加载共享自行车租赁数据集 BikeSharing.csv。
- 按以下要求处理数据集
(1)分离出仅含特征列的部分作为 X 和仅含目标列的部分作为 Y。 (2)将数据集拆分成训练集和测试集(70%和 30%)。 (3)对数据进行标准化处理 - 建立回归模型
分别用 LinearRegression 和 SGDRegression 两种方法建模。 - 结果比对
(1)分别对比两种模型在测试集上的预测性能(计算 score)。 (2)分别测试学习率(参数 eta0)取 0.005, 0.01, 0.015, 0.02, 0.025, 0.03, 0.035, 0.04, 0.045, 0.05 时,SGD 模型的性能得分,对测试结果进行绘图。 使用Jupyter对数据建立回归模型: 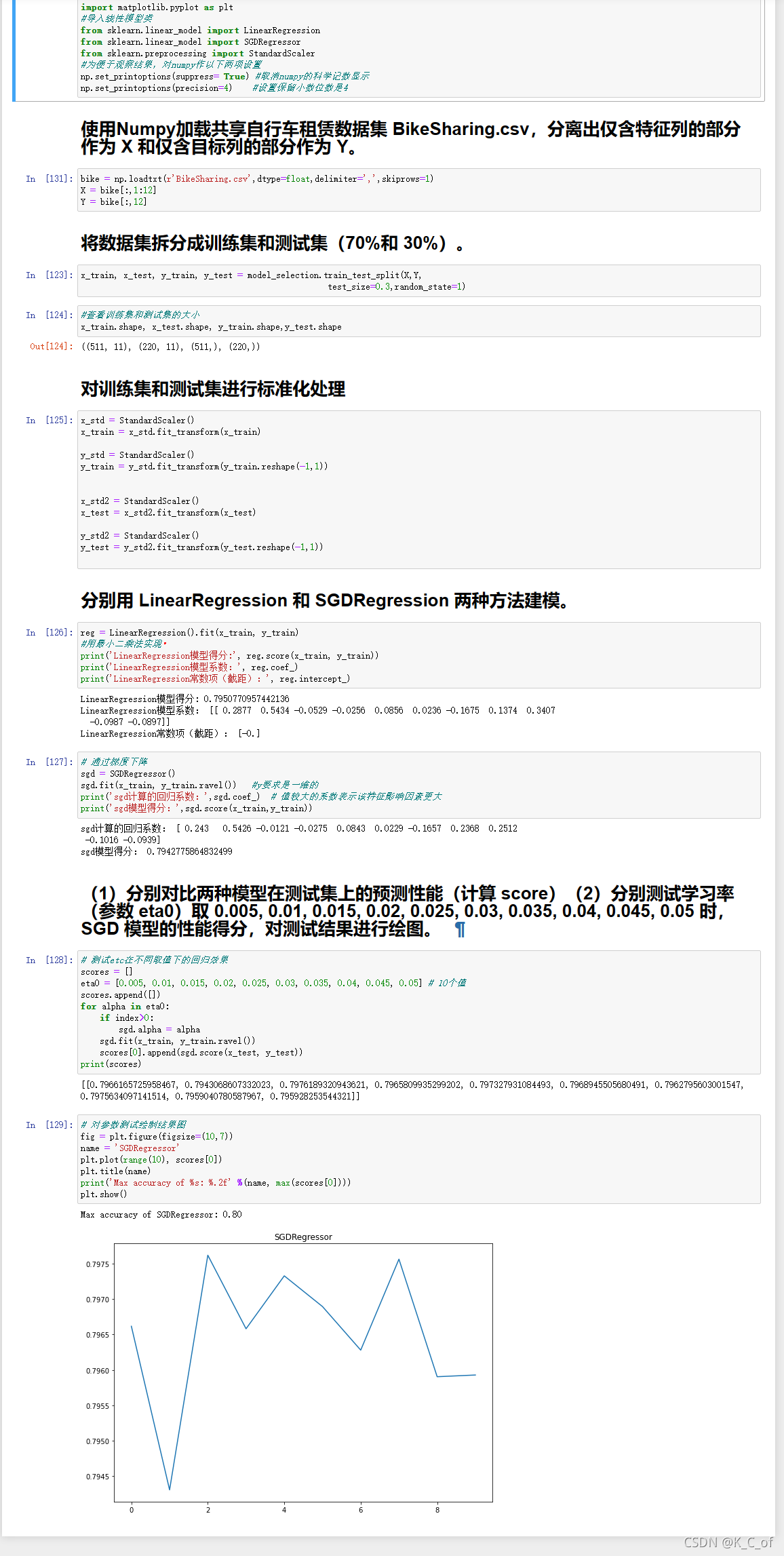
import numpy as np
from sklearn import model_selection
import matplotlib.pyplot as plt
from sklearn.linear_model import LinearRegression
from sklearn.linear_model import SGDRegressor
from sklearn.preprocessing import StandardScaler
np.set_printoptions(suppress= True)
np.set_printoptions(precision=4)
bike = np.loadtxt(r'BikeSharing.csv',dtype=float,delimiter=',',skiprows=1)
X = bike[:,1:12]
Y = bike[:,12]
print(X,X.shape)
print(Y,Y.shape)
x_train, x_test, y_train, y_test = model_selection.train_test_split(X,Y,
test_size=0.3,random_state=1)
x_train.shape, x_test.shape, y_train.shape,y_test.shape
x_std = StandardScaler()
x_train = x_std.fit_transform(x_train)
y_std = StandardScaler()
y_train = y_std.fit_transform(y_train.reshape(-1,1))
x_std2 = StandardScaler()
x_test = x_std2.fit_transform(x_test)
y_std2 = StandardScaler()
y_test = y_std2.fit_transform(y_test.reshape(-1,1))
reg = LinearRegression().fit(x_train, y_train)
print('LinearRegression模型得分:', reg.score(x_train, y_train))
print('LinearRegression模型系数:', reg.coef_)
print('LinearRegression常数项(截距):', reg.intercept_)
sgd = SGDRegressor()
sgd.fit(x_train, y_train.ravel())
print('sgd计算的回归系数:',sgd.coef_)
print('sgd模型得分:',sgd.score(x_train,y_train))
scores = []
eta0 = [0.005, 0.01, 0.015, 0.02, 0.025, 0.03, 0.035, 0.04, 0.045, 0.05]
scores.append([])
for alpha in eta0:
if index>0:
sgd.alpha = alpha
sgd.fit(x_train, y_train.ravel())
scores[0].append(sgd.score(x_test, y_test))
print(scores)
fig = plt.figure(figsize=(10,7))
name = 'SGDRegressor'
plt.plot(range(10), scores[0])
plt.title(name)
print('Max accuracy of %s: %.2f' %(name, max(scores[0])))
plt.show()
每次迭代结果都不一样,但是模型(最优)得分是一样的
|