训练误差:模型在训练数据上的误差
泛化误差:模型在新数据上的误差(*)
例:在过去的考试中表现很好(训练误差)不代表在未来考试一定会好(泛化误差)
验证数据集:一个用来评估模型好坏的数据集(和训练数据集不一样)
测试数据集:只用一次的数据集
K-折交叉验证:在没有足够多的数据时使用。(常用:K=5,10)
算法:将训练数据分割成K块;For i=1,...,K,使用第i块作为验证数据集,其余的作为训练数据集;报告K个验证集误差的平均
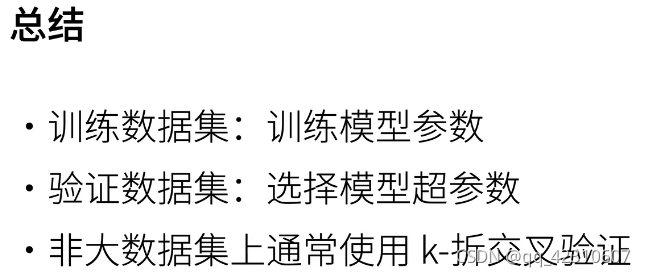
?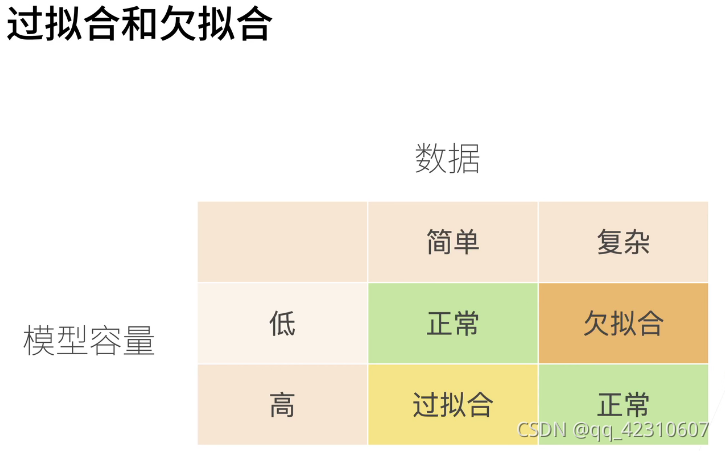
模型容量:模型复杂度
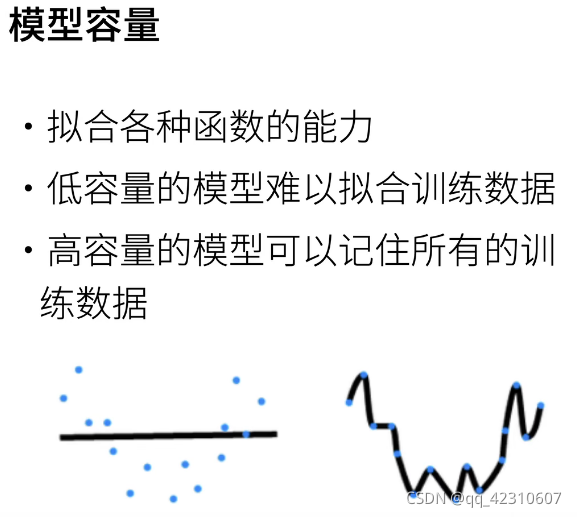 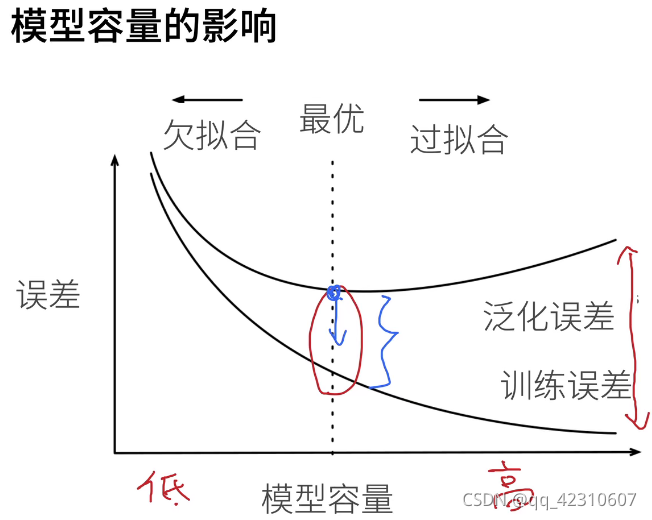
? d+1:d个w和1个b
?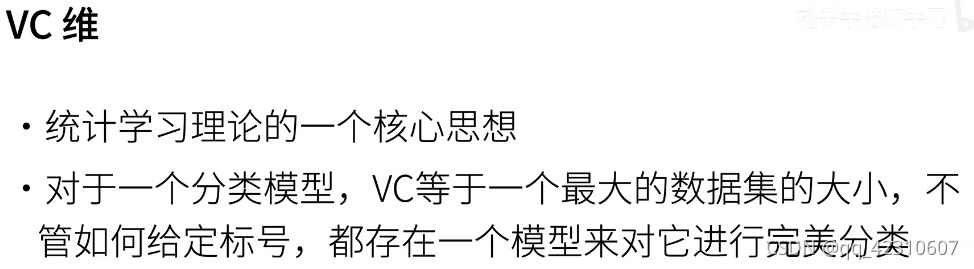
?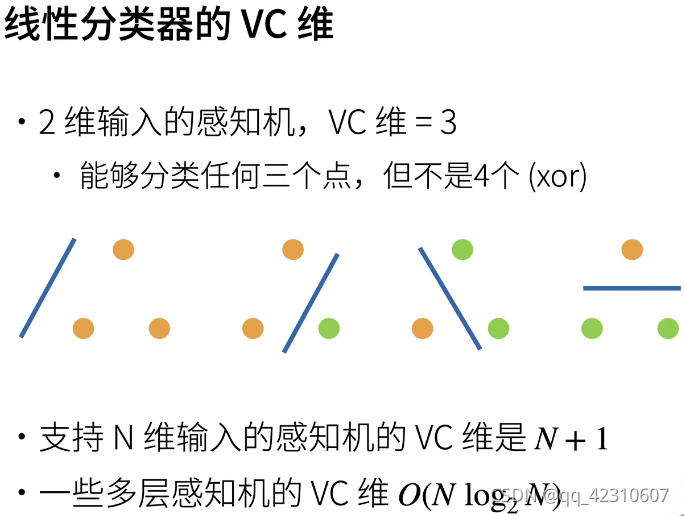
?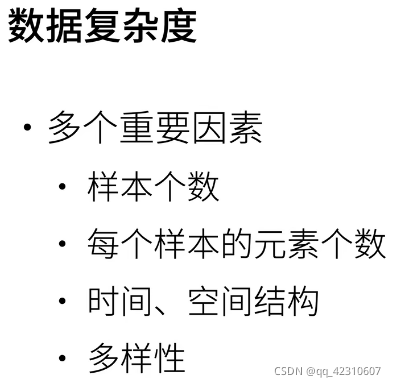
?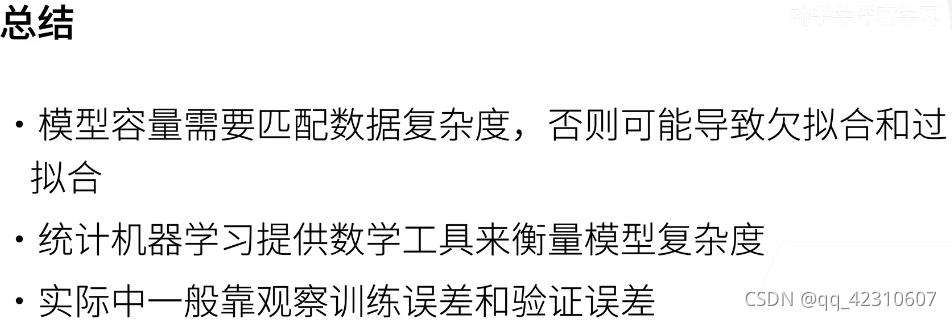
import math
import torch
import numpy as np
from torch import nn
from d2l import torch as d2l
# 使用三阶多项式来生成训练和测试数据的标签
max_degree = 20 # 多项式的最大阶数
n_train, n_test = 100, 100 # 训练和测试数据集大小
true_w = np.zeros(max_degree) # 分配大量的空间,长为20
true_w[0:4] = np.array([5, 1.2, -3.4, 5.6]) # 后面全为0
features = np.random.normal(size=(n_train + n_test, 1))
np.random.shuffle(features)
poly_features = np.power(features, np.arange(max_degree).reshape(1, -1))
