g2o_viewer问题解决
在进行位姿图优化时候,如果出现g2o_viewer: command not found,说明你的g2o_viewer并没有安装上,打开你之前安装的g2o文件夹,打开bin文件夹,如果没有找到g2o_viewer,说明没有安装上(下图是已经安装好的)。 如果还没有安装g2o的,请参照我之前写的博客:
Ubuntu18.04安装Ceres和g2o库_nudt一枚研究生-CSDN博客一、ceres安装:1.Ctrl+Alt+T打开终端:安装依赖:sudo apt-get install liblapack-dev libsuitesparse-dev libcxsparse3 libgflags-dev libgoogle-glog-dev libgtest-dev2. 下载源码:https://github.com/ceres-solver/ceres-solverGitHub - ceres-solver/ceres-solver: A large s https://blog.csdn.net/weixin_53660567/article/details/120295320Ubuntu20.04安装Ceres和g2o库_nudt一枚研究生-CSDN博客x-special/nautilus-clipboardcutfile:///home/liqiang/Desktop/DeepinScreenshot_Navigator_20210914203012.png一、ceres安装:1.Ctrl+Alt+T打开终端:安装依赖:sudo apt-get install liblapack-dev libsuitesparse-dev libcxsparse3 libgflags-dev libgoogle-glog-dev libgt https://blog.csdn.net/weixin_53660567/article/details/120295824
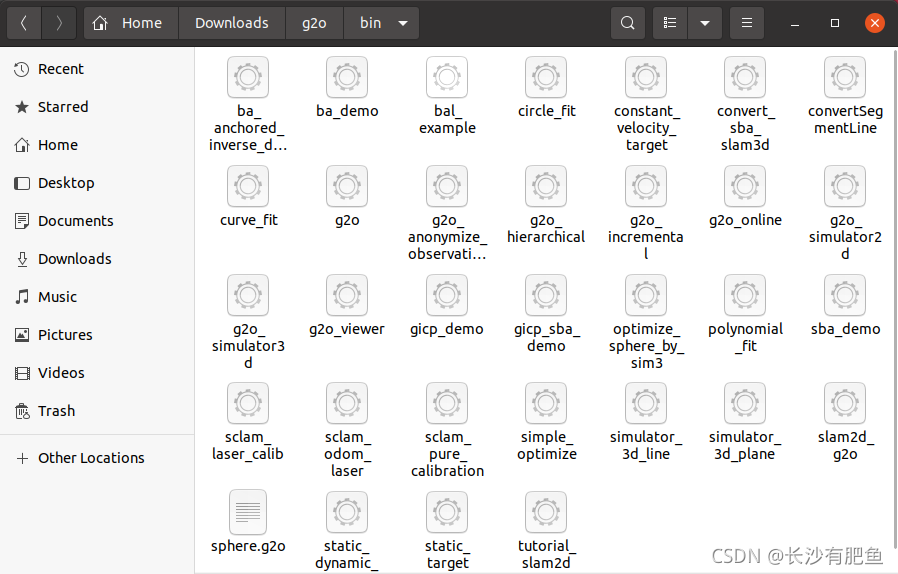
g2o_viewer安装:
安装依赖(可以在g2o/build下面打开终端安装依赖):
sudo apt-get install libeigen3-dev
sudo apt-get install libsuitesparse-dev
sudo apt-get install qtdeclarative5-dev
sudo apt-get install qt5-qmake
sudo apt-get install libqglviewer-dev
进入到g2o/build下面,打开终端:
cmake ..
make -j4
sudo make install
然后在g2o/bin文件夹下面会有可执行文件 g2o_viewer,输入g2o_viewer,就可以打开g2o Viewer。
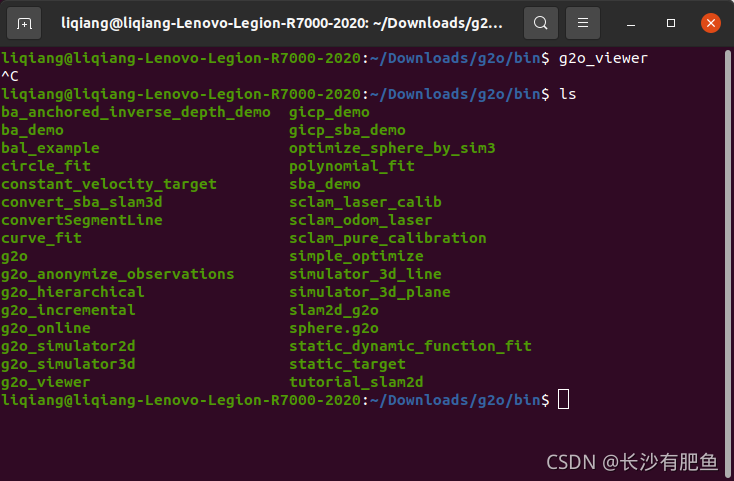
如果出现:
g2o_viewer: error while loading shared libraries: libg2o_viewer.so: cannot open shared object file: No such file or directory
出现这个错误时,进入到g2o/build下面,输入:
sudo ldconfig
然后使用Ctrl+Alt+T打开终端,输入:
g2o_viewer
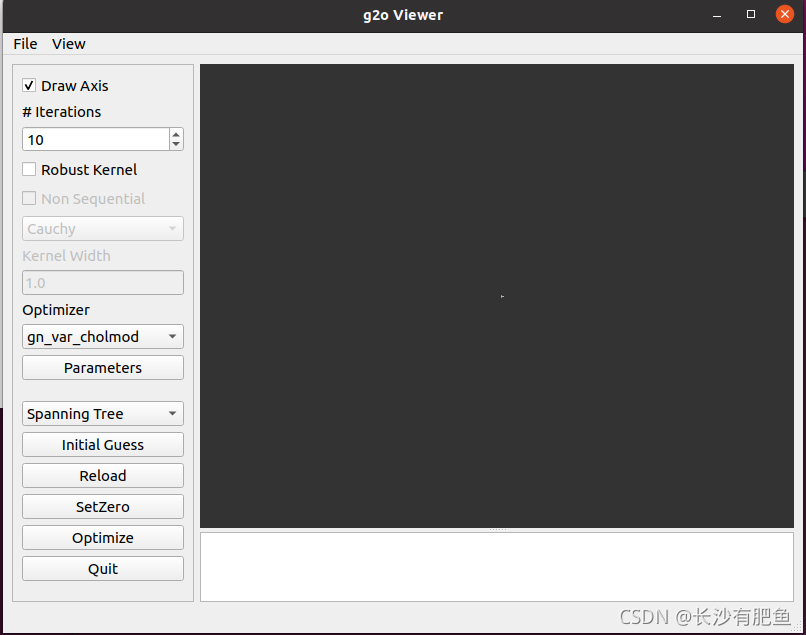
sphere.g2o:
VERTEX_SE3:QUAT 0 -0.125664 -1.53894e-17 99.9999 0.706662 4.32706e-17 0.707551 -4.3325e-17
节点类型是VERTEX_SE3,表达一个相机位姿。g2o默认使用四元数和平移向量表达位姿。上面的数字从左到右依次是: , , , , , , 。前三个为平移向量元素,后四个为表示旋转的单位四元数。
EDGE_SE3:QUAT 0 1 -0.0187953 0.0328449 -0.125146 0.0634648 -0.000250128 0.00237634 0.997981 10000 0 0 0 0 0 10000 0 0 0 0 10000 0 0 0 40000 0 0 40000 0 40000
EDGE_SE3表示边的信息,边的信息为两个节点的ID,? , , , , , , ?以及信息矩阵的右上角,在本例中,信息矩阵的大小为6x6,且被设成了对角阵。
查看sphere.g2o文件:
方法1:
点击File->load,打开sphere.g2o文件
方法2:
在ch10文件夹下打开终端,执行下面的命令
g2o_viewer sphere.g2o
点击Optimize:
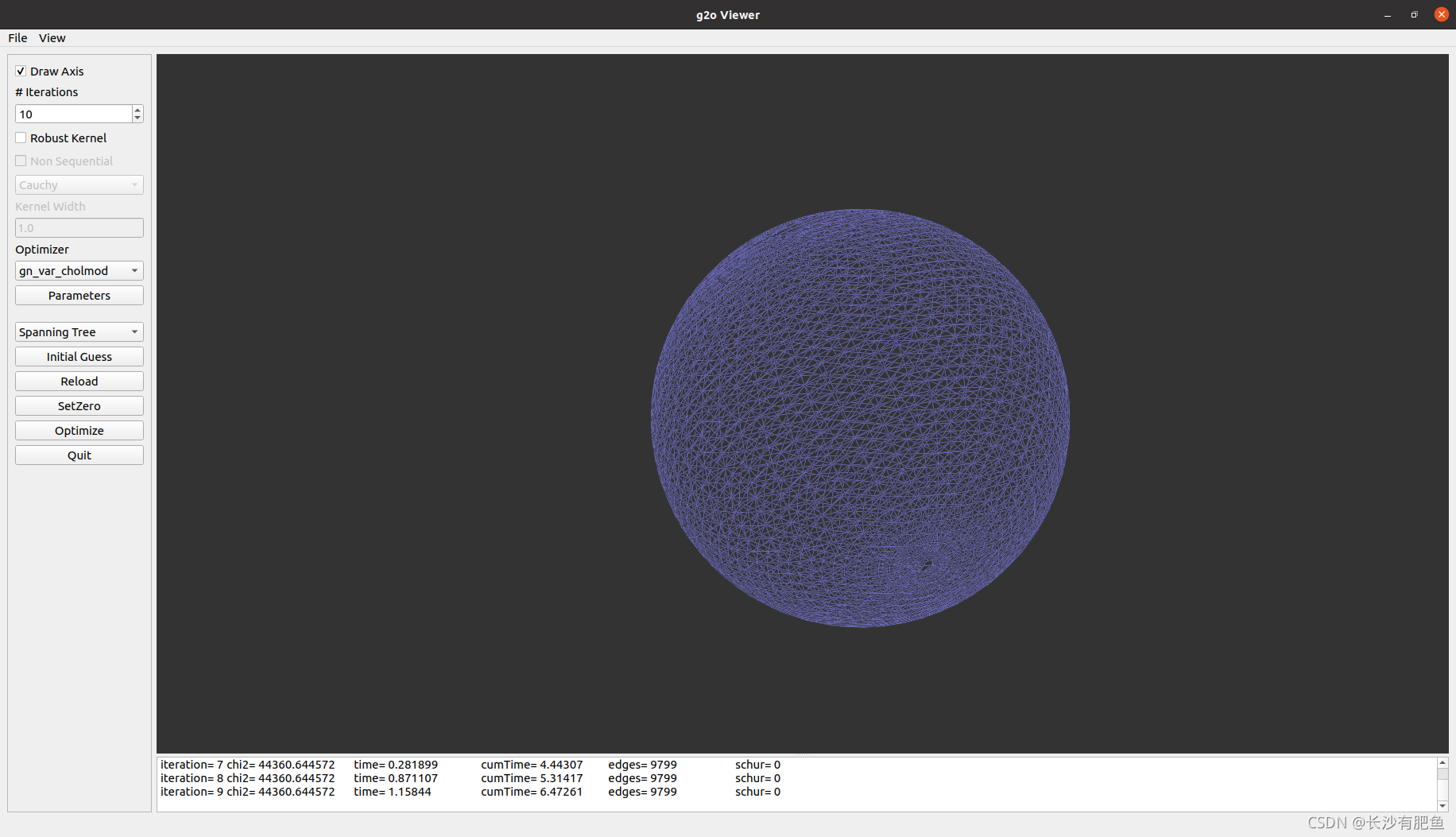
优化结果:
