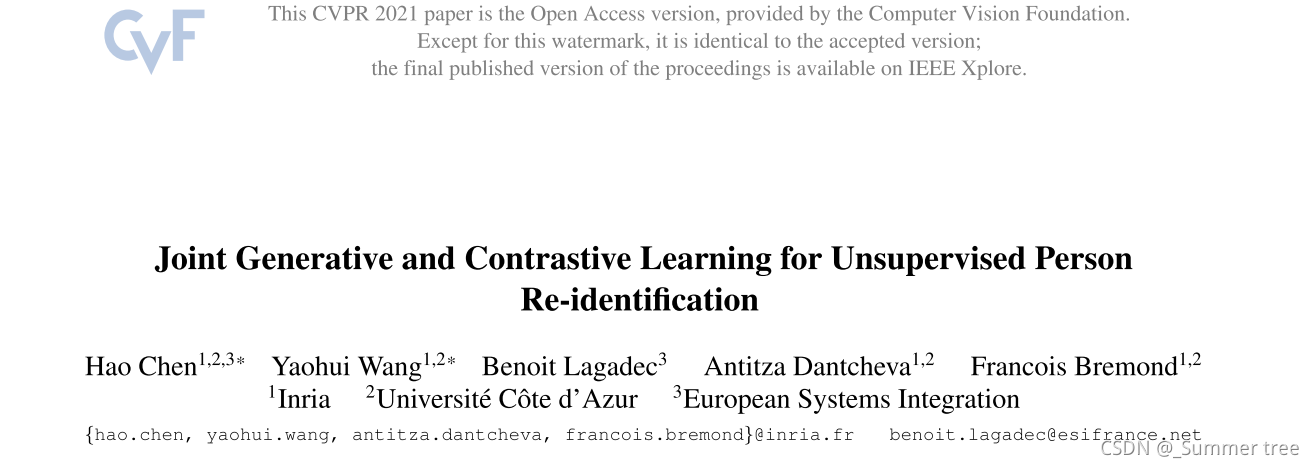
方法概述
1,提出了一种用于无监督行人重识别的联合生成对比学习框架,生成和对比模块互相提高对方的性能。 2, 在生成模块中,我们引入了3D网格生成器。 3, 在对比模块,我们提出了一种视角无关的损失,来减少生成样本和原始样本之间的类内变化。
内容概要
论文名称 | 简称 | 会议/期刊 | 出版年份 | baseline | backbone | 数据集 |
---|
Joint Generative and Contrastive Learning for Unsupervised Person Re-identification | GCL | CVPR | 2021 | 【JVTC】Li, J., Zhang, S.: Joint visual and temporal consistency for unsupervised domain adaptive person re- identification. pp. 1–14 (2020) | ImageNet [32] pre-trained ResNet50 [17] with slight modifications | Market-1501、DukeMTMC-reID, MSMT17 [41] |
在线链接:https://openaccess.thecvf.com/content/CVPR2021/html/Chen_Joint_Generative_and_Contrastive_Learning_for_Unsupervised_Person_Re-Identification_CVPR_2021_paper.html 源码链接: https: //github.com/chenhao2345/GCL.
工作概述
1, we incorporate a Generative Adversarial Network (GAN) and a contrastive learning module into one joint training framework. 2, While the GAN provides online data augmentation for contrastive learning, the contrastive module learns view-invariant fea- tures for generation. 3, we propose a mesh- based view generator. Specifically, mesh projections serve as references towards generating novel views of a per- son. 4,we propose a view-invariant loss to fa- cilitate contrastive learning between original and gener- ated views.
成果概述
our method significantly outperforms state-of-the-art methods under both, fully unsupervised and unsupervised domain adaptive settings on several large scale ReID dat- sets.
