【人工智能项目】深度学习实现白葡萄酒品质预测
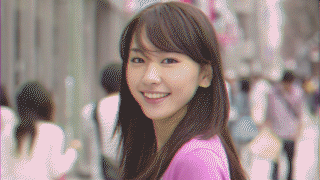
任务介绍
评价一款葡萄酒时不外乎从颜色、酸度、甜度、香气、风味等入手,而决定这些就是葡萄酒的挥发酸度、糖分、密度等。
根据给出的白葡萄酒酸度、糖分、PH值、柠檬酸等数据,判断葡萄酒品质。
导入数据
import numpy as np
import pandas as pd
import warnings
warnings.filterwarnings("ignore")
train_data = pd.read_csv("./winequality_dataset/train.csv",header=0,index_col=None)
train_data.head()

EDA
train_data.info()
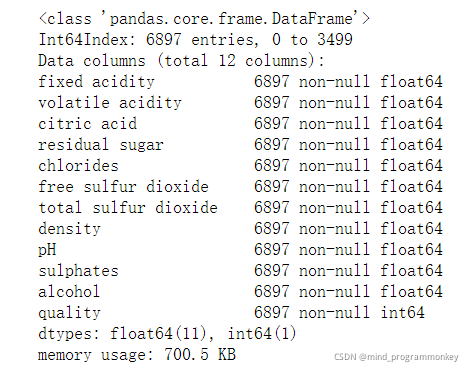
train_data.isnull().sum()
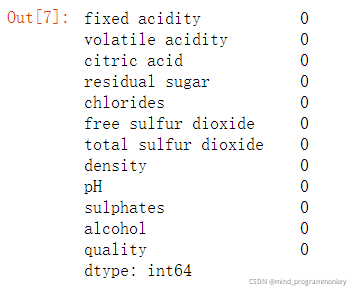
import matplotlib.pyplot as plt
%matplotlib inline
fig = plt.figure()
ax = fig.add_subplot(111)
ax.set(xlabel="total sulfur dioxide",ylabel="free sulfur dioxide")
ax.scatter(train_data["total sulfur dioxide"],train_data["free sulfur dioxide"],c="r")
plt.show()
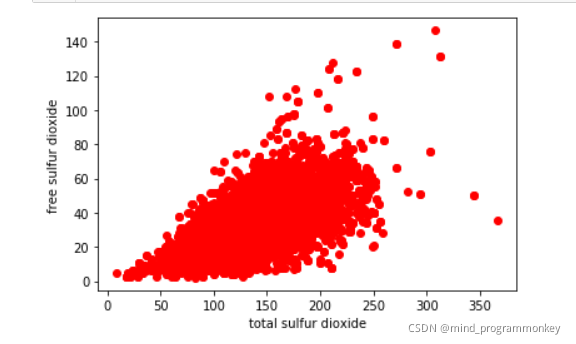
数据集划分
from sklearn.model_selection import train_test_split
X = train_data.iloc[:,:-1]
y = np.ravel(train_data.quality)
X_train,X_test,y_train,y_test = train_test_split(X,y,test_size=0.2,random_state=2019)
X.head()

y
print(X_train.shape)
print(y_train.shape)
print(X_test.shape)
print(y_test.shape)
(5517, 11) (5517,) (1380, 11) (1380,)
数据预处理
from sklearn.preprocessing import PolynomialFeatures
print("Shape of X_train before transformation:",X_train.shape)
poly = PolynomialFeatures(degree=2,include_bias=False)
X_train_poly = poly.fit_transform(X_train)
X_test_poly = poly.transform(X_test)
X_poly = poly.transform(X)
print("Shape of X_train after transformation:",X_train_poly.shape)
Shape of X_train before transformation: (3200, 11)
Shape of X_train after transformation: (3200, 77
from sklearn.preprocessing import StandardScaler
from sklearn.preprocessing import MinMaxScaler
scaler = MinMaxScaler().fit(X_train_poly)
X_train = scaler.transform(X_train_poly)
X_test = scaler.transform(X_test_poly)
X = scaler.transform(X_poly)
数据归一化处理
from sklearn.preprocessing import StandardScaler
from sklearn.preprocessing import MinMaxScaler
scaler = MinMaxScaler().fit(X_train)
X_train = scaler.transform(X_train)
X_test = scaler.transform(X_test)
X = scaler.transform(X)
ML模型
from sklearn.preprocessing import StandardScaler,RobustScaler
from sklearn.model_selection import train_test_split
from sklearn.model_selection import KFold
from sklearn.model_selection import cross_val_score
from sklearn.linear_model import LogisticRegression
from sklearn.naive_bayes import GaussianNB
from sklearn.neighbors import KNeighborsClassifier
from sklearn.tree import DecisionTreeClassifier
from sklearn.svm import SVC
from sklearn.discriminant_analysis import LinearDiscriminantAnalysis
