Pandas是入门Python做数据分析必须要掌握的一个库,是一个开放源码、BSD 许可的库,提供高性能、易于使用的数据结构和数据分析的工具。主要数据结构是 Series (一维数据)与 DataFrame(二维数据),这两种数据结构足以处理金融、统计、社会科学、工程等领域里的大多数典型用例。今天就来一起学习。 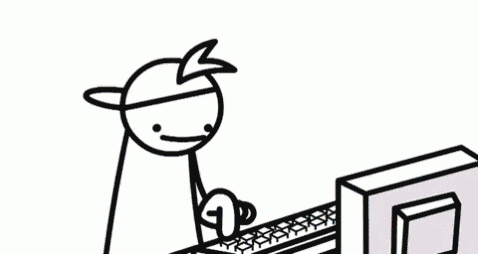
练习6-统计
探索风速数据
import pandas as pd
import datetime
import pandas as pd
path6 = "../input/pandas_exercise/pandas_exercise/exercise_data/wind.data"
import datetime
data = pd.read_table(path6, sep = "\s+", parse_dates = [[0,1,2]])
data.head()
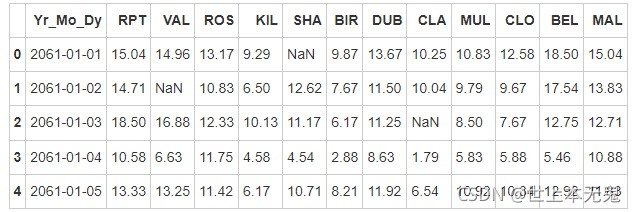
- 第四步:2061年?我们真的有这一年的数据?创建一个函数并用它去修复这个bug
def fix_century(x):
year = x.year - 100 if x.year > 1989 else x.year
return datetime.date(year, x.month, x.day)
data['Yr_Mo_Dy'] = data['Yr_Mo_Dy'].apply(fix_century)
data.head()
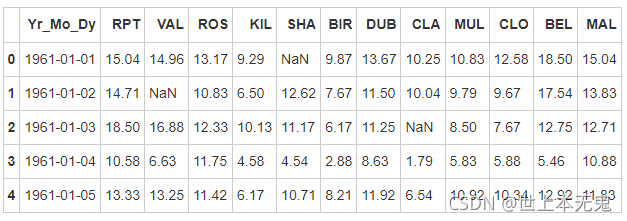
- 第五步:将日期设为索引,注意数据类型,应该是datetime64[ns]
data["Yr_Mo_Dy"] = pd.to_datetime(data["Yr_Mo_Dy"])
data = data.set_index('Yr_Mo_Dy')
data.head()
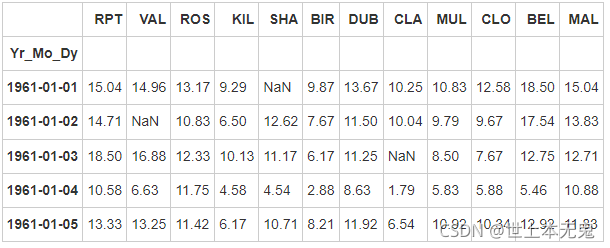