loaded sphere.g2o with 2500 vertices and 9799 measurements
graph is fixed by node 2499
# Using CHOLMOD poseDim -1 landMarkDim -1 blockordering 1
Preparing (no marginalization of Landmarks)
iteration= 0 chi2= 2080902190.865136 time= 0.116081 cumTime= 0.116081 edges= 9799 schur= 0
iteration= 1 chi2= 18794278.949871 time= 0.451063 cumTime= 0.567144 edges= 9799 schur= 0
iteration= 2 chi2= 59504.776209 time= 1.0643 cumTime= 1.63145 edges= 9799 schur= 0
iteration= 3 chi2= 44360.707890 time= 0.457449 cumTime= 2.0889 edges= 9799 schur= 0
iteration= 4 chi2= 44360.644572 time= 0.747359 cumTime= 2.83626 edges= 9799 schur= 0
iteration= 5 chi2= 44360.644572 time= 1.23642 cumTime= 4.07268 edges= 9799 schur= 0
iteration= 6 chi2= 44360.644572 time= 0.0884912 cumTime= 4.16117 edges= 9799 schur= 0
iteration= 7 chi2= 44360.644572 time= 0.281899 cumTime= 4.44307 edges= 9799 schur= 0
iteration= 8 chi2= 44360.644572 time= 0.871107 cumTime= 5.31417 edges= 9799 schur= 0
iteration= 9 chi2= 44360.644572 time= 1.15844 cumTime= 6.47261 edges= 9799 schur= 0
pose_graph_g2o_SE3.cpp
#include <iostream>
#include <fstream>//文件读取头文件
#include <string>
#include <g2o/types/slam3d/types_slam3d.h>// g2o/types/slam3d/中的SE3表示位姿
#include <g2o/core/block_solver.h>//求解器
#include <g2o/core/optimization_algorithm_levenberg.h>//列文伯格——马尔夸特算法头文件
#include <g2o/solvers/eigen/linear_solver_eigen.h>
using namespace std;
/************************************************
* 本程序演示如何用g2o solver进行位姿图优化
* sphere.g2o是人工生成的一个Pose graph,我们来优化它。
* 尽管可以直接通过load函数读取整个图,但我们还是自己来实现读取代码,以期获得更深刻的理解
* 这里使用g2o/types/slam3d/中的SE3表示位姿,它实质上是四元数而非李代数.
* **********************************************/
int main(int argc, char **argv)
{
//读取sphere.g2o文件
if (argc != 2) {
cout << "Usage: pose_graph_g2o_SE3 sphere.g2o" << endl;//输出用法
return 1;
}
ifstream fin(argv[1]);
if (!fin) {
cout << "file " << argv[1] << " does not exist." << endl;
return 1;
}
// 构建图优化,先设定g2o
typedef g2o::BlockSolver<g2o::BlockSolverTraits<6, 6>> BlockSolverType; //每个误差项优化变量维度为6,误差值维度为6
typedef g2o::LinearSolverEigen<BlockSolverType::PoseMatrixType> LinearSolverType;//线性求解器类型
// 梯度下降方法,可以从GN, LM, DogLeg 中选
