一、代码实现
1.导入包
# 导入包
import numpy as np
import os
import pandas as pd
import cv2
import dlib
import os
from skimage import io
import csv
import sys
import winsound # 系统音效
import time
import random
定义数据存储文件
#存储位置
output_dir = '..\\source\\faces\\data\\zxs'
size = 64
#判断文件夹是否存在,不存在就创建
if not os.path.exists(output_dir):
os.makedirs(output_dir)
定义函数改变图片的亮度与对比度
# 改变图片的亮度与对比度
def relight(img, light=1, bias=0):
w = img.shape[1]
h = img.shape[0]
#image = []
for i in range(0,w):
for j in range(0,h):
for c in range(3):
tmp = int(img[j,i,c]*light + bias)
if tmp > 255:
tmp = 255
elif tmp < 0:
tmp = 0
img[j,i,c] = tmp
return img
定义函数获得人脸检测器和训练好的人脸68特征点检测器
#获得默认的人脸检测器和训练好的人脸68特征点检测器
def get_detector_and_predicyor():
#使用dlib自带的frontal_face_detector作为我们的特征提取器
detector = dlib.get_frontal_face_detector()
"""
功能:人脸检测画框
参数:PythonFunction和in Classes
in classes表示采样次数,次数越多获取的人脸的次数越多,但更容易框错
返回值是矩形的坐标,每个矩形为一个人脸(默认的人脸检测器)
"""
#返回训练好的人脸68特征点检测器
predictor = dlib.shape_predictor('..\\source\\shape_predictor_68_face_landmarks.dat')
return detector,predictor
获取检测器
#获取检测器
detector,predictor=get_detector_and_predicyor()
读取20张图片,存放到文件夹中,读取完了之后就可以在文件夹中看到图片了
camera = cv2.VideoCapture(0)#打开摄像头
ok=True
# 打开摄像头 参数为输入流,可以为摄像头或视频文件
index=1
while ok:
#读取图片
ok,img = camera.read()
# 转换成灰度图像
img_gray = cv2.cvtColor(img,cv2.COLOR_BGR2GRAY)
#使用detector进行人脸检测
dets=detector(img_gray,1)
for i, d in enumerate(dets):
x1 = d.top() if d.top() > 0 else 0
y1 = d.bottom() if d.bottom() > 0 else 0
x2 = d.left() if d.left() > 0 else 0
y2 = d.right() if d.right() > 0 else 0
face = img[x1:y1,x2:y2]
# 调整图片的对比度与亮度, 对比度与亮度值都取随机数,这样能增加样本的多样性
face = relight(face, random.uniform(0.5, 1.5), random.randint(-50, 50))
face = cv2.resize(face, (size,size))
cv2.imshow('image', face)
cv2.imwrite(output_dir+'/'+str(index)+'.jpg', face)
index += 1
k = cv2.waitKey(1)
if k == 27: # press 'ESC' to quit
break
if index==21:
break
camera.release()
cv2.destroyAllWindows()
# 返回单张图像的 128D 特征,128D特征指的是128维特征向量,是通过68个特征点还原出的人脸计算出的
def return_128d_features(path_img):
img_rd = io.imread(path_img)#读取图片
img_gray = cv2.cvtColor(img_rd, cv2.COLOR_BGR2RGB)
faces = detector(img_gray, 1)
print("%-40s %-20s" % ("检测到人脸的图像 / image with faces detected:", path_img), '\n')
# 因为有可能截下来的人脸再去检测,检测不出来人脸了
# 所以要确保是 检测到人脸的人脸图像 拿去算特征
if len(faces) != 0:
shape = predictor(img_gray, faces[0])#获得训练好的人脸68特征点检测器
face_descriptor = face_rec.compute_face_descriptor(img_gray, shape)#获得128维人脸特征向量
else:
face_descriptor = 0
print("no face")
return face_descriptor
# 将文件夹中照片特征提取出来, 写入 CSV
def return_features_mean_personX(path_faces_personX):
features_list_personX = []
photos_list = os.listdir(path_faces_personX)
print(path_faces_personX + "/" + photos_list[0])
if photos_list:
for i in range(len(photos_list)):
