阅读总结
Google的TPU是AI_ASIC芯片的鼻祖,从论文的作者数量之庞大,及论文少有的出现了致谢,就可以看出一定是历经了一番磨砺才创造出来。该论文发表在 2017 年,让我们回到那个年代,一同看看是什么样的背景诞生了如此伟大的艺术品~
In-Datacenter Performance Analysis of a Tensor Processing Unit
To appear at the 44th International Symposium on Computer Architecture (ISCA), Toronto, Canada, June 26, 2017.
0. Abstract
摘要主要介绍我们用了什么,和什么相比较,有什么样的结果.
1. Introduction to Neural Networks
A step called ?quantization transforms floating-point numbers into narrow integers—often just 8 bits—which are usually good enough for inference. Eight-bit integer multiplies can be 6X less energy and 6X less area than IEEE 754 16-bit floating-point multiplies, and the 1advantage for integer addition is 13X in energy and 38X in area [Dal16] 介绍INT8,为什么需要INT8,以及量化(quantization)的概念
 NN – Neural Networks ,当前主要有三类,多层感知器,CNN,RNN. 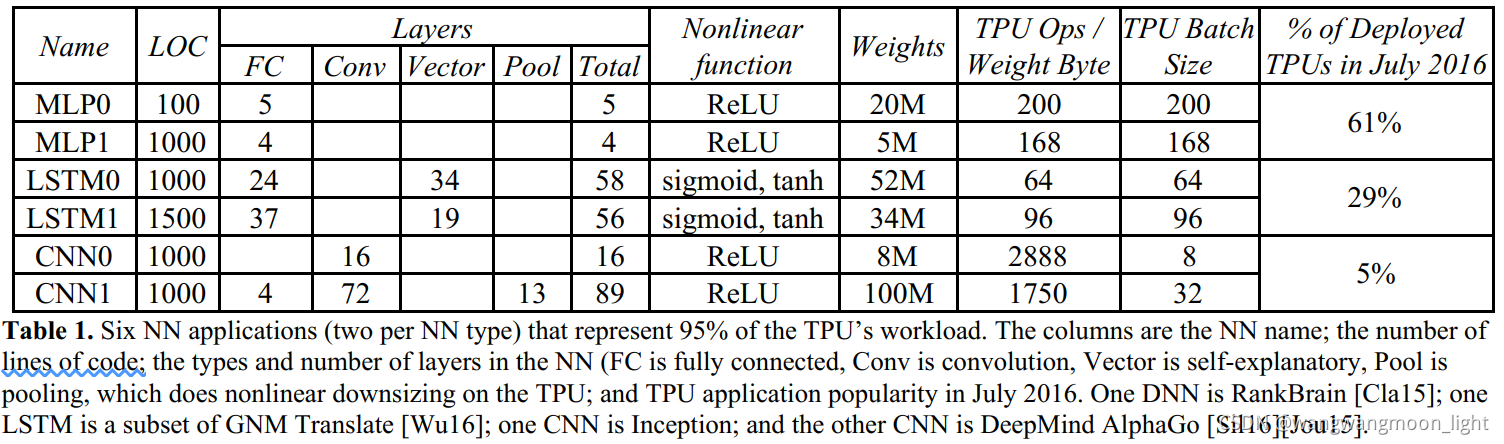
2. TPU Origin, Architecture, and Implementation
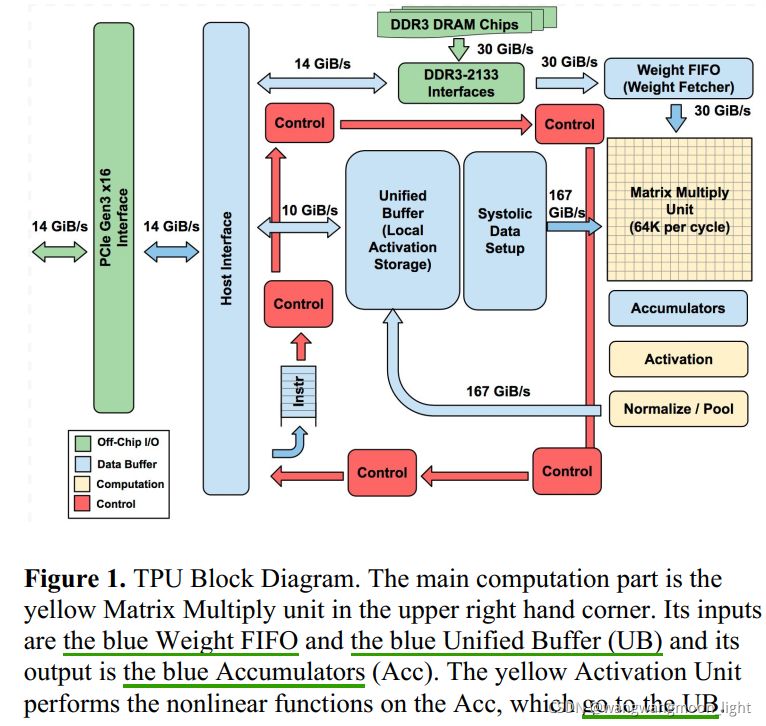 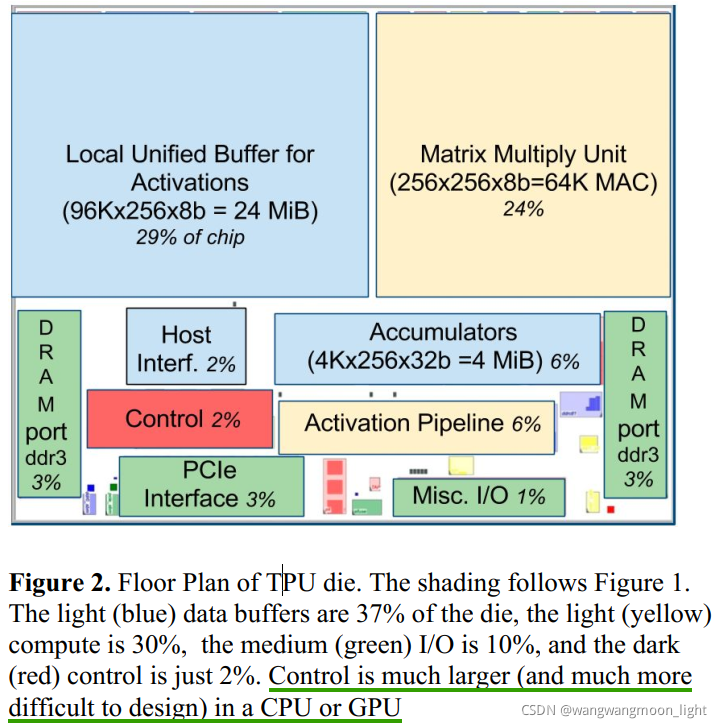 脉动矩阵 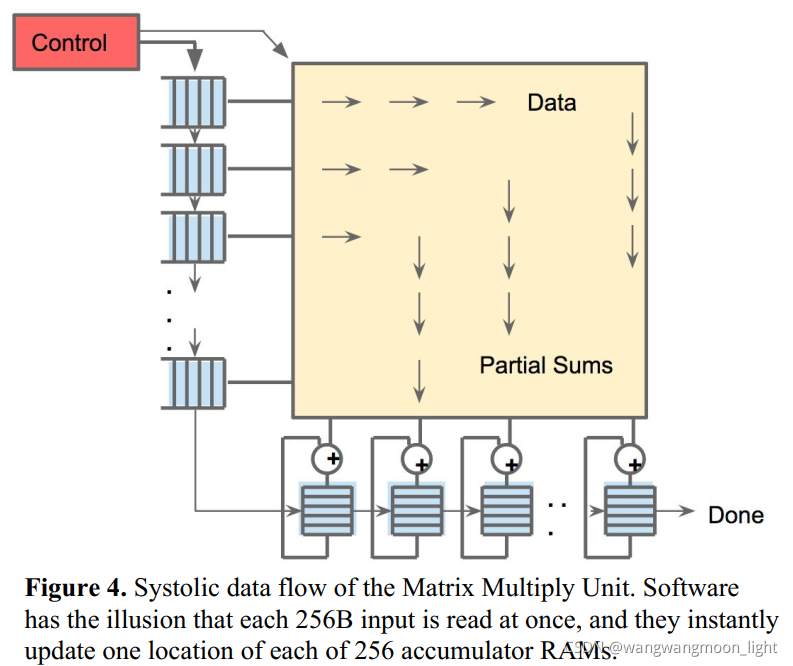 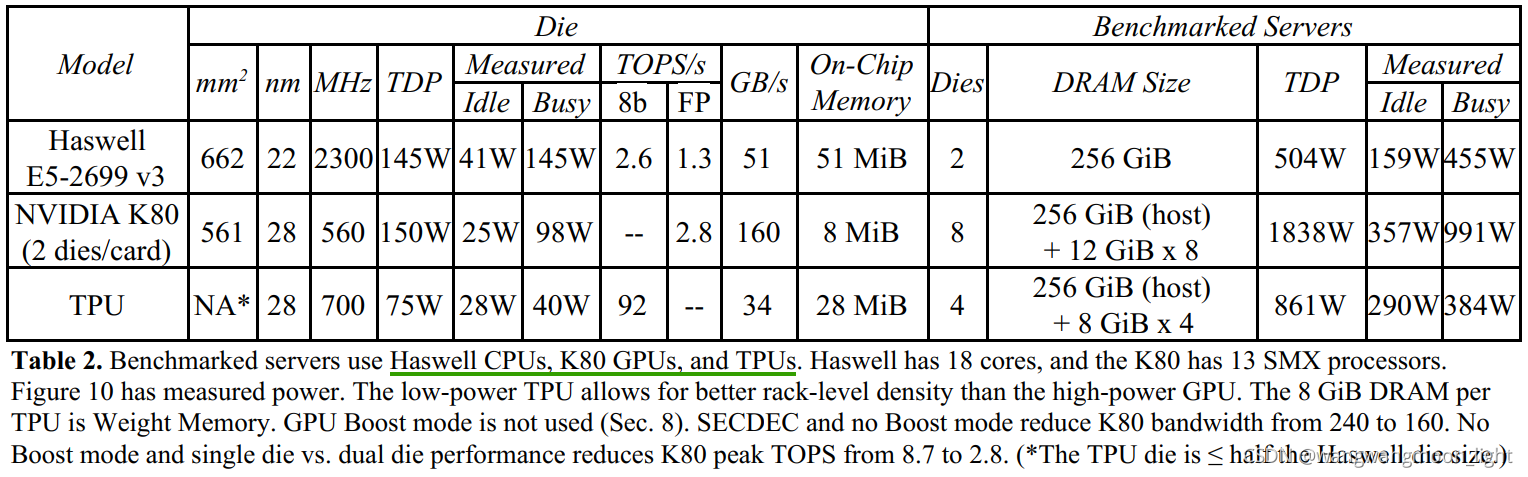
4. Performance: Rooflines, Response-Time, and Throughput
待续
|