主要记录关于视觉理解以及受限标签相关的论文,欢迎访问 Github 项目。
0. Grafit: Learning fine-grained image representations with coarse labels
作者将“训练中仅使用粗粒度标签,以此学习细粒度特征”这一任务视为无监督学习的一个泛化(在无监督学习中每个样本即为一个粗粒度标签)。在这篇文章中,作者在 BYOL 的基础上引入 KNN Loss [1],以便更好地探索潜在的细粒度特征空间。
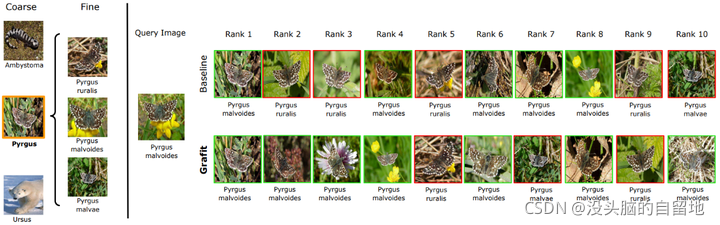
1. Causal Attention for Unbiased Visual Recognition
这是一篇Causal Presentation Learning 相关的论文,作者基于数据分割的因果干预方法引入对抗学习以便 “self-annotates the confounders in unsupervised fashion”。
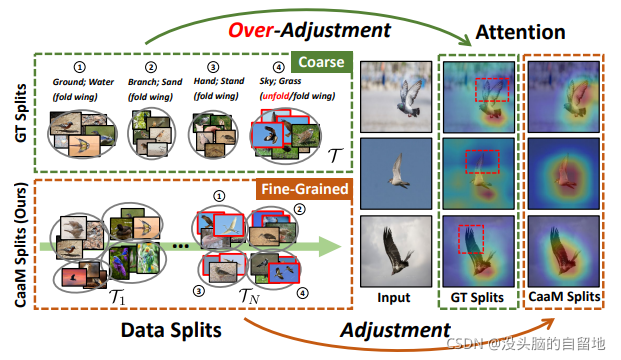
2. Semantic Diversity Learning for Zero-Shot Multi-label Classification
对于给定图像 i,模型生成多个 Principal Embedding Vector,并以标签向量 j 与其乘积的最大值作为该图像 i 与标签 j 的关联程度。在文章中,作者不仅依据任务细节设计了 Rank Loss,还根据图像的语义多样性动态调整样本权重。
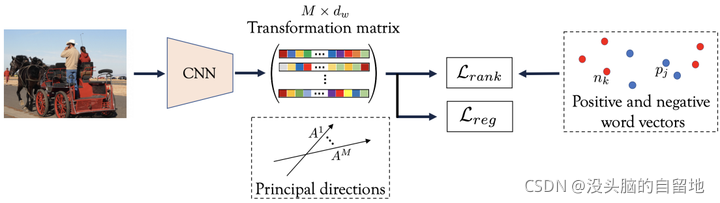
3. Scribble-Supervised Semantic Segmentation by Uncertainty Reduction on Neural Representation and Self-Supervision on Neural Eigenspace
作者通过 “uncertainty reduction on neural representation” 以及 “self-supervision on neural eigenspace” 期望解决 Scribble-supervised Semantic Segmentation 任务中常见的预测置信度不高以及不一致问题。 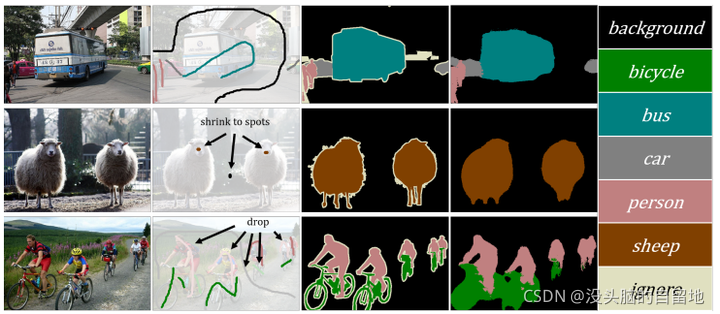
