数组的拼接
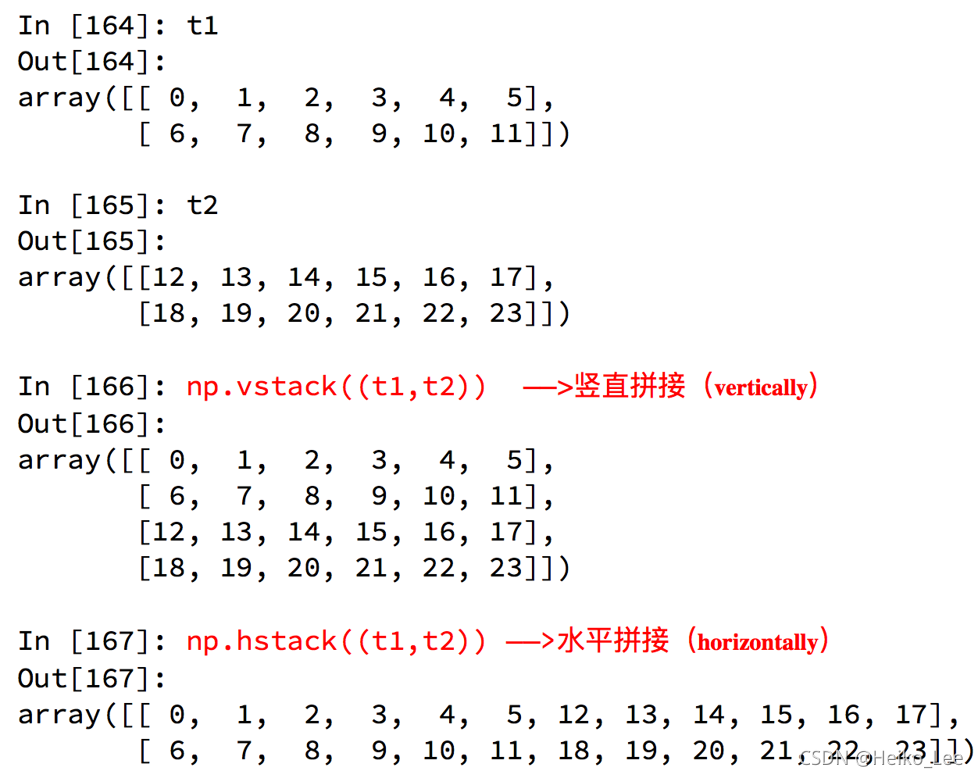
竖直拼接的时候:每一列代表的意义相同,否则牛头不对马嘴。
如果每一列的意义不同,这个时候应该交换某一组的数的列,让其和另外一类相同。
数组的行列交换
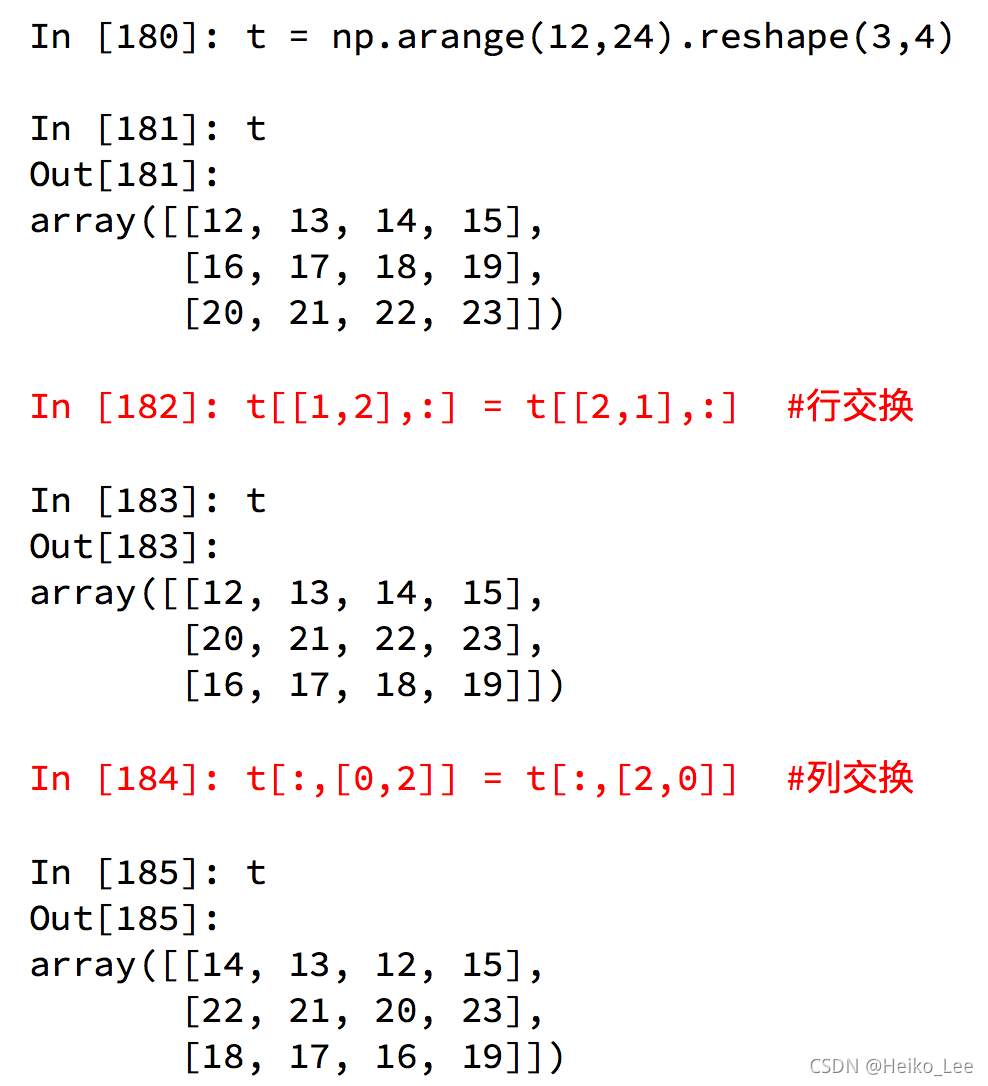
练习:现在希望把之前案例中两个国家的数据方法一起来研究分析,同时保留国家的信息(每条数据的国家来源),应该怎么办?
import numpy as np
us_data = "./youtube_video_data/US_video_data_numbers.csv"
uk_data = "./youtube_video_data/GB_video_data_numbers.csv"
us_data = np.loadtxt(us_data,delimiter=",",dtype=int)
uk_data = np.loadtxt(uk_data,delimiter=",",dtype=int)
zeros_data = np.zeros((us_data.shape[0],1)).astype(int)
ones_data = np.ones((uk_data.shape[0],1)).astype(int)
us_data = np.hstack((us_data,zeros_data))
uk_data = np.hstack((uk_data,ones_data))
final_data = np.vstack((us_data,uk_data))
print(final_data)
运行结果:
[[4394029 320053 5931 46245 0]
[7860119 185853 26679 0 0]
[5845909 576597 39774 170708 0]
...
