视频检测——获取帧数据
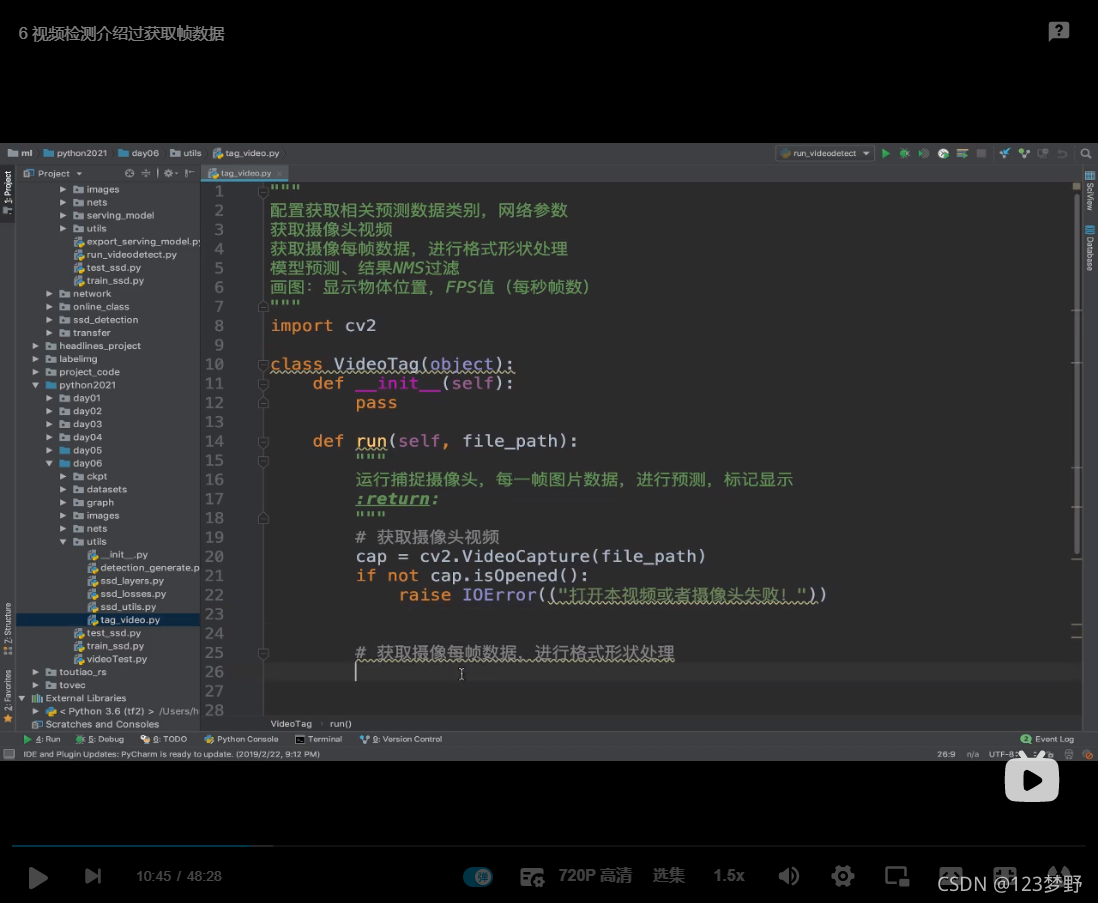 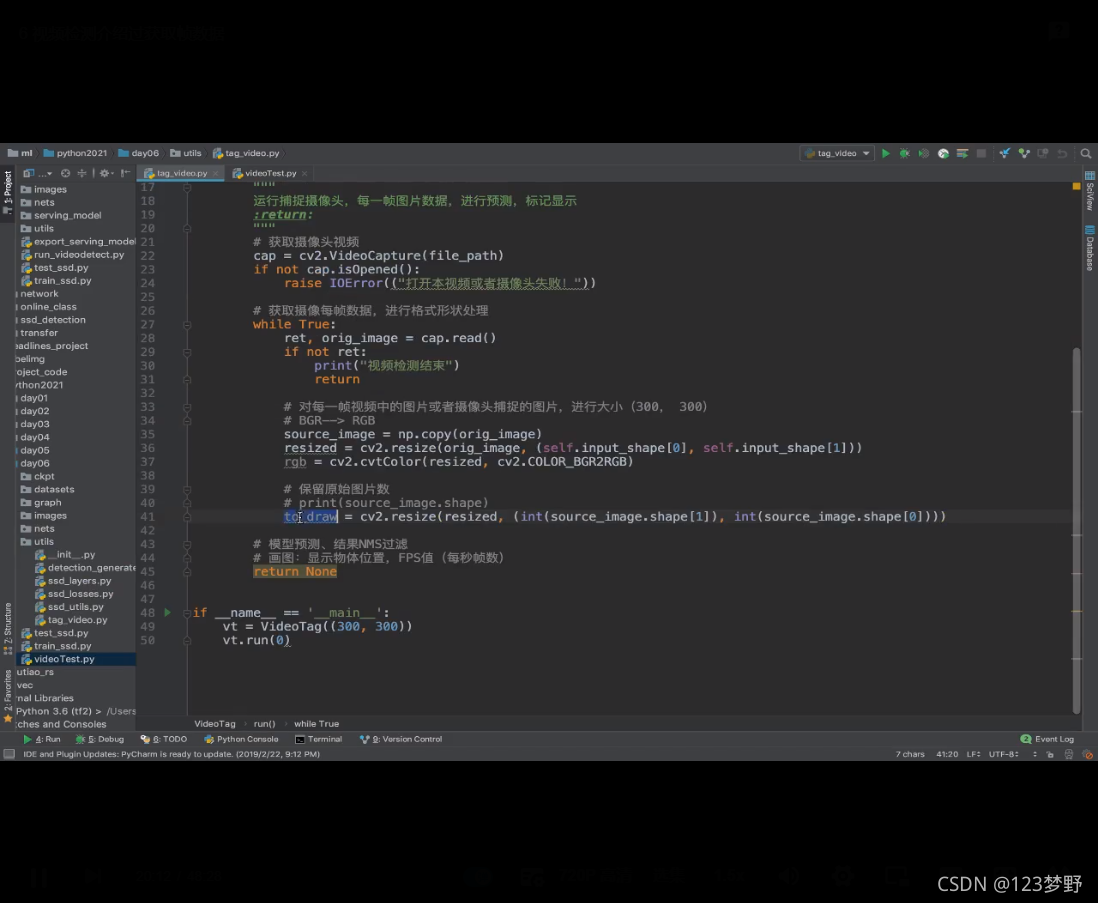 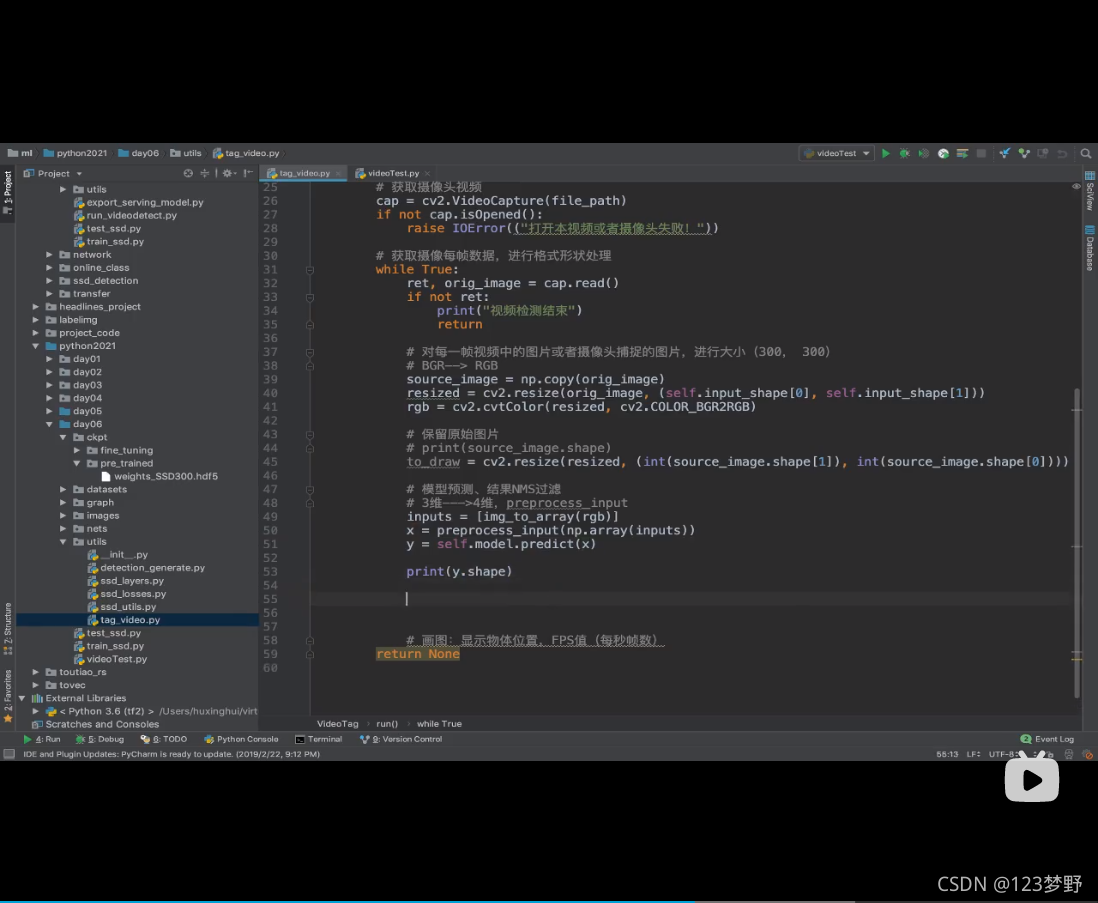 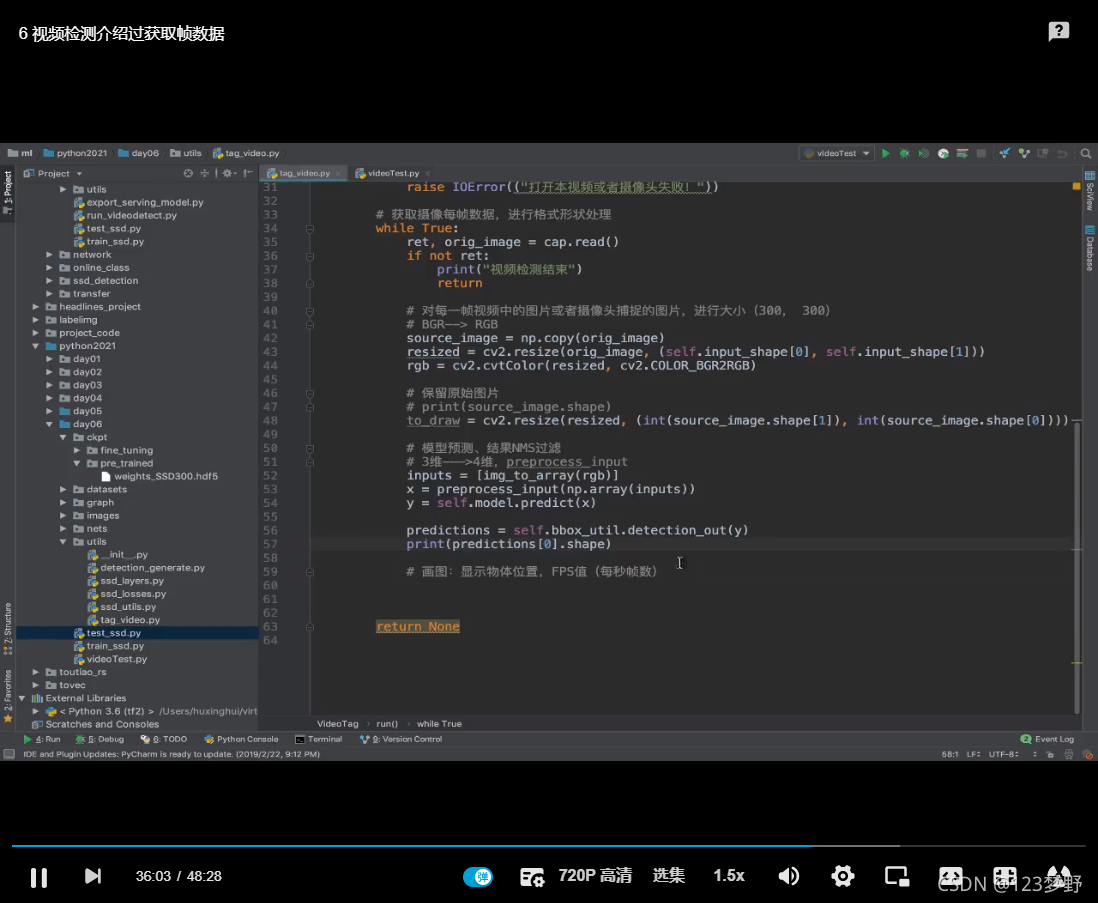 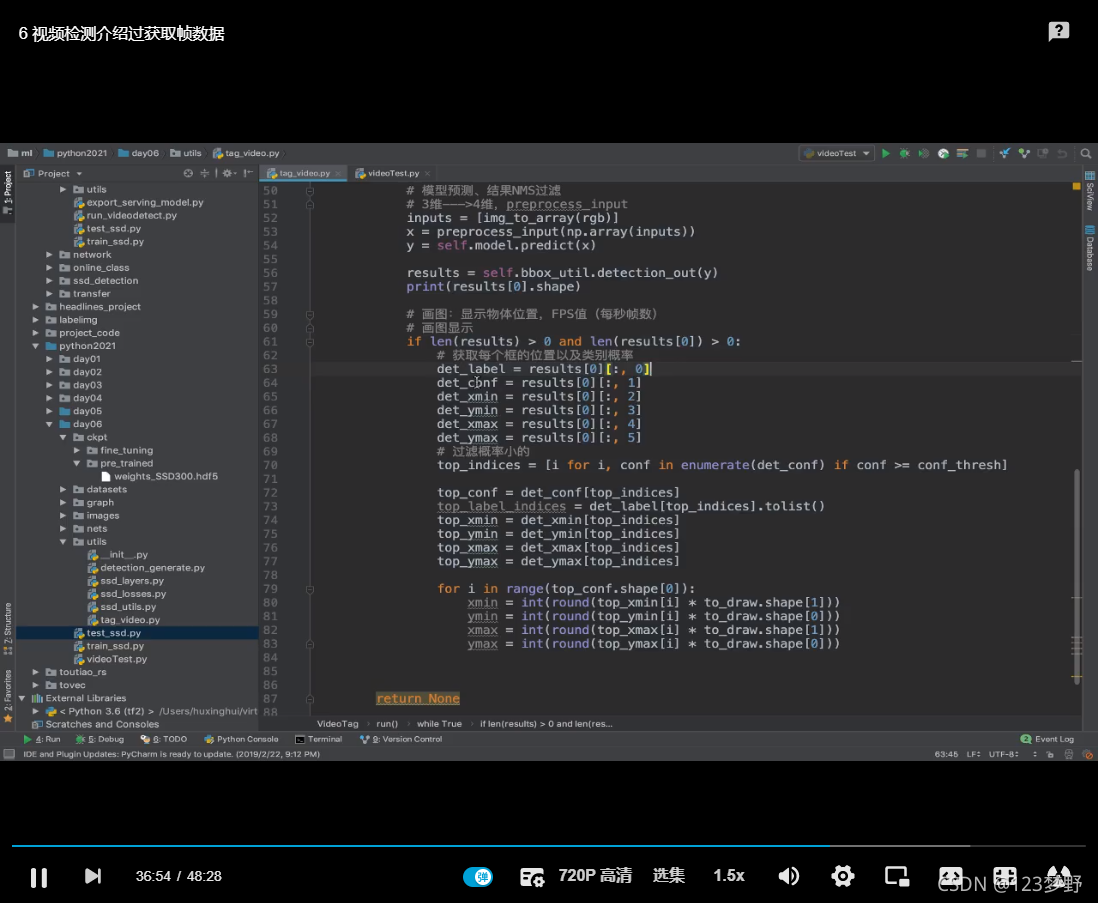 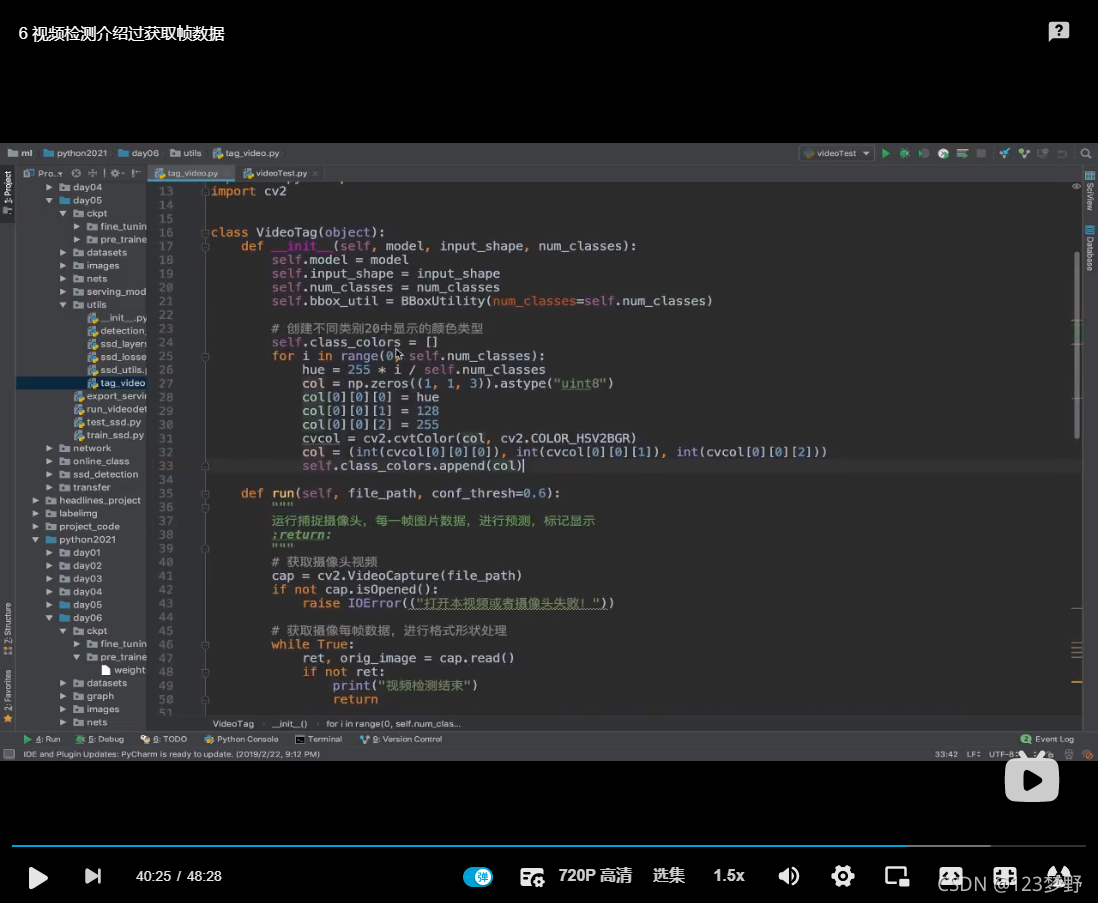 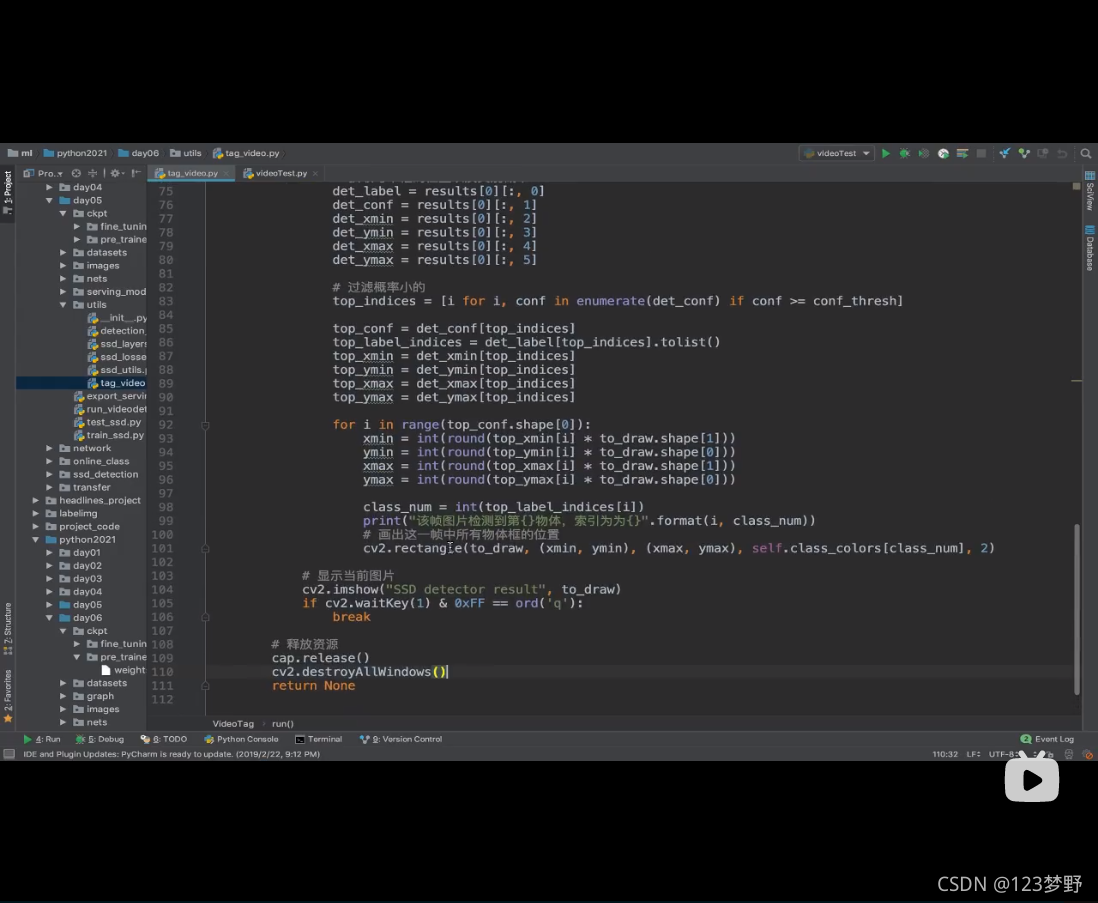
文本框显示
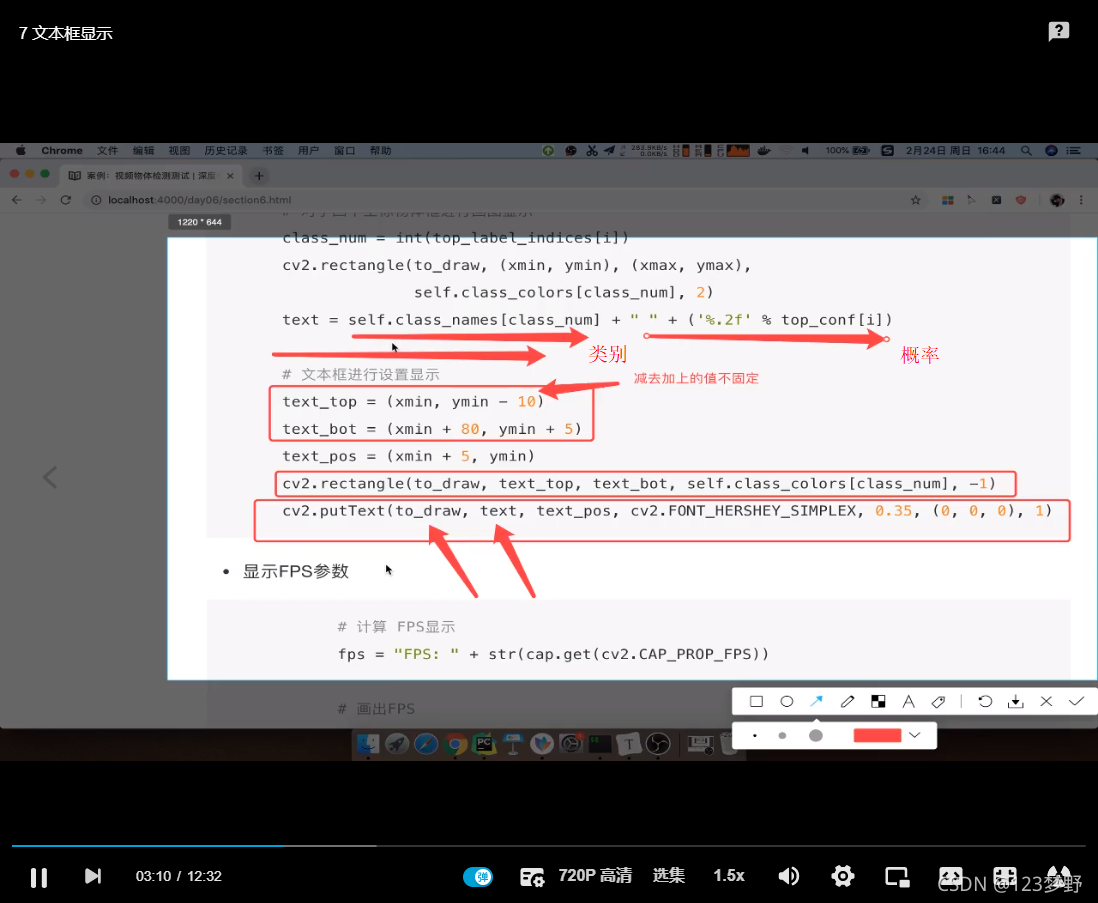 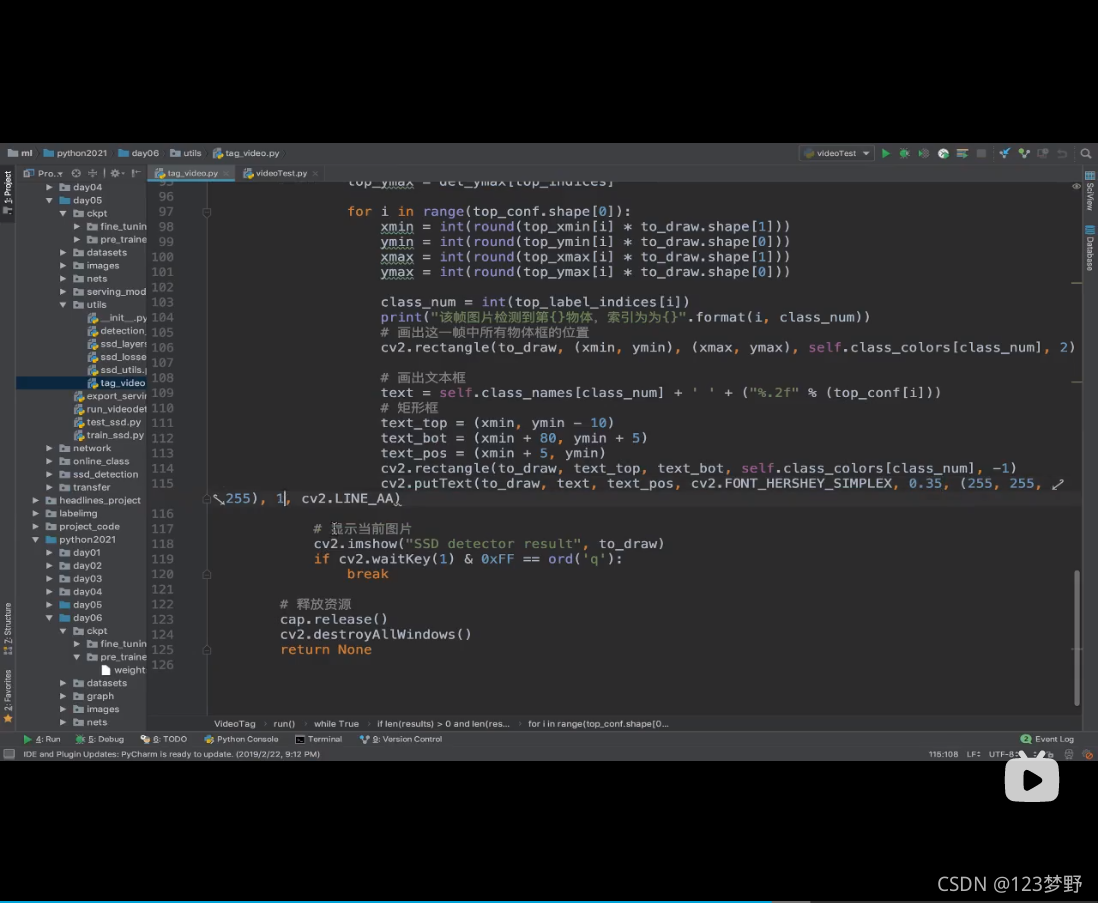
显示FPS参数
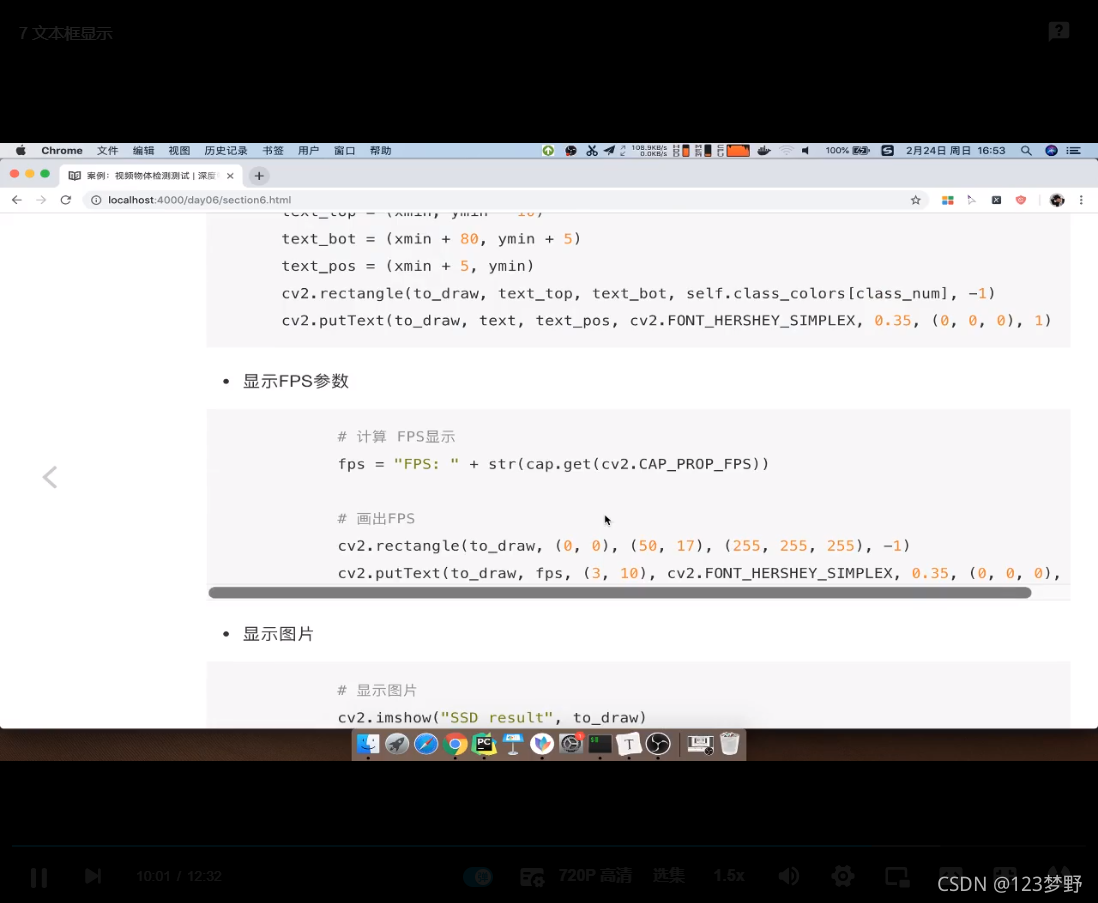 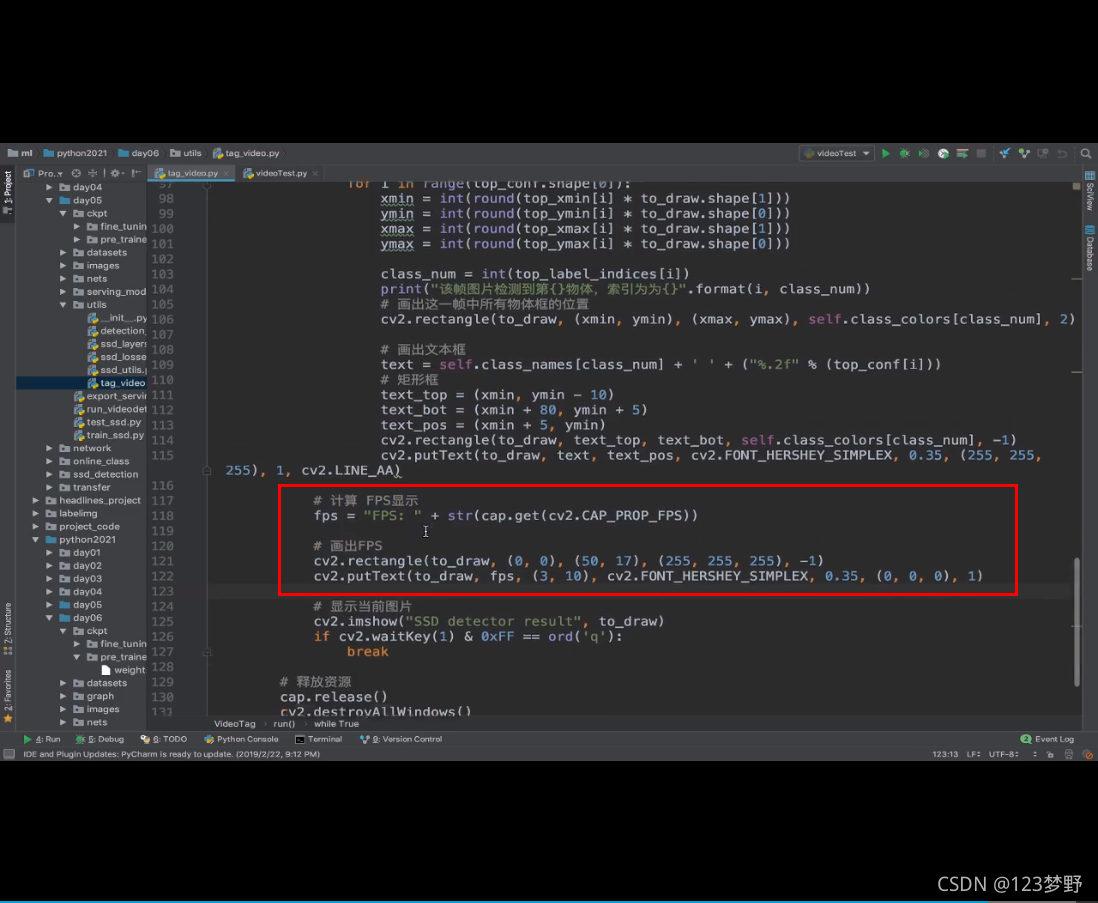