- 第六步:对应每一个location,一共有多少数据值缺失
data.isnull().sum()
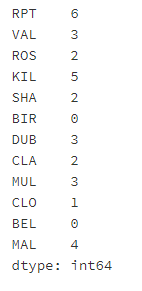
- 第七步:对应每一个location,一共有多少完整的数据值
data.shape[0] - data.isnull().sum()
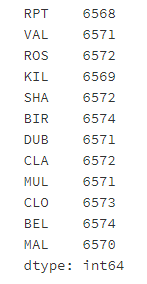
data.mean().mean()
10.227982360836924
- 第九步:创建一个名为loc_stats的数据框去计算并存储每个location的风速最小值,最大值,平均值和标准差
loc_stats = pd.DataFrame()
loc_stats['min'] = data.min()
loc_stats['max'] = data.max()
loc_stats['mean'] = data.mean()
loc_stats['std'] = data.std()
loc_stats
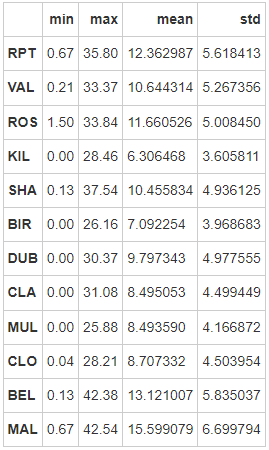
- 第十步:创建一个名为day_stats的数据框去计算并存储所有location的风速最小值,最大值,平均值和标准差
day_stats = pd.DataFrame()
day_stats['min'] = data.min(axis = 1)
day_stats['max'] = data.max(axis = 1)
day_stats['mean'] = data.mean(axis = 1)
day_stats['std'] = data.std(axis = 1)
day_stats.head()
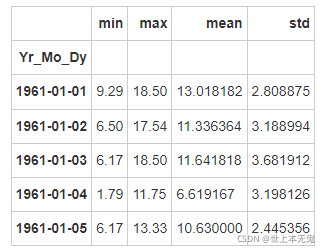
- 第十一步:对于每一个location,计算一月份的平均风速
注意:1961年的1月和1962年的1月应该区别对待
data['date'] = data.index
data['month'] = data['date'].apply(lambda date: date.month)