方法详解
方法框架
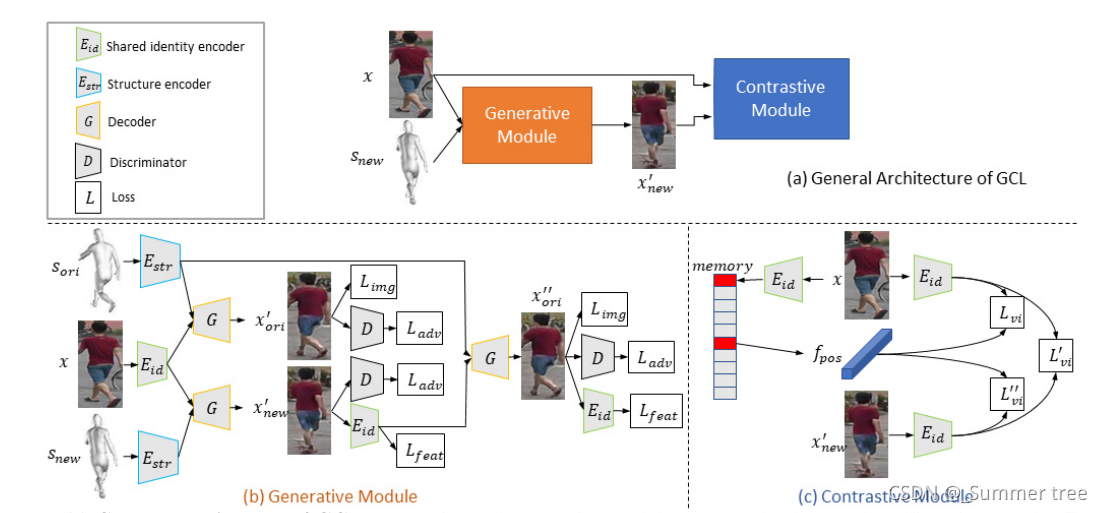
Figure 2: (a) General architecture of GCL: Generative and contrastive modules are coupled by the shared identity encoder Eid. (b) Generative module: The decoder G combines the identity features encoded by Eid and structure features Estr to generate a novel view x′ new with a cycle consistency. ? Contrastive module: View-invariance is enhanced by maximizing the agreement between original Eid(x), synthesized Eid(x′ new) and memory fpos representations.
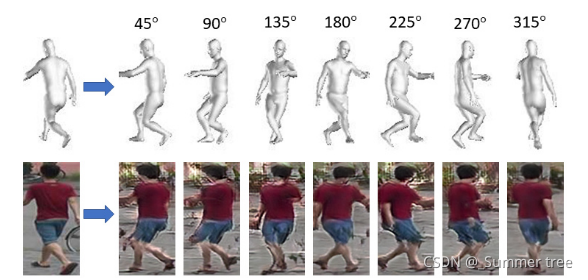
Figure 3: Example images as generated by the View Generator via 3D mesh rotation based on left input image.
具体实现
1,GCL框架主要包含了 生成模块和 对比模块两个模块。 2, 在生成模块中,文章通过HMR构建3D网格,提取图像的外观和姿势。 然后通过对姿势进行不同角度的旋转来重新构成样本,以此从样本、特征和解码结果三个层面构成损失gan。   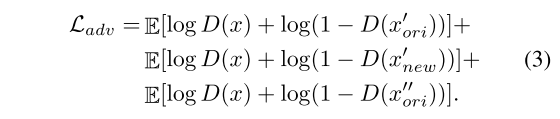 
3, 在对比模块中,文章维护了一个内存条(memory bank)来存储样本的特征向量,并在迭代过程中根据公式5更新。然后从前面诸多的样本中构造正负样本对,然后求对比损失。  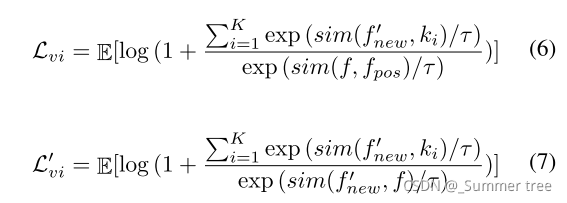 
4,联合训练采用热启动的形式,基于baseline工作训练先进行40epoch学习gan损失,在最后20个epoch才学习总体损失(公式9) 
实验结果
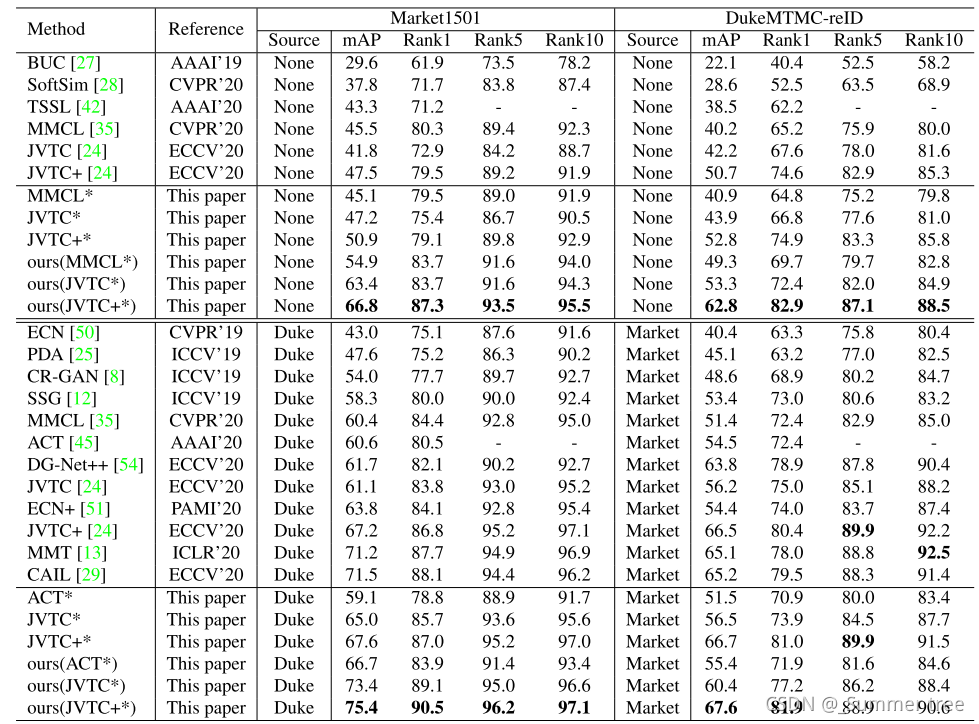 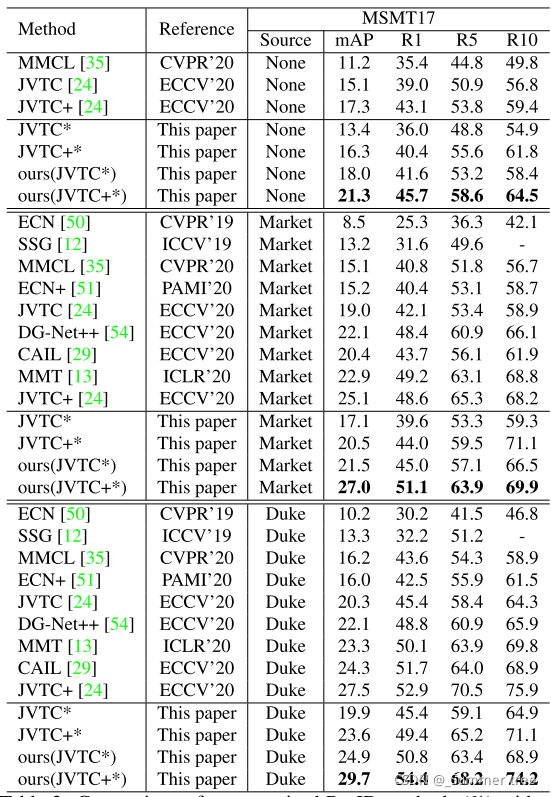
总体评价
1, 基本上所有创新点都基于一开始想到了是用3D网格来生成样本,在这个基础上,后面的创新点都水到渠成的出来了。 2,感觉各种样本的合成以及组合有点繁杂了。 3,当没有一个漂亮的大图的时候,多部分组图也可以成为framework。画图不够高端。
引用格式
@inproceedings{DBLP:conf/cvpr/ChenWLDB21, author = {Hao Chen and Yaohui Wang and Benoit Lagadec and Antitza Dantcheva and Fran{\c{c}}ois Br{’{e}}mond}, title = {Joint Generative and Contrastive Learning for Unsupervised Person Re-Identification}, booktitle = {{CVPR}}, pages = {2004–2013}, publisher = {Computer Vision Foundation / {IEEE}}, year = {2021} }
参考文献
[1] Slawomir Bak, Peter Carr, and Jean-Francois Lalonde. Do- main adaptation through synthesis for unsupervised person re-identification. In ECCV, 2018. 1 [2] Andrew Brock, Jeff Donahue, and Karen Simonyan. Large scale GAN training for high fidelity natural image synthesis. In ICLR, 2019. 2 [3] Caroline Chan, Shiry Ginosar, Tinghui Zhou, and Alexei A Efros. Everybody dance now. In ICCV, 2019. 2 [4] Hao Chen, Benoit Lagadec, and Francois Bremond. Learn- ing discriminative and generalizable representations by spatial-channel partition for person re-identification. In WACV, 2020. 