Introducing_TensorFlow_Graph_Neural_Networks
Today, we are excited to release TensorFlow Graph Neural Networks (GNNs), a library designed to make it easy to work with graph structured data using TensorFlow.
Graphs are all around us, in the real world and in our engineered systems. A set of objects, places, or people and the connections between them is generally describable as a graph. More often than not, the data we see in machine learning problems is structured or relational, and thus can also be described with a graph. And while fundamental research on GNNs is perhaps decades old, recent advances in the capabilities of modern GNNs have led to advances in domains as varied as traffic prediction, rumor and fake news detection, modeling disease spread, physics simulations, and understanding why molecules smell.
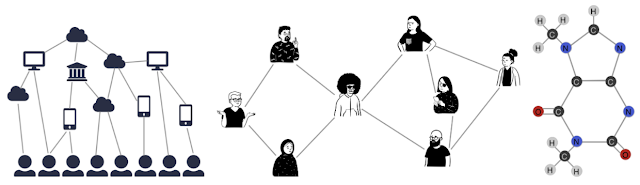 Graphs can model the relationships between many different types of data, including web pages (left), social connections (center), or molecules (right).
A graph represents the relations (edges) between a collection of entities (nodes or vertices). We can characterize each node, edge, or the entire graph, and thereby store information in each of these pieces of the graph. Additionally, we can ascribe directionality to edges to describe information or traffic flow, for example.
GNNs can be used to answer questions about multiple characteristics of these graphs. By working at the graph level, we try to predict characteristics of the entire graph. We can identify the presence of certain “shapes,” like circles in a graph that might represent sub-molecules or perhaps close social relationships. GNNs can be used on node-level tasks, to classify the nodes of a graph, and predict partitions and affinity in a graph similar to image classification or segmentation. Finally, we can use GNNs at the edge level to discover connections between entities, perhaps using GNNs to “prune” edges to identify the state of objects in a scene.
TF-GNN provides building blocks for implementing GNN models in TensorFlow. Beyond the modeling APIs, our library also provides extensive tooling around the difficult task of working with graph data: a Tensor-based graph data structure, a data handling pipeline, and some example models for users to quickly onboard.
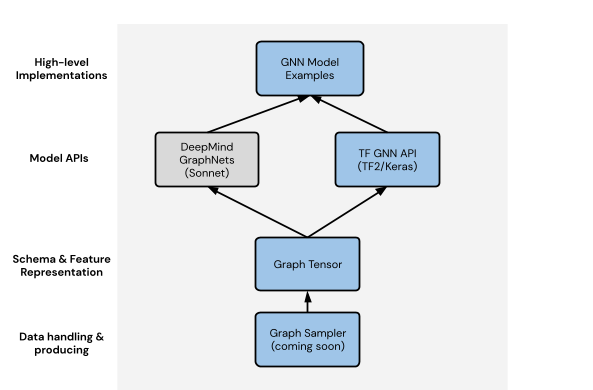 The various components of TF-GNN that make up the workflow
The initial release of the TF-GNN library contains a number of utilities and features for use by beginners and experienced users alike, including:
- A high-level Keras-style API to create GNN models that can easily be composed with other types of models. GNNs are often used in combination with ranking, deep-retrieval (dual-encoders) or mixed with other types of models (image, text, etc.)
- A well-defined schema to declare the topology of a graph, and tools to validate it. This schema describes the shape of its training data and serves to guide other tools.
- A GraphTensor composite tensor type which holds graph data, can be batched, and has graph manipulation routines available.
- An encoding of graph-shaped training data on disk, as well as a library used to parse this data into a data structure from which your model can extract the various features.
In the example below, we build a model using the TF-GNN Keras API to recommend movies to a user based on what they watched and genres that they liked
We use the ConvGNNBuilder method to specify the type of edge and node configuration, namely to use WeightedSumConvolution (defined below) for edges. And for each pass through the GNN, we will update the node values through a Dense interconnected layer:
import tensorflow as tf
import tensorflow_gnn as tfgnn
# Model hyper-parameters:
h_dims = {'user': 256, 'movie': 64, 'genre': 128}
# Model builder initialization:
gnn = tfgnn.keras.ConvGNNBuilder(
lambda edge_set_name: WeightedSumConvolution(),
lambda node_set_name: tfgnn.keras.layers.NextStateFromConcat(
tf.keras.layers.Dense(h_dims[node_set_name]))
)
# Two rounds of message passing to target node sets:
model = tf.keras.models.Sequential([
gnn.Convolve({'genre'}), # sends messages from movie to genre
gnn.Convolve({'user'}), # sends messages from movie and genre to users
tfgnn.keras.layers.Readout(node_set_name="user"),
tf.keras.layers.Dense(1)
])
The code above works great, but sometimes we may want to use a more powerful custom model architecture for our GNNs. For example, in our previous use case, we might want to specify that certain movies or genres hold more weight when we give our recommendation. In the following snippet, we define a more advanced GNN with custom graph convolutions, in this case with weighted edges. We define the WeightedSumConvolution class to pool edge values as a sum of weights across all edges:
class WeightedSumConvolution(tf.keras.layers.Layer):
"""Weighted sum of source nodes states."""
def call(self, graph: tfgnn.GraphTensor,
edge_set_name: tfgnn.EdgeSetName) -> tfgnn.Field:
messages = tfgnn.broadcast_node_to_edges(
graph,
edge_set_name,
tfgnn.SOURCE,
feature_name=tfgnn.DEFAULT_STATE_NAME)
weights = graph.edge_sets[edge_set_name]['weight']
weighted_messages = tf.expand_dims(weights, -1) * messages
pooled_messages = tfgnn.pool_edges_to_node(
graph,
edge_set_name,
tfgnn.TARGET,
reduce_type='sum',
feature_value=weighted_messages)
return pooled_messages
Note that even though the convolution was written with only the source and target nodes in mind, TF-GNN makes sure it’s applicable and works on heterogeneous graphs (with various types of nodes and edges) seamlessly.
|