auto solver = new g2o::OptimizationAlgorithmLevenberg(
g2o::make_unique<BlockSolverType>(g2o::make_unique<LinearSolverType>()));
//c++中的make_unique表示智能指针类型
g2o::SparseOptimizer optimizer; // 图模型
optimizer.setAlgorithm(solver); // 设置求解器
optimizer.setVerbose(true); // 打开调试输出
int vertexCnt = 0, edgeCnt = 0; // 顶点和边的数量
while (!fin.eof()) {
string name;
fin >> name;
if (name == "VERTEX_SE3:QUAT")//当数据是节点类型VERTEX_SE3:QUAT时
{
// SE3 顶点
g2o::VertexSE3 *v = new g2o::VertexSE3();//指针v
int index = 0;
fin >> index;
v->setId(index);//对边进行编号
v->read(fin);
optimizer.addVertex(v);
vertexCnt++;//遍历所有节点
if (index == 0)
v->setFixed(true);
} else if (name == "EDGE_SE3:QUAT") //当数据是边的信息EDGE_SE3:QUAT时
{
// SE3-SE3 边
g2o::EdgeSE3 *e = new g2o::EdgeSE3();//指针e
int idx1, idx2; // 关联的两个顶点
fin >> idx1 >> idx2;
e->setId(edgeCnt++);//遍历所有边
e->setVertex(0, optimizer.vertices()[idx1]);
e->setVertex(1, optimizer.vertices()[idx2]);
e->read(fin);
optimizer.addEdge(e);
}
if (!fin.good()) break;
}
cout << "read total " << vertexCnt << " vertices, " << edgeCnt << " edges." << endl;//输出共有多少个顶点和边
cout << "optimizing ..." << endl;//输出optimizing ...优化后
optimizer.initializeOptimization();//优化过程初始化
optimizer.optimize(30);//设置优化的迭代次数为30次
cout << "saving optimization results ..." << endl;//输出储存结果
optimizer.save("result.g2o");//在build文件夹下存储为result.g2o文件
return 0;
}
CMakeLists.txt
cmake_minimum_required(VERSION 2.8)
project(pose_graph)
set(CMAKE_BUILD_TYPE "Release")
set(CMAKE_CXX_FLAGS "-std=c++14 -O3")
list(APPEND CMAKE_MODULE_PATH ${PROJECT_SOURCE_DIR}/cmake_modules)
# Eigen
include_directories("/usr/include/eigen3")
# sophus
find_package(Sophus REQUIRED)
include_directories(${Sophus_INCLUDE_DIRS})
# g2o
find_package(G2O REQUIRED)
include_directories(${G2O_INCLUDE_DIRS})
add_executable(pose_graph_g2o_SE3 pose_graph_g2o_SE3.cpp)
target_link_libraries(pose_graph_g2o_SE3
g2o_core g2o_stuff g2o_types_slam3d ${CHOLMOD_LIBRARIES}
)
add_executable(pose_graph_g2o_lie pose_graph_g2o_lie_algebra.cpp)
target_link_libraries(pose_graph_g2o_lie
g2o_core g2o_stuff
${CHOLMOD_LIBRARIES}
${Sophus_LIBRARIES}
)
执行结果:
./pose_graph_g2o_SE3 ../sphere.g2o
read total 2500 vertices, 9799 edges.
