论文地址
前言
Ghostnet提出自己的架构设计思路:特征图的冗余是深度神经网络成功的一个重要特征。Ghostnet倾向于以一种代价低廉的线性操作来获取它们,而不是避免冗余的特性映射!
一、论文解读
1.Ghost卷积设计
在本文中,我们引入了一个新的Ghost模块,用更少的参数生成更多的特征。具体来说,将深度神经网络中的普通卷积层分为两部分。第一部分涉及普通的卷积,但它们的总数将被严格控制。给定第一部分的固有特征图,然后应用一系列简单的线性操作来生成更多的特征图。在不改变输出特征图大小的情况下,与普通卷积神经网络相比,Ghost模块所需的参数总数和计算复杂度都有所降低。实现过程如下: 首先通过主卷积生成基本特征图集合:X为输入特征图(尺寸参数为h:宽度,w:长度,c:通道数),f’为卷积层(尺寸参数为c:输入通道数,k:卷积核尺寸大小,m为输出通道数),X在与卷积层卷积后输出特征图集合Y’(尺寸参数为h’,w’,m)。此处省略了偏置参数。  然后,对输出的基本特征图集合Y’做线性变化,以生成Ghost特征图。其中 fi是基本特征图集合Y’中第i个特征图, 是通过 生成的第j个Ghost特征图所做的线性变化,此处操作即指对应基本特征图集合中每个特征图通过线性操作生成s个Ghost特征图,最后一个线性操作为恒等映射以保留固有特征。 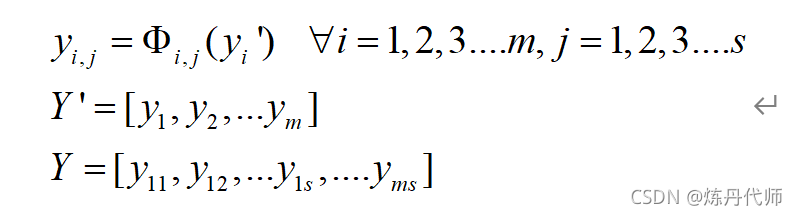 最后输出ms张特征图来作为最终输出特征图集合,相比于直接通过用ckkn(n=m*s)的卷积层做卷积,其计算量大幅减小(衰减比值大约为s)。整个特征图变化过程如下图: 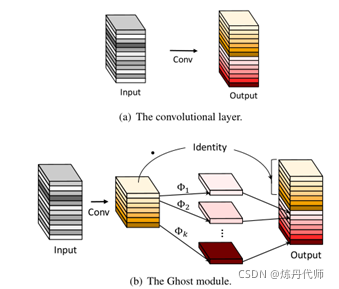
2.Gbneck残差模块设计
此处作者参照Resnet的残差模块设计出Ghost瓶颈模块,ghost瓶颈主要由两个堆叠的ghost模块组成。第一个Ghost模块作为扩展层,增加通道的数量。我们把输出通道的数量与输入通道的数量之比称为扩展比。第二个Ghost模块减少通道数量以匹配捷径路径。然后在这两个Ghost模块的输入和输出之间连接快捷方式。每一层之后都使用批处理归一化(BN)和ReLU非线性。 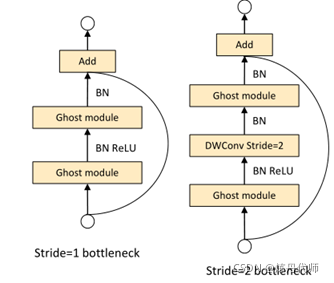
3.GhostNet网络架构
以本文之愚见,网络架构与残差模块设计基本是延续前人的思想,Ghostnet最重要的创新点在于卷积的设计(确实NB)! 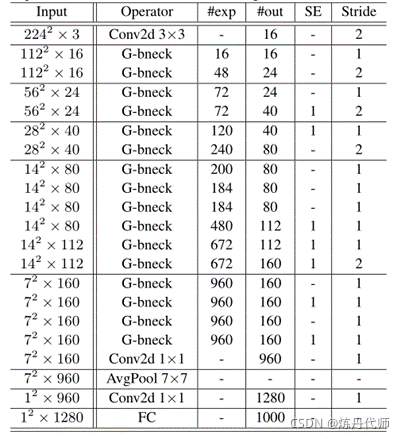
二、代码实现
源代码比较恶心,嫖几行代码根本无法实现,貌似它把所有的操作都重写了一遍,对于我们这种想快速嫖代码的人来说就很难受了,没得办法,只有参考源代码自己写一个简单的出来了。前面说了最重要的创新是卷积模块的设计,那如果能嫖卷积模块的设计,剩下来的不都是之前论文实现的工作了吗?当然自己写出的代码还是不敢直接就用,还是对比下模型参数及计算量的好。经过验证,果然嫖代码能力天赋异禀的我完美实现了该网络。 各位同志如果要嫖作者大大的代码的话,先交个一键三连的学费,谢谢各位!