for i in range(max_degree):
poly_features[:, i] /= math.gamma(i + 1) # `gamma(n)` = (n-1)!
# `labels`的维度: (`n_train` + `n_test`,)
labels = np.dot(poly_features, true_w)
labels += np.random.normal(scale=0.1, size=labels.shape)
# 查看前两个样本
# NumPy ndarray转换为tensor
true_w, features, poly_features, labels = [torch.tensor(x, dtype=d2l.float32) for x in [true_w, features, poly_features, labels]]
print(features[:2], poly_features[:2, :], labels[:2])
# 实现一个函数来评估模型在给定数据集上的损失
def evaluate_loss(net, data_iter, loss):
"""评估给定数据集上模型的损失"""
metric = d2l.Accumulator(2) # 损失的总和, 样本数量
for X, y in data_iter:
out = net(X)
y = y.reshape(out.shape)
l = loss(out, y)
metric.add(l.sum(), l.numel())
return metric[0] / metric[1]
# 定义训练函数
def train(train_features, test_features, train_labels, test_labels, num_epochs=400):
loss = nn.MSELoss()
input_shape = train_features.shape[-1]
# 不设置偏置,因为已经在多项式特征中实现了它
net = nn.Sequential(nn.Linear(input_shape, 1, bias=False))
batch_size = min(10, train_labels.shape[0])
train_iter = d2l.load_array((train_features, train_labels.reshape(-1,1)), batch_size)
