上一篇文章我们分析了如何使用g2o进行位姿图的优化.
由于g2o天然是进行位姿图优化的, 所以十分契合karto的位姿图的接口, 只需要将对应的顶点和约束分别赋值过去就可以了.
这篇文章我们来看一下另一个比较常用的优化库 Ceres solver.
1 ceres简介
Ceres solver 是谷歌开发的一款用于非线性优化的库, 在激光SLAM和V-SLAM的优化中均有着大量的应用, 如Cartographer, VINS 等等.
ceres的学习可以直接去它的官网上看教程, 谷歌出品的库的文档都很棒. 我在这里就不多说了. http://ceres-solver.org/tutorial.html
目前网上的很多教程及文章都是翻译的官网, 还是去看第一手资料比较好.
我之前看官方文档的时候做过一个笔记, 感兴趣的同学也可以看看.
ceres笔记 https://blog.csdn.net/tiancailx/article/details/117601117
2 基于ceres的后端优化的代码讲解
接下来就来看一看, 如何使用ceres进行后端优化的求解.
首先是头文件, 头文件基本上一样的, 就是对接口的继承, 就不多说了.
2.1 向位姿图中添加节点
由于ceres不是专门用于位姿图优化的, ceres的本质是求解最小二乘问题.
而目前的slam问题中的位姿图优化的本质都是依赖于最小二乘的, 所以位姿图优化是可以转成最小二乘问题的.
ceres内部没有节点和边的概念, 所以在AddNode函数中, 不像g2o那样可以直接将节点添加到g2o中, 这里首先将节点保存起来, 等到优化的时候再进行计算, 再传入到ceres中.
由于ceres中的数据类型是double, 所以在保存的时候进行了格式的转换.
void CeresSolver::AddNode(karto::Vertex<karto::LocalizedRangeScan> *pVertex)
{
karto::Pose2 pose = pVertex->GetObject()->GetCorrectedPose();
int pose_id = pVertex->GetObject()->GetUniqueId();
Pose2d pose2d;
pose2d.x = pose.GetX();
pose2d.y = pose.GetY();
pose2d.yaw_radians = pose.GetHeading();
poses_[pose_id] = pose2d;
}
struct Pose2d
{
double x;
double y;
double yaw_radians;
};
2.2 向位姿图中添加边(约束)
AddConstraint这个函数中的实现和AddNode函数是差不多的.
由于不能直接将边添加到ceres中, 所以在这先做了数据格式的转换, 然后将这个边保存起来.
这里保存的数据分别是 2个节点的 ID, 2个节点间的位姿变换.
在保存位姿的协方差矩阵时进行了求逆的操作, 所以保存的是信息矩阵.
void CeresSolver::AddConstraint(karto::Edge<karto::LocalizedRangeScan> *pEdge)
{
karto::LocalizedRangeScan *pSource = pEdge->GetSource()->GetObject();
karto::LocalizedRangeScan *pTarget = pEdge->GetTarget()->GetObject();
karto::LinkInfo *pLinkInfo = (karto::LinkInfo *)(pEdge->GetLabel());
karto::Pose2 diff = pLinkInfo->GetPoseDifference();
karto::Matrix3 precisionMatrix = pLinkInfo->GetCovariance().Inverse();
Eigen::Matrix<double, 3, 3> info;
info(0, 0) = precisionMatrix(0, 0);
info(0, 1) = info(1, 0) = precisionMatrix(0, 1);
info(0, 2) = info(2, 0) = precisionMatrix(0, 2);
info(1, 1) = precisionMatrix(1, 1);
info(1, 2) = info(2, 1) = precisionMatrix(1, 2);
info(2, 2) = precisionMatrix(2, 2);
Eigen::Vector3d measurement(diff.GetX(), diff.GetY(), diff.GetHeading());
Constraint2d constraint2d;
constraint2d.id_begin = pSource->GetUniqueId();
constraint2d.id_end = pTarget->GetUniqueId();
constraint2d.x = measurement(0);
constraint2d.y = measurement(1);
constraint2d.yaw_radians = measurement(2);
constraint2d.information = info;
constraints_.push_back(constraint2d);
}
struct Constraint2d
{
int id_begin;
int id_end;
double x;
double y;
double yaw_radians;
Eigen::Matrix3d information;
};
2.3 优化求解
进行求解, 并将优化后的位姿进行保存.