[ 109222 4840 35 212 1]
[ 626223 22962 532 1559 1]
[ 99228 1699 23 135 1]]
numpy更多方法
-
获取最大值最小值的位置 np.argmax(t,axis=0) #0轴 np.argmin(t,axis=1) #1轴 -
创建一个全0的数组: np.zeros((3,4)) -
创建一个全1的数组: np.ones((3,4)) -
创建一个对角线为1的正方形数组(方阵): np.eye(3)
numpy生成随机数
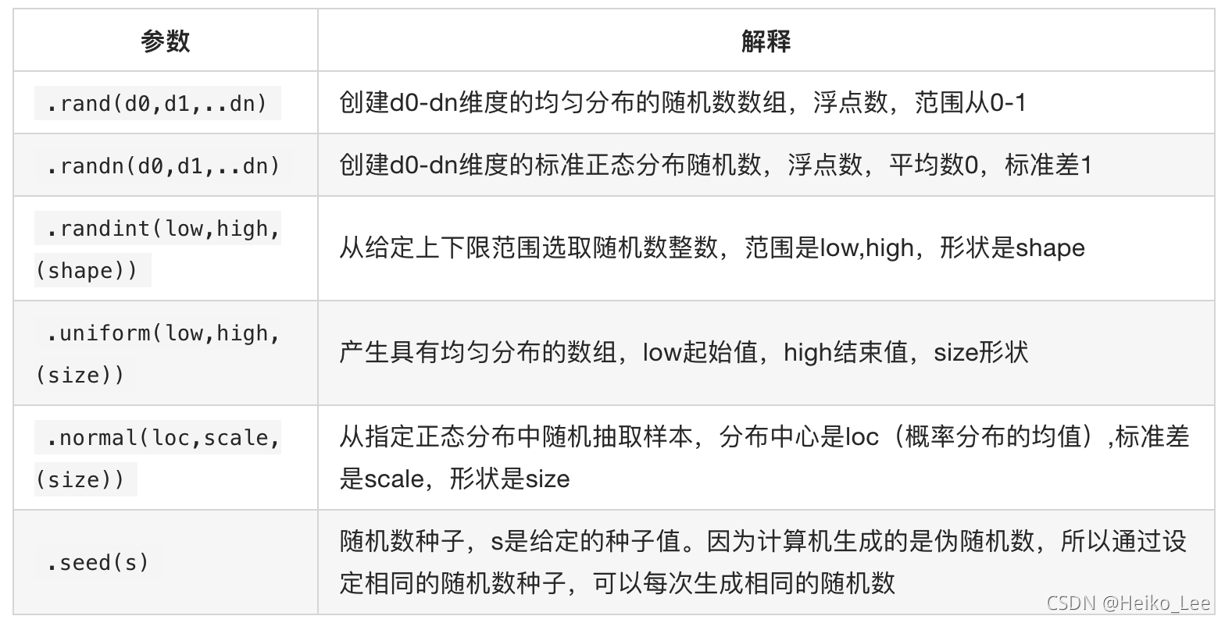
.random.seed(s)用法:
np.random.seed(0)
np.random.rand(10)
Out[357]:
array([0.5488135 , 0.71518937, 0.60276338, 0.54488318, 0.4236548 ,
0.64589411, 0.43758721, 0.891773 , 0.96366276, 0.38344152])
np.random.rand(10)
Out[358]:
array([0.79172504, 0.52889492, 0.56804456, 0.92559664, 0.07103606,
0.0871293 , 0.0202184 , 0.83261985, 0.77815675, 0.87001215])
第二遍的np.random.rand(10)已经不是在你设置的np.random.seed(0)下了,所以第二遍的随机数组只是在默认random下随机挑选的样本数值。 只需要再输入一遍np.random.seed(0)就好:
np.random.seed(0)
np.random.rand(4,3)
Out[362]:
array([[0.5488135 , 0.71518937, 0.60276338],
[0.54488318, 0.4236548 , 0.64589411],
[0.43758721, 0.891773 , 0.96366276],
[0.38344152, 0.79172504, 0.52889492]])
np.random.seed(0)
np.random.rand(4,3)
Out[364]:
array([[0.5488135 , 0.71518937, 0.60276338],
[0.54488318, 0.4236548 , 0.64589411],
[0.43758721, 0.891773 , 0.96366276],
[0.38344152, 0.79172504, 0.52889492]])
pandas学习
numpy能够帮我们处理处理数值型数据,但是这还不够。很多时候,我们的数据除了数值之外,还有字符串,还有时间序列等。
比如:我们通过爬虫获取到了存储在数据库中的数据。
比如:之前youtube的例子中除了数值之外还有国家的信息,视频的分类(tag)信息,标题信息等。