from catboost import CatBoostClassifier
from sklearn.metrics import accuracy_score,confusion_matrix,classification_report
def get_models():
models = []
models.append(("LR",LogisticRegression()))
models.append(("NB",GaussianNB()))
models.append(("KNN",KNeighborsClassifier()))
models.append(("DT",DecisionTreeClassifier()))
models.append(("SVM rbf",SVC()))
models.append(("SVM linear",SVC(kernel="linear")))
models.append(("LDA",LinearDiscriminantAnalysis()))
models.append(("Cat",CatBoostClassifier(silent=True)))
return models
def cross_validation_scores_for_various_ml_models(X_cv,y_cv):
print("cross validation accuracy".upper())
models = get_models()
results = []
names = []
for name,model in models:
kfold = KFold(n_splits=5,shuffle=True,random_state=2019)
cv_result = cross_val_score(model,X_cv,y_cv,cv=kfold,scoring="accuracy")
names.append(name)
results.append(cv_result)
print("{}cross validation,accuracy:{:0.2f}".format(name,cv_result.mean()))
cross_validation_scores_for_various_ml_models(X,y)
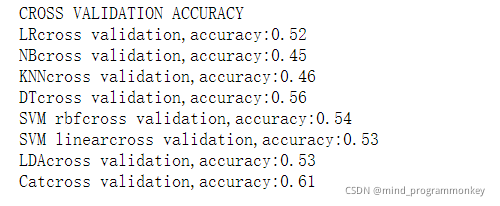 Random Forest
from sklearn.model_selection import train_test_split,cross_val_score,GridSearchCV
from sklearn.metrics import mean_absolute_error,classification_report
from sklearn.ensemble import RandomForestClassifier,RandomForestRegressor
from xgboost import XGBRegressor
scores = {}
for n_estimators in range(10,810,10):
RF_model = RandomForestClassifier(n_estimators=n_estimators,random_state=2019)
RF_model.fit(X_train,y_train)
RF_predictions = RF_model.predict(X_test)
RF_mae = mean_absolute_error(RF_predictions,y_test)
scores[n_estimators] = RF_mae
import matplotlib.pyplot as plt
%matplotlib inline
fig_RF,ax_RF = plt.subplots(figsize=(10,4))
ax_RF.set_title("Mean Absolute Error with Number of Estimators of a Random Forest")
ax_RF.set_xlabel("Number of Estimators")
ax_RF.set_ylabel("Mean Absolute Error")
plt.plot(list(scores.keys()),list(scores.values()))
best_n_estimators = 0
for n_estimators,score in scores.items():
if score == min(scores.values()):
best_n_estimators = n_estimators
print(f"Best Number of Estimators:{n_estimators}")
RF_model = RandomForestClassifier(n_estimators=best_n_estimators,random_state=2019)
RF_model.fit(X_train,y_train)
RF_predictions = RF_model.predict(X_test)
RF_mae = mean_absolute_error(RF_predictions,y_test)
print(f"Mean Absolute Error:{RF_mae}")
print(classification_report(y_test,RF_predictions))
from sklearn.model_selection import GridSearchCV
param_grid = {"n_estimators":[120,140,300,500,800,1200]}
RF_model_new = RandomForestClassifier(random_state=2019)