之前都是在保存数据, 真正的计算就在这2个函数里了, 分别是 BuildOptimizationProblem() 与 SolveOptimizationProblem().
void CeresSolver::Compute()
{
corrections_.clear();
ROS_INFO("[ceres] Calling ceres for Optimization");
ceres::Problem problem;
BuildOptimizationProblem(constraints_, &poses_, &problem);
SolveOptimizationProblem(&problem);
ROS_INFO("[ceres] Optimization finished\n");
for (std::map<int, Pose2d>::const_iterator pose_iter = poses_.begin(); pose_iter != poses_.end(); ++pose_iter)
{
karto::Pose2 pose(pose_iter->second.x, pose_iter->second.y, pose_iter->second.yaw_radians);
corrections_.push_back(std::make_pair(pose_iter->first, pose));
}
}
2.4 搭建模型
2.4.1 搭建最小二乘问题
简单解释一下代码.
首先, 通过AngleLocalParameterization::Create()函数定义了角度的更新方式.
这里要注意, 这个 ceres::LocalParameterization *angle_local_parameterization 指针的所有权是 在 problem->SetParameterization() 函数中 交给了 problem 的了, 也就是说, angle_local_parameterization会在problem析构的时候销毁掉.
注意, 这个指针是放在for循环的上边的, 可知, 同一个指针多次传入 AddResidualBlock() 与 SetParameterization() 是可以的. 只是在 problem 析构之后, 这个指针就被销毁了, 不能再使用了.
我在第一次代码写的时候将 angle_local_parameterization 这个指针放在类的成员变量中了, 并只在构造函数中 new 了一次, 当第二次进行优化的时候程序就崩了. 值得注意一下.
之后, 通过 PoseGraph2dErrorTerm::Create() 进行了 CostFunction 的构造.
然后, 将已有的约束信息通过 AddResidualBlock() 添加到优化问题中, 同时, 规定了角度值的更新方式.
最后, 将第一个节点的位姿设置成固定的, 不进行优化和改变.
void CeresSolver::BuildOptimizationProblem(const std::vector<Constraint2d> &constraints, std::map<int, Pose2d> *poses,
ceres::Problem *problem)
{
if (constraints.empty())
{
std::cout << "No constraints, no problem to optimize.";
return;
}
ceres::LocalParameterization *angle_local_parameterization = AngleLocalParameterization::Create();
for (std::vector<Constraint2d>::const_iterator constraints_iter = constraints.begin();
constraints_iter != constraints.end(); ++constraints_iter)
{
const Constraint2d &constraint = *constraints_iter;
std::map<int, Pose2d>::iterator pose_begin_iter = poses->find(constraint.id_begin);
std::map<int, Pose2d>::iterator pose_end_iter = poses->find(constraint.id_end);
const Eigen::Matrix3d sqrt_information = constraint.information.llt().matrixL();
ceres::CostFunction *cost_function =
PoseGraph2dErrorTerm::Create(constraint.x, constraint.y, constraint.yaw_radians, sqrt_information);
problem->AddResidualBlock(cost_function, nullptr,
&pose_begin_iter->second.x,
&pose_begin_iter->second.y,
&pose_begin_iter->second.yaw_radians,
&pose_end_iter->second.x,
&pose_end_iter->second.y,
&pose_end_iter->second.yaw_radians);
problem->SetParameterization(&pose_begin_iter->second.yaw_radians, angle_local_parameterization);
problem->SetParameterization(&pose_end_iter->second.yaw_radians, angle_local_parameterization);
}
std::map<int, Pose2d>::iterator pose_start_iter = poses->begin();
problem->SetParameterBlockConstant(&pose_start_iter->second.x);
problem->SetParameterBlockConstant(&pose_start_iter->second.y);
problem->SetParameterBlockConstant(&pose_start_iter->second.yaw_radians);
}
2.4.2 角度更新方式
通过重载 () 定义了角度的更新方式, 限定了角度更新后依然处于 [-pi, pi] 之间.代码很简单就不多说了.