所以,numpy能够帮助我们处理数值,但是pandas除了处理数值之外(基于numpy),还能够帮助我们处理其他类型的数据。
pandas的常用数据类型
- Series 一维,带标签数组
- DataFrame 二维,Series容器
pandas之Series创建
t1 = pd.Series([1,2,31,12,3,4])
print(t1)
print(type(t1))
t2 = pd.Series([1,2,31,12,3,4],index=list('abcdef'))
print(t2)
运行结果:
0 1
1 2
2 31
3 12
4 3
5 4
dtype: int64
<class 'pandas.core.series.Series'>
a 1
b 2
c 31
d 12
e 3
f 4
dtype: int64
还可以通过字典创建: 索引就是字典的键
temp_dict = {"name":"Heiko","age":24,"tel":10086}
t3 = pd.Series(temp_dict)
print(t3)
运行结果:
name Heiko
age 24
tel 10086
dtype: object
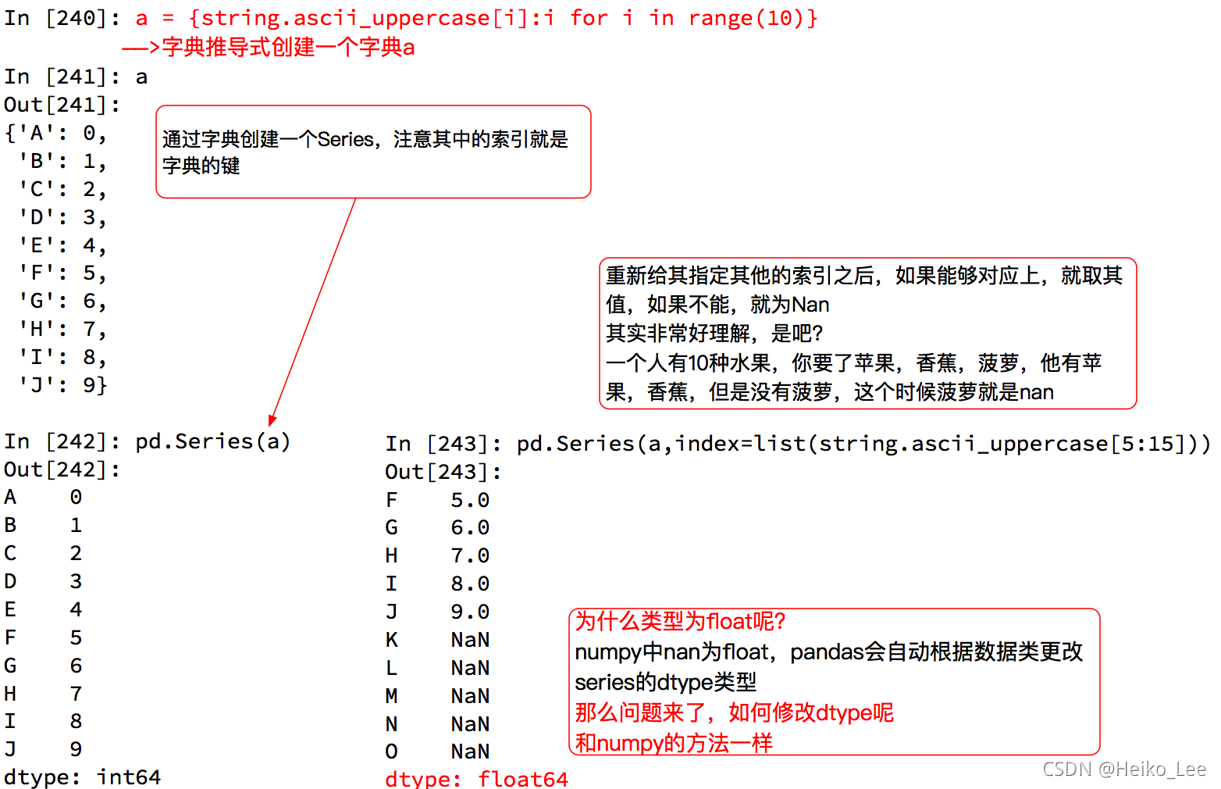
修改dtype:
t2f = t2.astype(float)
print(t2f)
运行结果:
a 1.0
b 2.0
c 31.0
d 12.0
e 3.0
f 4.0
dtype: float64
pandas之Series切片和索引
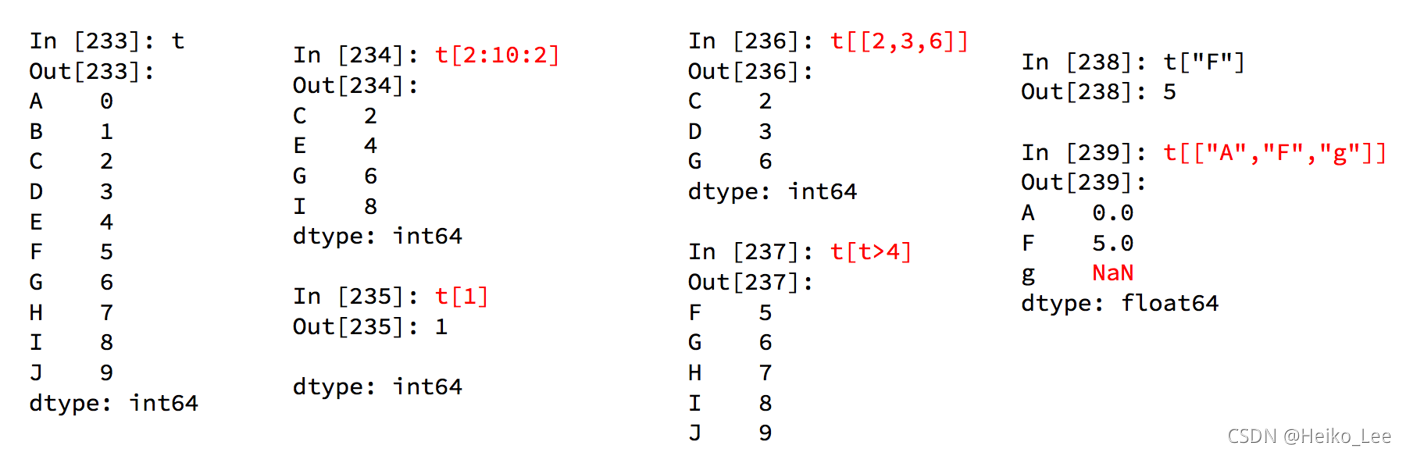
- 切片:直接传入start end 或者步长即可;
- 索引:一个的时候直接传入序号或者index,多个的时候传入序号或者index的列表。
pandas之Series的索引和值
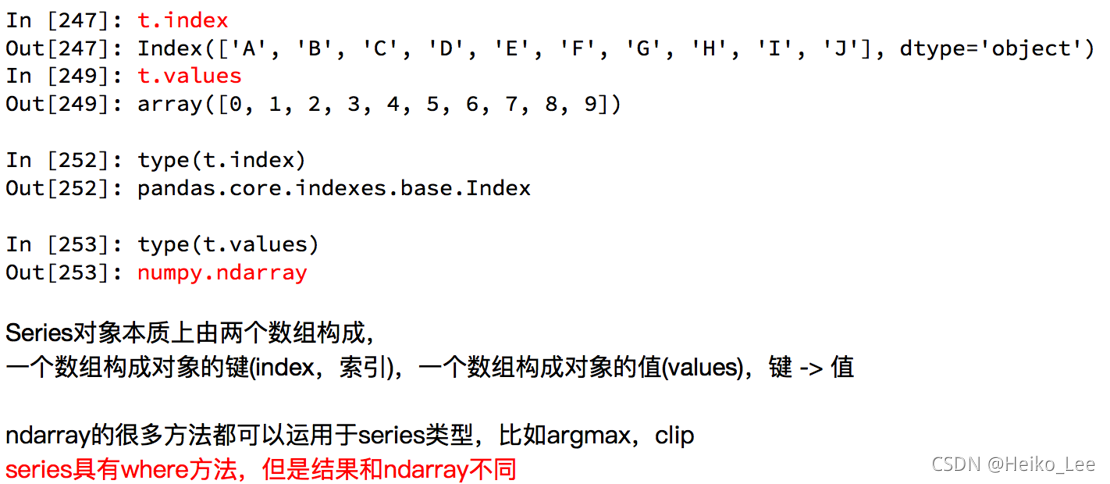
a = pd.Series(range(5))
print(a.where(a>0))
print(a.mask(a>0))
print(a.where(a>0,10))
运行结果:
0 NaN
1 1.0
2 2.0
3 3.0
4 4.0
dtype: float64
0 0.0
1 NaN
2 NaN
3 NaN
4 NaN
dtype: float64
0 10
1 1
2 2
3 3
4 4
dtype: int64
pandas之读取外部数据
现在假设我们有一个组关于狗的名字的统计数据,那么为了观察这组数据的情况,我们应该怎么做呢?
我们的这组数据存在csv中,我们直接使用pd. read_csv即可
和我们想象的有些差别,我们以为他会是一个Series类型,但是他是一个DataFrame,那么接下来我们就来了解这种数据类型
但是,还有一个问题: 对于数据库比如mysql或者mongodb中数据我们如何使用呢?
pd.read_sql(sql_sentence,connection)
那么,mongodb呢?
