1.神经网络模型的搭建
定义一个基础的神经网络架构,同时新建一个net=Net(1, 100, 100, 10, 1) ,包含三个隐藏层的神经网络:
import torch
import os
os.environ["KMP_DUPLICATE_LIB_OK"] = "TRUE"
class Net(torch.nn.Module):
def __init__(self, n_feature, n_hidden, n_hidden1, n_hidden2, n_output):
super(Net, self).__init__()
self.hidden0 = torch.nn.Linear(n_feature, n_hidden)
self.hidden1 = torch.nn.Linear(n_hidden, n_hidden1)
self.hidden2 = torch.nn.Linear(n_hidden1, n_hidden2)
self.predict = torch.nn.Linear(n_hidden2, n_output)
def forward(self, x):
x = torch.relu(self.hidden0(x))
x = torch.relu(self.hidden1(x))
x = torch.relu(self.hidden2(x))
x = self.predict(x)
return x
net = Net(1, 100, 100, 10, 1)
print(net)
输出结果为:
Net(
(hidden0): Linear(in_features=1, out_features=100, bias=True)
(hidden1): Linear(in_features=100, out_features=100, bias=True)
(hidden2): Linear(in_features=100, out_features=100, bias=True)
(predict): Linear(in_features=100, out_features=1, bias=True)
)
这里可以清楚的看到所搭建的神经网络的架构细节
2.神经网络实现非线性回归
import torch
import matplotlib.pyplot as plt
import os
os.environ["KMP_DUPLICATE_LIB_OK"] = "TRUE"
x = torch.unsqueeze(torch.linspace(-3, 5, 421), dim=1)
y = 0.3 * x.pow(2) + 0.2 * torch.rand(x.size())
class Net(torch.nn.Module):
def __init__(self, n_feature, n_hidden, n_output):
super(Net, self).__init__()
self.hidden = torch.nn.Linear(n_feature, n_hidden)
self.predict = torch.nn.Linear(n_hidden, n_output)
def forward(self, x):
x = torch.relu(self.hidden(x))
x = self.predict(x)
return x
net = Net(1, 100, 1)
plt.ion()
plt.show()
optimizer = torch.optim.Adam(net.parameters(), lr=0.1)
loss_func = torch.nn.MSELoss()
for t in range(200):
prediction = net(x)
loss = loss_func(prediction, y)
optimizer.zero_grad()
loss.backward()
optimizer.step()
if t % 5 == 0:
plt.cla()
plt.scatter(x, y)
plt.plot(x, prediction.data.numpy(), 'r-', lw=3)
plt.text(0.5, 0, 'Loss=%.4f' % loss.item(), fontdict={'size': 20, 'color': 'red'})
plt.pause(0.1)
plt.ioff()
plt.show()
2.结果展示
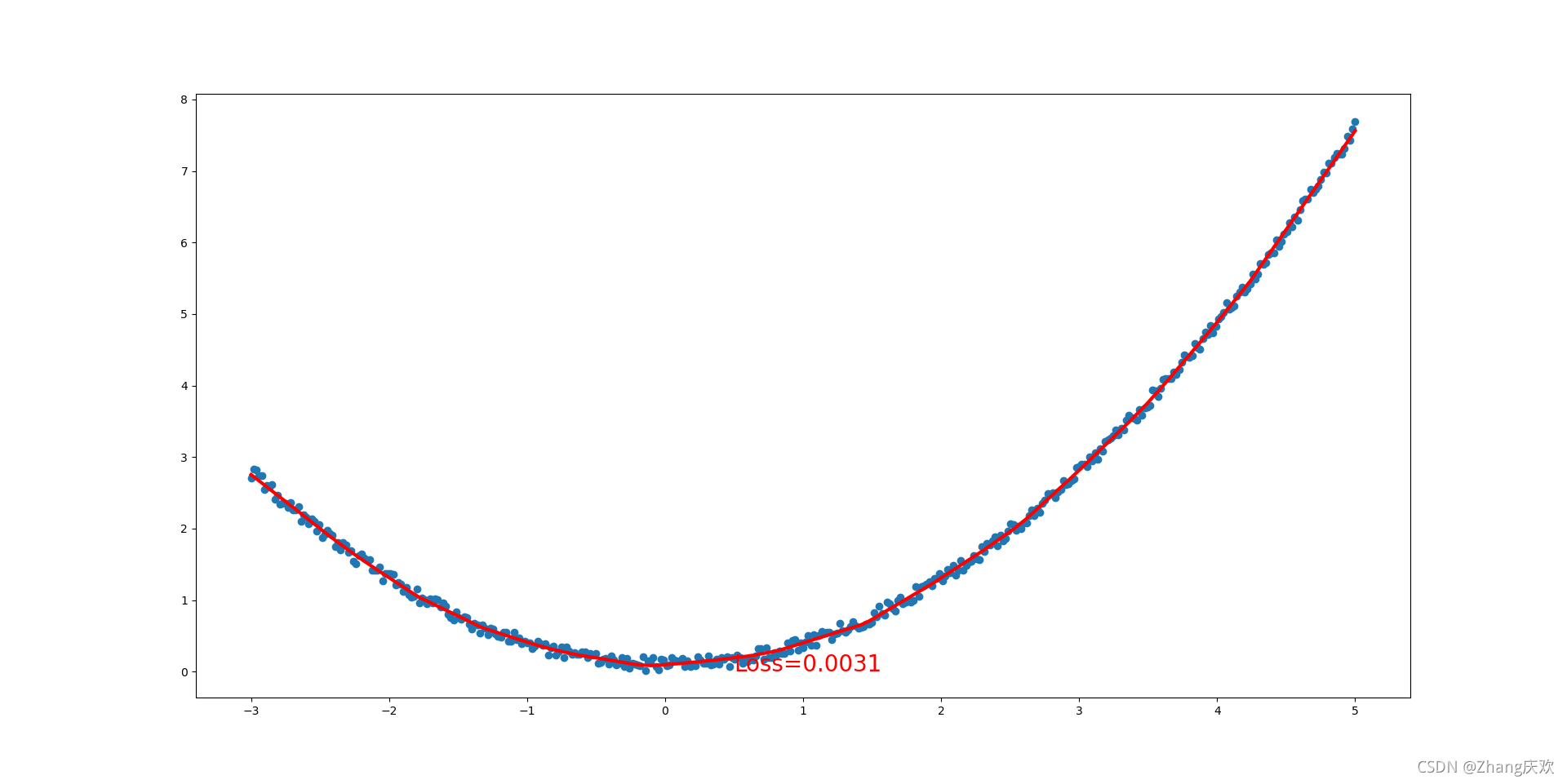
3.关键代码解析
1.代码:
import os
os.environ["KMP_DUPLICATE_LIB_OK"] = "TRUE"
是为了解决下面的问题
OMP: Error
2.代码:
x = torch.unsqueeze(torch.linspace(-3, 5, 421), dim=1)
y = 0.3 * x.pow(2) + 0.2 * torch.rand(x.size())
产生数据,x和y
|