视频地址:B站 刘二大人 传送门
1:线性层的作用:把某一维度映射到另一个维度 2:代码说明:
input_size //输入维度
hidden_size //隐层维度
tanh //激活函数,取值在[-1,1]之间
batch_size //批量
seqLen //序列长度
self.rnncell=torch.nn.RNNCell(input_size=self.input_size,
hidden_size=self.hidden_size)
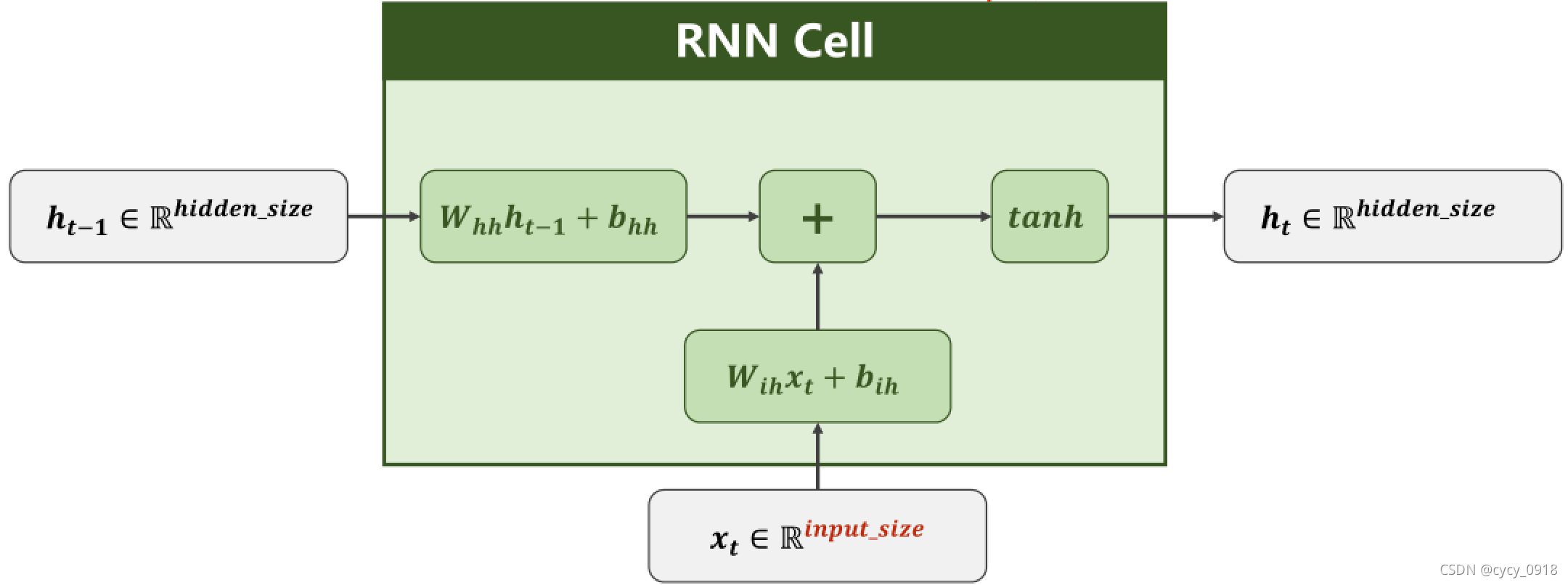
use RNNCell:
初始化参数:
batch_size=1
seq_len=3
input_size=4
hidden_size=2
构造RNNCell:
cell=torch.nn.RNNCell(input_size=input_size,hidden_size=hidden_size)
初始化隐层h0:
dataset=torch.randn(seq_len,batch_size,input_size)
hidden=torch.zeros(batch_size,hidden_size)
全部代码:
import torch
batch_size=1
seq_len=3
input_size=4
hidden_size=2
cell=torch.nn.RNNCell(input_size=input_size,hidden_size=hidden_size)
dataset=torch.randn(seq_len,batch_size,input_size)
hidden=torch.zeros(batch_size,hidden_size)
for idx, input in enumerate(dataset):
print('='*20,idx,'='*20)
print('Input Size:',input.shape)
hidden=cell(input,hidden)
print('outputs size:',hidden.shape)
print(hidden)
输出结果:
==================== 0 ====================
Input Size: torch.Size([1, 4])
outputs size: torch.Size([1, 2])
tensor([[-0.8999, -0.3907]], grad_fn=<TanhBackward>)
==================== 1 ====================
Input Size: torch.Size([1, 4])
outputs size: torch.Size([1, 2])
tensor([[ 0.9884, -0.9827]], grad_fn=<TanhBackward>)
==================== 2 ====================
Input Size: torch.Size([1, 4])
outputs size: torch.Size([1, 2])
tensor([[-0.9612, 0.1561]], grad_fn=<TanhBackward>)
use RNN:
全部代码:
import torch
batch_size=1
seq_len=3
input_size=4
hidden_size=2
num_layers=1
cell=torch.nn.RNN(input_size=input_size,hidden_size=hidden_size,
num_layers=num_layers)
inputs=torch.randn(seq_len,batch_size,input_size)
hidden=torch.zeros(num_layers,batch_size,hidden_size)
out,hidden=cell(inputs,hidden)
print('Output Size:',out.shape)