之后, 通过 problem->SetParameterization(&pose_begin_iter->second.yaw_radians, angle_local_parameterization); 将这个角度更新方式的限定与角度变量关联起来.
class AngleLocalParameterization
{
public:
template <typename T>
bool operator()(const T *theta_radians, const T *delta_theta_radians, T *theta_radians_plus_delta) const
{
*theta_radians_plus_delta = NormalizeAngle(*theta_radians + *delta_theta_radians);
return true;
}
static ceres::LocalParameterization *Create()
{
return (new ceres::AutoDiffLocalParameterization<AngleLocalParameterization, 1, 1>);
}
};
template <typename T>
inline T NormalizeAngle(const T &angle_radians)
{
T two_pi(2.0 * M_PI);
return angle_radians - two_pi * ceres::floor((angle_radians + T(M_PI)) / two_pi);
}
2.4.3 残差的计算
首先通过 PoseGraph2dErrorTerm::Create() 函数进行 ceres::CostFunction 的声明, 并传入 约束的 x, y, theta 以及开方后的信息矩阵 到 PoseGraph2dErrorTerm类的构造函数中将数据进行保存.
之后, 通过重载 () 定义了残差的计算方式. 仿函数的参数就是通过 problem->AddResidualBlock() 传入进来的, 分别是2个位姿的xy与角度.
2个位姿间的残差, 可以这样计算. 构造时传入了这2个位姿间的坐标变换, 而通过这两个节点的位姿又可以算出一个坐标变换, 在正常情况下这2个坐标变换应该是相等的, 所以, 可以让这两个坐标变换的差作为残差.
代码中的 RotationMatrix2D(*yaw_a).transpose() * (p_b - p_a) 就是根据2个节点的位姿算出的坐标变换, p_ab_.cast<T>() 就是传入的这2个位姿间的坐标变换, 通过让2者相减得到 xy的残差.
同理, 角度相减得到角度的残差.
这里, 在计算完成之后, 又将残差左乘了开方后的信息矩阵, 通过协方差矩阵来对计算出的残差添加噪声.
template <typename T>
Eigen::Matrix<T, 2, 2> RotationMatrix2D(T yaw_radians)
{
const T cos_yaw = ceres::cos(yaw_radians);
const T sin_yaw = ceres::sin(yaw_radians);
Eigen::Matrix<T, 2, 2> rotation;
rotation << cos_yaw, -sin_yaw, sin_yaw, cos_yaw;
return rotation;
}
class PoseGraph2dErrorTerm
{
public:
PoseGraph2dErrorTerm(double x_ab, double y_ab, double yaw_ab_radians, const Eigen::Matrix3d &sqrt_information)
: p_ab_(x_ab, y_ab), yaw_ab_radians_(yaw_ab_radians), sqrt_information_(sqrt_information)
{
}
template <typename T>
bool operator()(const T *const x_a, const T *const y_a, const T *const yaw_a,
const T *const x_b, const T *const y_b, const T *const yaw_b,
T *residuals_ptr) const
{
const Eigen::Matrix<T, 2, 1> p_a(*x_a, *y_a);
const Eigen::Matrix<T, 2, 1> p_b(*x_b, *y_b);
Eigen::Map<Eigen::Matrix<T, 3, 1>> residuals_map(residuals_ptr);
residuals_map.template head<2>() = RotationMatrix2D(*yaw_a).transpose() * (p_b - p_a) - p_ab_.cast<T>();
residuals_map(2) = NormalizeAngle((*yaw_b - *yaw_a) - static_cast<T>(yaw_ab_radians_));
residuals_map = sqrt_information_.template cast<T>() * residuals_map;
return true;
}
static ceres::CostFunction *Create(double x_ab, double y_ab, double yaw_ab_radians,
const Eigen::Matrix3d &sqrt_information)
{
return (new ceres::AutoDiffCostFunction<PoseGraph2dErrorTerm, 3, 1, 1, 1, 1, 1, 1>(
new PoseGraph2dErrorTerm(x_ab, y_ab, yaw_ab_radians, sqrt_information)));
}
EIGEN_MAKE_ALIGNED_OPERATOR_NEW
private:
const Eigen::Vector2d p_ab_;
const double yaw_ab_radians_;
const Eigen::Matrix3d sqrt_information_;
};
2.5 进行求解
这块的代码就比较简单了.