RF_grid = GridSearchCV(RF_model_new,param_grid,verbose=1,n_jobs=-1,cv=3,scoring="neg_mean_absolute_error")
RF_grid.fit(X_train,y_train)
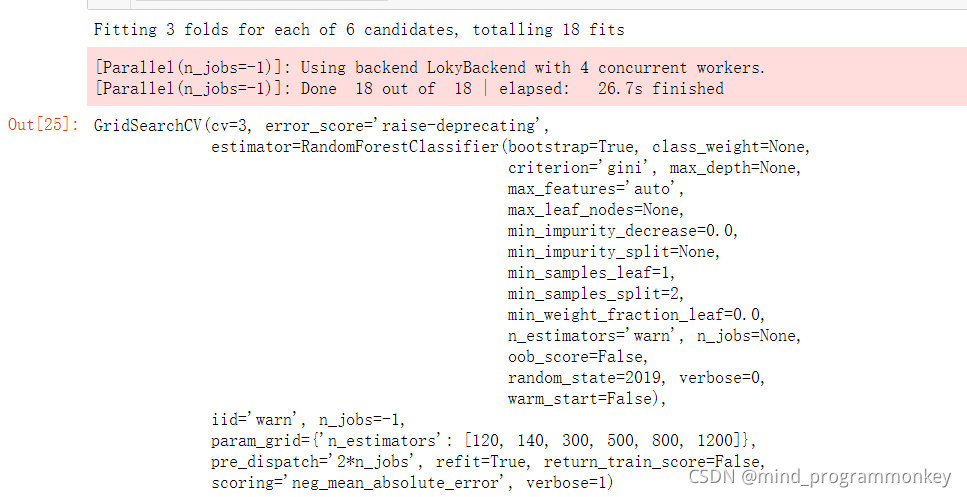
RF_grid.best_params_
RF_model = RandomForestClassifier(n_estimators=140,random_state=2019)
RF_model.fit(X_train,y_train)
RF_predictions = RF_model.predict(X_test)
RF_mae = mean_absolute_error(RF_predictions,y_test)
print(f"Mean Absolute Error:{RF_mae}")
print(classification_report(y_test,RF_predictions))
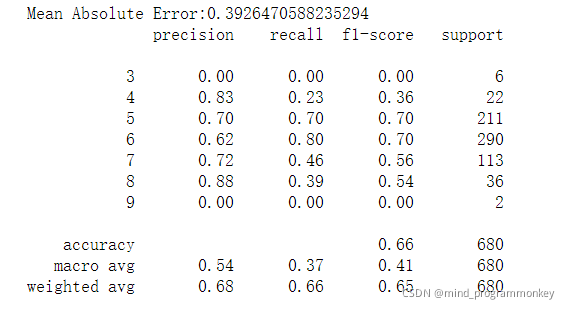 ExtraTreeClassifier
from sklearn.ensemble import ExtraTreesClassifier
from sklearn.ensemble import ExtraTreesRegressor
scores = {}
for n_estimators in range(10,600,10):
extra_model = ExtraTreesClassifier(n_estimators=n_estimators,random_state=2019)
extra_model.fit(X_train,y_train)
extra_predictions = extra_model.predict(X_test)
extra_mae = mean_absolute_error(extra_predictions,y_test)
scores[n_estimators] = extra_mae
import matplotlib.pyplot as plt
%matplotlib inline
fig_RF,ax_RF = plt.subplots(figsize=(10,4))
ax_RF.set_title("Mean Absolute Error with Number of Estimators of a Random Forest")
ax_RF.set_xlabel("Number of Estimators")
ax_RF.set_ylabel("Mean Absolute Error")
plt.plot(list(scores.keys()),list(scores.values()))
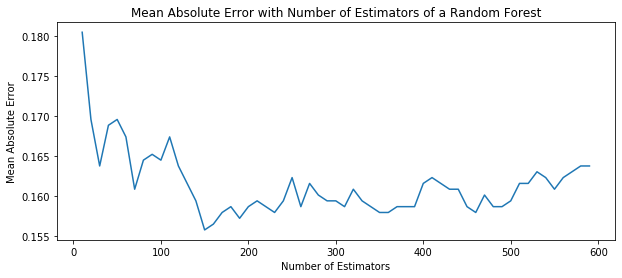
best_n_estimators = 0
for n_estimators,score in scores.items():
if score == min(scores.values()):
best_n_estimators = n_estimators
print(f"Best Number of Estimators:{n_estimators}")
Best Number of Estimators:150
extra_classifier = ExtraTreesClassifier(n_estimators=530,random_state=2019)
extra_classifier = extra_classifier.fit(X_train,y_train)
extra_classifier_prediction = extra_classifier.predict(X_test)
extra_mae = mean_absolute_error(extra_classifier_prediction,y_test)
print(f"Mean Absolute Error:{extra_mae}")
Mean Absolute Error:0.16304347826086957
Ensemble K-Nearest Neighbors
from sklearn.model_selection import GridSearchCV
from sklearn.model_selection import RandomizedSearchCV
import scipy.stats as st
from sklearn.neighbors import KNeighborsClassifier
param_grid = {"n_neighbors":st.randint(1,40),
"weights":["uniform","distance"]}
KN_model = KNeighborsClassifier()
KN_grid = RandomizedSearchCV(KN_model,param_grid,verbose=1,n_jobs=-1,cv=3)
KN_grid.fit(X_train,y_train)
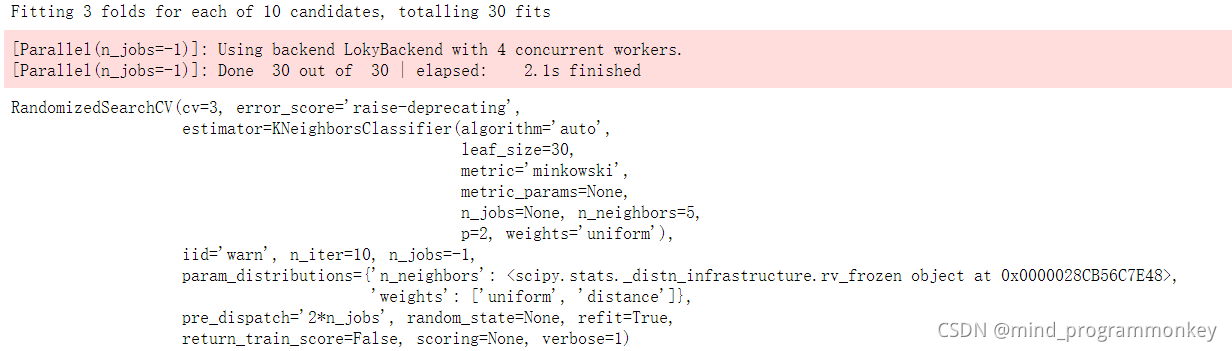
print(KN_grid.best_params_)
{‘n_neighbors’: 32, ‘weights’: ‘distance’}
KN_model = KNeighborsClassifier(n_neighbors=30,weights="distance")
KN_model.fit(X_train,y_train)
KN_predictions = KN_model.predict(X_test)