4. LocTex: Learning Data-Efficient Visual Representations from Localized Textual Supervision
与常见的图像分类或者目标检测任务不同,作者提出的 LocTex 任务旨在利用 captions 和 synchronized mouseover gestures 作为标注信息的设置下,更好地提高模型的性能。 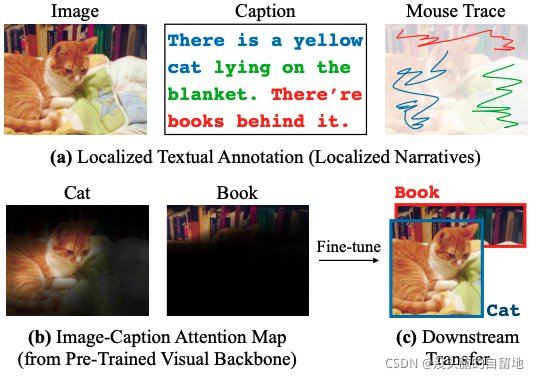
5. Transformer-based Dual Relation Graph for Multi-label Image Recognition
除了常见的 semantic relation graph,作者还引入了 Transofrmer 去学习 structural relation graph。 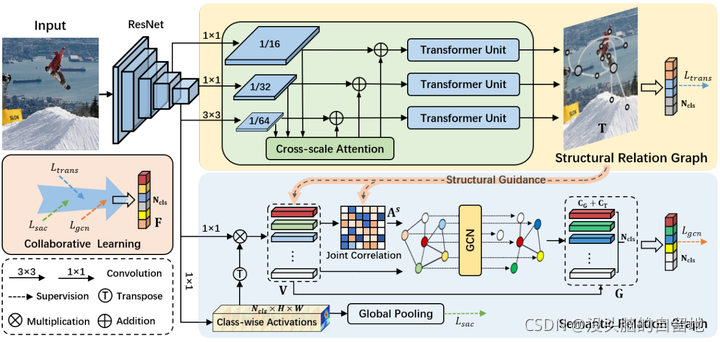
6. Pri3D: Can 3D Priors Help 2D Representation Learning?
通过引入 View-invariant Contrastive Loss 和 Geometric Prior Contrastive Loss 令模型利用 3D 图像数据进行预训练。
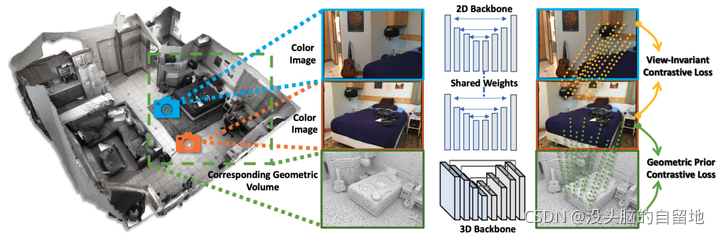
7. Interactive Prototype Learning for Egocentric Action Recognition
作者设计 Interactive Prototype Learning 框架以便提高对于活动对象的识别准确率。 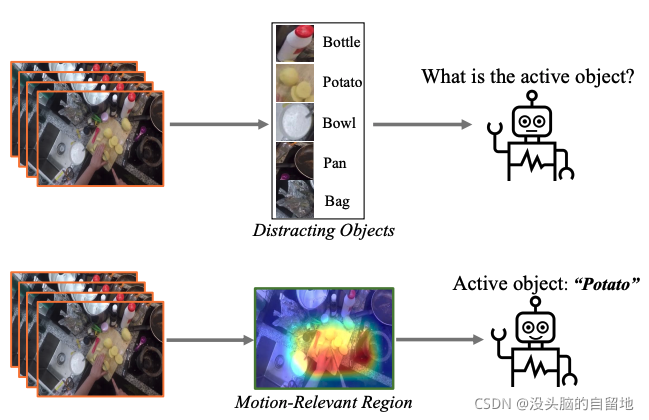
Reference
[1] Zhirong Wu et al., Improving Generalization via Scalable Neighborhood Component Analysis, ECCV 2018.
|