data['year'] = data['date'].apply(lambda date: date.year)
data['day'] = data['date'].apply(lambda date: date.day)
january_winds = data.query('month == 1')
january_winds.loc[:,'RPT':"MAL"].mean()
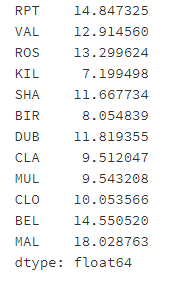
data.query('month == 1 and day == 1')
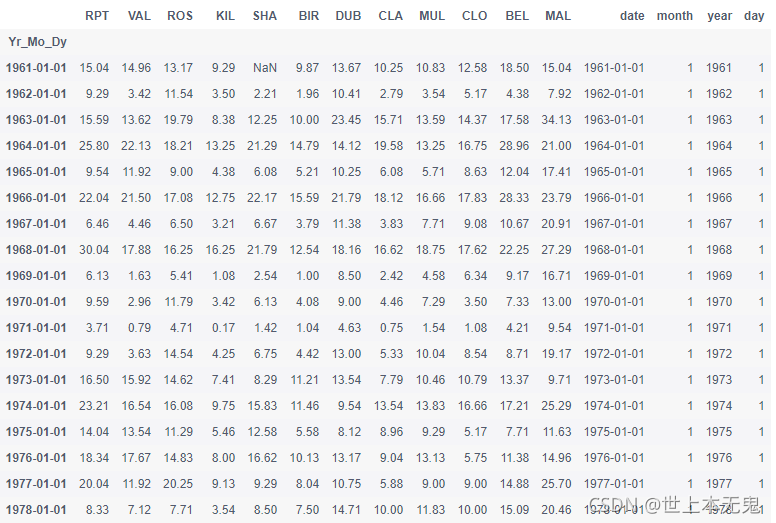
data.query('day == 1')
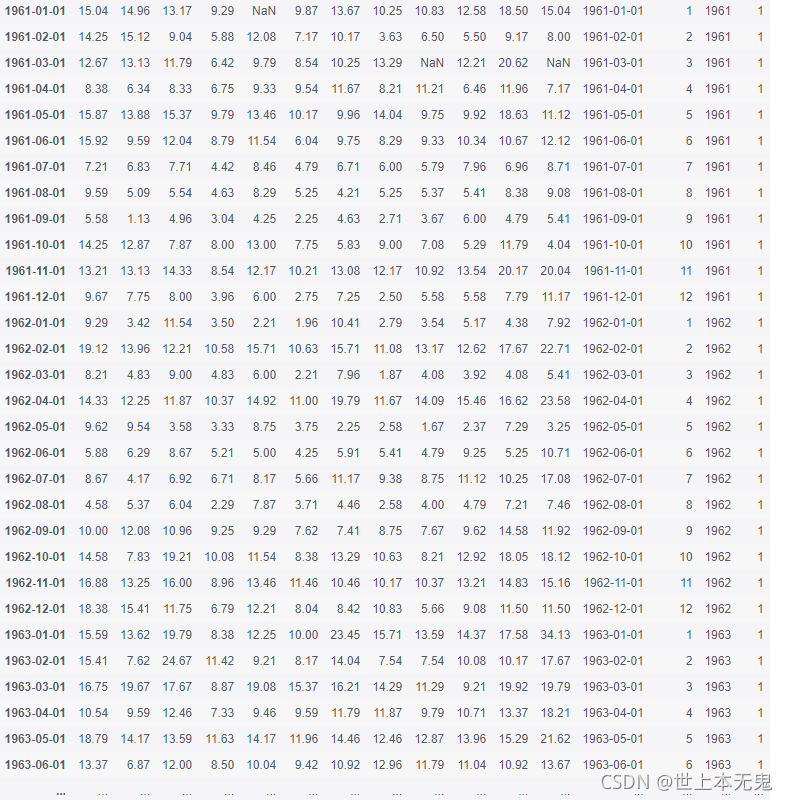 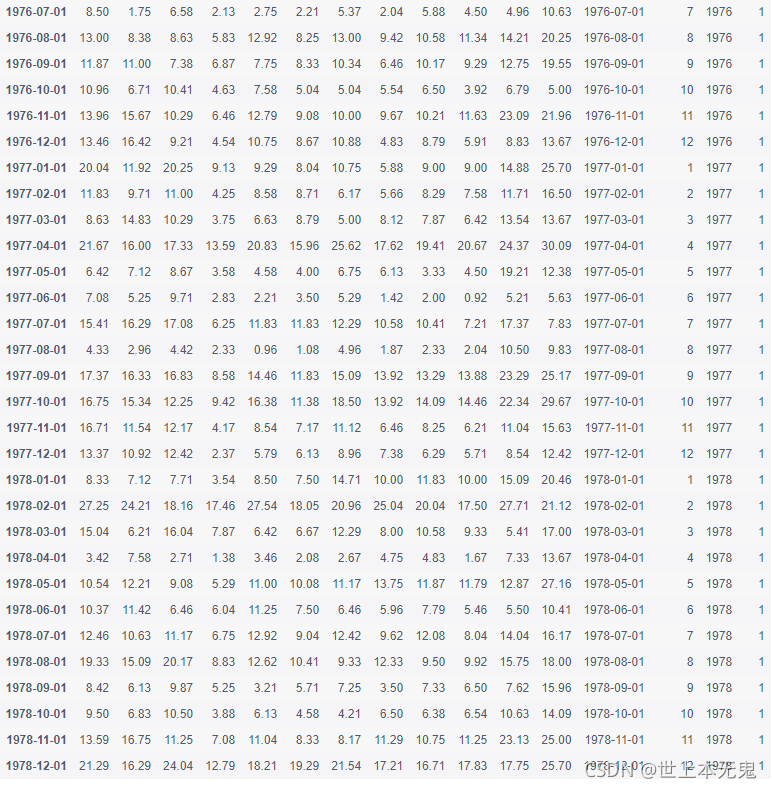
练习7-可视化
探索泰坦尼克灾难数据
import pandas as pd
import matplotlib.pyplot as plt
import seaborn as sns
import numpy as np
%matplotlib inline
path7 = '../input/pandas_exercise/pandas_exercise/exercise_data/train.csv'
titanic = pd.read_csv(path7)
titanic.head()

titanic.set_index('PassengerId').head()
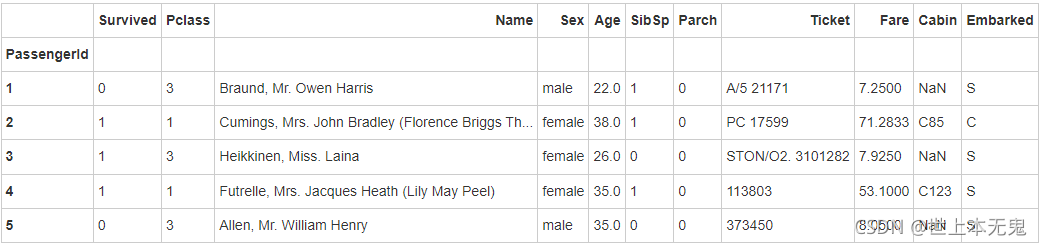
males = (titanic['Sex'] == 'male').sum()
females = (titanic['Sex'] == 'female').sum()
proportions = [males, females]
plt.pie(
proportions,
labels = ['Males', 'Females'],