KN_mae = mean_absolute_error(KN_predictions,y_test)
print(f"Mean Absolute Error:{KN_mae}")
Mean Absolute Error:0.5441176470588235
Logistic Regression
from sklearn.linear_model import LogisticRegression
logistic_regression = LogisticRegression()
logistic_regression.fit(X_train,y_train)
logistic_prediction = logistic_regression.predict(X_test)
logistic_mae = mean_absolute_error(logistic_prediction,y_test)
print(f"Mean Absolute Error:{logistic_mae}")
Mean Absolute Error:0.5970588235294118
LinearRegression
from sklearn.linear_model import LinearRegression
lin_regression = LinearRegression()
lin_regression.fit(X_train,y_train)
lin_prediction = lin_regression.predict(X_test)
lin_mae = mean_absolute_error(lin_prediction,y_test)
print(f"Mean Absolute Error:{lin_mae}")
Mean Absolute Error:0.6353137915187634
ElasticNet
from sklearn.linear_model import ElasticNet
ela_regression = ElasticNet()
ela_regression.fit(X_train,y_train)
els_prediction = ela_regression.predict(X_test)
ela_mae = mean_absolute_error(els_prediction,y_test)
print(f"Mean Absolute Error:{ela_mae}")
Mean Absolute Error:0.698948525093073
PolynomialFeatures
from sklearn.preprocessing import PolynomialFeatures
from sklearn.linear_model import LinearRegression
poly_features = PolynomialFeatures(degree=5,include_bias=False)
X_train_poly = poly_features.fit_transform(X_train)
X_test_poly = poly_features.transform(X_test)
poly_reg = LinearRegression()
poly_reg.fit(X_train_poly,y_train)
poly_reg_prediction = poly_reg.predict(X_test_poly)
poly_reg_mae = mean_absolute_error(poly_reg_prediction,y_test)
print(f"Mean Absolute Error:{poly_reg_mae}")
Mean Absolute Error:19.971436771294478
DL模型
from keras.models import Sequential
from keras.layers import Dense,Dropout
model = Sequential()
model.add(Dense(32,activation="relu"))
model.add(Dropout(0.2))
model.add(Dense(16,activation="relu"))
model.add(Dropout(0.2))
model.add(Dense(1))
from keras.callbacks import EarlyStopping,ReduceLROnPlateau,ModelCheckpoint,LearningRateScheduler
checkpoint = ModelCheckpoint("dl.h5",
monitor="val_loss",
mode="min",
save_best_only = True,
verbose=1)
earlystop = EarlyStopping(monitor = 'val_loss',
min_delta = 0,
patience = 5,
verbose = 1,
restore_best_weights = True)
reduce_lr = ReduceLROnPlateau(monitor = 'val_loss',
factor = 0.2,
patience = 3,
verbose = 1)
callbacks = [earlystop, checkpoint, reduce_lr]
model.compile(loss="mae",optimizer="adam",metrics=["mae"])
model.fit(X_train,y_train,epochs=100,batch_size=1,verbose=1,validation_data=(X_test,y_test),callbacks=callbacks)
|