总结
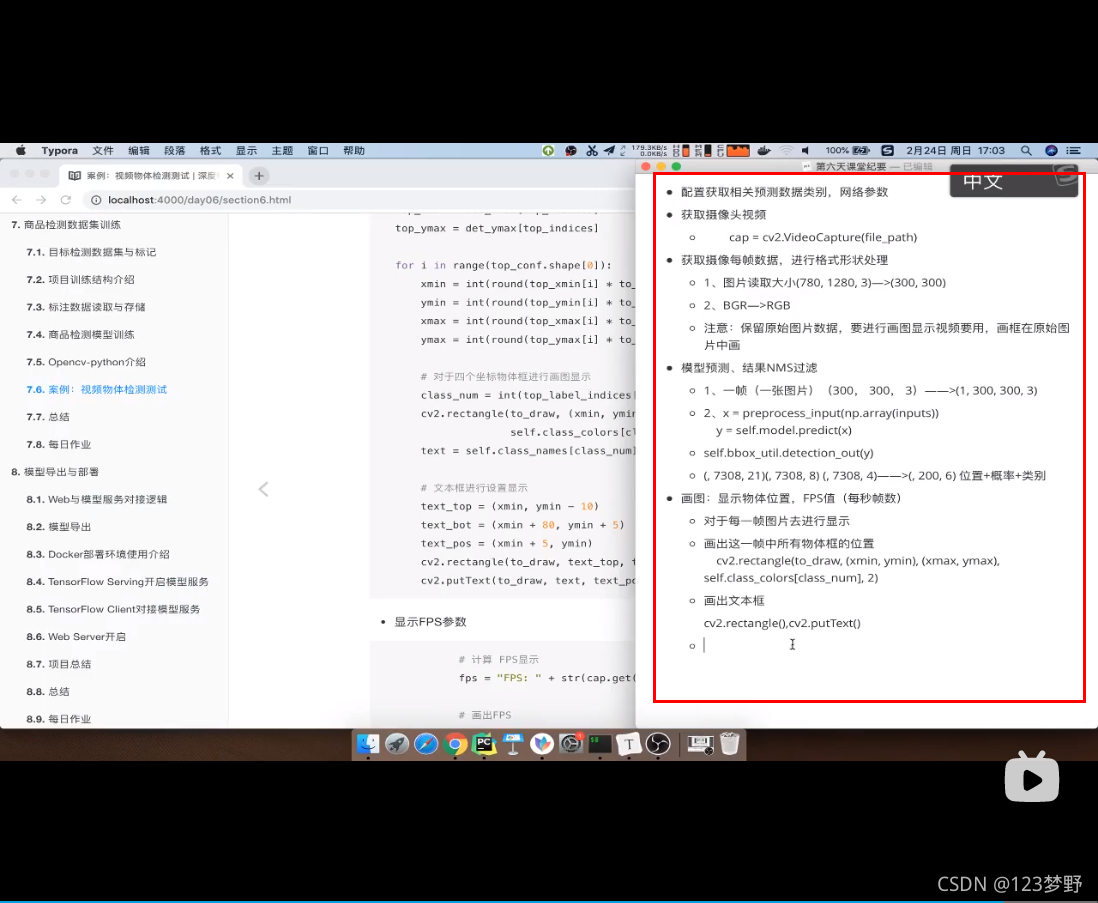 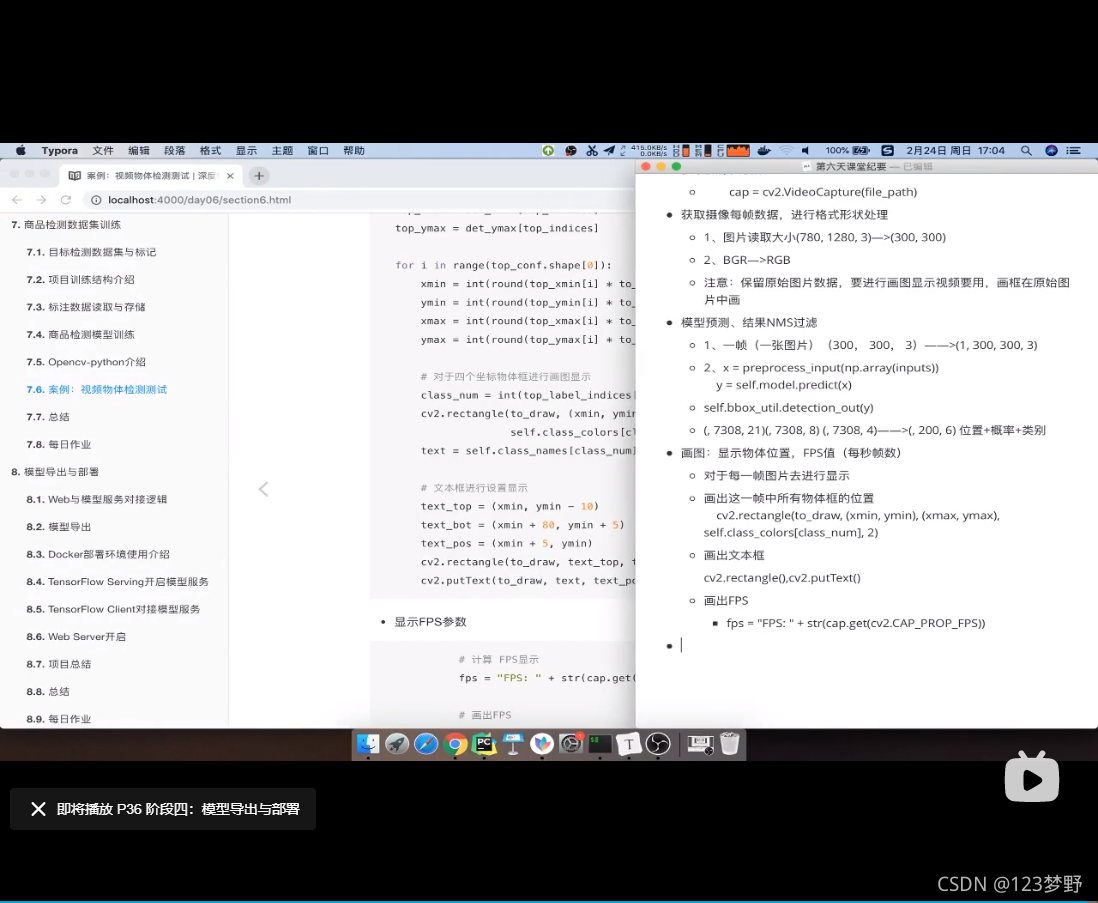
"""
配置获取相关预测数据类别,网络参数
获取摄像头视频
获取摄像每帧数据,进行格式形状处理
模型预测、结果NMS过滤
画图:显示物体位置,FPS值(每秒帧数)
"""
from tensorflow.python.keras.preprocessing.image import img_to_array
from tensorflow.python.keras.applications.imagenet_utils import preprocess_input
import numpy as np
import cv2
from nets.ssd_net import SSD300
from utils.ssd_utils import BBoxUtility
class VideoTag(object):