1 [5] Hao Chen, Benoit Lagadec, and Francois Bremond. En- hancing diversity in teacher-student networks via asymmet- ric branches for unsupervised person re-identification. In WACV, 2021. 3 [6] Ting Chen, Simon Kornblith, Mohammad Norouzi, and Ge- offrey Hinton. A simple framework for contrastive learning of visual representations. In ICML, 2020. 1, 2 [7] Xinlei Chen, Haoqi Fan, Ross Girshick, and Kaiming He. Improved baselines with momentum contrastive learning. arXiv preprint arXiv:2003.04297, 2020. 1, 2 [8] Yanbei Chen, Xiatian Zhu, and Shaogang Gong. Instance- guided context rendering for cross-domain person re- identification. In ICCV, 2019. 3, 6 [9]Weijian Deng, Liang Zheng, Qixiang Ye, Guoliang Kang, Yi Yang, and Jianbin Jiao. Image-image domain adaptation with preserved self-similarity and domain-dissimilarity for person re-identification. In CVPR, 2018. 1, 3 [10] Chanho Eom and Bumsub Ham. Learning disentangled rep- resentation for robust person re-identification. In NeurIPS, 2019. 2, 7, 8 [11] Martin Ester, Hans-Peter Kriegel, J¨org Sander, and Xiaowei Xu. A density-based algorithm for discovering clusters in large spatial databases with noise. In KDD, 1996. 4 [12] Yang Fu, Yunchao Wei, Guanshuo Wang, Yuqian Zhou, Honghui Shi, and Thomas S Huang. Self-similarity group- ing: A simple unsupervised cross domain adaptation ap- proach for person re-identification. In ICCV, 2019. 3, 6, 7 [13] Yixiao Ge, Dapeng Chen, and Hongsheng Li. Mutual mean- teaching: Pseudo label refinery for unsupervised domain adaptation on person re-identification. In ICLR, 2020. 3, 6, 7 [14] Yixiao Ge, Zhuowan Li, Haiyu Zhao, Guojun Yin, Shuai Yi, Xiaogang Wang, and Hongsheng Li. Fd-gan: Pose-guided feature distilling gan for robust person re-identification. In NeurIPS, 2018. 2, 7, 8 [15] Ian Goodfellow, Jean Pouget-Abadie, Mehdi Mirza, Bing Xu, David Warde-Farley, Sherjil Ozair, Aaron Courville, and Yoshua Bengio. Generative adversarial nets. In NeurIPS, 2014. 