shadow = False,
colors = ['blue','red'],
explode = (0.15 , 0),
startangle = 90,
autopct = '%1.1f%%'
)
plt.axis('equal')
plt.title("Sex Proportion")
plt.tight_layout()
plt.show()
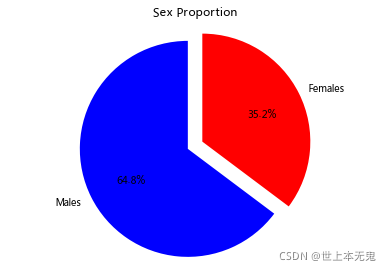
- 第六步:绘制一个展示船票Fare, 与乘客年龄和性别的散点图
lm = sns.lmplot(x = 'Age', y = 'Fare', data = titanic, hue = 'Sex', fit_reg=False)
lm.set(title = 'Fare x Age')
axes = lm.axes
axes[0,0].set_ylim(-5,)
axes[0,0].set_xlim(-5,85)
(-5, 85)
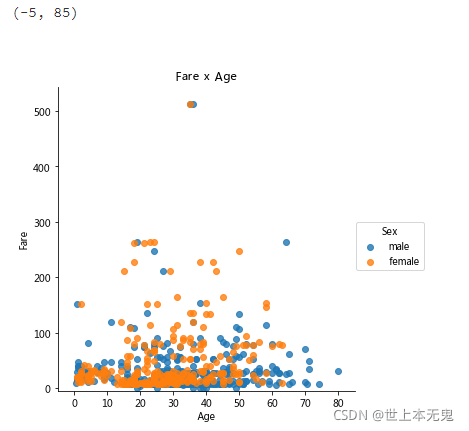
titanic.Survived.sum()
342
df = titanic.Fare.sort_values(ascending = False)
df
binsVal = np.arange(0,600,10)
binsVal
plt.hist(df, bins = binsVal)
plt.xlabel('Fare')
plt.ylabel('Frequency')
plt.title('Fare Payed Histrogram')
plt.show()
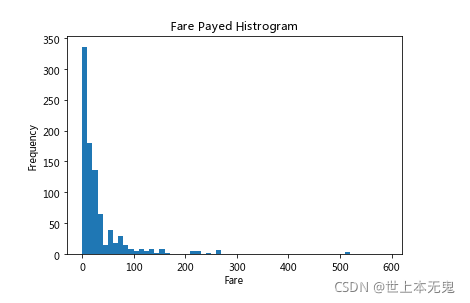
练习8-创建数据框
探索Pokemon数据
import pandas as pd
raw_data = {"name": ['Bulbasaur', 'Charmander','Squirtle','Caterpie'],
"evolution": ['Ivysaur','Charmeleon','Wartortle','Metapod'],