def __init__(self,model,input_shape,num_classes):
self.model = model
self.input_shape = input_shape
self.num_classes = num_classes
self.bbox_util = BBoxUtility(num_classes=self.num_classes)
self.class_colors=[]
for i in range(0,self.num_classes):
hue = 255*i/self.num_classes
col = np.zeros((1,1,3)).astype("uint8")
col[0][0][0] = hue
col[0][0][1] = 128
col[0][0][2] = 255
cvcol = cv2.cvtColor(col,cv2.COLOR_HSV2BGR)
col = (int(cvcol[0][0][0]),int(cvcol[0][0][1]),int(cvcol[0][0][2]))
self.class_colors.append(col)
def run(self,file_path,conf_thresh=0.6):
"""
运行捕捉摄像头,每一帧图片数据,进行预测,标记显示
:param file_path:
:return:
"""
cap=cv2.VideoCapture(file_path)
if not cap.isOpened():
raise IOError(("打开本视频或者摄像头失败!"))
while True:
ret,orig_image = cap.read()
if not ret:
print("视频检测结束")
return
source_image = np.copy(orig_image)
resized=cv2.resize(orig_image,(self.input_shape[0],self.input_shape[1]))
rgb = cv2.cvtColor(resized,cv2.COLOR_BGR2RGB)