1.卷积模块
代码如下:
class Ghostconv(nn.Module):
"""Ghostnet的基础卷积模块"""
def __init__(self,in_channels,out_channels):
super(Ghostconv, self).__init__()
main_channels=math.ceil(0.5*out_channels)
cheap_channels=out_channels-main_channels
self.piontconv1=nn.Sequential(nn.Conv2d(in_channels,main_channels,kernel_size=1,stride=1,bias=False),
nn.BatchNorm2d(main_channels,eps=1e-5),
nn.ReLU(inplace=True))
self.linear_option=nn.Sequential(nn.Conv2d(main_channels,cheap_channels,kernel_size=3,padding=1,stride=1,groups=cheap_channels),
nn.BatchNorm2d(cheap_channels,eps=1e-5),
nn.ReLU(inplace=True))
def forward(self,x):
x=self.piontconv1(x)
y=self.linear_option(x)
return torch.cat((x,y),dim=1)
2.残差模块实现
代码如下(示例):
class GhostUnit(nn.Module):
"""实现基本残差块和降维残差块"""
def __init__(self,in_channels,out_channels,stride,use_se,ex_factor,k_size,padding):
super(GhostUnit, self).__init__()
self.stride=stride
self.use_se=use_se
self.res=nn.Sequential()
mid_channels=math.ceil(ex_factor*in_channels)
self.expconv=Ghostconv(in_channels,mid_channels)
if stride==2:
self.dwconv=nn.Sequential(nn.Conv2d(mid_channels,mid_channels,stride=stride,kernel_size=k_size,groups=mid_channels,bias=False,padding=padding),
nn.BatchNorm2d(mid_channels,eps=1e-5),
nn.ReLU(inplace=True))
if stride==2 or in_channels!=out_channels:
self.res=nn.Sequential(nn.Conv2d(in_channels,in_channels,kernel_size=3,groups=in_channels,padding=1,stride=stride,bias=False),
nn.BatchNorm2d(in_channels,eps=1e-5),
nn.ReLU(inplace=True),
nn.Conv2d(in_channels,out_channels,kernel_size=1,stride=1,bias=False),
nn.BatchNorm2d(out_channels)
)
self.pwconv=Ghostconv(mid_channels,out_channels)
if use_se==True:
self.se=SElayer(4,mid_channels)
def forward(self,x):
residual=self.res(x)
x=self.expconv(x)
if self.stride==2:
x=self.dwconv(x)
if self.use_se:
x=self.se(x)
x=self.pwconv(x)
return x+residual
3.网络实现
代码如下:
class Ghostnet(nn.Module):
def __init__(self,num_calss,width_ratio=1):
super(Ghostnet, self).__init__()
self.inital=nn.Sequential(nn.Conv2d(in_channels=3,out_channels=int(16*width_ratio),kernel_size=3,padding=1,stride=2,bias=False),
nn.BatchNorm2d(int(16*width_ratio)),
nn.ReLU(inplace=True))
self.block1=nn.Sequential(GhostUnit(in_channels=int(16*width_ratio),out_channels=int(16*width_ratio),stride=1,use_se=False,ex_factor=1,k_size=3,padding=1),
GhostUnit(in_channels=int(16*width_ratio),out_channels=int(24*width_ratio),stride=2,use_se=False,ex_factor=3,k_size=3,padding=1))
self.block2 = nn.Sequential(
GhostUnit(in_channels=int(24*width_ratio), out_channels=int(24*width_ratio), stride=1, use_se=False, ex_factor=3, k_size=3, padding=1),
GhostUnit(in_channels=int(24*width_ratio), out_channels=int(40*width_ratio), stride=2, use_se=True, ex_factor=3, k_size=5, padding=2))
self.block3 = nn.Sequential(
GhostUnit(in_channels=int(40*width_ratio), out_channels=int(40*width_ratio), stride=1, use_se=True, ex_factor=3, k_size=5, padding=2),
