参考:https://blog.csdn.net/wsp_1138886114/article/details/82935839
步骤:
彩色图像转换为灰度图像(以灰度图或者单通道图读入) 对图像进行高斯模糊(去噪) 计算图像梯度,根据梯度计算图像边缘幅值与角度 沿梯度方向进行非极大值抑制(边缘细化) 双阈值边缘连接处理 二值化图像输出结果
"""
cv2.Canny(image, # 输入原图(必须为单通道图)
threshold1,
threshold2, # 较大的阈值2用于检测图像中明显的边缘
[, edges[,
apertureSize[, # apertureSize:Sobel算子的大小
L2gradient ]]]) # 参数(布尔值):
true: 使用更精确的L2范数进行计算(即两个方向的倒数的平方和再开放),
false:使用L1范数(直接将两个方向导数的绝对值相加)。
"""
import cv2
import numpy as np
original_img = cv2.imread("qingwen.png", 0)
# canny(): 边缘检测
img1 = cv2.GaussianBlur(original_img,(3,3),0)
canny = cv2.Canny(img1, 50, 150)
# 形态学:边缘检测
_,Thr_img = cv2.threshold(original_img,210,255,cv2.THRESH_BINARY)#设定红色通道阈值210(阈值影响梯度运算效果)
kernel = cv2.getStructuringElement(cv2.MORPH_RECT,(5,5)) #定义矩形结构元素
gradient = cv2.morphologyEx(Thr_img, cv2.MORPH_GRADIENT, kernel) #梯度
cv2.imshow("original_img", original_img)
cv2.imshow("gradient", gradient)
cv2.imshow('Canny', canny)
cv2.waitKey(0)
cv2.destroyAllWindows()
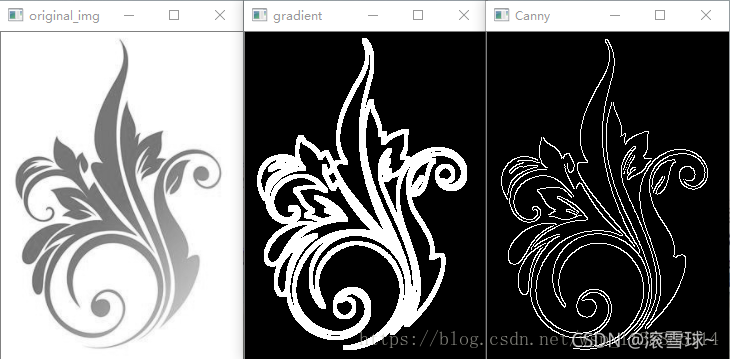 可调整阈值大小的程序
import cv2
import numpy as np
def CannyThreshold(lowThreshold):
detected_edges = cv2.GaussianBlur(gray,(3,3),0)
detected_edges = cv2.Canny(detected_edges,
lowThreshold,
lowThreshold*ratio,
apertureSize = kernel_size)
dst = cv2.bitwise_and(img,img,mask = detected_edges) # just add some colours to edges from original image.
cv2.imshow('canny demo',dst)
lowThreshold = 0
max_lowThreshold = 100
ratio = 3
kernel_size = 3
img = cv2.imread('qingwen.png')
gray = cv2.cvtColor(img,cv2.COLOR_BGR2GRAY)
cv2.namedWindow('canny demo')
cv2.createTrackbar('Min threshold','canny demo',lowThreshold, max_lowThreshold, CannyThreshold)
CannyThreshold(0) # initialization
if cv2.waitKey(0) == 27:
cv2.destroyAllWindows()
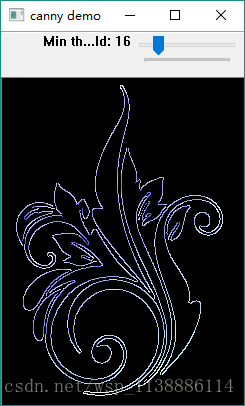
|