#导入所需模块
import matplotlib.pyplot as plt
import numpy as np
from sklearn.cluster import KMeans
from sklearn.datasets import load_iris
#导入鸢尾花数据集
iris = load_iris()
X = iris.data[:]
# print(X)
print(X.shape)
#肘方法看k值
d=[]
for i in range(1,11): #k取值1~11,做kmeans聚类,看不同k值对应的簇内误差平方和
km=KMeans(n_clusters=i,init='k-means++',n_init=10,max_iter=300,random_state=0)
km.fit(X)
d.append(km.inertia_) #inertia簇内误差平方和
plt.plot(range(1,11),d,marker='o')
plt.xlabel('number of clusters')
plt.ylabel('distortions')
plt.show()
#建立簇为3的聚类器
estimator = KMeans(n_clusters=3,random_state=0)
estimator.fit(X)
#获取标签
label_pred = estimator.labels_
print(label_pred)
x0 = X[label_pred==0]
x1 = X[label_pred==1]
x2 = X[label_pred==2]
#绘制聚类结果
plt.scatter(x0[:,0],x0[:,2],c='red',marker='o',label='label0')
plt.scatter(x1[:,0],x1[:,2],c='green',marker='*',label='label1')
plt.scatter(x2[:,0],x2[:,2],c='blue',marker='v',label='label2')
centers = estimator.cluster_centers_
plt.scatter(centers[:,0],centers[:,2],marker='x',c='black',alpha=1,s=300)
plt.xlabel('petal length')
plt.ylabel('petal width')
plt.legend(loc=2)
plt.show()
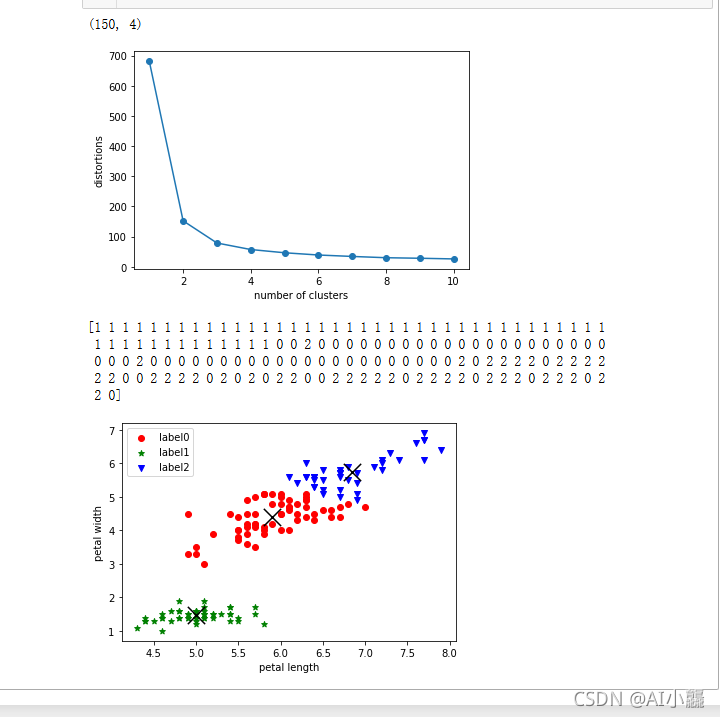
敬请指正!
|