optimizing ...
iteration= 0 chi2= 1023011093.967642 time= 0.412224 cumTime= 0.412224 edges= 9799 schur= 0 lambda= 805.622433 levenbergIter= 1
iteration= 1 chi2= 385118688.233188 time= 0.396826 cumTime= 0.809049 edges= 9799 schur= 0 lambda= 537.081622 levenbergIter= 1
iteration= 2 chi2= 166223726.693658 time= 0.395324 cumTime= 1.20437 edges= 9799 schur= 0 lambda= 358.054415 levenbergIter= 1
iteration= 3 chi2= 86610874.269316 time= 0.40099 cumTime= 1.60536 edges= 9799 schur= 0 lambda= 238.702943 levenbergIter= 1
iteration= 4 chi2= 40582782.710189 time= 0.404917 cumTime= 2.01028 edges= 9799 schur= 0 lambda= 159.135295 levenbergIter= 1
iteration= 5 chi2= 15055383.753040 time= 0.407228 cumTime= 2.41751 edges= 9799 schur= 0 lambda= 101.425210 levenbergIter= 1
iteration= 6 chi2= 6715193.487654 time= 0.414222 cumTime= 2.83173 edges= 9799 schur= 0 lambda= 37.664667 levenbergIter= 1
iteration= 7 chi2= 2171949.168383 time= 0.402862 cumTime= 3.23459 edges= 9799 schur= 0 lambda= 12.554889 levenbergIter= 1
iteration= 8 chi2= 740566.827049 time= 0.403515 cumTime= 3.63811 edges= 9799 schur= 0 lambda= 4.184963 levenbergIter= 1
iteration= 9 chi2= 313641.802464 time= 0.406507 cumTime= 4.04461 edges= 9799 schur= 0 lambda= 2.583432 levenbergIter= 1
iteration= 10 chi2= 82659.743578 time= 0.397021 cumTime= 4.44164 edges= 9799 schur= 0 lambda= 0.861144 levenbergIter= 1
iteration= 11 chi2= 58220.369189 time= 0.427051 cumTime= 4.86869 edges= 9799 schur= 0 lambda= 0.287048 levenbergIter= 1
iteration= 12 chi2= 52214.188561 time= 0.410727 cumTime= 5.27941 edges= 9799 schur= 0 lambda= 0.095683 levenbergIter= 1
iteration= 13 chi2= 50948.580336 time= 0.407471 cumTime= 5.68689 edges= 9799 schur= 0 lambda= 0.031894 levenbergIter= 1
iteration= 14 chi2= 50587.776729 time= 0.396986 cumTime= 6.08387 edges= 9799 schur= 0 lambda= 0.016436 levenbergIter= 1
iteration= 15 chi2= 50233.038802 time= 0.394694 cumTime= 6.47856 edges= 9799 schur= 0 lambda= 0.010957 levenbergIter= 1
iteration= 16 chi2= 49995.082836 time= 0.395463 cumTime= 6.87403 edges= 9799 schur= 0 lambda= 0.007305 levenbergIter= 1
iteration= 17 chi2= 48876.738968 time= 0.784587 cumTime= 7.65861 edges= 9799 schur= 0 lambda= 0.009298 levenbergIter= 2
iteration= 18 chi2= 48806.625520 time= 0.394058 cumTime= 8.05267 edges= 9799 schur= 0 lambda= 0.006199 levenbergIter= 1
iteration= 19 chi2= 47790.891374 time= 0.780777 cumTime= 8.83345 edges= 9799 schur= 0 lambda= 0.008265 levenbergIter= 2
iteration= 20 chi2= 47713.626578 time= 0.39445 cumTime= 9.2279 edges= 9799 schur= 0 lambda= 0.005510 levenbergIter= 1
iteration= 21 chi2= 46869.323691 time= 0.780449 cumTime= 10.0083 edges= 9799 schur= 0 lambda= 0.007347 levenbergIter= 2
iteration= 22 chi2= 46802.585509 time= 0.397007 cumTime= 10.4054 edges= 9799 schur= 0 lambda= 0.004898 levenbergIter= 1
iteration= 23 chi2= 46128.758046 time= 0.7841 cumTime= 11.1895 edges= 9799 schur= 0 lambda= 0.006489 levenbergIter= 2
iteration= 24 chi2= 46069.133544 time= 0.395788 cumTime= 11.5852 edges= 9799 schur= 0 lambda= 0.004326 levenbergIter= 1
iteration= 25 chi2= 45553.862168 time= 0.781823 cumTime= 12.3671 edges= 9799 schur= 0 lambda= 0.005595 levenbergIter= 2
iteration= 26 chi2= 45511.762622 time= 0.395914 cumTime= 12.763 edges= 9799 schur= 0 lambda= 0.003730 levenbergIter= 1
iteration= 27 chi2= 45122.763002 time= 0.780705 cumTime= 13.5437 edges= 9799 schur= 0 lambda= 0.004690 levenbergIter= 2
iteration= 28 chi2= 45095.174401 time= 0.39546 cumTime= 13.9391 edges= 9799 schur= 0 lambda= 0.003127 levenbergIter= 1
iteration= 29 chi2= 44811.248507 time= 0.781468 cumTime= 14.7206 edges= 9799 schur= 0 lambda= 0.003785 levenbergIter= 2
saving optimization results ...