print('Output:',out)
print('Hidden size:',hidden.shape)
print('Hidden:',hidden)
输出结果:
Output Size: torch.Size([3, 1, 2])
Output: tensor([[[-0.2092, 0.7386]],
[[ 0.0670, -0.1176]],
[[-0.8666, 0.8464]]], grad_fn=<StackBackward>)
Hidden size: torch.Size([1, 1, 2])
Hidden: tensor([[[-0.8666, 0.8464]]], grad_fn=<StackBackward>)
训练一个学习模型: “hello”-》“ohlol”
实现了将文本转换为向量 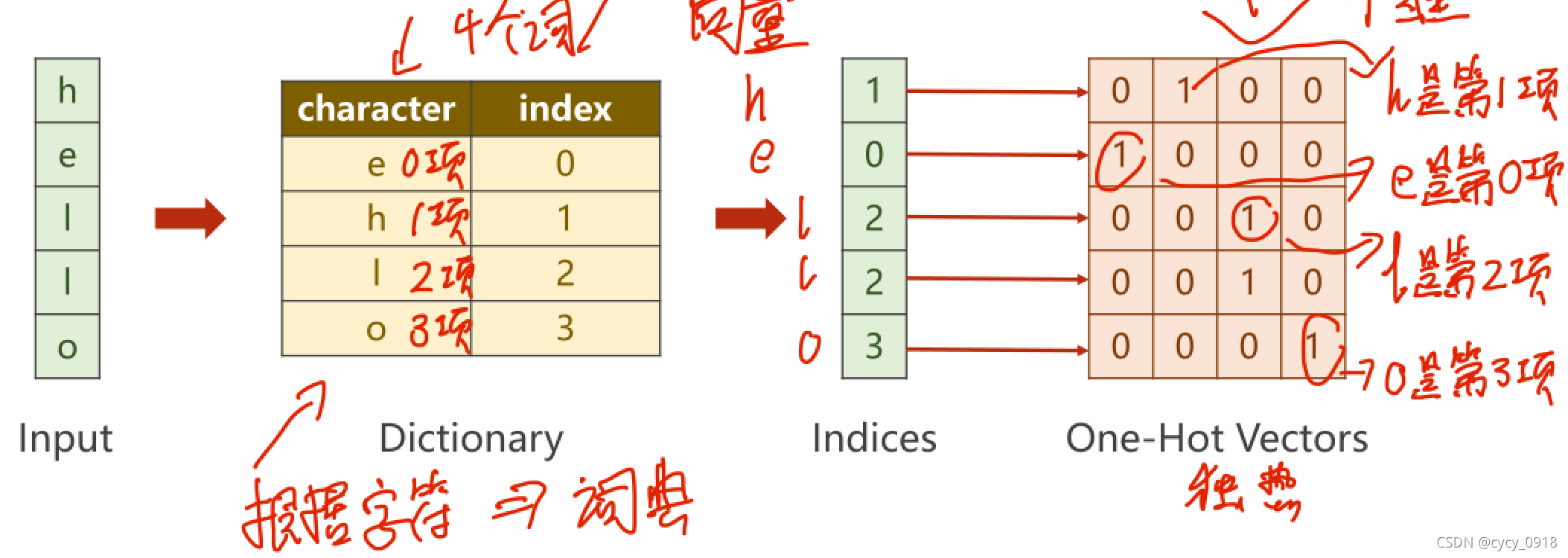 softmax层所做的工作: 1:线性变换 2:和 3:除 NLLLoss层所做的工作: 交叉熵损失函数 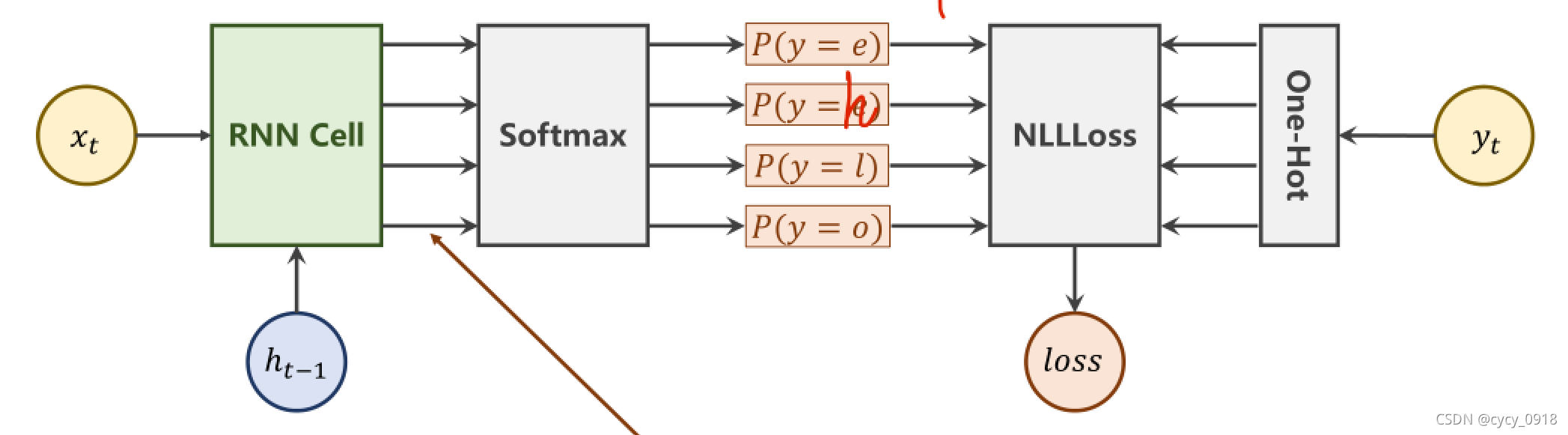 全部代码:
import torch
input_size=4
hidden_size=4
batch_size=1
idx2char=['e','h','l','o']
x_data=[1,0,2,2,3]
y_data=[3,1,2,3,2]
one_hot_lookup=[[1,0,0,0],
[0,1,0,0],
[0,0,1,0],
[0,0,0,1]]
x_one_hot=[one_hot_lookup[x] for x in x_data]
print('x_one_hot:',x_one_hot)
inputs=torch.Tensor(x_one_hot).view(-1,batch_size,input_size)
labels=torch.LongTensor(y_data).view(-1,1)
class Model(torch.nn.Module):
def __init__(self,input_size,hidden_size,batch_size):
super(Model, self).__init__()
self.batch_size=batch_size
self.input_size=input_size
self.hidden_size=hidden_size
self.rnncell=torch.nn.RNNCell(input_size=self.input_size,
hidden_size=self.hidden_size)
def forward(self,input,hidden):
hidden=self.rnncell(input,hidden)
return hidden
def init_hidden(self):
return torch.zeros(self.batch_size,self.hidden_size)
net=Model(input_size,hidden_size,batch_size)
criterion=torch.nn.CrossEntropyLoss()
optimizer=torch.optim.Adam(net.parameters(), lr=0.1)
for epoch in range(20):
loss=0
optimizer.zero_grad()
hidden = net.init_hidden()
print('Predicted String:',end='')
for input ,lable in zip(inputs,labels):
hidden = net(input,hidden)
loss+=criterion(hidden,lable)
_, idx=hidden.max(dim=1)
print(idx2char[idx.item()],end='')
loss.backward()
optimizer.step()