pandas之DataFrame
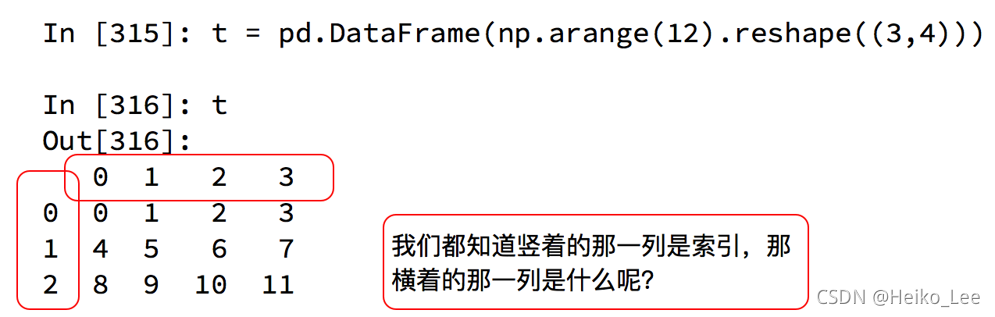
DataFrame对象既有行索引,又有列索引 行索引,表明不同行,横向索引,叫index,0轴,axis=0 列索引,表名不同列,纵向索引,叫columns,1轴,axis=1
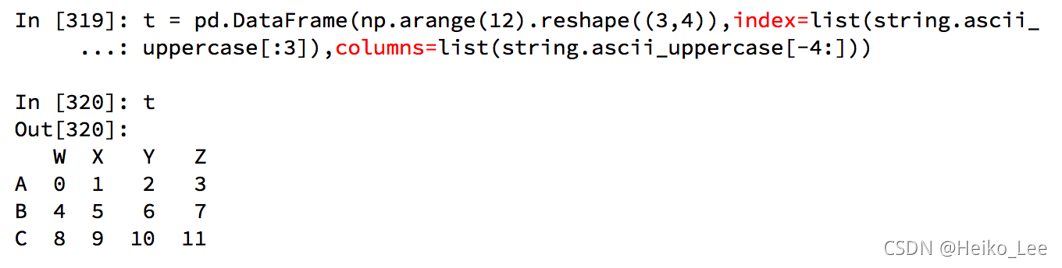
- DataFrame相当于是Series的容器
- DataFrame能够传入字典作为数据
import pandas as pd
d1 = {"name":["Heiko","Lee"],"age":[24,30],"tel":[10086,10010]}
t1 = pd.DataFrame(d1)
print(t1)
print(type(t1))
d2 = [{"name":"Heiko","age":24,"tel":10086},{"name":"Lee","tel":10086},{"name":"Lyle","age":27}]
t2 = pd.DataFrame(d2)
print(d2)
print(t2)
print(type(t2))
运行结果:
name age tel
0 Heiko 24 10086
1 Lee 30 10010
<class 'pandas.core.frame.DataFrame'>
[{'name': 'Heiko', 'age': 24, 'tel': 10086}, {'name': 'Lee', 'tel': 10086}, {'name': 'Lyle', 'age': 27}]
name age tel
0 Heiko 24.0 10086.0
1 Lee NaN 10086.0
2 Lyle 27.0 NaN
DataFrame基础属性和情况查询
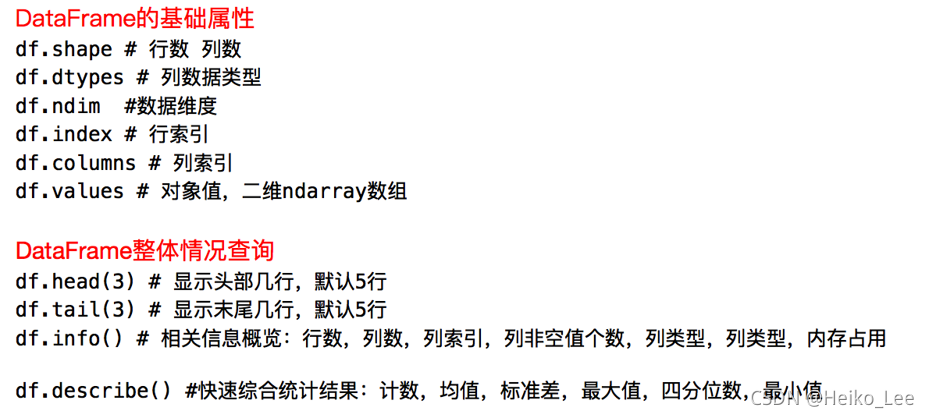
练习:统计读取的狗名字统计的数据中使用次数最高的前几个名字。
import pandas as pd
df = pd.read_csv("./dogNames2.csv")
df = df.sort_values(by="Count_AnimalName",ascending=False)
print(df.head())
运行结果:
Row_Labels Count_AnimalName
1156 BELLA 1195
9140 MAX 1153
2660 CHARLIE 856
3251 COCO 852
12368 ROCKY 823
pandas之取行或者列
pandas取行或者列的注意点
- 方括号写数组,表示取行,对行进行操作
- 写字符串,表示的取列索引,对列进行操作
import pandas as pd
df = pd.read_csv("./dogNames2.csv")
print(df[:10])
print(df["Row_Labels"])
print(type(df["Row_Labels"]))
运行结果:
Row_Labels Count_AnimalName
1156 BELLA 1195
9140 MAX 1153
2660 CHARLIE 856
3251 COCO 852
12368 ROCKY 823
Row_Labels Count_AnimalName
1156 BELLA 1195
9140 MAX 1153
2660 CHARLIE 856
3251 COCO 852
12368 ROCKY 823
8417 LOLA 795
8552 LUCKY 723
8560 LUCY 710
2032 BUDDY 677
3641 DAISY 649
1156 BELLA
9140 MAX
2660 CHARLIE
3251 COCO
12368 ROCKY
...
6884 J-LO
6888 JOANN
6890 JOAO
6891 JOAQUIN
16219 39743
Name: Row_Labels, Length: 16220, dtype: object
<class 'pandas.core.series.Series'>
pandas之loc和iloc
还有更多的经过pandas优化过的选择方式:
- df.loc 通过标签索引行数据
- df.iloc 通过位置获取行数据
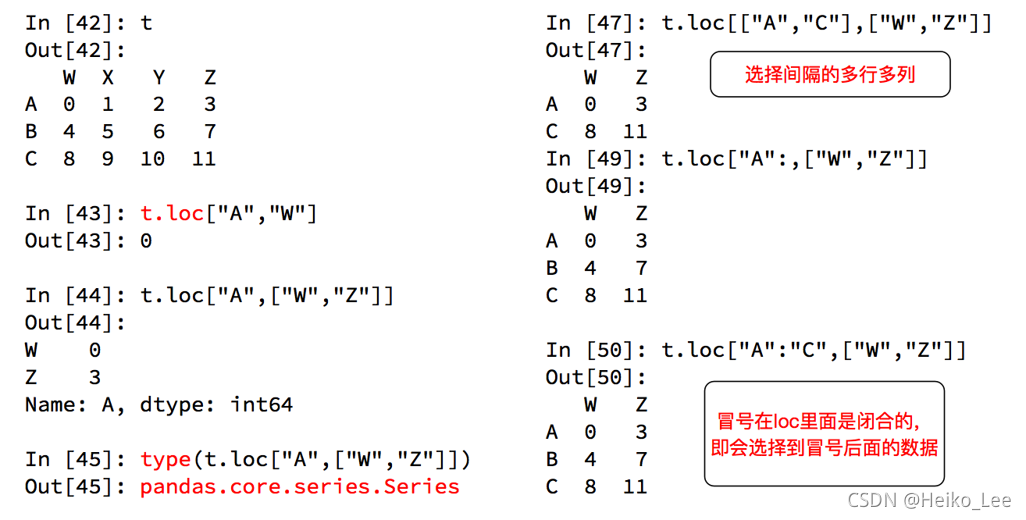

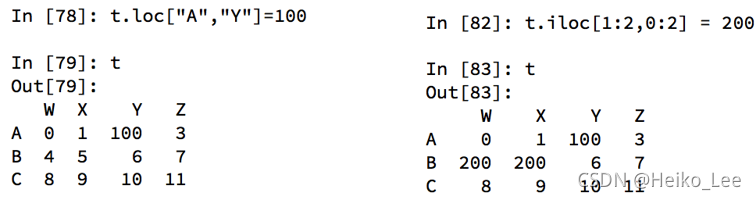
pandas之布尔索引
回到之前狗的名字的问题上,假如我们想找到所有的使用次数超过800的狗的名字,应该怎么选择?