?sphere.g2o:
VERTEX_SE3:QUAT 0 -0.125664 -1.53894e-17 99.9999 0.706662 4.32706e-17 0.707551 -4.3325e-17
VERTEX_SE3:QUAT 1 -0.250786 -0.0328449 99.981 0.705413 0.0432253 0.705946 -0.0465295
VERTEX_SE3:QUAT 2 -0.384479 -0.102155 99.9722 0.701473 0.0869233 0.701311 -0.0924285
VERTEX_SE3:QUAT 3 -0.488061 -0.195958 99.9833 0.689802 0.131752 0.698923 -0.135355
VERTEX_SE3:QUAT 4 -0.577441 -0.319656 99.9749 0.683889 0.173189 0.684097 -0.185235
VERTEX_SE3:QUAT 5 -0.626233 -0.455465 99.9639 0.675686 0.20944 0.672757 -0.216751
VERTEX_SE3:QUAT 6 -0.653603 -0.602648 99.9579 0.658288 0.246239 0.662316 -0.259541
VERTEX_SE3:QUAT 7 -0.660457 -0.786874 99.9504 0.637158 0.294767 0.642758 -0.306601
VERTEX_SE3:QUAT 8 -0.618421 -0.981472 99.946 0.627982 0.330033 0.615145 -0.343967
...........
EDGE_SE3:QUAT 0 1 -0.0187953 0.0328449 -0.125146 0.0634648 -0.000250128 0.00237634 0.997981 10000 0 0 0 0 0 10000 0 0 0 0 10000 0 0 0 40000 0 0 40000 0 40000
EDGE_SE3:QUAT 1 2 -0.00836587 0.0518559 -0.141405 0.0636098 -0.000538 0.00162238 0.997973 10000 0 0 0 0 0 10000 0 0 0 0 10000 0 0 0 40000 0 0 40000 0 40000
EDGE_SE3:QUAT 2 3 0.0116518 0.0646362 -0.123843 0.0628369 0.0060981 -0.00213561 0.998003 10000 0 0 0 0 0 10000 0 0 0 0 10000 0 0 0 40000 0 0 40000 0 40000
EDGE_SE3:QUAT 3 4 -0.00635662 0.0817346 -0.128989 0.0661221 -0.0051306 0.00750755 0.99777 10000 0 0 0 0 0 10000 0 0 0 0 10000 0 0 0 40000 0 0 40000 0 40000
EDGE_SE3:QUAT 4 5 -0.00900338 0.0946003 -0.109164 0.0498363 -0.00340117 -0.00255259 0.998748 10000 0 0 0 0 0 10000 0 0 0 0 10000 0 0 0 40000 0 0 40000 0 40000
EDGE_SE3:QUAT 5 6 -0.00495715 0.104779 -0.106982 0.0596275 0.00563598 0.00254199 0.998202 10000 0 0 0 0 0 10000 0 0 0 0 10000 0 0 0 40000 0 0 40000 0 40000
EDGE_SE3:QUAT 6 7 -0.00385872 0.132603 -0.128231 0.0734198 0.000112718 -0.000902908 0.997301 10000 0 0 0 0 0 10000 0 0 0 0 10000 0 0 0 40000 0 0 40000 0 40000
EDGE_SE3:QUAT 7 8 -0.000783881 0.156605 -0.122999 0.0574284 -0.0114936 0.00730844 0.998257 10000 0 0 0 0 0 10000 0 0 0 0 10000 0 0 0 40000 0 0 40000 0 40000
EDGE_SE3:QUAT 8 9 -0.00262376 0.131903 -0.124046 0.0674511 0.00698356 0.00882454 0.997659 10000 0 0 0 0 0 10000 0 0 0 0 10000 0 0 0 40000 0 0 40000 0 40000
EDGE_SE3:QUAT 9 10 0.00417907 0.167845 -0.123652 0.0678472 0.00148376 0.0030903 0.99769 10000 0 0 0 0 0 10000 0 0 0 0 10000 0 0 0 40000 0 0 40000 0 40000
result.g2o:
VERTEX_SE3:QUAT 0 -0.125664 -1.53894e-17 99.9999 0.706662 4.32706e-17 0.707551 -4.3325e-17
FIX 0
VERTEX_SE3:QUAT 1 -0.2447 -0.0287003 99.9832 0.704617 0.0231823 0.70586 -0.0688426
VERTEX_SE3:QUAT 2 -0.370162 -0.0884744 99.9776 0.697957 0.0591073 0.703232 -0.121767
VERTEX_SE3:QUAT 3 -0.478226 -0.175864 99.9885 0.68874 0.100025 0.698127 -0.16808
VERTEX_SE3:QUAT 4 -0.56488 -0.291548 99.975 0.676789 0.139138 0.689709 -0.216564
VERTEX_SE3:QUAT 5 -0.624303 -0.425521 99.9516 0.661416 0.182572 0.679895 -0.258725
VERTEX_SE3:QUAT 6 -0.660524 -0.576074 99.933 0.644081 0.223744 0.666222 -0.30207
VERTEX_SE3:QUAT 7 -0.659218 -0.741865 99.9144 0.622089 0.265154 0.652111 -0.34271
VERTEX_SE3:QUAT 8 -0.615012 -0.927527 99.8896 0.601222 0.303189 0.633667 -0.380887
.................
