一.Libsvm
LIBSVM是台湾大学林智仁(LinChih-Jen)教授等开发设计的一个简单、易于使用和快速有效的SVM模式识别与回归的软件包,他不但提供了编译好的可在Windows系列系统的执行文件,还提供了源代码,方便改进、修改以及在其它操作系统上应用;该软件对SVM所涉及的参数调节相对比较少,提供了很多的默认参数,利用这些默认参数可以解决很多问题;并提供了交互检验(CrossValidation)的功能。该软件可以解决C-SVM、ν-SVM、ε-SVR和ν-SVR等问题,包括基于一对一算法的多类模式识别问题。
官网下载j解压后如下:LibSvm 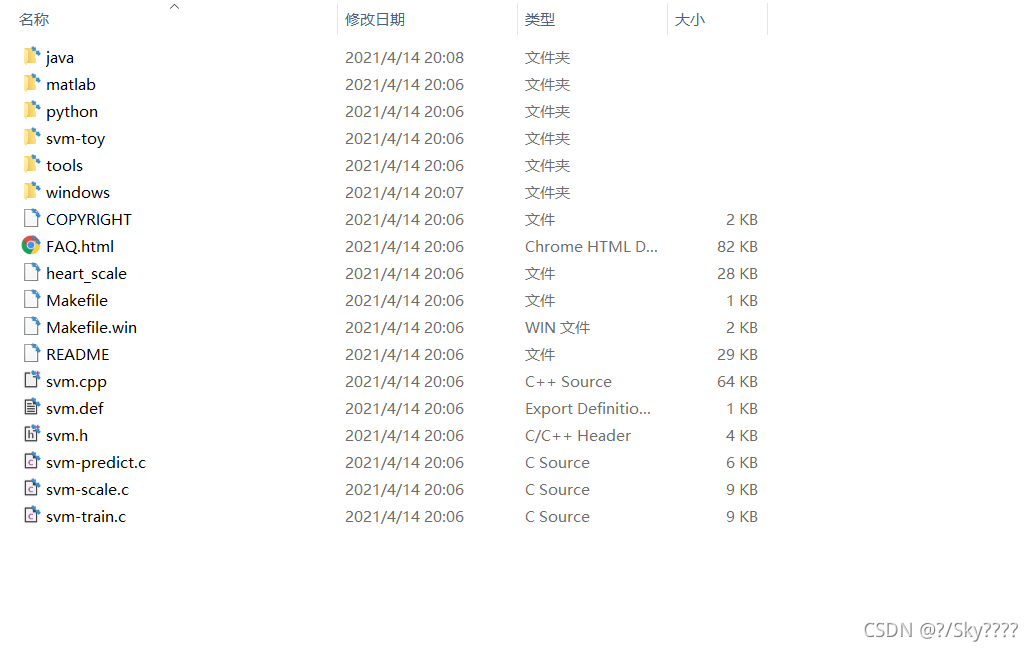
使用步骤
1) 按照LIBSVM软件包所要求的格式准备数据集; 2) 对数据进行简单的缩放操作; 3) 考虑选用RBF 核函数; 4) 采用交叉验证选择最佳参数C与g ; 5) 采用最佳参数C与g 对整个训练集进行训练获取支持向量机模型; 6) 利用获取的模型进行测试与预测。
数据格式
该软件使用的训练数据和检验数据文件格式如下: 
其中<label> 是训练数据集的目标值,对于分类,它是标识某类的整数(支持多个类);对于回归,是任意实数。<index> 是以1开始的整数,可以是不连续的;<value> ;为实数,也就是我们常说的自变量。检验数据文件中的label只用于计算准确度或误差,如果它是未知的,只需用一个数填写这一栏,也可以空着不填。在程序包中,还包括有一个训练数据实例:heart_scale,方便参考数据文件格式以及练习使用软件。 可以编写小程序,将自己常用的数据格式转换成这种格式。其中formatdatalibsvm.xls文件可以方便的将excel数据转化为符合LIBSVM要求的数据格式。
创建项目
先建好一个java项目 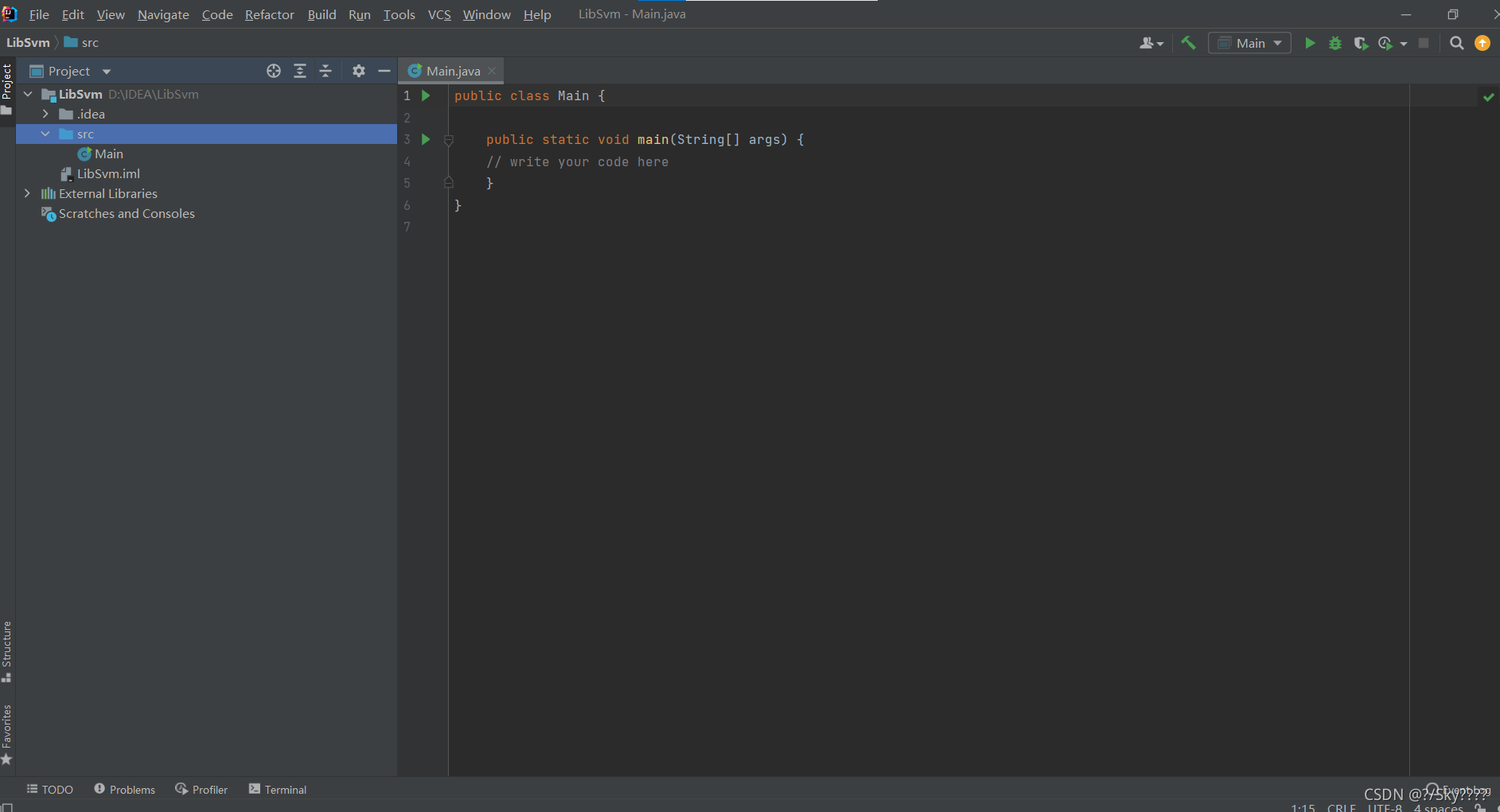 在将java文件夹下的文件导入 新建test文件夹存放数据 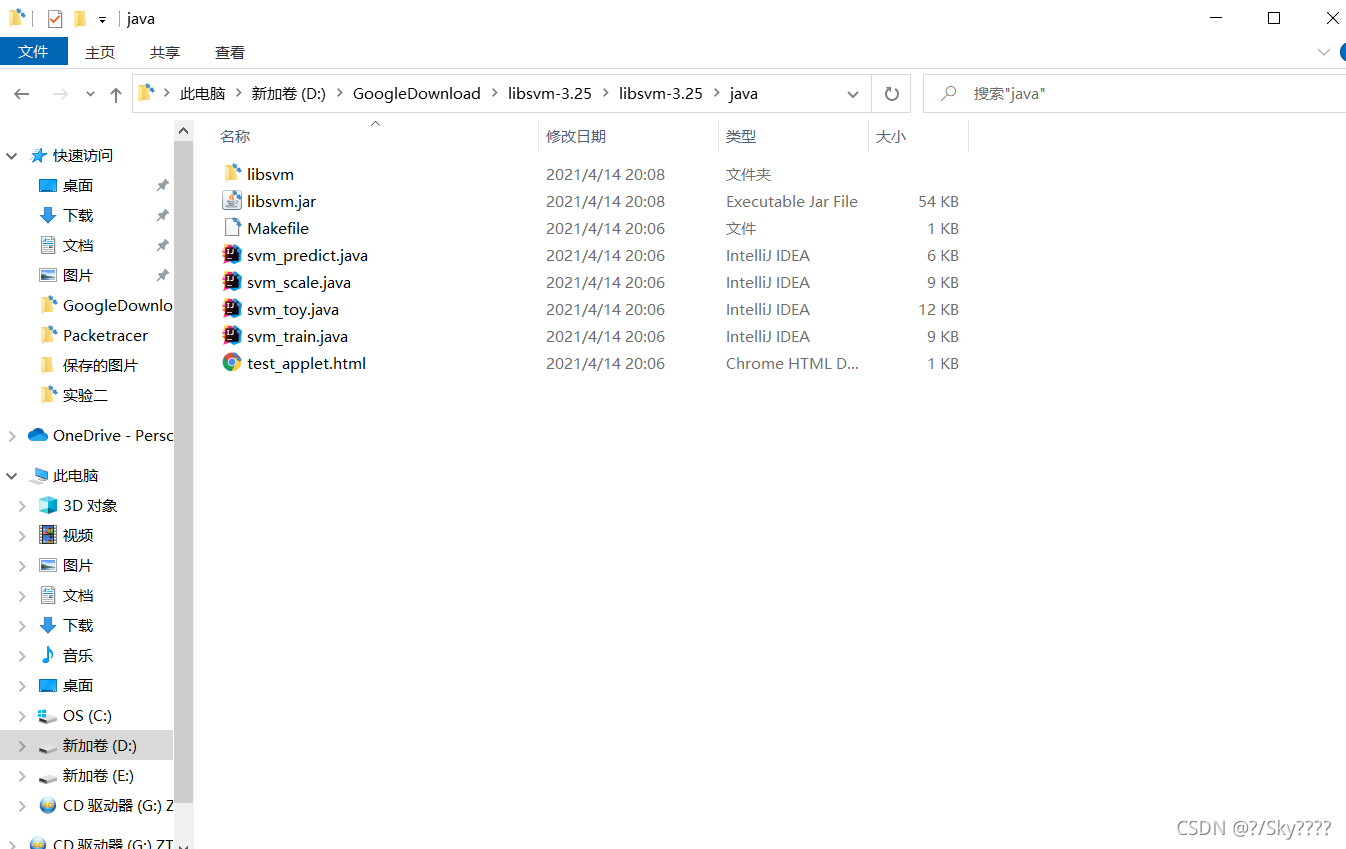 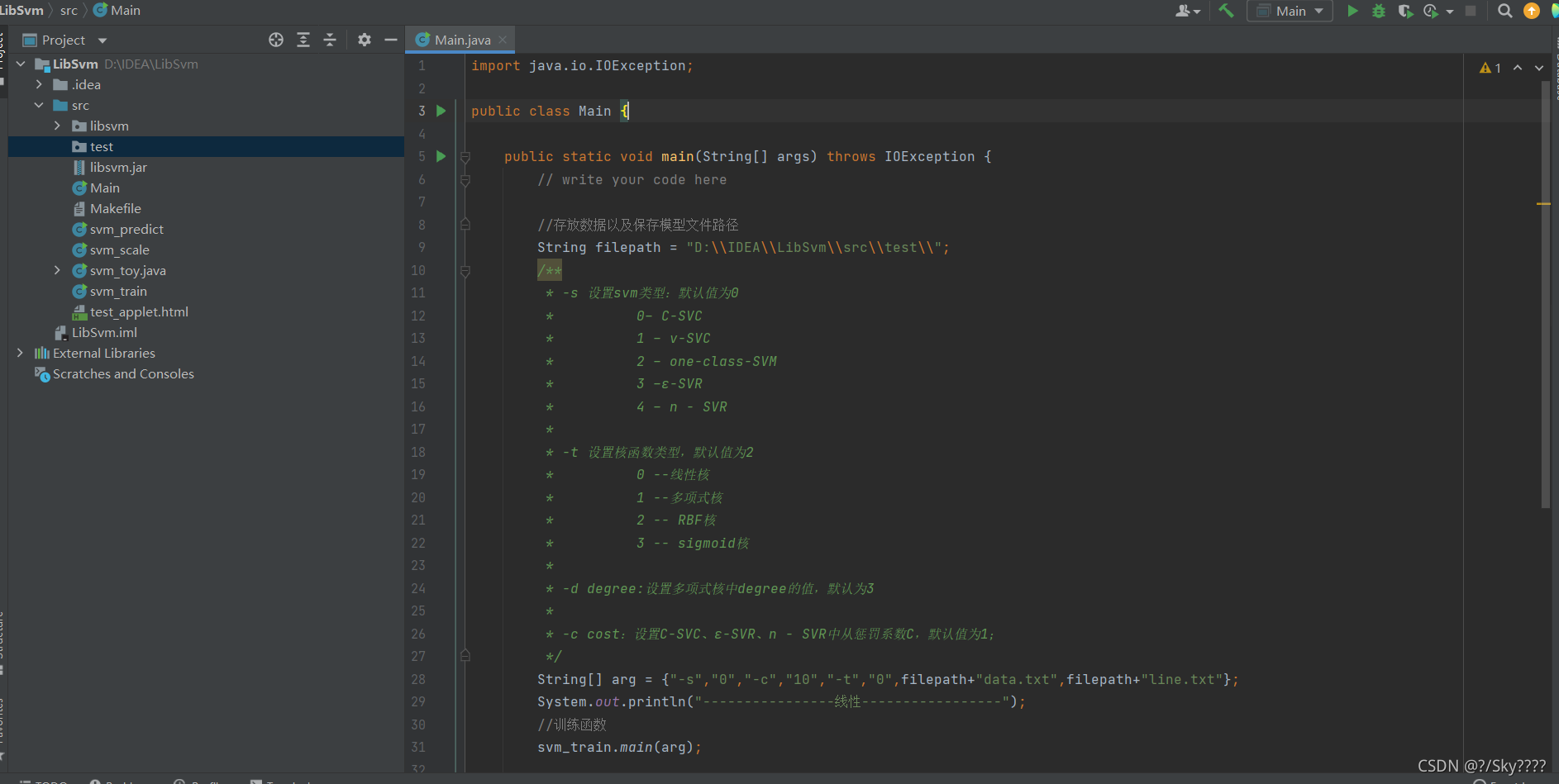 打开工具进行训练 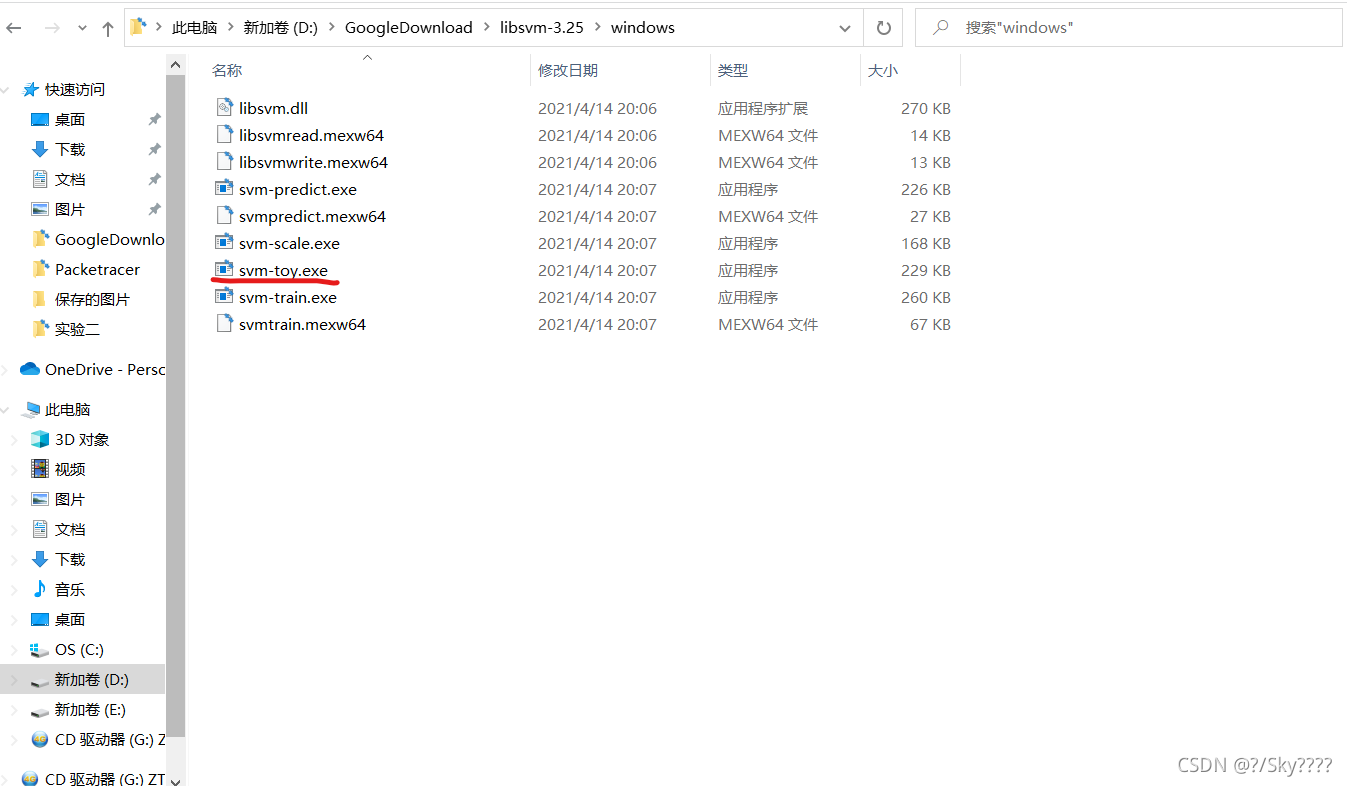 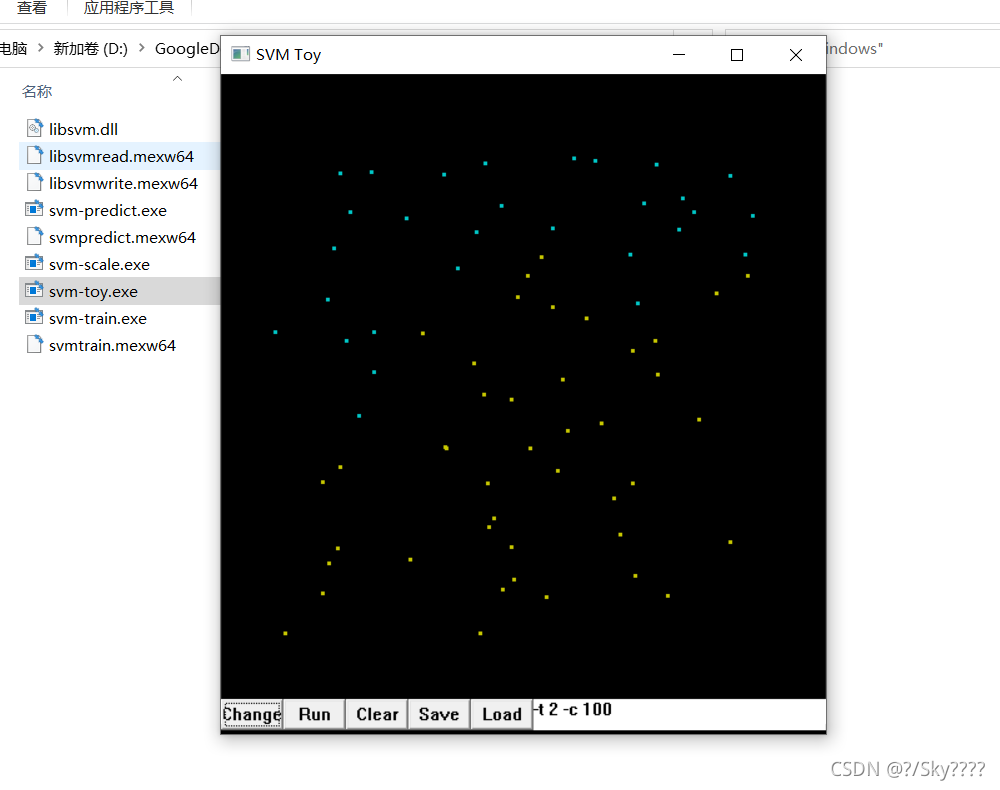
运行程序,得出结果 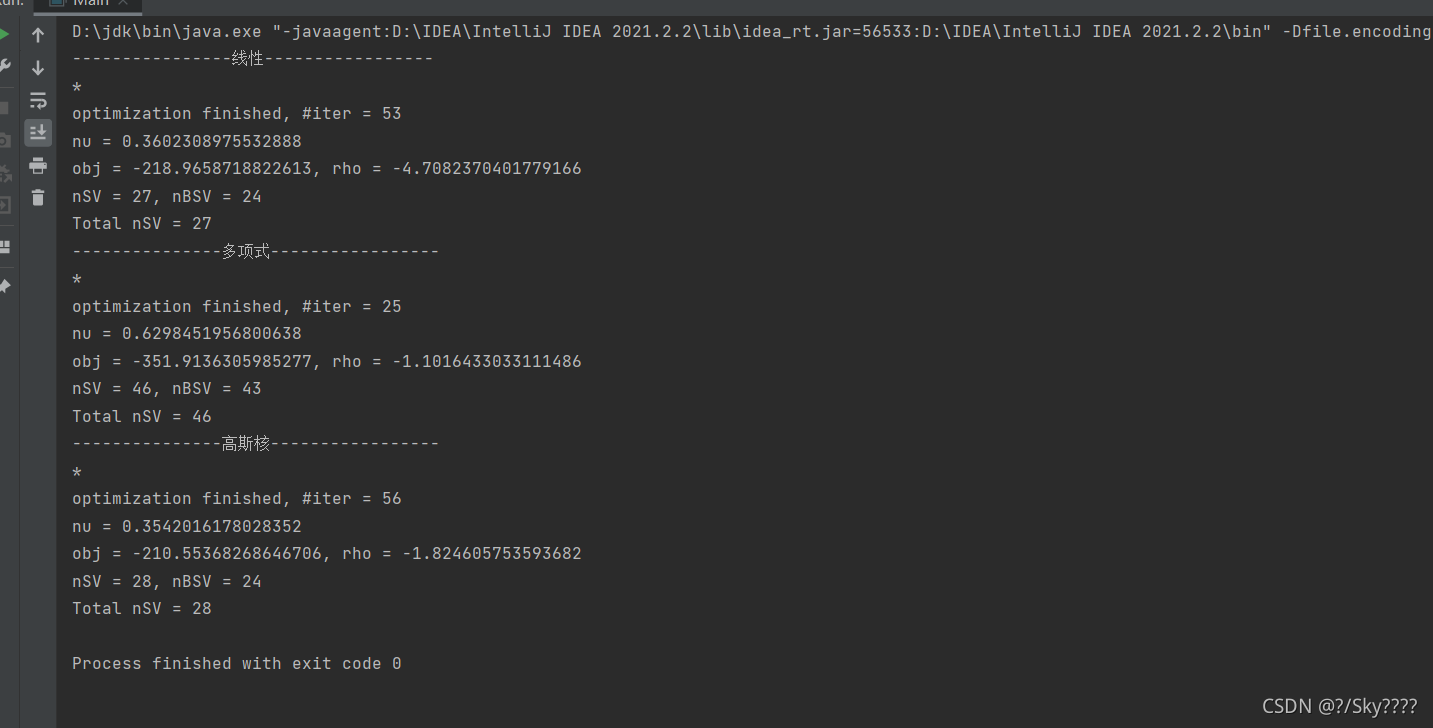 此时test文件夹下出现了三个新的txt文件 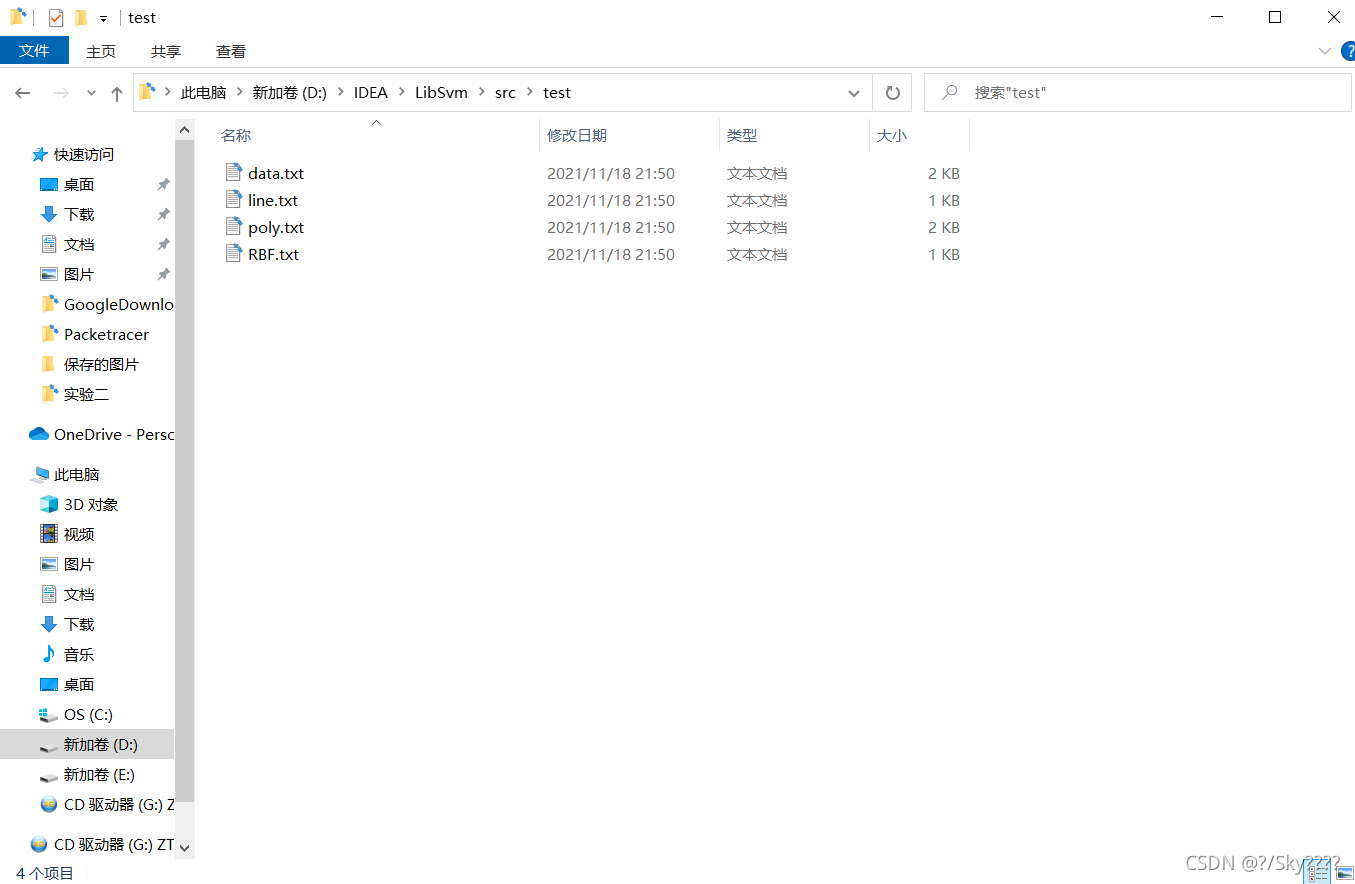
文件数据