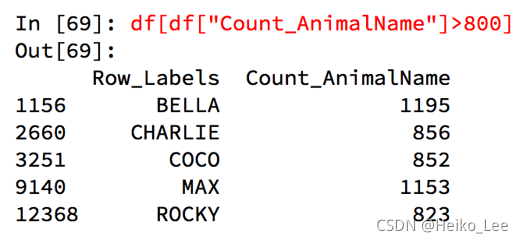
回到之前狗的名字的问题上,假如我们想找到所有的使用次数超过700并且名字的字符串的长度大于4的狗的名字,应该怎么选择?
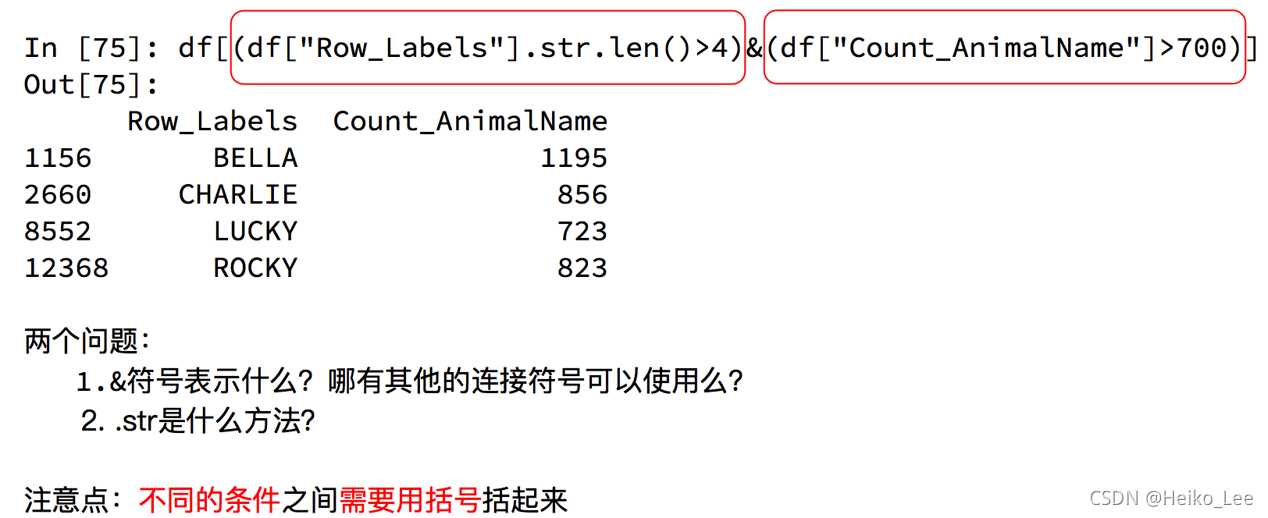
dataFrame中,多个条件需要用逻辑运算符连接。
pandas之字符串方法
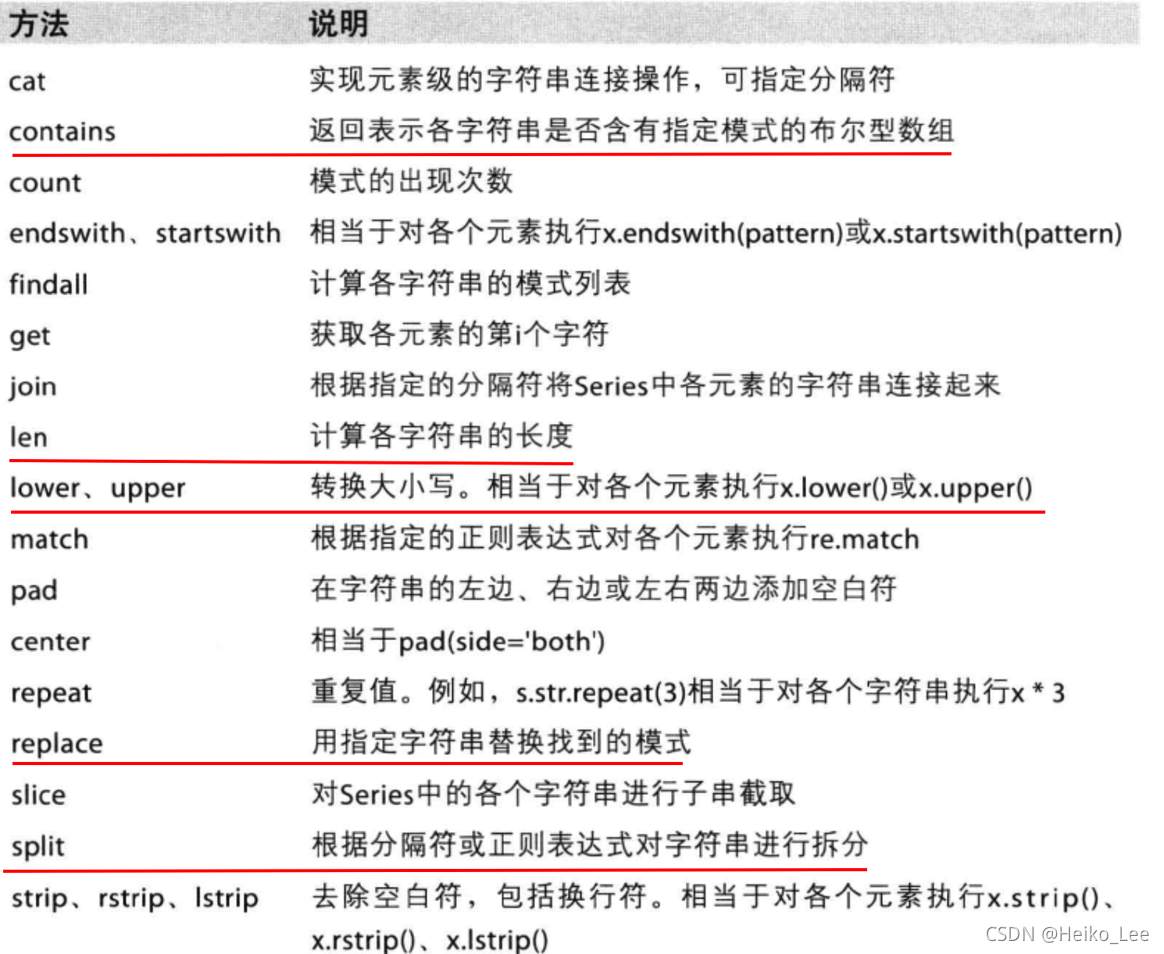
缺失数据的处理
数据缺失通常有两种情况: 一种就是空,None等,在pandas是NaN(和np.nan一样);另一种是我们有意让其为0。
判断数据是否为NaN:
- pd.isnull(df)
- pd.notnull(df)
- 处理方式1:删除NaN所在的行列dropna (axis=0, how=‘any’, inplace=False)
- how=‘any’:删除有nan的行或列;
- how=‘all’:删除全部为nan的行或列;
- inplace:原地修改,省去再赋值自己的步骤。
- 处理方式2:填充数据,
- t.fillna(t.mean()):填充平均值;
- t.fiallna(t.median()):填充中值;
- t.fillna(0):填充0。
处理为0的数据:
t[t==0]=np.nan
当然并不是每次为0的数据都需要处理,计算平均值等情况,nan是不参与计算的,但是0会。
pandas常用统计方法
假设现在我们有一组从2006年到2016年1000部最流行的电影数据,我们想知道这些电影数据中评分的平均分,导演的人数等信息,我们应该怎么获取?