print(',Epoch [%d/20] loss=%.4f' % (epoch+1, loss.item()))
输出结果:
x_one_hot: [[0, 1, 0, 0], [1, 0, 0, 0], [0, 0, 1, 0], [0, 0, 1, 0], [0, 0, 0, 1]]
Predicted String:eeeel,Epoch [1/20] loss=7.4964
Predicted String:ellll,Epoch [2/20] loss=6.4440
Predicted String:ellll,Epoch [3/20] loss=5.6802
Predicted String:ooool,Epoch [4/20] loss=5.0243
Predicted String:ohool,Epoch [5/20] loss=4.4601
Predicted String:ohool,Epoch [6/20] loss=3.9765
Predicted String:ohool,Epoch [7/20] loss=3.5182
Predicted String:ohool,Epoch [8/20] loss=3.1421
Predicted String:ohlol,Epoch [9/20] loss=2.8956
Predicted String:ohlol,Epoch [10/20] loss=2.7375
Predicted String:ohlol,Epoch [11/20] loss=2.6229
Predicted String:ohlol,Epoch [12/20] loss=2.5303
Predicted String:ohlol,Epoch [13/20] loss=2.4499
Predicted String:ohlol,Epoch [14/20] loss=2.3756
Predicted String:ohlol,Epoch [15/20] loss=2.3010
Predicted String:ohlol,Epoch [16/20] loss=2.2216
Predicted String:ohlol,Epoch [17/20] loss=2.1437
Predicted String:ohlol,Epoch [18/20] loss=2.0968
Predicted String:ohlol,Epoch [19/20] loss=2.0884
Predicted String:ohlol,Epoch [20/20] loss=2.0438
使用RNN
全部代码:
import torch
input_size=4
hidden_size=4
num_layers=1
batch_size=1
seq_len=5
idx2char=['e','h','l','o']
x_data=[1,0,2,2,3]
y_data=[3,1,2,3,2]
one_hot_lookup=[[1,0,0,0],
[0,1,0,0],
[0,0,1,0],
[0,0,0,1]]
x_one_hot=[one_hot_lookup[x] for x in x_data]
print('x_one_hot:',x_one_hot)
inputs=torch.Tensor(x_one_hot).view(seq_len,batch_size,input_size)
labels=torch.LongTensor(y_data)
class Model(torch.nn.Module):
def __init__(self,input_size,hidden_size,batch_size,num_layers=1):
super(Model, self).__init__()
self.num_layers=num_layers
self.batch_size=batch_size
self.input_size=input_size
self.hidden_size=hidden_size