svm_type 所选择的svm类型,默认为c_svc kernel_type 训练采用的核函数类型,此处为RBF核 gamma RBF核的参数γ nr_class 类别数 total_sv 支持向量总个数 rho 判决函数的偏置项b label 原始文件中的类别标识 nr_sv 每个类的支持向量机的个数 SV 各个类的权系数及相应的支持向量
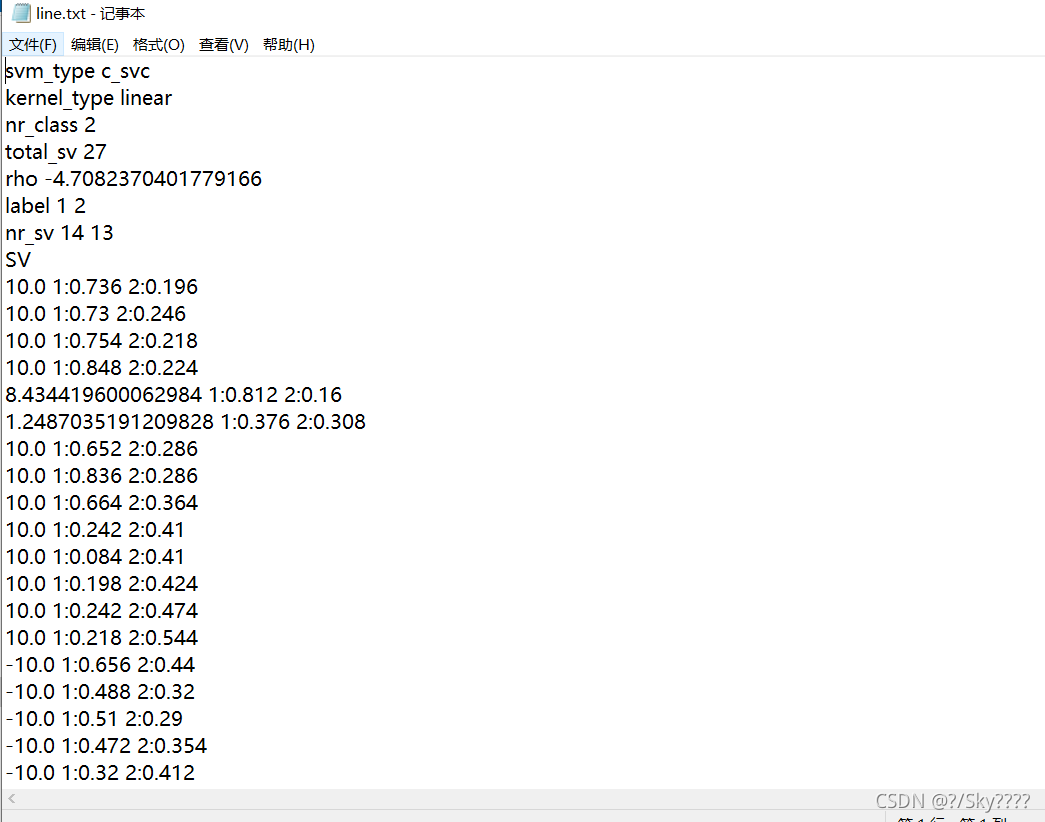
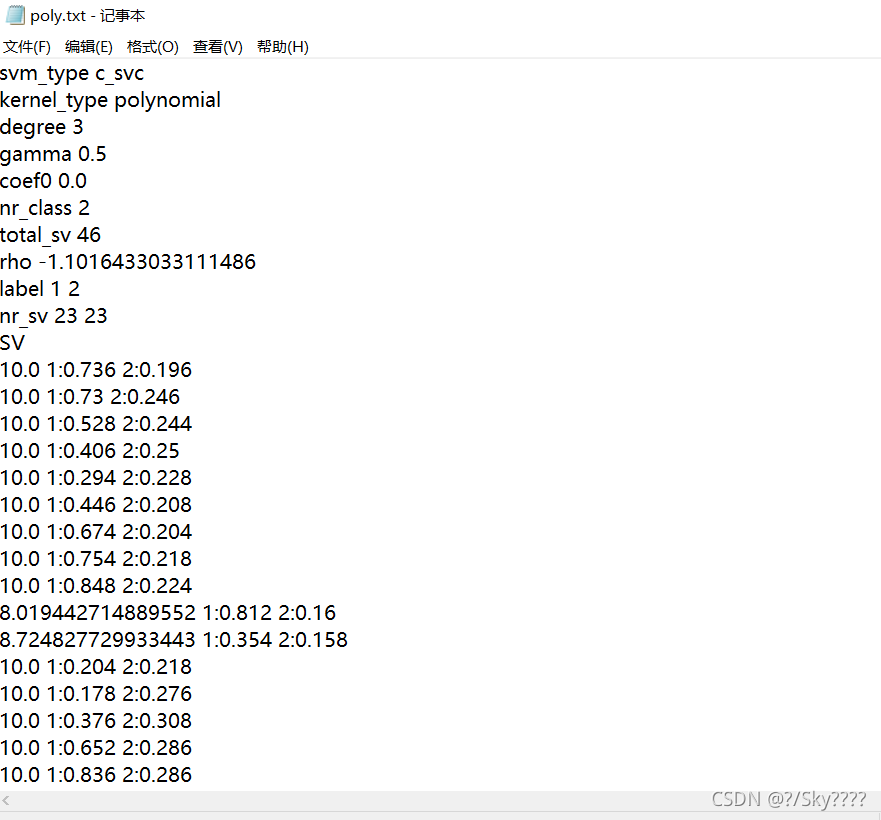
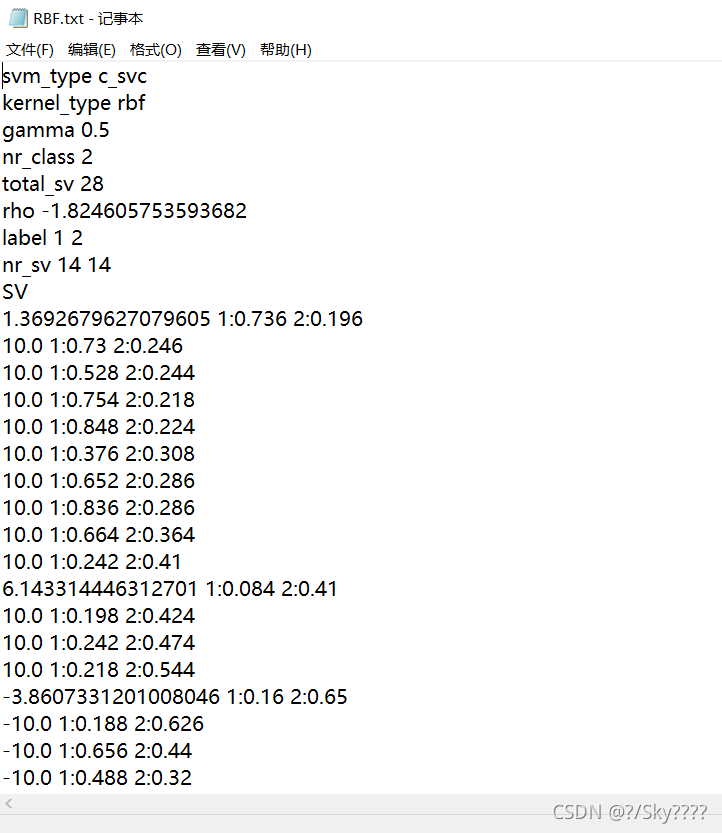
二.人脸识别数据集的建立
收集20张照片
import cv2
import dlib
import os
import sys
import random
output_dir = 'D:/30612/Pictures/Camera Roll'
size = 64
if not os.path.exists(output_dir):
os.makedirs(output_dir)
def relight(img, light=1, bias=0):
w = img.shape[1]
h = img.shape[0]
for i in range(0,w):
for j in range(0,h):
for c in range(3):
tmp = int(img[j,i,c]*light + bias)
if tmp > 255:
tmp = 255
elif tmp < 0:
tmp = 0
img[j,i,c] = tmp
return img
detector = dlib.get_frontal_face_detector()
camera = cv2.VideoCapture(0)
index = 1
while True:
if (index <= 20):
print('Being processed picture %s' % index)
success, img = camera.read()
gray_img = cv2.cvtColor(img, cv2.COLOR_BGR2GRAY)
dets = detector(gray_img, 1)
for i, d in enumerate(dets):
x1 = d.top() if d.top() > 0 else 0
y1 = d.bottom() if d.bottom() > 0 else 0
x2 = d.left() if d.left() > 0 else 0
y2 = d.right() if d.right() > 0 else 0
face = img[x1:y1,x2:y2]