# 调用return_128d_features()得到128d特征
print("%-40s %-20s" % ("正在读的人脸图像 / image to read:", path_faces_personX + "\\" + photos_list[i]))
features_128d = return_128d_features(path_faces_personX + "\\" + photos_list[i])
# print(features_128d)
# 遇到没有检测出人脸的图片跳过
if features_128d == 0:
i += 1
else:
features_list_personX.append(features_128d)
else:
print("文件夹内图像文件为空 / Warning: No images in " + path_faces_personX + '/', '\n')
# 计算 128D 特征的均值
# N x 128D -> 1 x 128D
if features_list_personX:
features_mean_personX = np.array(features_list_personX).mean(axis=0)
else:
features_mean_personX = '0'
return features_mean_personX
读取人脸特征并存入scv文件中
# 读取某人所有的人脸图像的数据
feature_path='..\\source\\faces\\'+'feature\\'+'features_all.csv'
with open(feature_path, "w", newline="") as csvfile:
writer = csv.writer(csvfile)
# Get the mean/average features of face/personX, it will be a list with a length of 128D
features_mean_personX = return_features_mean_personX(output_dir)
writer.writerow(features_mean_personX)
print("特征均值 / The mean of features:", list(features_mean_personX))
print('\n')
print("所有录入人脸数据存入 ")
进行实时检测了,实时检测要计算欧氏距离,所以先定义一个计算欧氏距离的函数
#计算欧氏距离
def return_euclidean_distance(feature_1, feature_2):
feature_1 = np.array(feature_1)
feature_2 = np.array(feature_2)
dist = np.sqrt(np.sum(np.square(feature_1 - feature_2)))
return dist
义一些要使用的变量,人脸检测器记得重定义一下,不重定义识别可能有问题
# 用来存放所有录入人脸特征的数组
# the array to save the features of faces in the database
features_known_arr = []
#读取人脸数据集
csv_rd = pd.read_csv(feature_path, header=None)
#重定义检测器
detector = dlib.get_frontal_face_detector()
#将数据集保存在list里
for i in range(csv_rd.shape[0]):
features_someone_arr = []
for j in range(0, len(csv_rd.iloc[i, :])):
features_someone_arr.append(csv_rd.iloc[i, :][j])
features_known_arr.append(features_someone_arr)
print("Faces in Database:", len(features_known_arr))
实时检测并保存检测的图片
#创建cv2摄像头对象
cap = cv2.VideoCapture(0)
# 设置视频参数,propId 设置的视频参数,value 设置的参数值
cap.set(3, 480)
while cap.isOpened():
flag, img_rd = cap.read()
kk = cv2.waitKey(1)
# 取灰度
img_gray = cv2.cvtColor(img_rd, cv2.COLOR_RGB2GRAY)
# 人脸数 faces
faces = detector(img_gray, 0)
# 待会要写的字体 font to write later
font = cv2.FONT_HERSHEY_COMPLEX
# 存储当前摄像头中捕获到的所有人脸的坐标/名字
# the list to save the positions and names of current faces captured
pos_namelist = []
name_namelist = []
# 按下 q 键退出
# press 'q' to exit
if kk == ord('q'):
break
else:
# 检测到人脸 when face detected
if len(faces) != 0:
# 获取当前捕获到的图像的所有人脸的特征,存储到 features_cap_arr
# get the features captured and save into features_cap_arr
features_cap_arr = []
for i in range(len(faces)):
shape = predictor(img_rd, faces[i])
features_cap_arr.append(face_rec.compute_face_descriptor(img_rd, shape))
# 遍历捕获到的图像中所有的人脸
# traversal all the faces in the database
for k in range(len(faces)):