1, 2 [16] Kaiming He, Haoqi Fan, Yuxin Wu, Saining Xie, and Ross Girshick. Momentum contrast for unsupervised visual rep- resentation learning. In CVPR, 2020. 1, 2 [17] Kaiming He, Xiangyu Zhang, Shaoqing Ren, and Jian Sun. Deep residual learning for image recognition. In CVPR, 2016. 5 [18] Martin Heusel, Hubert Ramsauer, Thomas Unterthiner, Bernhard Nessler, and Sepp Hochreiter. Gans trained by a two time-scale update rule converge to a local nash equilib- rium. In NeurIPS, 2017. 8 [19] Xun Huang and Serge Belongie. Arbitrary style transfer in real-time with adaptive instance normalization. In ICCV, 2017. 5 [20] Phillip Isola, Jun-Yan Zhu, Tinghui Zhou, and Alexei A Efros. Image-to-image translation with conditional adver- sarial networks. In CVPR, 2017. 5 [21] Angjoo Kanazawa, Michael J. Black, David W. Jacobs, and Jitendra Malik. End-to-end recovery of human shape and pose. In CVPR, 2018. 2, 3 [22] Tero Karras, Samuli Laine, and Timo Aila. A style-based generator architecture for generative adversarial networks. In CVPR, 2019. 2 [23] Tero Karras, Samuli Laine, Miika Aittala, Janne Hellsten, Jaakko Lehtinen, and Timo Aila. Analyzing and improving the image quality of StyleGAN. In CVPR, 2020. 2 [24] Jianing Li and Shiliang Zhang. Joint visual and tempo- ral consistency for unsupervised domain adaptive person re- identification. In ECCV, 2020. 1, 2, 3, 5, 6, 7 [25] Yu-Jhe Li, Ci-Siang Lin, Yan-Bo Lin, and Yu-Chiang Frank Wang. Cross-dataset person re-identification via unsuper- vised pose disentanglement and adaptation. In ICCV, 2019. 1, 2, 3, 6, 8 [26]Shan Lin, Haoliang Li, Chang-Tsun Li, and Alex Chichung Kot. Multi-task mid-level feature alignment network for un- supervised cross-dataset person re-identification. In BMVC, 2018. 3 [27] Yutian Lin, Xuanyi Dong, Liang Zheng, Yan Yan, and Yi Yang. A bottom-up clustering approach to unsupervised per- son re-identification. In AAAI, 2019. 1, 3, 6 [28] Yutian Lin, Lingxi Xie, Yu Wu, Chenggang Yan, and Qi Tian. Unsupervised person re-identification via softened similarity learning. In CVPR, 2020. 