face = relight(face, random.uniform(0.5, 1.5), random.randint(-50, 50))
face = cv2.resize(face, (size,size))
cv2.imshow('image', face)
cv2.imwrite(output_dir+'/'+str(index)+'.jpg', face)
index += 1
key = cv2.waitKey(30) & 0xff
if key == 27:
break
else:
print('Finished!')
camera.release()
cv2.destroyAllWindows()
break
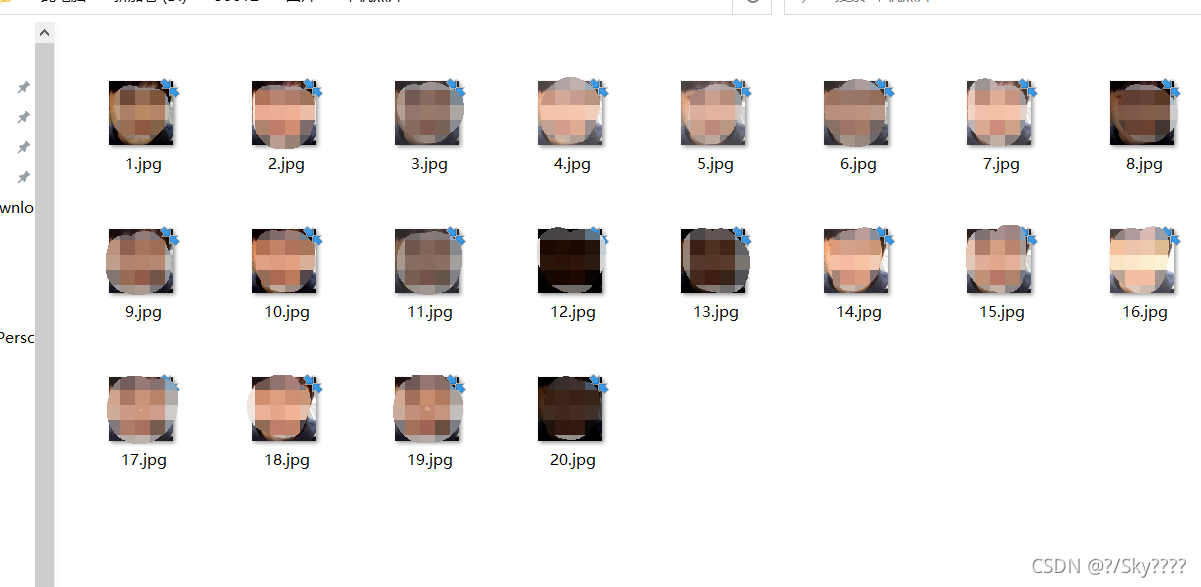
采集对应20张图片的68个特征点数组
import cv2
import os
import dlib
from skimage import io
import csv
import numpy as np
path_images_from_camera = "D:/30612/Pictures/Camera Roll/person/"
detector = dlib.get_frontal_face_detector()
predictor = dlib.shape_predictor("D:/GoogleDownload/shape_predictor_68_face_landmarks.dat")
face_rec = dlib.face_recognition_model_v1("D:/GoogleDownload/dlib_face_recognition_resnet_model_v1.dat")
def return_128d_features(path_img):
img_rd = io.imread(path_img)
img_gray = cv2.cvtColor(img_rd, cv2.COLOR_BGR2RGB)
faces = detector(img_gray, 1)
print("%-40s %-20s" % ("检测到人脸的图像 / image with faces detected:", path_img), '\n')
if len(faces) != 0:
shape = predictor(img_gray, faces[0])
face_descriptor = face_rec.compute_face_descriptor(img_gray, shape)
else:
face_descriptor = 0
print("no face")
return face_descriptor