"type": ['grass', 'fire', 'water', 'bug'],
"hp": [45, 39, 44, 45],
"pokedex": ['yes', 'no','yes','no']
}
- 第三步:将数据字典存为一个名叫pokemon的数据框中
pokemon = pd.DataFrame(raw_data)
pokemon.head()
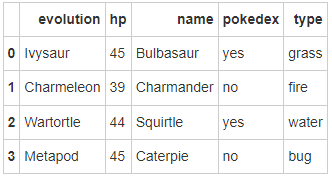
- 第四步:数据框的列排序是字母顺序,请重新修改为name, type, hp, evolution, pokedex这个顺序
pokemon = pokemon[['name', 'type', 'hp', 'evolution','pokedex']]
pokemon
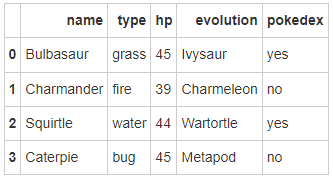
pokemon['place'] = ['park','street','lake','forest']
pokemon
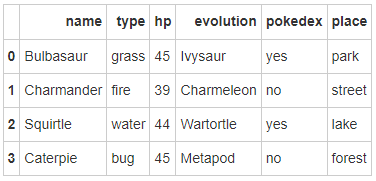
pokemon.dtypes
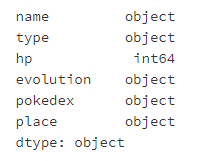
练习9-时间序列
探索Apple公司股价数据
import pandas as pd
import numpy as np
import matplotlib.pyplot as plt
%matplotlib inline
path9 = '../input/pandas_exercise/pandas_exercise/exercise_data/Apple_stock.csv'
apple = pd.read_csv(path9)
apple.head()
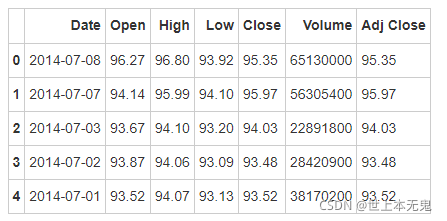
apple.dtypes
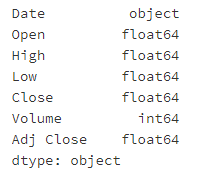
- 第五步:将Date这个列转换为datetime类型
