数据集介绍
aeroscapes数据集下载链接 AeroScapes 航空语义分割基准包括使用商用无人机在 5 到 50 米的高度范围内捕获的图像。该数据集提供 3269 张 720p 图像和 11 个类别的真实掩码。 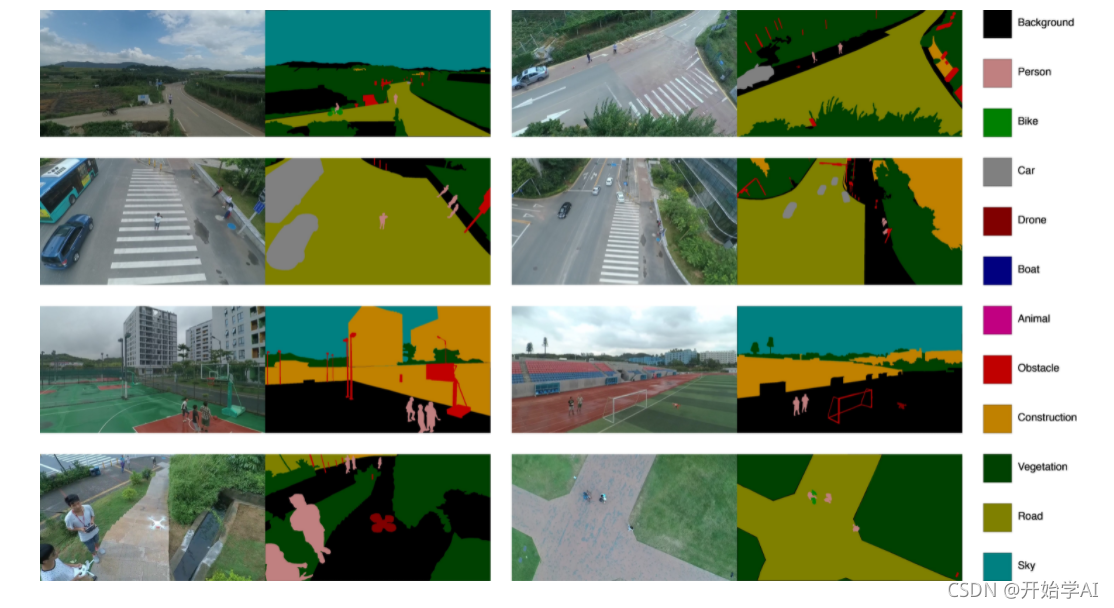
获取Class类别及其RGB值
由于本数据集未提供类别ID对应的RGB值,可以通过以下代码获取:
from PIL import Image
import os
base_dir = "Visualizations/"
base_seg_dir = "SegmentationClass/"
files = os.listdir(base_dir)
list1 = []
for file in files:
img_dir = base_dir + file
segimg_dir = base_seg_dir + file
im = Image.open(img_dir)
segimg = Image.open(segimg_dir)
pix = im.load()
pix_seg = segimg.load()
width = im.size[0]
height = im.size[1]
for x in range(width):
for y in range(height):
r, g, b = pix[x, y]
c = pix_seg[x,y]
if [c,r,g,b] not in list1:
list1.append([c,r,g,b])
print(list1)
print(list1)
结果如下:
Person [192,128,128]--------------1
Bike [0,128,0]----------------------2
Car [128,128,128]----------------- 3
Drone [128,0,0]--------------------4
Boat [0,0,128]--------------------- 5
Animal [192,0,128]---------------- 6
Obstacle [192,0,0]------------------7
Construction [192,128,0]-----------8
Vegetation [0,64,0]-----------------9
Road [128,128,0]-------------------10
Sky [0,128,128]---------------------11
数据加载dataloder写法(基于pytorch)
由于该数据集提供了掩码图,因此不需要进行掩码图转换。下载完成后,文件结构如下:
- ImageSets文件夹:存放了两个txt文件,划分了训练集和验证集。
- JPEGImages文件夹:存放了RGB图像。
- SegmentationClass:存放了标签的掩模图。
- Visualizations:存放了标签图像。
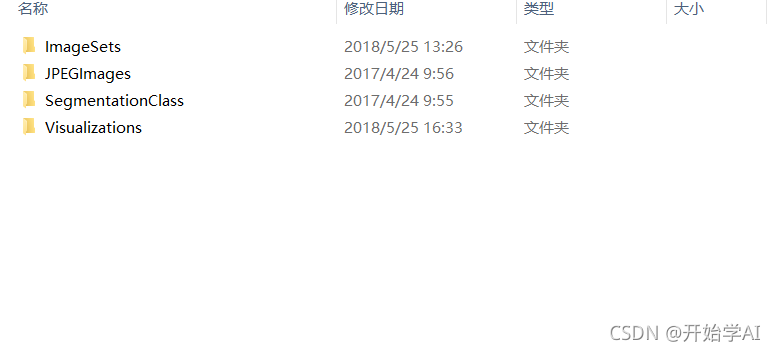 为了使用此数据集,需要根据划分好的txt文件读取图像,然后采用Pytorch的Dataloader模块进行加载。具体代码:
'''
dataset.py
'''
import torch
import torch.utils.data
import numpy as np
import cv2
import os
class DatasetTrain(torch.utils.data.Dataset):
def __init__(self, base_dir):
self.base_dir = base_dir
self.img_dir = base_dir + "JPEGImages/"
self.label_dir = base_dir + "SegmentationClass/"
self.new_img_h = 512
self.new_img_w = 1024
self.examples = []
txt_path = self.base_dir + "ImageSets/trn.txt"
txt_file = open(txt_path)
train_filenames = txt_file.readlines()
train_img_dir_path = self.img_dir
label_img__dir_path = self.label_dir
for train_filename in train_filenames:
train_filename=train_filename.strip('\n')
img_path = train_img_dir_path + train_filename + '.jpg'
label_img_path = label_img__dir_path + train_filename + '.png'
example = {}
example["img_path"] = img_path
example["label_img_path"] = label_img_path
self.examples.append(example)
self.num_examples = len(self.examples)
def __getitem__(self, index):
example = self.examples[index]
img_path = example["img_path"]
print(img_path)
img = cv2.imread(img_path, -1)
img = cv2.resize(img, (self.new_img_w, self.new_img_h),
interpolation=cv2.INTER_NEAREST)
label_img_path = example["label_img_path"]
print(label_img_path)
label_img = cv2.imread(label_img_path, cv2.IMREAD_GRAYSCALE)
label_img = cv2.resize(label_img, (self.new_img_w, self.new_img_h),