def return_features_mean_personX(path_faces_personX):
features_list_personX = []
photos_list = os.listdir(path_faces_personX)
if photos_list:
for i in range(len(photos_list)):
print("%-40s %-20s" % ("正在读的人脸图像 / image to read:", path_faces_personX + "/" + photos_list[i]))
features_128d = return_128d_features(path_faces_personX + "/" + photos_list[i])
if features_128d == 0:
i += 1
else:
features_list_personX.append(features_128d)
i1=str(i+1)
add="D:/30612/Pictures/Camera Roll/feature/face_feature"+i1+".csv"
print(add)
with open(add, "w", newline="") as csvfile:
writer1 = csv.writer(csvfile)
writer1.writerow(features_128d)
else:
print("文件夹内图像文件为空 / Warning: No images in " + path_faces_personX + '/', '\n')
if features_list_personX:
features_mean_personX = np.array(features_list_personX).mean(axis=0)
else:
features_mean_personX = '0'
return features_mean_personX
people = os.listdir(path_images_from_camera)
people.sort()
with open("D:/30612/Pictures/Camera Roll/feature/features2_all.csv", "w", newline="") as csvfile:
writer = csv.writer(csvfile)
for person in people:
print("##### " + person + " #####")
features_mean_personX = return_features_mean_personX(path_images_from_camera + person)