self.rnn=torch.nn.RNN(input_size=self.input_size,
hidden_size=self.hidden_size,
num_layers=self.num_layers)
def forward(self,input):
hidden=torch.zeros(self.num_layers,self.batch_size,self.hidden_size)
out, _=self.rnn(input,hidden)
return out.view(-1,self.hidden_size)
net=Model(input_size,hidden_size,batch_size,num_layers)
criterion=torch.nn.CrossEntropyLoss()
optimizer=torch.optim.Adam(net.parameters(), lr=0.05)
for epoch in range(20):
optimizer.zero_grad()
outputs=net(inputs)
loss=criterion(outputs,labels)
loss.backward()
optimizer.step()
_, idx=outputs.max(dim=1)
idx=idx.data.numpy()
print('Predicted: ',''.join([idx2char[x] for x in idx]),end='')
print(',Epoch [%d/20] loss=%.3f' % (epoch+1, loss.item()))
输出结果:
x_one_hot: [[0, 1, 0, 0], [1, 0, 0, 0], [0, 0, 1, 0], [0, 0, 1, 0], [0, 0, 0, 1]]
Predicted: lhlll,Epoch [1/20] loss=1.395
Predicted: lhlll,Epoch [2/20] loss=1.269
Predicted: lhlll,Epoch [3/20] loss=1.141
Predicted: lhlhl,Epoch [4/20] loss=1.015
Predicted: lhlol,Epoch [5/20] loss=0.896
Predicted: ohlol,Epoch [6/20] loss=0.794
Predicted: ohlol,Epoch [7/20] loss=0.713
Predicted: ohlol,Epoch [8/20] loss=0.650
Predicted: ohlol,Epoch [9/20] loss=0.599
Predicted: ohlol,Epoch [10/20] loss=0.557
Predicted: ohlol,Epoch [11/20] loss=0.523
Predicted: ohlol,Epoch [12/20] loss=0.499
Predicted: ohlol,Epoch [13/20] loss=0.481
Predicted: ohlol,Epoch [14/20] loss=0.467
Predicted: ohlol,Epoch [15/20] loss=0.456
Predicted: ohlol,Epoch [16/20] loss=0.446
Predicted: ohlol,Epoch [17/20] loss=0.436
Predicted: ohlol,Epoch [18/20] loss=0.426
Predicted: ohlol,Epoch [19/20] loss=0.417
Predicted: ohlol,Epoch [20/20] loss=0.410
参考博客: https://blog.csdn.net/Dianaia/article/details/112813576 https://blog.csdn.net/segegse/article/details/120929542
|