一、标准化:将每个特征做均值为0,标准差为1处理。
import numpy as np
import pandas as pd
import sklearn.preprocessing as ps
a = np.array([[17,100,4000],
[20,80,5000],
[23,75,5500]])
one_process = ps.scale(a)
print(one_process )

二、范围缩放:将整列数据缩放到某个范围之间。
mss = ps.MinMaxScaler(feature_range=(0,1))
mss_tm = mss.fit_transform(a)
print(mss_tm)
new = []
num = 0
for i in a.T:
s = i.min()
b = i.max()
arr1 = np.array([[s,1],[b,1]])
arr2 = np.array([0,1])
solve = np.linalg.solve(arr1,arr2)
new.append(i*solve[0]+solve[1])
num += 1
new = np.array(new).T
print(new)
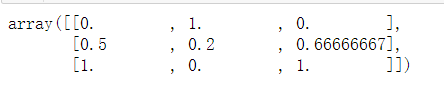 三、归一化:为了找出样本之间的相似性,即每个特征在样本中的比重。 在sklearn中l1范数指每个特征除以各个特征的绝对值之和,l2范数指每个特征除以各个特征的平方之和。
b = np.array([[12,3,9],[20,5,15],[1,3,20]])
p = ps.normalize(b,norm="l1")
print(p)
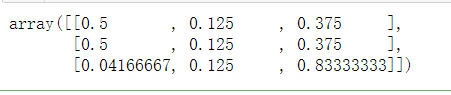 由此可见:样本一和样本二较像。 四、二值化:有些业务并不需要分析矩阵的详细完整数据(比如图片边缘识别只需要分析出图片边缘即可) 可以根据一个事先给定的阈值,用0和1表示特征不高于或高于阈值。二值化后的数组中每个元素非0即1。 达到简化模型的目的。
bins = ps.Binarizer(threshold=81)
anay = bins.transform(a)
print(anay)
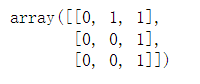
from sklearn.datasets import fetch_olivetti_faces
import matplotlib.pyplot as plt
feaces = fetch_olivetti_faces()
plt.imshow(feaces.images[0],cmap="gray")
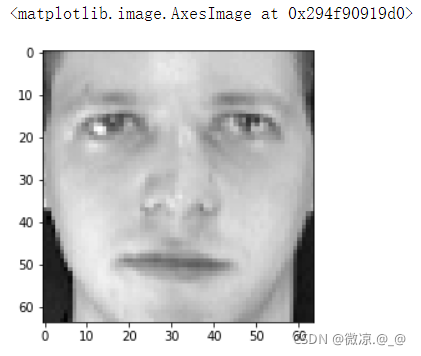
one = feaces.images[0]
mid = np.median(one)
ones = ps.Binarizer(threshold=mid)
ones_data = ones.fit_transform(one)
print(ones_data)
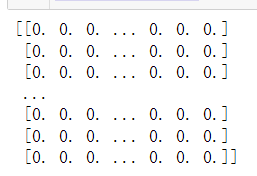
plt.imshow(ones_data,cmap="gray")
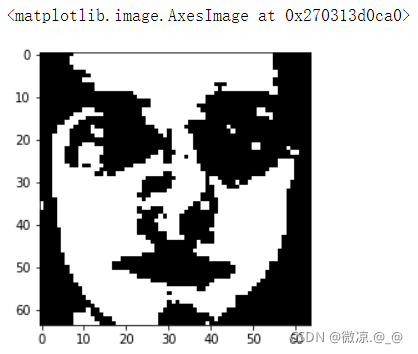 五、独热编码(OneHotEncoding):为样本特征的每个值建立一个由一个1和若干个0组成的序列,用该序列对所有的特征值进行编码。
simple = np.array([[1,3,2],
[7,5,4],
[1,8,6],
[7,3,9]])
ohe = ps.OneHotEncoder(sparse=False,dtype="int64")
rult = ohe.fit_transform(simple)
print(rult)
print(ohe.categories_)
 
simple = np.array([[1,3,2],
[7,5,4],
[1,8,6],
[7,3,9]])
ohe = ps.OneHotEncoder(sparse=True,dtype="int32")
rult = ohe.fit_transform(simple)
print(rult)
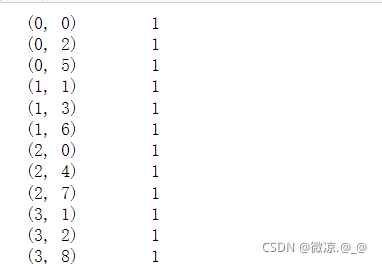 六、标签编码:根据字符串形式的标签在标签序列中的位置,为其指定一个数字标签,用于提供给基于数值算法的学习模型。
label = np.array(["香蕉","苹果","西瓜","香蕉","橙子","西瓜","西瓜","葡萄","杏子","梨子","猕猴桃"])
le = ps.LabelEncoder()
le_label = le.fit_transform(label)
print(le_label)
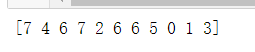
i_list = [0,5,6,7]
inverse = le.inverse_transform(i_list)
print(inverse)
 七、特征编码:根据字符串形式的特征值在特征序列中的位置,为其指定一个数字标签,用于提供给基于数值算法的学习模型。
"""
feathers = np.array([["香蕉","猫","鸡"],
["葡萄","狗","鸭"],
["桃子","猫","鹅"],
["梨子","狗","鹅"]])
oe = ps.OrdinalEncoder(dtype="int64")
oe_feather = oe.fit_transform(feathers)
print(oe_feather)
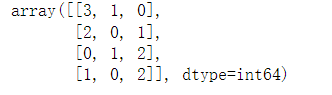 八、k近邻缺失值填补:用k近邻算法填补缺失值。
from sklearn.impute import KNNImputer
df = np.array([[1,2,np.NaN,9],
[4,np.NaN,3,8],
[np.NaN,5,6,7],
[6,4,9,np.NaN]])
KI = KNNImputer(n_neighbors=3)
k_t=KI.fit_transform(df)
print(k_t)
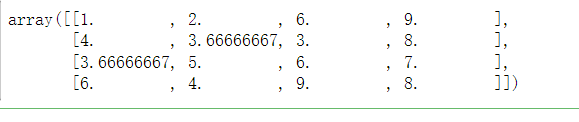
|