writer.writerow(features_mean_personX)
print("特征均值 / The mean of features:", list(features_mean_personX))
print('\n')
print("所有录入人脸数据存入 / Save all the features of faces registered into: D:/30612/Pictures/Camera Roll/feature/features_all2.csv")
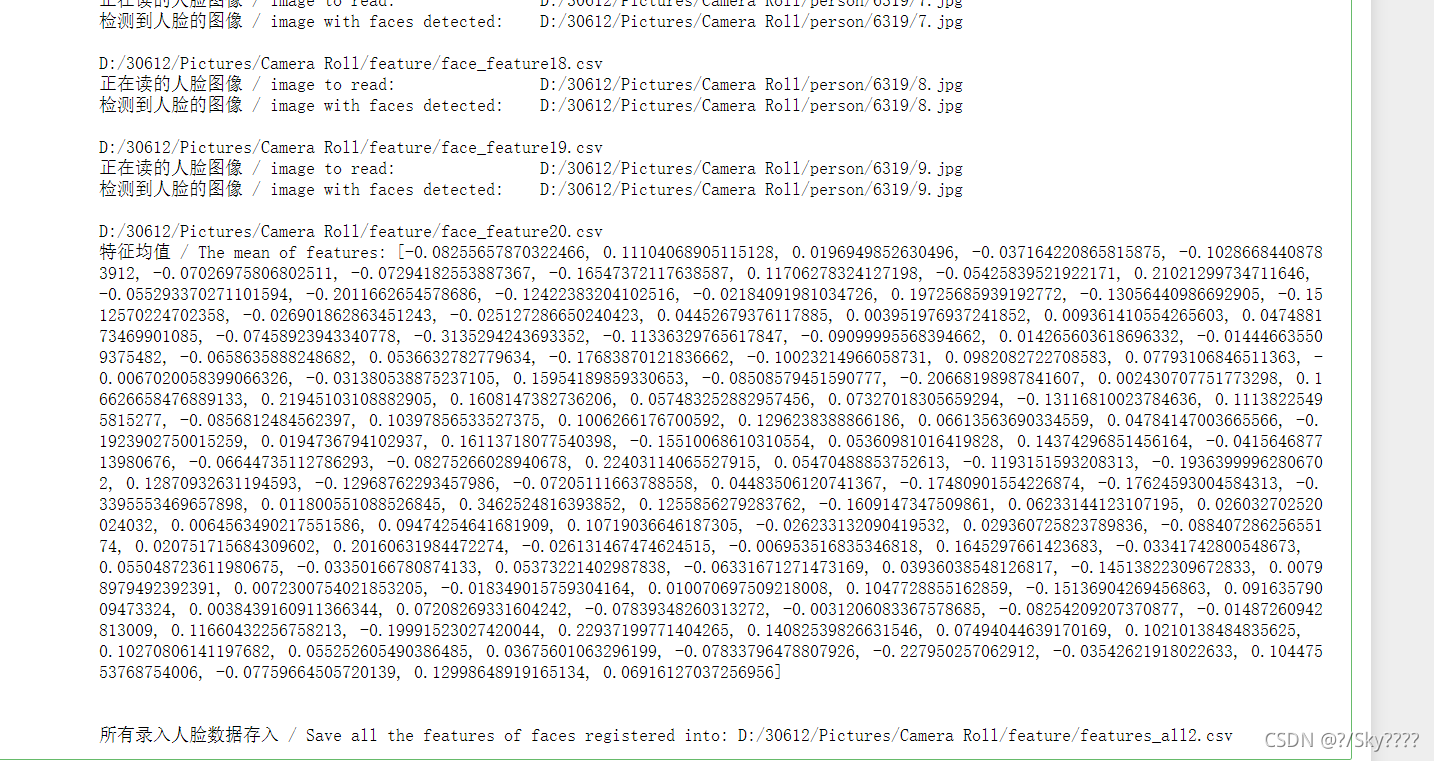
通过20个特征,计算出平均(mean)特征数组 face_feature_mean.txt.
import os
import winsound
from playsound import playsound
import dlib
import csv
import time
import sys
import numpy as np
import cv2
import pandas as pd
facerec = dlib.face_recognition_model_v1("D:/GoogleDownload/dlib_face_recognition_resnet_model_v1.dat")
def return_euclidean_distance(feature_1, feature_2):
feature_1 = np.array(feature_1)
feature_2 = np.array(feature_2)
dist = np.sqrt(np.sum(np.square(feature_1 - feature_2)))
return dist
path_features_known_csv = "D:/30612/Pictures/Camera Roll/feature/features2_all.csv"
csv_rd = pd.read_csv(path_features_known_csv, header=None)
features_known_arr = []
for i in range(csv_rd.shape[0]):
features_someone_arr = []
for j in range(0, len(csv_rd.iloc[i, :])):
features_someone_arr.append(csv_rd.iloc[i, :][j])
features_known_arr.append(features_someone_arr)
print("Faces in Database:", len(features_known_arr))