interpolation=cv2.INTER_NEAREST)
img = img/255.0
img = img - np.array([0.485, 0.456, 0.406])
img = img/np.array([0.229, 0.224, 0.225])
img = np.transpose(img, (2, 0, 1))
img = img.astype(np.float32)
img = torch.from_numpy(img)
label_img = torch.from_numpy(label_img)
return (img, label_img)
def __len__(self):
return self.num_examples
class DatasetVal(torch.utils.data.Dataset):
def __init__(self, base_dir):
self.base_dir = base_dir
self.img_dir = base_dir + "JPEGImages/"
self.label_dir = base_dir + "SegmentationClass/"
self.new_img_h = 512
self.new_img_w = 1024
self.examples = []
txt_path = self.base_dir + "ImageSets/val.txt"
txt_file = open(txt_path)
valid_filenames = txt_file.readlines()
train_img_dir_path = self.img_dir
label_img__dir_path = self.label_dir
for valid_filename in valid_filenames:
valid_filename=valid_filename.strip('\n')
img_path = train_img_dir_path + valid_filename + '.jpg'
label_img_path = label_img__dir_path + valid_filename + '.png'
example = {}
example["img_path"] = img_path
example["label_img_path"] = label_img_path
self.examples.append(example)
self.num_examples = len(self.examples)
def __getitem__(self, index):
example = self.examples[index]
img_path = example["img_path"]
print(img_path)
img = cv2.imread(img_path, -1)
img = cv2.resize(img, (self.new_img_w, self.new_img_h),
interpolation=cv2.INTER_NEAREST)
label_img_path = example["label_img_path"]
print(label_img_path)
label_img = cv2.imread(label_img_path, cv2.IMREAD_GRAYSCALE)
label_img = cv2.resize(label_img, (self.new_img_w, self.new_img_h),
interpolation=cv2.INTER_NEAREST)
img = img/255.0
img = img - np.array([0.485, 0.456, 0.406])
img = img/np.array([0.229, 0.224, 0.225])
img = np.transpose(img, (2, 0, 1))
img = img.astype(np.float32)
img = torch.from_numpy(img)
label_img = torch.from_numpy(label_img)
return (img, label_img)
def __len__(self):
return self.num_examples
'''
以下代码为测试功能,正式使用时需要注释掉
'''
if __name__ == "__main__":
base_dir = "aeroscapes/"
train_dataset = DatasetTrain(base_dir = base_dir)
train_loader = torch.utils.data.DataLoader(dataset=train_dataset,
batch_size=3, shuffle=True,
num_workers=1,drop_last=True)
val_dataset = DatasetVal(base_dir = base_dir)
val_loader = torch.utils.data.DataLoader(dataset=val_dataset,
batch_size=3, shuffle=True,
num_workers=1,drop_last=True)
from torch.autograd import Variable
for step, (imgs, label_imgs) in enumerate(train_loader):
imgs = Variable(imgs).cuda()
print(imgs.shape)
label_imgs = Variable(label_imgs.type(torch.LongTensor)).cuda()
print(label_imgs.shape)
for step, (imgs, label_imgs) in enumerate(val_loader):
imgs = Variable(imgs).cuda()
print(imgs.shape)
label_imgs = Variable(label_imgs.type(torch.LongTensor)).cuda()
print(label_imgs.shape)
使用前根据自己数据集存放的路径修改base_dir 变量。
|