticRegression
from pickle import dump
from pickle import load
validation_size=0.33
seed=4
X_train,X_test,Y_train,Y_test=train_test_split(iris.data,iris.target,test_size=validation_size,random_state=seed)
model=LogisticRegression(max_iter=1000)
model.fit(X_train,Y_train)
model_file='finalized_model.sav'
with open(model_file,'wb') as model_f:
dump(model,model_f)
with open(model_file,'rb') as model_f:
load_model=load(model_f)
result=load_model.score(X_test,Y_test)
result
0.98
通过joblib序列化和反序列化机器学习的模型
from sklearn.model_selection import train_test_split
from sklearn.linear_model import LogisticRegression
from joblib import dump
from joblib import load
validation_size=0.33
seed=4
X_train,X_test,Y_train,Y_test=train_test_split(iris.data,iris.target,test_size=validation_size,random_state=seed)
model=LogisticRegression(max_iter=1000)
model.fit(X_train,Y_train)
model_file='finalized_model_joblib.sav'
with open(model_file,'wb') as model_f:
dump(model,model_f)
with open(model_file,'rb') as model_f:
load_model=load(model_f)
result=load_model.score(X_test,Y_test)
result
0.98
|