import pandas as pd
file_path = "IMDB-Movie-Data.csv"
df = pd.read_csv(file_path)
print(df.head(1))
print(df["Rating"].mean())
print(len(df["Director"].unique()))
temp_actors_list = df["Actors"].str.split(", ").tolist()
actors_list = [i for j in temp_actors_list for i in j]
actors_num = len(set(actors_list))
print(actors_num)
unique():对于一维数组或者列表,unique函数去除其中重复的元素,并按元素由大到小返回一个新的无元素重复的元组或者列表。
**set() **:set()函数创建一个无序不重复元素集,可进行关系测试,删除重复数据,还可以计算交集、差集、并集等。
运行结果:
Rank Title ... Revenue (Millions) Metascore
0 1 Guardians of the Galaxy ... 333.13 76.0
[1 rows x 12 columns]
6.723200000000003
644
2015
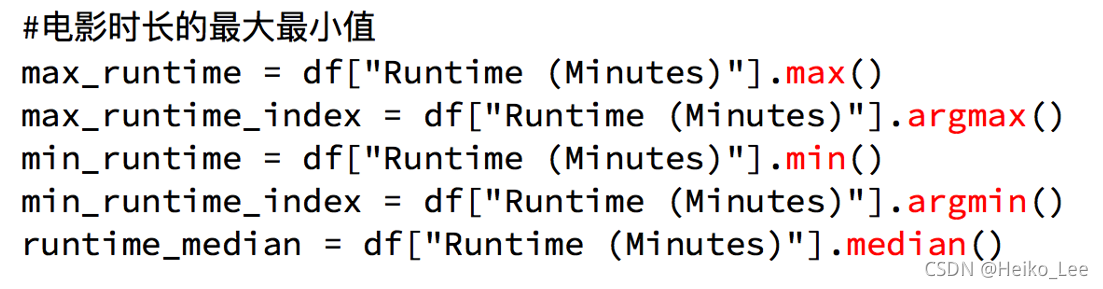
练习:对于这一组电影数据,如果我们想rating,runtime的分布情况,应该如何呈现数据?
Runtime (Minutes):
import pandas as pd
from matplotlib import pyplot as plt
file_path = "./IMDB-Movie-Data.csv"
df = pd.read_csv(file_path)
runtime_data = df["Runtime (Minutes)"].values
print(runtime_data)
max_runtime = runtime_data.max()
min_runtime = runtime_data.min()
print(max_runtime-min_runtime)
num_bin = (max_runtime-min_runtime)//5
plt.figure(figsize=(20,8),dpi=80)
plt.hist(runtime_data,num_bin)
plt.xticks(range(min_runtime,max_runtime+5,5))
plt.grid()
plt.show()
运行结果:
125
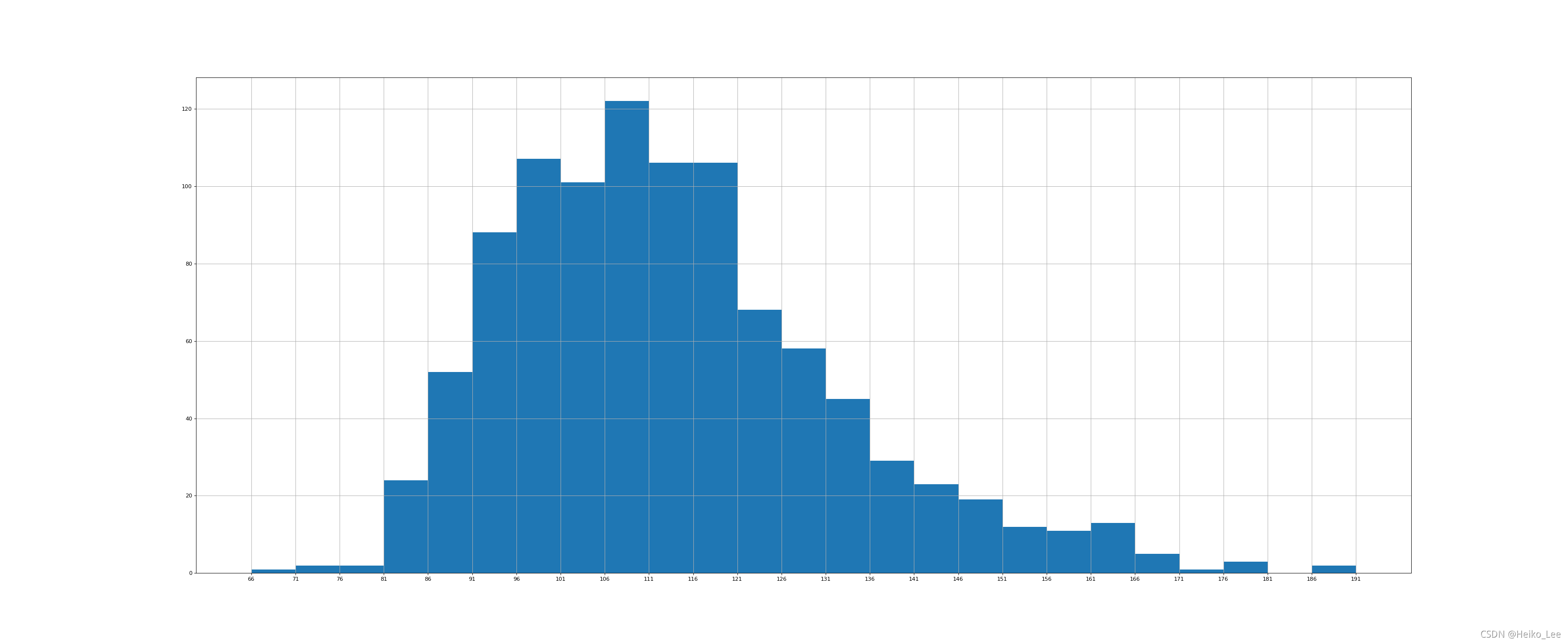
Rating:
import numpy as np
from matplotlib import pyplot as plt
runtime_data = np.array([8.1, 7.0, 7.3, 7.2, 6.2, 6.1, 8.3, 6.4, 7.1, 7.0, 7.5, 7.8, 7.9, 7.7, 6.4, 6.6, 8.2, 6.7, 8.1, 8.0, 6.7, 7.9, 6.7, 6.5, 5.3, 6.8, 8.3, 4.7, 6.2, 5.9, 6.3, 7.5, 7.1, 8.0, 5.6, 7.9, 8.6, 7.6, 6.9, 7.1, 6.3, 7.5, 2.7, 7.2, 6.3, 6.7, 7.3, 5.6, 7.1, 3.7, 8.1, 5.8, 5.6, 7.2, 9.0, 7.3, 7.2, 7.4, 7.0, 7.5, 6.7, 6.8, 6.5, 4.1, 8.5, 7.7, 7.4, 8.1, 7.5, 7.2, 5.9, 7.1, 7.5, 6.8, 8.1, 7.1, 8.1, 8.3, 7.3, 5.3, 8.8, 7.9, 8.2, 8.1, 7.2, 7.0, 6.4, 7.8, 7.8, 7.4, 8.1, 7.0, 8.1, 7.1, 7.4, 7.4, 8.6, 5.8, 6.3, 8.5, 7.0, 7.0, 8.0, 7.9, 7.3, 7.7, 5.4, 6.3, 5.8, 7.7, 6.3, 8.1, 6.1, 7.7, 8.1, 5.8, 6.2, 8.8, 7.2, 7.4, 6.7, 6.7, 6.0, 7.4, 8.5, 7.5, 5.7, 6.6, 6.4, 8.0, 7.3, 6.0, 6.4, 8.5, 7.1, 7.3, 8.1, 7.3, 8.1, 7.1, 8.0, 6.2, 7.8, 8.2, 8.4, 8.1, 7.4, 7.6, 7.6, 6.2, 6.4, 7.2, 5.8, 7.6, 8.1, 4.7, 7.0, 7.4, 7.5, 7.9, 6.0, 7.0, 8.0, 6.1, 8.0, 5.2, 6.5, 7.3, 7.3, 6.8, 7.9, 7.9, 5.2, 8.0, 7.5, 6.5, 7.6, 7.0, 7.4, 7.3, 6.7, 6.8, 7.0, 5.9, 8.0, 6.0, 6.3, 6.6, 7.8, 