detector = dlib.get_frontal_face_detector()
predictor = dlib.shape_predictor('D:/GoogleDownload/shape_predictor_68_face_landmarks.dat')
cap = cv2.VideoCapture(0)
cap.set(3, 480)
while cap.isOpened():
flag, img_rd = cap.read()
kk = cv2.waitKey(1)
img_gray = cv2.cvtColor(img_rd, cv2.COLOR_RGB2GRAY)
faces = detector(img_gray, 0)
font = cv2.FONT_HERSHEY_COMPLEX
pos_namelist = []
name_namelist = []
if kk == ord('q'):
break
else:
if len(faces) != 0:
features_cap_arr = []
for i in range(len(faces)):
shape = predictor(img_rd, faces[i])
features_cap_arr.append(facerec.compute_face_descriptor(img_rd, shape))
for k in range(len(faces)):
print("##### camera person", k+1, "#####")
name_namelist.append("unknown")
pos_namelist.append(tuple([faces[k].left(), int(faces[k].bottom() + (faces[k].bottom() - faces[k].top())/4)]))
e_distance_list = []
for i in range(len(features_known_arr)):
if str(features_known_arr[i][0]) != '0.0':
print("with person", str(i + 1), "the e distance: ", end='')
e_distance_tmp = return_euclidean_distance(features_cap_arr[k], features_known_arr[i])
print(e_distance_tmp)
e_distance_list.append(e_distance_tmp)
else:
e_distance_list.append(999999999)