3, 6 [29] Chuanchen Luo, Chunfeng Song, and Zhaoxiang Zhang. Generalizing person re-identification by camera-aware in- variance learning and cross-domain mixup. In ECCV, 2020. 2, 6, 7 [30] Aaron van den Oord, Yazhe Li, and Oriol Vinyals. Repre- sentation learning with contrastive predictive coding. arXiv preprint arXiv:1807.03748, 2018. 2, 4 [31] Ergys Ristani, Francesco Solera, Roger Zou, Rita Cucchiara, and Carlo Tomasi. Performance measures and a data set for multi-target, multi-camera tracking. In ECCVW, 2016. 5 [32] Olga Russakovsky, J. Deng, H. Su, J. Krause, S. Satheesh, S. Ma, Zhiheng Huang, A. Karpathy, A. Khosla, M. Bern- stein, A. Berg, and Li Fei-Fei. Imagenet large scale visual recognition challenge. IJCV, 2015. 5 [33] Yifan Sun, Liang Zheng, Yi Yang, Qi Tian, and Shengjin Wang. Beyond part models: Person retrieval with refined part pooling (and a strong convolutional baseline). In ECCV, 2018. 1 [34] Sergey Tulyakov, Ming-Yu Liu, Xiaodong Yang, and Jan Kautz. MoCoGAN: Decomposing motion and content for video generation. In CVPR, 2018. 2 [35] DongkaiWang and Shiliang Zhang. Unsupervised person re- identification via multi-label classification. In CVPR, 2020. 1, 2, 3, 5, 6, 7 [36] Jingya Wang, Xiatian Zhu, Shaogang Gong, and Wei Li. Transferable joint attribute-identity deep learning for unsu- pervised person re-identification. CVPR, 2018. 3 [37] Yaohui Wang, Piotr Bilinski, Francois Bremond, and Antitza Dantcheva. G3AN: Disentangling appearance and motion for video generation. In CVPR, 2020. 2 [38] Yaohui Wang, Piotr Bilinski, Francois Bremond, and Antitza Dantcheva. Imaginator: Conditional spatio-temporal gan for video generation. In WACV, 2020. 2 [39] Yaohui Wang, Francois Bremond, and Antitza Dantcheva. Inmodegan: Interpretable motion decomposition generative adversarial network for video generation. arXiv preprint arXiv:2101.03049, 2021. 2 [40] Zhou Wang, Alan C Bovik, Hamid R Sheikh, and Eero P Simoncelli. Image quality assessment: from error visibility to structural similarity. TIP, 2004. 8 [41] Longhui Wei, Shiliang Zhang, Wen Gao, and Qi Tian. Person transfer gan to bridge domain gap for person re- identification. In CVPR, 2018. 