6.3, 7.2, 5.6, 8.1, 5.8, 8.2, 6.9, 6.3, 8.1, 8.1, 6.3, 7.9, 6.5, 7.3, 7.9, 5.7, 7.8, 7.5, 7.5, 6.8, 6.7, 6.1, 5.3, 7.1, 5.8, 7.0, 5.5, 7.8, 5.7, 6.1, 7.7, 6.7, 7.1, 6.9, 7.8, 7.0, 7.0, 7.1, 6.4, 7.0, 4.8, 8.2, 5.2, 7.8, 7.4, 6.1, 8.0, 6.8, 3.9, 8.1, 5.9, 7.6, 8.2, 5.8, 6.5, 5.9, 7.6, 7.9, 7.4, 7.1, 8.6, 4.9, 7.3, 7.9, 6.7, 7.5, 7.8, 5.8, 7.6, 6.4, 7.1, 7.8, 8.0, 6.2, 7.0, 6.0, 4.9, 6.0, 7.5, 6.7, 3.7, 7.8, 7.9, 7.2, 8.0, 6.8, 7.0, 7.1, 7.7, 7.0, 7.2, 7.3, 7.6, 7.1, 7.0, 6.0, 6.1, 5.8, 5.3, 5.8, 6.1, 7.5, 7.2, 5.7, 7.7, 7.1, 6.6, 5.7, 6.8, 7.1, 8.1, 7.2, 7.5, 7.0, 5.5, 6.4, 6.7, 6.2, 5.5, 6.0, 6.1, 7.7, 7.8, 6.8, 7.4, 7.5, 7.0, 5.2, 5.3, 6.2, 7.3, 6.5, 6.4, 7.3, 6.7, 7.7, 6.0, 6.0, 7.4, 7.0, 5.4, 6.9, 7.3, 8.0, 7.4, 8.1, 6.1, 7.8, 5.9, 7.8, 6.5, 6.6, 7.4, 6.4, 6.8, 6.2, 5.8, 7.7, 7.3, 5.1, 7.7, 7.3, 6.6, 7.1, 6.7, 6.3, 5.5, 7.4, 7.7, 6.6, 7.8, 6.9, 5.7, 7.8, 7.7, 6.3, 8.0, 5.5, 6.9, 7.0, 5.7, 6.0, 6.8, 6.3, 6.7, 6.9, 5.7, 6.9, 7.6, 7.1, 6.1, 7.6, 7.4, 6.6, 7.6, 7.8, 7.1, 5.6, 6.7, 6.7, 6.6, 6.3, 5.8, 7.2, 5.0, 5.4, 7.2, 6.8, 5.5, 6.0, 6.1, 6.4, 3.9, 7.1, 7.7, 6.7, 6.7, 7.4, 7.8, 6.6, 6.1, 7.8, 6.5, 7.3, 7.2, 5.6, 5.4, 6.9, 7.8, 7.7, 7.2, 6.8, 5.7, 5.8, 6.2, 5.9, 7.8, 6.5, 8.1, 5.2, 6.0, 8.4, 4.7, 7.0, 7.4, 6.4, 7.1, 7.1, 7.6, 6.6, 5.6, 6.3, 7.5, 7.7, 7.4, 6.0, 6.6, 7.1, 7.9, 7.8, 5.9, 7.0, 7.0, 6.8, 6.5, 6.1, 8.3, 6.7, 6.0, 6.4, 7.3, 7.6, 6.0, 6.6, 7.5, 6.3, 7.5, 6.4, 6.9, 8.0, 6.7, 7.8, 6.4, 5.8, 7.5, 7.7, 7.4, 8.5, 5.7, 8.3, 6.7, 7.2, 6.5, 6.3, 7.7, 6.3, 7.8, 6.7, 6.7, 6.6, 8.0, 6.5, 6.9, 7.0, 5.3, 6.3, 7.2, 6.8, 7.1, 7.4, 8.3, 6.3, 7.2, 6.5, 7.3, 7.9, 5.7, 6.5, 7.7, 4.3, 7.8, 7.8, 7.2, 5.0, 7.1, 5.7, 7.1, 6.0, 6.9, 7.9, 6.2, 7.2, 5.3, 4.7, 6.6, 7.0, 3.9, 6.6, 5.4, 6.4, 6.7, 6.9, 5.4, 7.0, 6.4, 7.2, 6.5, 7.0, 5.7, 7.3, 6.1, 7.2, 7.4, 6.3, 7.1, 5.7, 6.7, 6.8, 6.5, 6.8, 7.9, 5.8, 7.1, 4.3, 6.3, 7.1, 4.6, 7.1, 6.3, 6.9, 6.6, 6.5, 6.5, 6.8, 7.8, 6.1, 5.8, 6.3, 7.5, 6.1, 6.5, 6.0, 7.1, 7.1, 7.8, 6.8, 5.8, 6.8, 6.8, 7.6, 6.3, 4.9, 4.2, 5.1, 5.7, 7.6, 5.2, 7.2, 6.0, 7.3, 7.2, 7.8, 6.2, 7.1, 6.4, 6.1, 7.2, 6.6, 6.2, 7.9, 7.3, 6.7, 6.4, 6.4, 7.2, 5.1, 7.4, 7.2, 6.9, 8.1, 7.0, 6.2, 7.6, 6.7, 7.5, 6.6, 6.3, 4.0, 6.9, 6.3, 7.3, 7.3, 6.4, 6.6, 5.6, 6.0, 6.3, 6.7, 6.0, 6.1, 6.2, 6.7, 6.6, 7.0, 4.9, 8.4, 7.0, 7.5, 7.3, 