similar_person_num = e_distance_list.index(min(e_distance_list))
print("Minimum e distance with person", int(similar_person_num)+1)
if min(e_distance_list) < 0.4:
folder_name = 'D:/30612/Pictures/Camera Roll/person'
sum=similar_person_num+1
key_id=1
file_names = os.listdir(folder_name)
for name in file_names:
if sum ==key_id:
name_namelist[k] = name[0:]
key_id += 1
for i, d in enumerate(faces):
x1 = d.top() if d.top() > 0 else 0
y1 = d.bottom() if d.bottom() > 0 else 0
x2 = d.left() if d.left() > 0 else 0
y2 = d.right() if d.right() > 0 else 0
face = img_rd[x1:y1,x2:y2]
size = 64
face = cv2.resize(face, (size,size))
path_visitors_save_dir = "D:/30612/Pictures/Camera Roll/person/know"
now_time = time.strftime("%Y-%m-%d-%H-%M-%S", time.localtime())
save_name = str(now_time)+str(name_namelist[k])+'.jpg'
save_path = path_visitors_save_dir+'/'+ save_name
visitor_names = os.listdir(path_visitors_save_dir)
visitor_name=''
for name in visitor_names:
visitor_name=(name[0:16]+'-00'+name[19:])
visitor_save=(save_name[0:16]+'-00'+save_name[19:])
if visitor_save!=visitor_name:
cv2.imwrite(save_path, face)
print('新存储:'+path_visitors_save_dir+'/'+str(now_time)+str(name_namelist[k])+'.jpg')
else:
print('重复,未保存!')