首先设置了求解时的参数, 然后调用 ceres::Solve() 进行求解, 并将求解结果的简要报告打印出来.
bool CeresSolver::SolveOptimizationProblem(ceres::Problem *problem)
{
assert(problem != NULL);
ceres::Solver::Options options;
options.max_num_iterations = 100;
options.linear_solver_type = ceres::SPARSE_NORMAL_CHOLESKY;
ceres::Solver::Summary summary;
ceres::Solve(options, problem, &summary);
std::cout << summary.BriefReport() << '\n';
return summary.IsSolutionUsable();
}
3 运行
3.1 依赖
这篇文章的代码是需要依赖 1.13.0 版本的ceres库, 如果没装ceres的需要先安装一下. 我将ceres库的安装包放在了 工程Creating-2D-laser-slam-from-scratch/TrirdParty文件加内, 可以直接解压安装.
安装的方法可以看一下 install_dependence.sh 脚本中的安装指令, 也可以直接执行这个脚本进行所有依赖项的安装.
安装完了ceres之后, 编译代码, 如果一切顺利的话是可以编译通过的.
这里要说一下, 很多同学说代码在ubuntu 18 或者其他的ubuntu版本上编译不过, 这我也没啥办法, 我一直用的是 1604, 没试过其他的版本. 如果实在编译不过就看看文章吧, 或者看看源码.
3.2 运行
本篇文章对应的数据包, 请在我的公众号中回复 lesson6 获得,并将launch中的bag_filename更改成您实际的目录名。
我将之前使用过的数据包的链接都放在腾讯文档里了, 腾讯文档的地址如下: https://docs.qq.com/sheet/DVElRQVNlY0tHU01I?tab=BB08J2
通过如下命令运行本篇文章对应的程序 roslaunch lesson6 karto_slam_outdoor.launch solver_type:=ceres_solver
3.3 结果分析
启动之后, 会显示出使用的优化器的具体类型.
[ INFO] [1637029966.740001825]: ----> Karto SLAM started.
[ INFO] [1637029966.790614727]: Use back end.
[ INFO] [1637029966.790716666]: solver type is CeresSolver.
在运行前期, 由于没有找到回环, 所以一直没有进行优化. 在最后阶段产生回环时, 会调用基于ceres的优化, 并打印处如下的log.
[ INFO] [1637030162.060933669, 1606808844.440984444]: [ceres] Calling ceres for Optimization
