https://docs.nvidia.com/deeplearning/tensorrt/api/index.html includes implementations for the most common deep learning layers.
https://docs.nvidia.com/deeplearning/tensorrt/api/c_api/classnvinfer1_1_1_i_plugin.html to provide implementations for infrequently used or more innovative layers that are not supported natively by TensorRT.
Alternatives to using TensorRT include: ? Using the training framework itself to perform inference. ? Writing a custom application that is designed specifically to execute the network using low-level libraries and math operations.
Generally, the workflow for developing and deploying a deep learning model goes through three phases. ? Phase 1 is training ? Phase 2 is developing a deployment solution, and ? Phase 3 is the deployment of that solution TensorRT is generally not used during any part of the training phase. 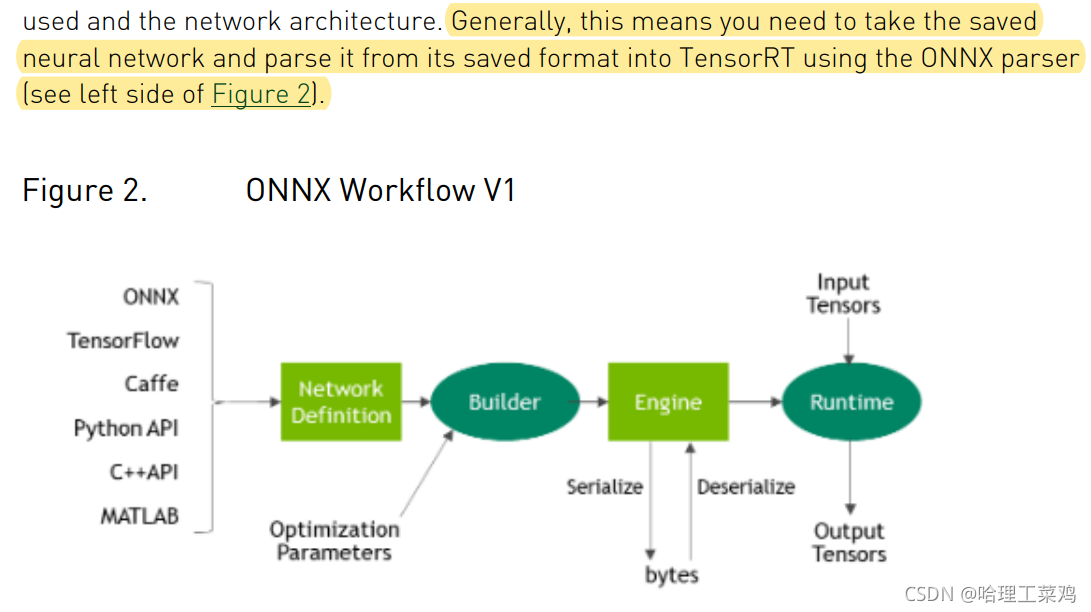 After the network is parsed, consider your optimization options – batch size, workspace size, mixed precision, and bounds on dynamic shapes. These options are chosen and specified as part of the TensorRT build step, where you build an optimized inference engine based on your network.
To initialize the inference engine, the application first deserializes the model from the plan file into an inference engine. TensorRT is usually used asynchronously; therefore, when the input data arrives, the program calls an enqueue function with the input buffer and the buffer in which TensorRT should put the result.
To optimize your model for inference, TensorRT takes your network definition, performs network-specific and platform-specific optimizations, and generates the inference engine. This process is referred to as the build phase. The build phase can take considerable time, especially when running on embedded platforms. Therefore, a typical application builds an engine once and then serializes it as a plan file for later use.
The build phase optimizes the network graph by eliminating dead computations, folding constants, and reordering and combining operations to run more efficiently on the GPU. The builder can also be configured to reduce the precision of computations. It can automatically reduce 32-bit floating-point calculations to 16-bit and supports quantization of floating-point values so that calculations can be performed using 8-bit integers. Quantization requires dynamic range information, which can be provided by the application, or calculated by TensorRT using representative network inputs, a process called calibration. The build phase also runs multiple implementations of operators to find those which, when combined with any intermediate precision conversions and layout transformations, yield the fastest overall implementation of the network.
The Network Definition interface provides methods for the application to define a network. Input and output tensors can be specified, and layers can be added and configured. As well as layer types, such as convolutional and recurrent layers, a Plugin layer type allows the application to implement functionality not natively supported by TensorRT. For more information, refer to the Network Definition API. https://docs.nvidia.com/deeplearning/tensorrt/api/c_api/classnvinfer1_1_1_i_network_definition.html
|