apple.Date = pd.to_datetime(apple.Date)
apple['Date'].head()
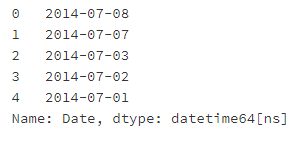
apple = apple.set_index('Date')
apple.head()
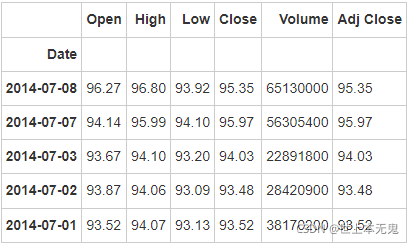
apple.index.is_unique
True
apple.sort_index(ascending = True).head()
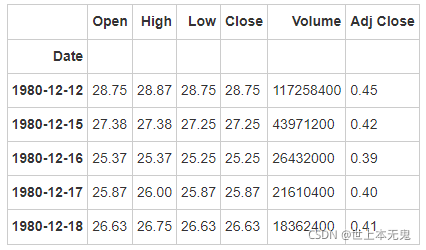
- 第九步:找到每个月的最后一个交易日(business day)
apple_month = apple.resample('BM')
apple_month.head()

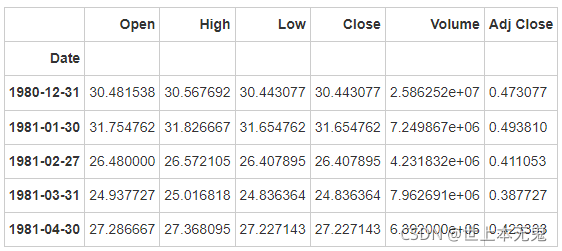
(apple.index.max() - apple.index.min()).days
12261
apple_months = apple.resample('BM').mean()
len(apple_months.index)
404
appl_open = apple['Adj Close'].plot(title = "Apple Stock")
fig = appl_open.get_figure()
fig.set_size_inches(13.5, 9)
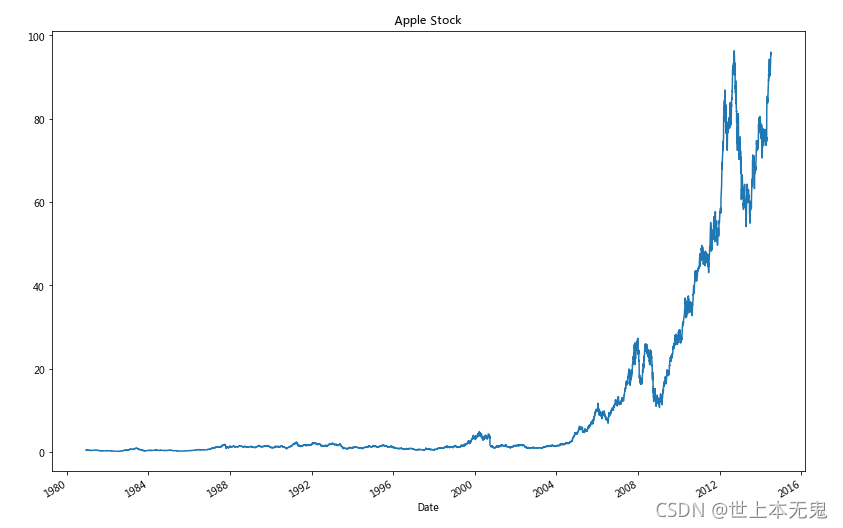
练习10-删除数据
探索Iris纸鸢花数据
import pandas as pd
path10 ='../input/pandas_exercise/pandas_exercise/exercise_data/iris.csv'
iris = pd.read_csv(path10)
iris.head()
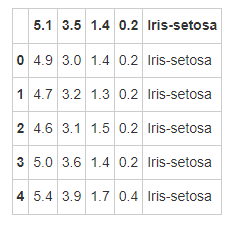