5.6, 6.7, 8.0, 8.1, 4.8, 7.5, 5.5, 8.2, 6.6, 3.2, 5.3, 5.6, 7.4, 6.4, 6.8, 6.7, 6.4, 7.0, 7.9, 5.9, 7.7, 6.7, 7.0, 6.9, 7.7, 6.6, 7.1, 6.6, 5.7, 6.3, 6.5, 8.0, 6.1, 6.5, 7.6, 5.6, 5.9, 7.2, 6.7, 7.2, 6.5, 7.2, 6.7, 7.5, 6.5, 5.9, 7.7, 8.0, 7.6, 6.1, 8.3, 7.1, 5.4, 7.8, 6.5, 5.5, 7.9, 8.1, 6.1, 7.3, 7.2, 5.5, 6.5, 7.0, 7.1, 6.6, 6.5, 5.8, 7.1, 6.5, 7.4, 6.2, 6.0, 7.6, 7.3, 8.2, 5.8, 6.5, 6.6, 6.2, 5.8, 6.4, 6.7, 7.1, 6.0, 5.1, 6.2, 6.2, 6.6, 7.6, 6.8, 6.7, 6.3, 7.0, 6.9, 6.6, 7.7, 7.5, 5.6, 7.1, 5.7, 5.2, 5.4, 6.6, 8.2, 7.6, 6.2, 6.1, 4.6, 5.7, 6.1, 5.9, 7.2, 6.5, 7.9, 6.3, 5.0, 7.3, 5.2, 6.6, 5.2, 7.8, 7.5, 7.3, 7.3, 6.6, 5.7, 8.2, 6.7, 6.2, 6.3, 5.7, 6.6, 4.5, 8.1, 5.6, 7.3, 6.2, 5.1, 4.7, 4.8, 7.2, 6.9, 6.5, 7.3, 6.5, 6.9, 7.8, 6.8, 4.6, 6.7, 6.4, 6.0, 6.3, 6.6, 7.8, 6.6, 6.2, 7.3, 7.4, 6.5, 7.0, 4.3, 7.2, 6.2, 6.2, 6.8, 6.0, 6.6, 7.1, 6.8, 5.2, 6.7, 6.2, 7.0, 6.3, 7.8, 7.6, 5.4, 7.6, 5.4, 4.6, 6.9, 6.8, 5.8, 7.0, 5.8, 5.3, 4.6, 5.3, 7.6, 1.9, 7.2, 6.4, 7.4, 5.7, 6.4, 6.3, 7.5, 5.5, 4.2, 7.8, 6.3, 6.4, 7.1, 7.1, 6.8, 7.3, 6.7, 7.8, 6.3, 7.5, 6.8, 7.4, 6.8, 7.1, 7.6, 5.9, 6.6, 7.5, 6.4, 7.8, 7.2, 8.4, 6.2, 7.1, 6.3, 6.5, 6.9, 6.9, 6.6, 6.9, 7.7, 2.7, 5.4, 7.0, 6.6, 7.0, 6.9, 7.3, 5.8, 5.8, 6.9, 7.5, 6.3, 6.9, 6.1, 7.5, 6.8, 6.5, 5.5, 7.7, 3.5, 6.2, 7.1, 5.5, 7.1, 7.1, 7.1, 7.9, 6.5, 5.5, 6.5, 5.6, 6.8, 7.9, 6.2, 6.2, 6.7, 6.9, 6.5, 6.6, 6.4, 4.7, 7.2, 7.2, 6.7, 7.5, 6.6, 6.7, 7.5, 6.1, 6.4, 6.3, 6.4, 6.8, 6.1, 4.9, 7.3, 5.9, 6.1, 7.1, 5.9, 6.8, 5.4, 6.3, 6.2, 6.6, 4.4, 6.8, 7.3, 7.4, 6.1, 4.9, 5.8, 6.1, 6.4, 6.9, 7.2, 5.6, 4.9, 6.1, 7.8, 7.3, 4.3, 7.2, 6.4, 6.2, 5.2, 7.7, 6.2, 7.8, 7.0, 5.9, 6.7, 6.3, 6.9, 7.0, 6.7, 7.3, 3.5, 6.5, 4.8, 6.9, 5.9, 6.2, 7.4, 6.0, 6.2, 5.0, 7.0, 7.6, 7.0, 5.3, 7.4, 6.5, 6.8, 5.6, 5.9, 6.3, 7.1, 7.5, 6.6, 8.5, 6.3, 5.9, 6.7, 6.2, 5.5, 6.2, 5.6, 5.3])
max_runtime = runtime_data.max()
min_runtime = runtime_data.min()
print(min_runtime,max_runtime)
num_bin_list = [1.9,3.5]
i=3.5
while i<=max_runtime:
i += 0.5
num_bin_list.append(i)
print(num_bin_list)
plt.figure(figsize=(20,8),dpi=80)
plt.hist(runtime_data,num_bin_list)
plt.xticks(num_bin_list)
plt.show()
运行结果:
1.9 9.0
[1.9, 3.5, 4.0, 4.5, 5.0, 5.5, 6.0, 6.5, 7.0, 7.5, 8.0, 8.5, 9.0, 9.5]
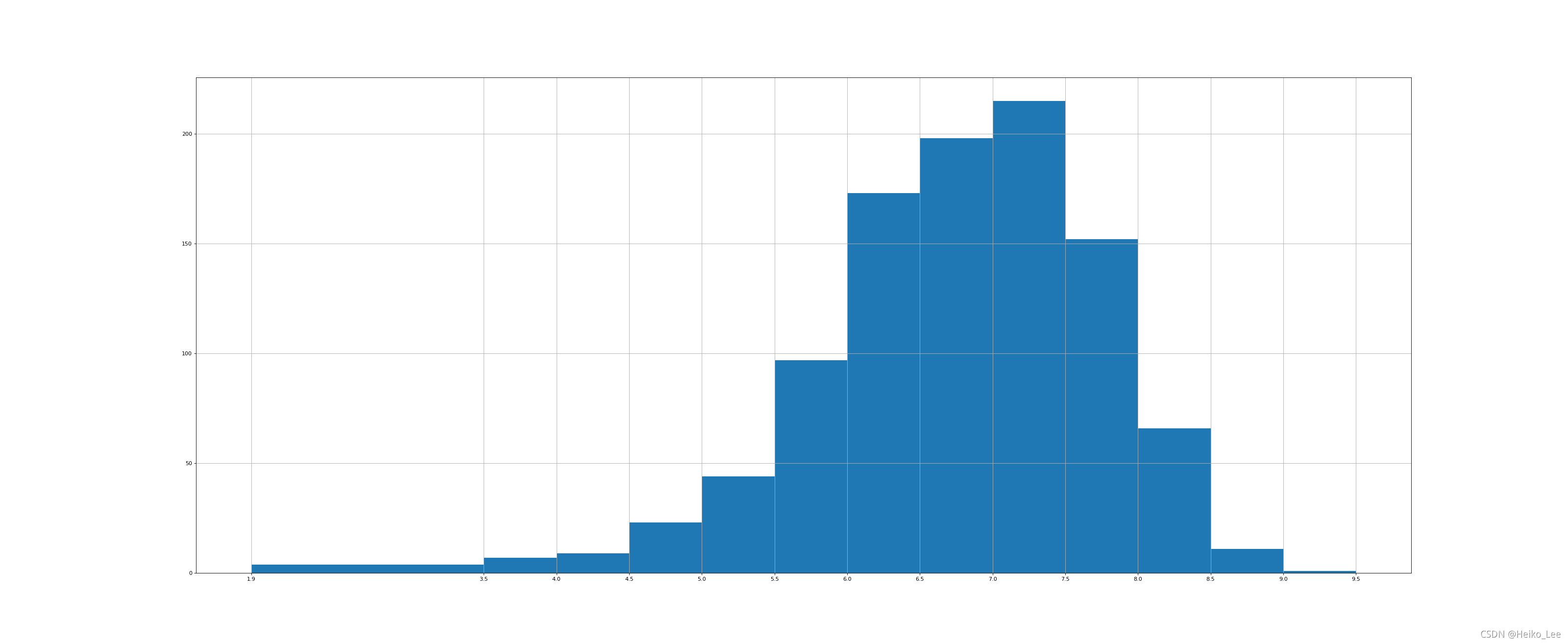
|