print("##### camera person", k+1, "#####")
# 让人名跟随在矩形框的下方
# 确定人名的位置坐标
# 先默认所有人不认识,是 unknown
# set the default names of faces with "unknown"
name_namelist.append("unknown")
# 每个捕获人脸的名字坐标 the positions of faces captured
pos_namelist.append(tuple([faces[k].left(), int(faces[k].bottom() + (faces[k].bottom() - faces[k].top())/4)]))
# 对于某张人脸,遍历所有存储的人脸特征
# for every faces detected, compare the faces in the database
e_distance_list = []
for i in range(len(features_known_arr)):
# 如果 person_X 数据不为空
if str(features_known_arr[i][0]) != '0.0':
print("with person", str(i + 1), "the e distance: ", end='')
e_distance_tmp = return_euclidean_distance(features_cap_arr[k], features_known_arr[i])
print(e_distance_tmp)
e_distance_list.append(e_distance_tmp)
else:
# 空数据 person_X
e_distance_list.append(999999999)
# 找出最接近的一个人脸数据是第几个
# Find the one with minimum e distance
similar_person_num = e_distance_list.index(min(e_distance_list))
print("Minimum e distance with person", int(similar_person_num)+1)
# 计算人脸识别特征与数据集特征的欧氏距离
# 距离小于0.4则标出为可识别人物
if min(e_distance_list) < 0.4:
# 这里可以修改摄像头中标出的人名
# Here you can modify the names shown on the camera
# 1、遍历文件夹目录
folder_name = '..\\source\\faces\\data'
# 最接近的人脸
sum=similar_person_num+1
key_id=1 # 从第一个人脸数据文件夹进行对比
# 获取文件夹中的文件名:LQH、YYQX、WY、WL...
file_names = os.listdir(folder_name)
for name in file_names:
# print(name+'->'+str(key_id))
if sum ==key_id:
#winsound.Beep(300,500)# 响铃:300频率,500持续时间
name_namelist[k] = name[0:]#人名删去第一个数字(用于视频输出标识)
key_id += 1
# 播放欢迎光临音效
#playsound('D:/myworkspace/JupyterNotebook/People/music/welcome.wav')
# print("May be person "+str(int(similar_person_num)+1))
# -----------筛选出人脸并保存到visitor文件夹------------
for i, d in enumerate(faces):
x1 = d.top() if d.top() > 0 else 0
y1 = d.bottom() if d.bottom() > 0 else 0
x2 = d.left() if d.left() > 0 else 0
y2 = d.right() if d.right() > 0 else 0
face = img_rd[x1:y1,x2:y2]
size = 64
face = cv2.resize(face, (size,size))
# 要存储visitor人脸图像文件的路径
path_visitors_save_dir = "..\\source\\faces\\visitor\\know"
# 存储格式:2019-06-24-14-33-40LQH.jpg
now_time = time.strftime("%Y-%m-%d-%H-%M-%S", time.localtime())
save_name = str(now_time)+str(name_namelist[k])+'.jpg'
# print(save_name)
# 本次图片保存的完整url
save_path = path_visitors_save_dir+'\\'+ save_name
# 遍历visitor文件夹所有文件名
visitor_names = os.listdir(path_visitors_save_dir)
visitor_name=''
for name in visitor_names:
# 名字切片到分钟数:2019-06-26-11-33-00LQH.jpg
visitor_name=(name[0:16]+'-00'+name[19:])
# print(visitor_name)
visitor_save=(save_name[0:16]+'-00'+save_name[19:])
# print(visitor_save)
# 一分钟之内重复的人名不保存
if visitor_save!=visitor_name:
cv2.imwrite(save_path, face)
print('新存储:'+path_visitors_save_dir+'/'+str(now_time)+str(name_namelist[k])+'.jpg')
else:
print('重复,未保存!')