EDGE_SE3:QUAT 0 1 -0.0187953 0.0328449 -0.125146 0.0634648 -0.000250128 0.00237634 0.997981 10000 0 0 0 0 0 10000 0 0 0 0 10000 0 0 0 40000 0 0 40000 0 40000
EDGE_SE3:QUAT 1 2 -0.00836587 0.0518559 -0.141405 0.0636098 -0.000538 0.00162238 0.997973 10000 0 0 0 0 0 10000 0 0 0 0 10000 0 0 0 40000 0 0 40000 0 40000
EDGE_SE3:QUAT 2 3 0.0116518 0.0646362 -0.123843 0.0628369 0.0060981 -0.00213561 0.998003 10000 0 0 0 0 0 10000 0 0 0 0 10000 0 0 0 40000 0 0 40000 0 40000
EDGE_SE3:QUAT 3 4 -0.00635662 0.0817346 -0.128989 0.0661221 -0.0051306 0.00750755 0.99777 10000 0 0 0 0 0 10000 0 0 0 0 10000 0 0 0 40000 0 0 40000 0 40000
EDGE_SE3:QUAT 4 5 -0.00900338 0.0946003 -0.109164 0.0498363 -0.00340117 -0.00255259 0.998748 10000 0 0 0 0 0 10000 0 0 0 0 10000 0 0 0 40000 0 0 40000 0 40000
EDGE_SE3:QUAT 5 6 -0.00495715 0.104779 -0.106982 0.0596275 0.00563598 0.00254199 0.998202 10000 0 0 0 0 0 10000 0 0 0 0 10000 0 0 0 40000 0 0 40000 0 40000
EDGE_SE3:QUAT 6 7 -0.00385872 0.132603 -0.128231 0.0734198 0.000112718 -0.000902908 0.997301 10000 0 0 0 0 0 10000 0 0 0 0 10000 0 0 0 40000 0 0 40000 0 40000
EDGE_SE3:QUAT 7 8 -0.000783881 0.156605 -0.122999 0.0574284 -0.0114936 0.00730844 0.998257 10000 0 0 0 0 0 10000 0 0 0 0 10000 0 0 0 40000 0 0 40000 0 40000
EDGE_SE3:QUAT 8 9 -0.00262376 0.131903 -0.124046 0.0674511 0.00698356 0.00882454 0.997659 10000 0 0 0 0 0 10000 0 0 0 0 10000 0 0 0 40000 0 0 40000 0 40000
EDGE_SE3:QUAT 9 10 0.00417907 0.167845 -0.123652 0.0678472 0.00148376 0.0030903 0.99769 10000 0 0 0 0 0 10000 0 0 0 0 10000 0 0 0 40000 0 0 40000 0 40000
?result.g2o查看:
在ch10/build下面:
g2o_viewer result.g2o
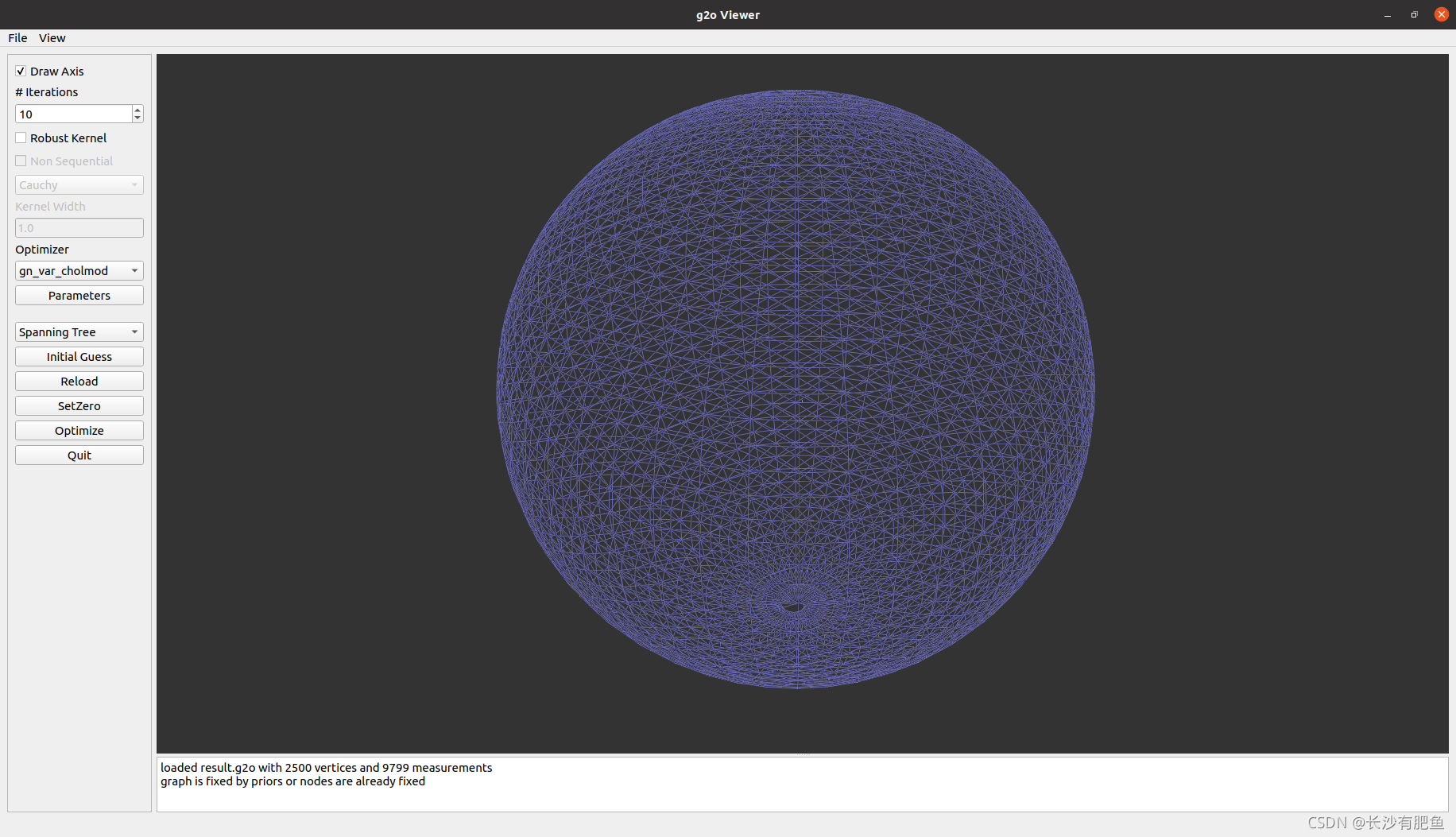
和上面点击Optimize进行优化后的结果没有什么区别。
pose_graph_g2o_lie_algebra.cpp
#include <iostream>
#include <fstream>//文件读取头文件
#include <string>
#include <Eigen/Core>//Eigen核心模块
#include <g2o/core/base_vertex.h>//g2o顶点(Vertex)头文件 视觉slam十四讲p141用顶点表示优化变量,用边表示误差项
#include <g2o/core/base_binary_edge.h>//g2o边(edge)头文件
#include <g2o/core/block_solver.h>//求解器头文件
#include <g2o/core/optimization_algorithm_levenberg.h>//列文伯格——马尔夸特算法头文件
#include <g2o/solvers/eigen/linear_solver_eigen.h>
#include <sophus/se3.hpp>
using namespace std;
using namespace Eigen;
using Sophus::SE3d;//在SE3d子类中引用基类Sophus的成员
using Sophus::SO3d;//在SO3d子类中引用基类Sophus的成员
/************************************************
* 本程序演示如何用g2o solver进行位姿图优化
* sphere.g2o是人工生成的一个Pose graph,我们来优化它。
* 尽管可以直接通过load函数读取整个图,但我们还是自己来实现读取代码,以期获得更深刻的理解
* 本节使用李代数表达位姿图,节点和边的方式为自定义
* **********************************************/
typedef Matrix<double, 6, 6> Matrix6d;
// 给定误差求J_R^{-1}的近似
Matrix6d JRInv(const SE3d &e) {
Matrix6d J;//雅可比矩阵
//视觉SLAM十四讲p272式(10.11)
// | φe(^) ρe(^) | | E 0 | | φe(^) ρe(^) |
//Jr(-1)≈I+(1/2)| | = | | + (1/2)| |
// | 0 φe(^) | | 0 E | | 0 φe(^) |
J.block(0, 0, 3, 3) = SO3d::hat(e.so3().log());//E+(1/2)φe(^)
J.block(0, 3, 3, 3) = SO3d::hat(e.translation());//(1/2)ρe(^)
J.block(3, 0, 3, 3) = Matrix3d::Zero(3, 3);//0
J.block(3, 3, 3, 3) = SO3d::hat(e.so3().log());//E+(1/2)φe(^)
// J = J * 0.5 + Matrix6d::Identity();
J = Matrix6d::Identity(); // try Identity if you want 用单位阵来近似雅可比矩阵
return J;
}
// 李代数顶点
typedef Matrix<double, 6, 1> Vector6d;