Ceres Solver Report: Iterations: 8, Initial cost: 4.364248e-02, Final cost: 7.465524e-15, Termination: CONVERGENCE
[ INFO] [1637030162.089423589, 1606808844.471175613]: [ceres] Optimization finished
可以看到, 优化前的cost是 e-02 量级的, 优化后的cost是 e-15 量级的, 效果十分明显.
优化前
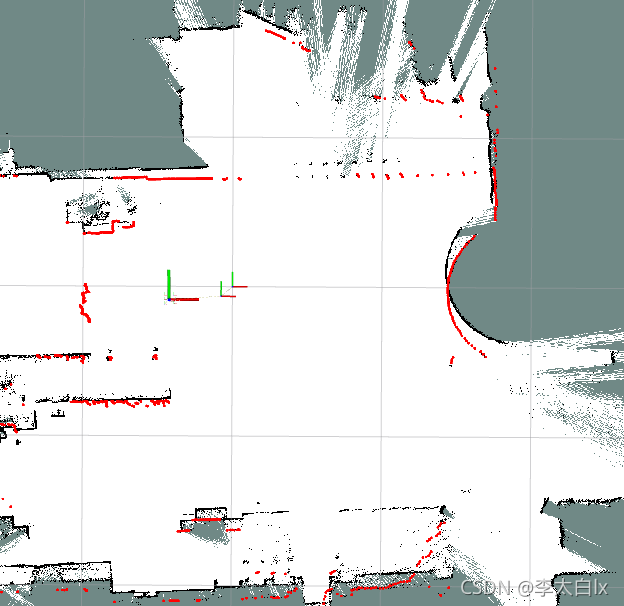
优化后
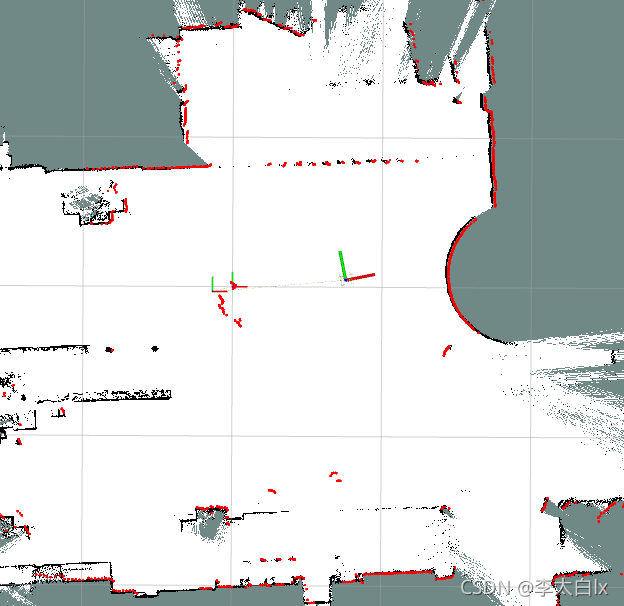
最终的地图
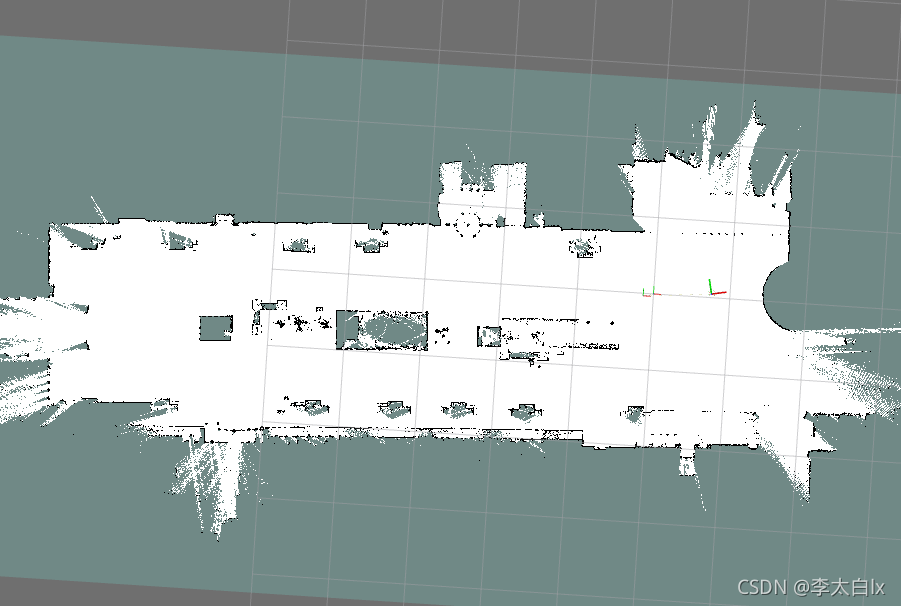
最终的cost
看一下最终的cost, 可以看到, 之前优化后的cost都是 e-15 这种级别的, 但是最后一次的cost是 e-02, 变大了很多. 原因还不清楚.
猜测可能是由于数据数据突然结束了, 导致其实还存在优化的空间???
有了解的同学可以评论告诉我一下, 十分感谢.
[ INFO] [1637032488.825845405, 1606808848.018522836]: [ceres] Calling ceres for Optimization
Ceres Solver Report: Iterations: 8, Initial cost: 6.562447e-02, Final cost: 5.582333e-15, Termination: CONVERGENCE
[ INFO] [1637032488.850353017, 1606808848.038643242]: [ceres] Optimization finished
[ INFO] [1637032489.159521180, 1606808848.351362847]: [ceres] Calling ceres for Optimization
Ceres Solver Report: Iterations: 8, Initial cost: 2.219165e-02, Final cost: 6.116063e-15, Termination: CONVERGENCE
[ INFO] [1637032489.183078270, 1606808848.371497779]: [ceres] Optimization finished
[ INFO] [1637032489.493828053, 1606808848.683609037]: [ceres] Calling ceres for Optimization
Ceres Solver Report: Iterations: 9, Initial cost: 4.919947e+01, Final cost: 1.920770e-02, Termination: CONVERGENCE
[ INFO] [1637032489.519517167, 1606808848.703747883]: [ceres] Optimization finished
4 总结
通过这篇文章, 我们知道了如何使用ceres进行后端优化问题的搭建与计算, 并体验了优化前后的地图效果与cost值.
下篇文章将使用gtsam来进行后端优化的计算.
|