iris = pd.read_csv(path10,names = ['sepal_length','sepal_width', 'petal_length', 'petal_width', 'class'])
iris.head()
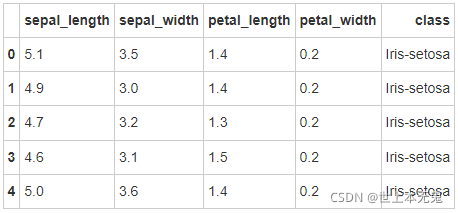
pd.isnull(iris).sum()
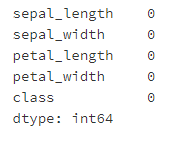
- 第六步:将列petal_length的第10到19行设置为缺失值
iris.iloc[10:20,2:3] = np.nan
iris.head(20)
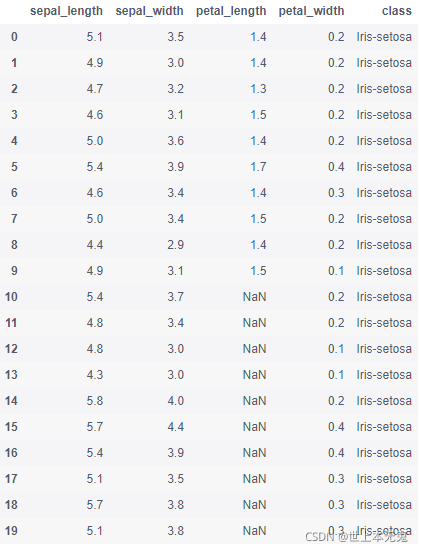
iris.petal_length.fillna(1, inplace = True)
iris
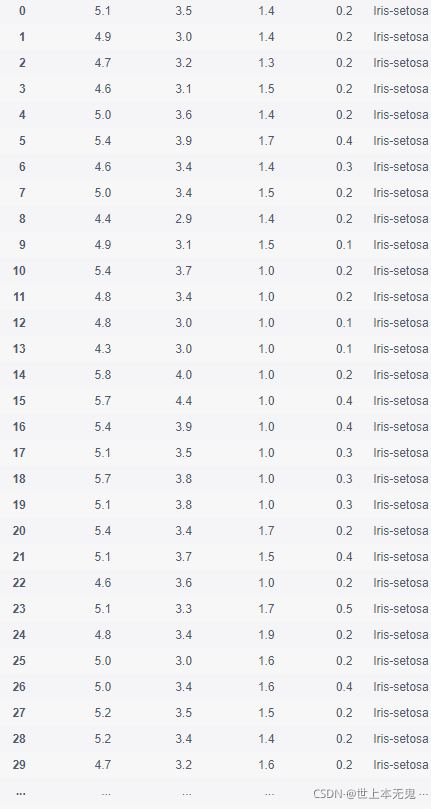 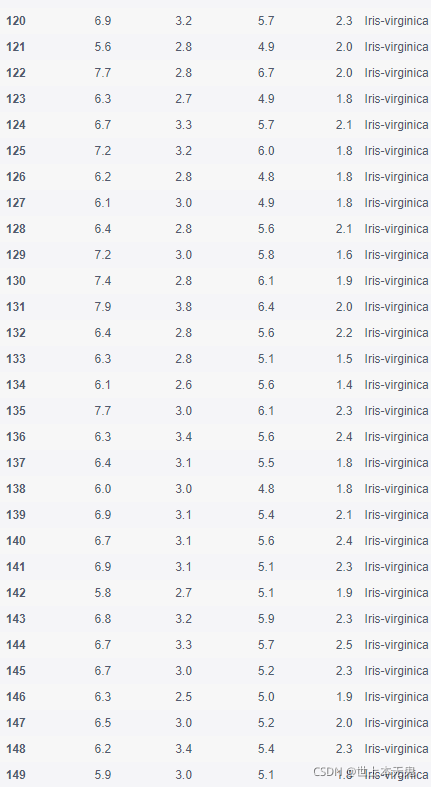
del iris['class']
iris.head()
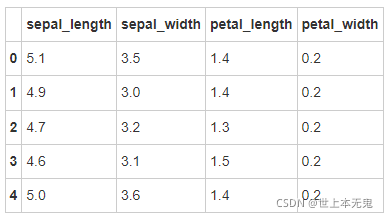
iris.iloc[0:3 ,:] = np.nan
iris.head()
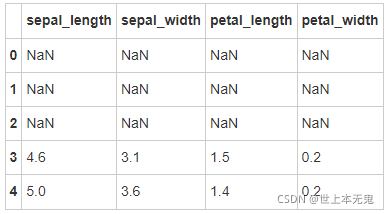
iris = iris.dropna(how='any')
iris.head()
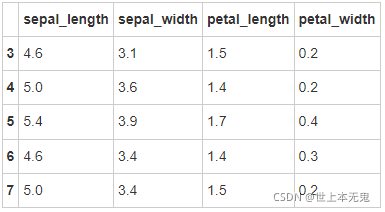
iris = iris.reset_index(drop = True)
iris.head()
你们的支持是我持续更新下去的动力,(点赞,关注,评论) 这篇文还未结束,想了解后续的可以关注我,持续更新。 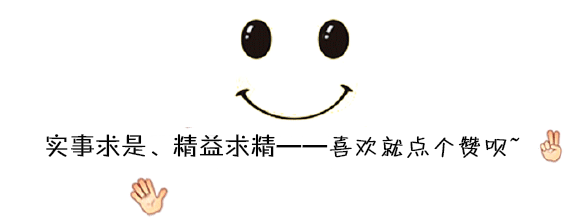
点击领取🎁🎁 Q群号: 943192807(纯技术交流和资源共享)以自助拿走。
①行业咨询、专业解答 ②Python开发环境安装教程 ③400集自学视频 ④软件开发常用词汇 ⑤最新学习路线图 ⑥3000多本Python电子书
|