to_draw = cv2.resize(resized,(int(source_image.shape[1]),int(source_image.shape[0])))
inputs = [img_to_array(rgb)]
x = preprocess_input(np.array(inputs))
y = self.model.predict(x)
results = self.bbox_util.detection_out(y)
print(results[0].shape)
if len(results) >0 and len(results[0])>0:
det_label = results[0][:,0]
det_conf = results[0][:,1]
det_xmin = results[0][:,2]
det_ymin = results[0][:,3]
det_xmax = results[0][:,4]
det_ymax = results[0][:,5]
top_indices = [i for i,conf in enumerate(det_conf) if conf >=conf_thresh]
top_conf = det_conf[top_indices]
top_label_indices = det_label[top_indices].tolist()
top_xmin = det_xmin[top_indices]
top_ymin = det_ymin[top_indices]
top_xmax = det_xmax[top_indices]
top_ymax = det_ymax[top_indices]
for i in range(top_conf.shape[0]):
xmin = int(round(top_xmin[i]*to_draw.shape[1]))
ymin = int(round(top_ymin[i]*to_draw.shape[0]))
xmax = int(round(top_xmax[i]*to_draw.shape[1]))
ymax = int(round(top_ymax[i]*to_draw.shape[0]))
class_num = int(top_label_indices[i])
print("该帧图片检测到第{}物体,索引为{}".format(i,class_num))
cv2.rectangle(to_draw,(xmin,ymin),(xmax,ymax),self.class_colors[class_num],2)
text = self.class_names[class_num]+' '+("%.2f"%(top_conf[i]))
text_top = (xmin,ymin-10)
text_bot = (xmin+80,ymin+5)
text_pos = (xmin+5,ymin)
cv2.rectangle(to_draw,text_top,text_bot,self.class_colors[class_num],-1)
cv2.putText(to_draw,text,text_pos,cv2.FONT_HERSHEY_SIMPLEX,0.35,(255,255,255),1,cv2.LINE_AA)
fps = "FPS:"+str(cap.get(cv2.CAP_PROP_FPS))
cv2.rectangle(to_draw,(0,0),(50,17),(255,255,255),-1)
cv2.putText(to_draw,fps,(3,10),cv2.FONT_HERSHEY_SIMPLEX,0.35,(0,0,0),1)
cv2.imshow("SSD detector result",to_draw)
if cv2.waitKey(1) & 0xFF == ord('q'):
break
cap.release()
cv2.destroyWindow()
return None
if __name__=='__name__':
input_shape=(300,300,3)
class_name=[]
model = SSD300(class_name,num_classes=len(class_name))
vt = VideoTag(model,input_shape,len(class_name))
vt.run(0)
|