1, 3, 5 [42] Guile Wu, Xiatian Zhu, and Shaogang Gong. Track- let self-supervised learning for unsupervised person re- identification. In AAAI, 2020. 3, 6 [43] YuWu, Yutian Lin, Xuanyi Dong, Yan Yan, Wei Bian, and Yi Yang. Progressive learning for person re-identification with one example. TIP, 2019. 3 [44] Zhirong Wu, Yuanjun Xiong, Stella X. Yu, and Dahua Lin. Unsupervised feature learning via non-parametric instance discrimination. In CVPR, 2018. 2, 4 [45] Fengxiang Yang, Ke Li, Zhun Zhong, Zhiming Luo, Xing Sun, Hao Cheng, Xiaowei Guo, Feiyue Huang, Rongrong Ji, and Shaozi Li. Asymmetric co-teaching for unsupervised cross-domain person re-identification. In AAAI, 2020. 3, 5, 6 [46] Liang Zheng, Liyue Shen, Lu Tian, Shengjin Wang, Jing- dong Wang, and Qi Tian. Scalable person re-identification: A benchmark. ICCV, 2015. 5 [47] Zhedong Zheng, Xiaodong Yang, Zhiding Yu, Liang Zheng, Yi Yang, and Jan Kautz. Joint discriminative and generative learning for person re-identification. In CVPR, 2019. 3, 5, 7, 8 [48] Zhun Zhong, Liang Zheng, Guoliang Kang, Shaozi Li, and Yi Yang. Random erasing data augmentation. In AAAI, 2020. 2 [49] Zhun Zhong, Liang Zheng, Shaozi Li, and Yi Yang. Gener- alizing a person retrieval model hetero- and homogeneously. In ECCV, 2018. 1, 3 [50] Zhun Zhong, Liang Zheng, Zhiming Luo, Shaozi Li, and Yi Yang. Invariance matters: Exemplar memory for domain adaptive person re-identification. In CVPR, 2019. 3, 6, 7 [51] Zhun Zhong, Liang Zheng, Zhiming Luo, Shaozi Li, and Yi Yang. Learning to adapt invariance in memory for person re-identification. PAMI, 2020. 3, 6, 7 [52] Zhun Zhong, Liang Zheng, Zhedong Zheng, Shaozi Li, and Yi Yang. Camera style adaptation for person re- identification. In CVPR, 2018. 2 [53] Jun-Yan Zhu, Taesung Park, Phillip Isola, and Alexei A Efros. Unpaired image-to-image translation using cycle- consistent adversarial networks. In ICCV, 2017. 2, 3 [54] Yang Zou, Xiaodong Yang, Zhiding Yu, B. V. K. Vijaya Ku- mar, and Jan Kautz. Joint disentangling and adaptation for cross-domain person re-identification. In ECCV, 2020. 1, 3, 5, 6, 7, 8
|