GhostUnit(in_channels=int(40*width_ratio), out_channels=int(80*width_ratio), stride=2, use_se=False, ex_factor=6, k_size=3, padding=1))
self.block4 = nn.Sequential(
GhostUnit(in_channels=int(80*width_ratio), out_channels=int(80*width_ratio), stride=1, use_se=False, ex_factor=2.5, k_size=3, padding=1),
GhostUnit(in_channels=int(80*width_ratio), out_channels=int(80*width_ratio), stride=1, use_se=False, ex_factor=2.3, k_size=3, padding=1),
GhostUnit(in_channels=int(80*width_ratio), out_channels=int(80*width_ratio), stride=1, use_se=False, ex_factor=2.3, k_size=3, padding=1),
GhostUnit(in_channels=int(80*width_ratio), out_channels=int(112*width_ratio), stride=1, use_se=True, ex_factor=6, k_size=3, padding=1),
GhostUnit(in_channels=int(112*width_ratio), out_channels=int(112*width_ratio), stride=1, use_se=True, ex_factor=6, k_size=3, padding=1),
GhostUnit(in_channels=int(112*width_ratio), out_channels=int(160*width_ratio), stride=2, use_se=True, ex_factor=6, k_size=5, padding=2),
)
self.block5 = nn.Sequential(
GhostUnit(in_channels=int(160*width_ratio), out_channels=int(160*width_ratio), stride=1, use_se=False, ex_factor=6, k_size=5, padding=2),
GhostUnit(in_channels=int(160*width_ratio), out_channels=int(160*width_ratio), stride=1, use_se=True, ex_factor=6, k_size=5, padding=2),
GhostUnit(in_channels=int(160*width_ratio), out_channels=int(160*width_ratio), stride=1, use_se=False, ex_factor=6, k_size=5, padding=2),
GhostUnit(in_channels=int(160*width_ratio), out_channels=int(160*width_ratio), stride=1, use_se=True, ex_factor=6, k_size=5, padding=2),
)
self.conv_last=nn.Sequential(nn.Conv2d(in_channels=int(160*width_ratio),out_channels=960,kernel_size=1,stride=1,bias=False),
nn.BatchNorm2d(960),
nn.ReLU(inplace=True))
self.pool=nn.AdaptiveAvgPool2d(1)
self.finally_conv=nn.Conv2d(in_channels=960,out_channels=1280,kernel_size=1,stride=1,bias=True)
self.fc=nn.Linear(in_features=1280,out_features=num_calss)
def forward(self,x):
x=self.inital(x)
x=self.block1(x)
x=self.block2(x)
x = self.block3(x)
x = self.block4(x)
x = self.block5(x)
x=self.conv_last(x)
x=self.pool(x)
x=self.finally_conv(x)
x=x.view(x.size(0),-1)
x=self.fc(x)
return F.softmax(x,dim=1)
三、验证模型
代码如下:
with torch.cuda.device(0):
net = Ghostnet(1000,1)
macs, params = get_model_complexity_info(net, (3, 224, 224), as_strings=True,
print_per_layer_stat=True, verbose=True)
print('{:<30} {:<8}'.format('Computational complexity: ', macs))
print('{:<30} {:<8}'.format('Number of parameters: ', params))
原文中给出了模型的参数量和计算量,这不就可以验证一下模型有没有问题吗! 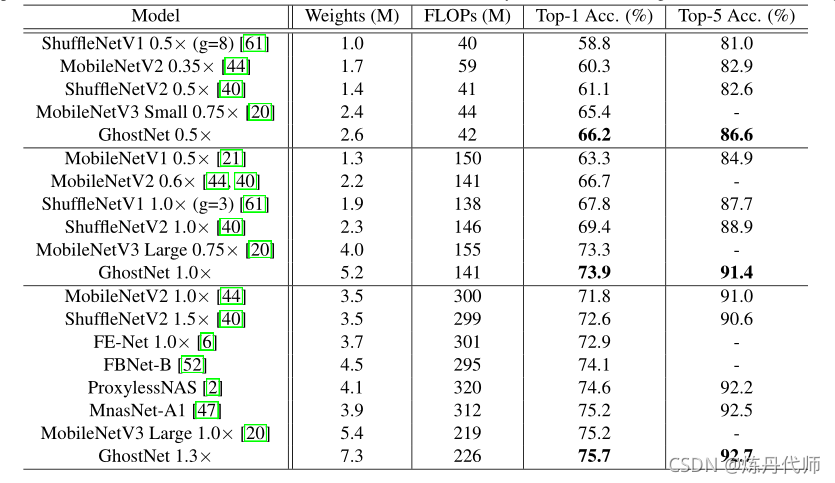 我模型跑出来的参数量(Weight)和计算量(FLOPs)如下: 1)当宽度因子取到0.5时:  这里和2.6M的Weight及42M的FLOPs相差无几! 2)当宽度因子取到1时:  这里和5.2M的Weight及142M的FLOPs差的一般! 总的来说:还行(此处省略一万只草泥马)!!!
总结
本文介绍了GhostNet的核心思想及其代码实现,以供大家交流讨论! 往期回顾: (1)CBAM论文解读+CBAM-ResNeXt的Pytorch实现 (2)SENet论文解读及代码实例 (3)ShuffleNet-V1论文理解及代码复现 (4) ShuffleNet-V2论文理解及代码复现 下期预告: EfficientNet论文阅读及代码实现
|