class VertexSE3LieAlgebra : public g2o::BaseVertex<6, SE3d> //public表示公有继承;VertexSE3LieAlgebra是派生类,BaseVertex<3, Eigen::Vector3d>是基类
{
public://以下定义的成员变量和成员函数都是公有的
EIGEN_MAKE_ALIGNED_OPERATOR_NEW//解决Eigen库数据结构内存对齐问题
virtual bool read(istream &is) override //istream类是c++标准输入流的一个基类
//可参照C++ Primer Plus第六版的6.8节
{
double data[7];//定义数组
for (int i = 0; i < 7; i++)
is >> data[i];
setEstimate(SE3d(
Quaterniond(data[6], data[3], data[4], data[5]),
Vector3d(data[0], data[1], data[2])
));//Quaterniond表示四元数qw qx qy qz Vector3d表示平移向量元素tx ty tz
//return true;
}
virtual bool write(ostream &os) const override //ostream类是c++标准输出流的一个基类
//可参照C++ Primer Plus第六版的6.8节
{
os << id() << " ";
Quaterniond q = _estimate.unit_quaternion();
os << _estimate.translation().transpose() << " ";
os << q.coeffs()[0] << " " << q.coeffs()[1] << " " << q.coeffs()[2] << " " << q.coeffs()[3] << endl;
return true;
}
virtual void setToOriginImpl() override //virtual表示该函数为虚函数,override保留字表示当前函数重写了基类的虚函数
{
_estimate = SE3d();
}
// 左乘更新
virtual void oplusImpl(const double *update) override
{
Vector6d upd;
upd << update[0], update[1], update[2], update[3], update[4], update[5];
_estimate = SE3d::exp(upd) * _estimate;
}
};
// 两个李代数节点之边
class EdgeSE3LieAlgebra : public g2o::BaseBinaryEdge<6, SE3d, VertexSE3LieAlgebra, VertexSE3LieAlgebra> {
public://以下定义的成员变量和成员函数都是公有的
EIGEN_MAKE_ALIGNED_OPERATOR_NEW//解决Eigen库数据结构内存对齐问题
virtual bool read(istream &is) override //istream类是c++标准输入流的一个基类
//可参照C++ Primer Plus第六版的6.8节
{
double data[7];
for (int i = 0; i < 7; i++)
is >> data[i];
Quaterniond q(data[6], data[3], data[4], data[5]);//四元数
q.normalize();//归一化
setMeasurement(SE3d(q, Vector3d(data[0], data[1], data[2])));
for (int i = 0; i < information().rows() && is.good(); i++)
for (int j = i; j < information().cols() && is.good(); j++) {
is >> information()(i, j);
if (i != j)
information()(j, i) = information()(i, j);
}
return true;
}
virtual bool write(ostream &os) const override
{
VertexSE3LieAlgebra *v1 = static_cast<VertexSE3LieAlgebra *> (_vertices[0]);//定义指针v1
VertexSE3LieAlgebra *v2 = static_cast<VertexSE3LieAlgebra *> (_vertices[1]);//定义指针v2
os << v1->id() << " " << v2->id() << " ";
SE3d m = _measurement;
Eigen::Quaterniond q = m.unit_quaternion();
os << m.translation().transpose() << " ";
os << q.coeffs()[0] << " " << q.coeffs()[1] << " " << q.coeffs()[2] << " " << q.coeffs()[3] << " ";//输出四元数
// information matrix 信息矩阵
for (int i = 0; i < information().rows(); i++)
for (int j = i; j < information().cols(); j++) {
os << information()(i, j) << " ";
}
os << endl;
return true;
}
// 误差计算与书中推导一致
virtual void computeError() override {
SE3d v1 = (static_cast<VertexSE3LieAlgebra *> (_vertices[0]))->estimate();
SE3d v2 = (static_cast<VertexSE3LieAlgebra *> (_vertices[1]))->estimate();
//视觉SLAM十四讲p271式(10.4)
//eij(^)=Δξij*In(Tij(-1)Ti(-1)Tj)^
_error = (_measurement.inverse() * v1.inverse() * v2).log();
//_measurement.inverse() -> Tij(-1)
//v1.inverse() -> Ti(-1)
//Tj -> v2
//.log()表示In()
}
// 雅可比计算
virtual void linearizeOplus() override {
SE3d v1 = (static_cast<VertexSE3LieAlgebra *> (_vertices[0]))->estimate();
SE3d v2 = (static_cast<VertexSE3LieAlgebra *> (_vertices[1]))->estimate();
Matrix6d J = JRInv(SE3d::exp(_error));//使用TRInv()函数提供近似的Jr(-1)
// 尝试把J近似为I?