test_iter = d2l.load_array((test_features, test_labels.reshape(-1,1)), batch_size, is_train=False)
trainer = torch.optim.SGD(net.parameters(), lr=0.01)
animator = d2l.Animator(xlabel='epoch', ylabel='loss', yscale='log',
xlim=[1, num_epochs], ylim=[1e-3, 1e2],
legend=['train', 'test'])
for epoch in range(num_epochs):
d2l.train_epoch_ch3(net, train_iter, loss, trainer)
if epoch == 0 or (epoch + 1) % 20 == 0:
animator.add(epoch + 1, (evaluate_loss(net, train_iter, loss),
evaluate_loss(net, test_iter, loss)))
print('weight:', net[0].weight.data.numpy())
# 三阶多项式函数拟合(正态)
# 从多项式特征中选择前4个维度,即 1, x, x^2/2!, x^3/3!
print(train(poly_features[:n_train, :4], poly_features[n_train:, :4],
labels[:n_train], labels[n_train:]))
# 线性函数拟合(欠拟合)
# 从多项式特征中选择前2个维度,即1,x
print(train(poly_features[:n_train, :2], poly_features[n_train:, :2],labels[:n_train],labels[n_train:]))
# 高阶多项式函数拟合(过拟合)
print(train(poly_features[:n_train, :], poly_features[n_train:, :],labels[:n_train],labels[n_train:]))
?
?
?
?
?
|