else:
print("Unknown person")
for i, d in enumerate(faces):
x1 = d.top() if d.top() > 0 else 0
y1 = d.bottom() if d.bottom() > 0 else 0
x2 = d.left() if d.left() > 0 else 0
y2 = d.right() if d.right() > 0 else 0
face = img_rd[x1:y1,x2:y2]
size = 64
face = cv2.resize(face, (size,size))
path_visitors_save_dir = "D:/30612/Pictures/Camera Roll/person/unknow"
now_time = time.strftime("%Y-%m-%d-%H-%M-%S", time.localtime())
save_path = path_visitors_save_dir+'/'+ str(now_time)+'unknown.jpg'
cv2.imwrite(save_path, face)
print('新存储:'+path_visitors_save_dir+'/'+str(now_time)+'unknown.jpg')
for kk, d in enumerate(faces):
cv2.rectangle(img_rd, tuple([d.left(), d.top()]), tuple([d.right(), d.bottom()]), (0, 255, 255), 2)
print('\n')
for i in range(len(faces)):
cv2.putText(img_rd, name_namelist[i], pos_namelist[i], font, 0.8, (0, 255, 255), 1, cv2.LINE_AA)
print("Faces in camera now:", name_namelist, "\n")
cv2.putText(img_rd, "Face Recognition", (20, 40), font, 1, (0, 0, 255), 1, cv2.LINE_AA)
cv2.putText(img_rd, "Visitors: " + str(len(faces)), (20, 100), font, 1, (0, 0, 255), 1, cv2.LINE_AA)
cv2.imshow("camera", img_rd)
cap.release()
cv2.destroyAllWindows()
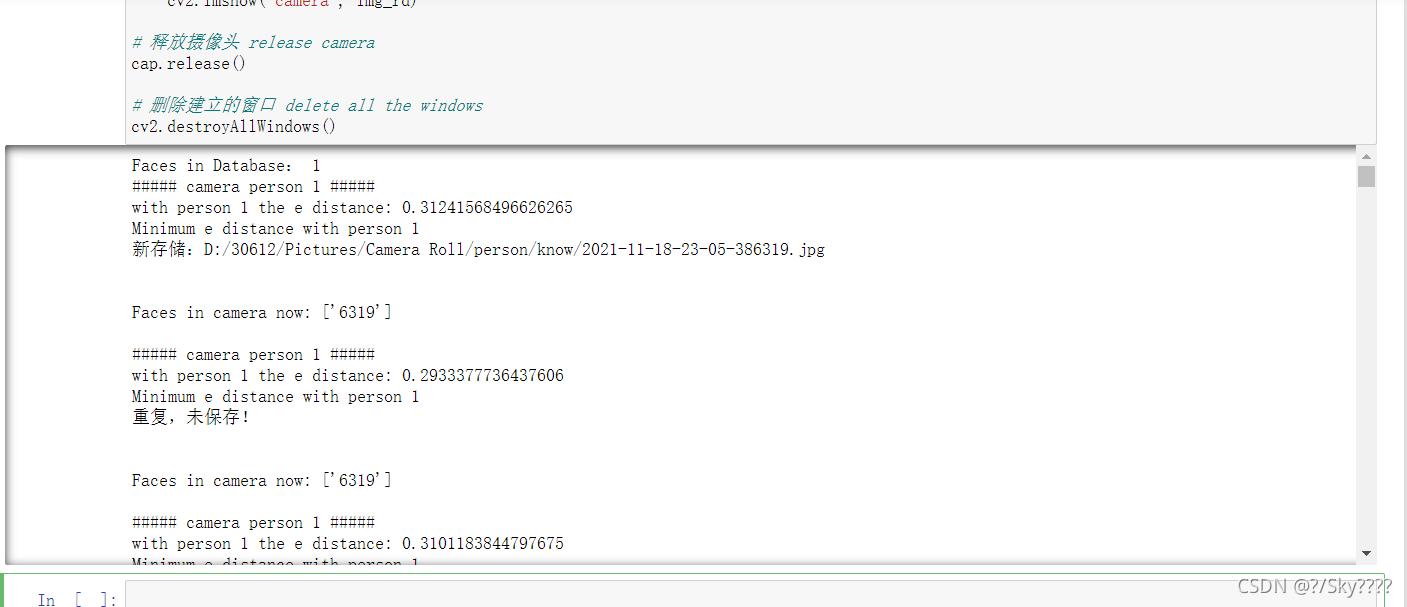 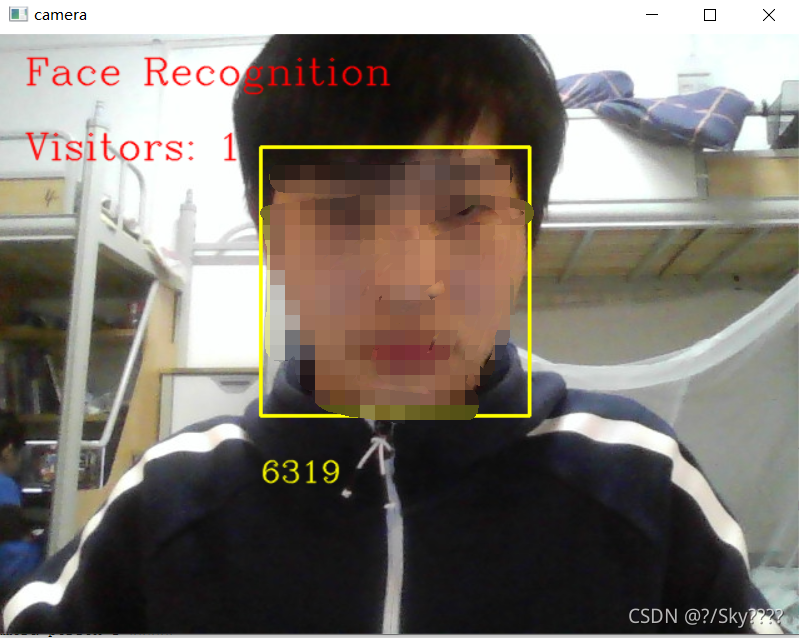
参考
https://blog.csdn.net/m0_51120713/article/details/121372289
|