else:
# 播放无法识别音效
#playsound('D:/myworkspace/JupyterNotebook/People/music/sorry.wav')
print("Unknown person")
# -----保存图片-------
# -----------筛选出人脸并保存到visitor文件夹------------
for i, d in enumerate(faces):
x1 = d.top() if d.top() > 0 else 0
y1 = d.bottom() if d.bottom() > 0 else 0
x2 = d.left() if d.left() > 0 else 0
y2 = d.right() if d.right() > 0 else 0
face = img_rd[x1:y1,x2:y2]
size = 64
face = cv2.resize(face, (size,size))
# 要存储visitor-》unknown人脸图像文件的路径
path_visitors_save_dir = "..\\source\\faces\\visitor\\unknow"
# 存储格式:2019-06-24-14-33-40unknown.jpg
now_time = time.strftime("%Y-%m-%d-%H-%M-%S", time.localtime())
# print(save_name)
# 本次图片保存的完整url
save_path = path_visitors_save_dir+'\\'+ str(now_time)+'unknown.jpg'
print(save_path)
cv2.imwrite(save_path, face)
print('新存储:'+path_visitors_save_dir+'/'+str(now_time)+'unknown.jpg')
# 矩形框
# draw rectangle
for kk, d in enumerate(faces):
# 绘制矩形框
cv2.rectangle(img_rd, tuple([d.left(), d.top()]), tuple([d.right(), d.bottom()]), (0, 255, 255), 2)
print('\n')
# 在人脸框下面写人脸名字
# write names under rectangle
for i in range(len(faces)):
cv2.putText(img_rd, name_namelist[i], pos_namelist[i], font, 0.8, (0, 255, 255), 1, cv2.LINE_AA)
print("Faces in camera now:", name_namelist, "\n")
#cv2.putText(img_rd, "Press 'q': Quit", (20, 450), font, 0.8, (84, 255, 159), 1, cv2.LINE_AA)
cv2.putText(img_rd, "Face Recognition", (20, 40), font, 1, (0, 0, 255), 1, cv2.LINE_AA)
cv2.putText(img_rd, "Visitors: " + str(len(faces)), (20, 100), font, 1, (0, 0, 255), 1, cv2.LINE_AA)
# 窗口显示 show with opencv
cv2.imshow("camera", img_rd)
# 释放摄像头 release camera
cap.release()
# 删除建立的窗口 delete all the windows
cv2.destroyAllWindows()
效果
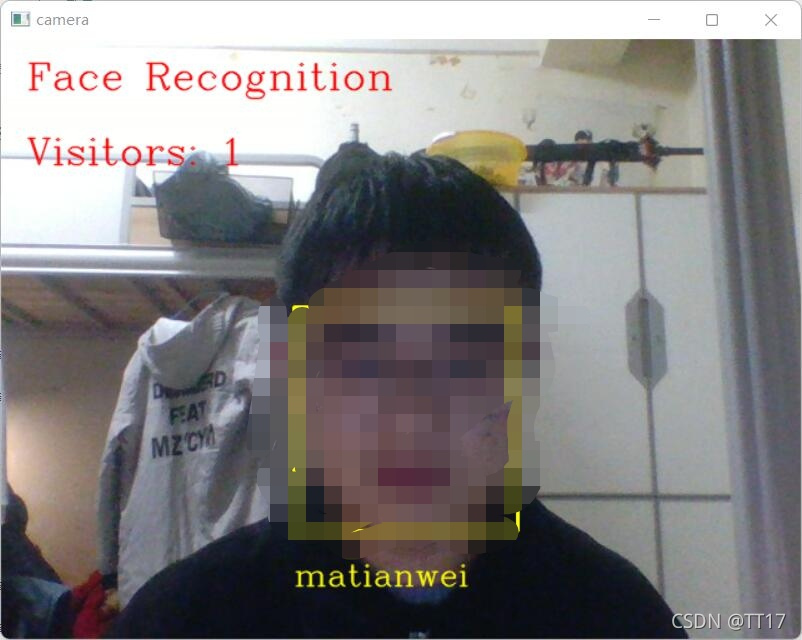
?二、总结
128D特征向量并不是通过68个特征点计算出,而是通过68个特征点还原出脸,再通过神经网络来计算的
三、参考
利用dlib库实现人脸识别
|