_jacobianOplusXi = -J * v2.inverse().Adj();//视觉SLAM十四讲式(10.9)
_jacobianOplusXj = J * v2.inverse().Adj();//视觉SLAM十四讲式(10.10)
}
};
int main(int argc, char **argv) {
if (argc != 2) {
cout << "Usage: pose_graph_g2o_SE3_lie sphere.g2o" << endl;//输出使用方法
return 1;
}
ifstream fin(argv[1]);
if (!fin) {
cout << "file " << argv[1] << " does not exist." << endl;
return 1;
}
// 设定g2o
typedef g2o::BlockSolver<g2o::BlockSolverTraits<6, 6>> BlockSolverType;//每个误差项优化变量维度为6,误差值维度为6
typedef g2o::LinearSolverEigen<BlockSolverType::PoseMatrixType> LinearSolverType;//线性求解器类型
// 梯度下降方法,可以从GN, LM, DogLeg 中选
auto solver = new g2o::OptimizationAlgorithmLevenberg(
g2o::make_unique<BlockSolverType>(g2o::make_unique<LinearSolverType>()));
g2o::SparseOptimizer optimizer; // 图模型
optimizer.setAlgorithm(solver); // 设置求解器
optimizer.setVerbose(true); // 打开调试输出
int vertexCnt = 0, edgeCnt = 0; // 顶点和边的数量
vector<VertexSE3LieAlgebra *> vectices;
vector<EdgeSE3LieAlgebra *> edges;
while (!fin.eof()) {
string name;
fin >> name;
if (name == "VERTEX_SE3:QUAT") //当数据是节点类型VERTEX_SE3:QUAT时
{
// 顶点
VertexSE3LieAlgebra *v = new VertexSE3LieAlgebra();//指针v
int index = 0;
fin >> index;
v->setId(index);//对边进行编号
v->read(fin);
optimizer.addVertex(v);
vertexCnt++;//遍历所有节点
vectices.push_back(v);
if (index == 0)
v->setFixed(true);
} else if (name == "EDGE_SE3:QUAT") {
// SE3-SE3 边
EdgeSE3LieAlgebra *e = new EdgeSE3LieAlgebra();//指针e
int idx1, idx2; // 关联的两个顶点
fin >> idx1 >> idx2;
e->setId(edgeCnt++);//遍历所有边
e->setVertex(0, optimizer.vertices()[idx1]);
e->setVertex(1, optimizer.vertices()[idx2]);
e->read(fin);
optimizer.addEdge(e);
edges.push_back(e);
}
if (!fin.good()) break;
}
cout << "read total " << vertexCnt << " vertices, " << edgeCnt << " edges." << endl;//输出共有多少个顶点和边
cout << "optimizing ..." << endl;//输出optimizing ...优化后
optimizer.initializeOptimization();//优化过程初始化
optimizer.optimize(30);//设置优化的迭代次数为30次
cout << "saving optimization results ..." << endl;
// 因为用了自定义顶点且没有向g2o注册,这里保存自己来实现
// 伪装成 SE3 顶点和边,让 g2o_viewer 可以认出
ofstream fout("result_lie.g2o");
for (VertexSE3LieAlgebra *v:vectices) {
fout << "VERTEX_SE3:QUAT ";
v->write(fout);
}
for (EdgeSE3LieAlgebra *e:edges) {
fout << "EDGE_SE3:QUAT ";
e->write(fout);
}
fout.close();
return 0;
}
CMakeLists.txt
cmake_minimum_required(VERSION 2.8)
project(pose_graph)
set(CMAKE_BUILD_TYPE "Release")
set(CMAKE_CXX_FLAGS "-std=c++14 -O3")
list(APPEND CMAKE_MODULE_PATH ${PROJECT_SOURCE_DIR}/cmake_modules)
# Eigen
include_directories("/usr/include/eigen3")
# sophus
find_package(Sophus REQUIRED)
include_directories(${Sophus_INCLUDE_DIRS})
# g2o
find_package(G2O REQUIRED)
include_directories(${G2O_INCLUDE_DIRS})
add_executable(pose_graph_g2o_SE3 pose_graph_g2o_SE3.cpp)
target_link_libraries(pose_graph_g2o_SE3
g2o_core g2o_stuff g2o_types_slam3d ${CHOLMOD_LIBRARIES}
)
add_executable(pose_graph_g2o_lie pose_graph_g2o_lie_algebra.cpp)
target_link_libraries(pose_graph_g2o_lie
g2o_core g2o_stuff
${CHOLMOD_LIBRARIES}
${Sophus_LIBRARIES}
)
报错解决:
Sophus ensure failed in function 'void Sophus::SO3Base<Derived>::normalize() [with Derived = Sophus::SO3<double>]', file '/usr/local/include/sophus/so3.hpp', line 299.
Quaternion ( 0.706662 4.32706e-17 0.707551 -4.3325e-17) should not be close to zero!
Aborted (core dumped)
在63行代码出加入return true;
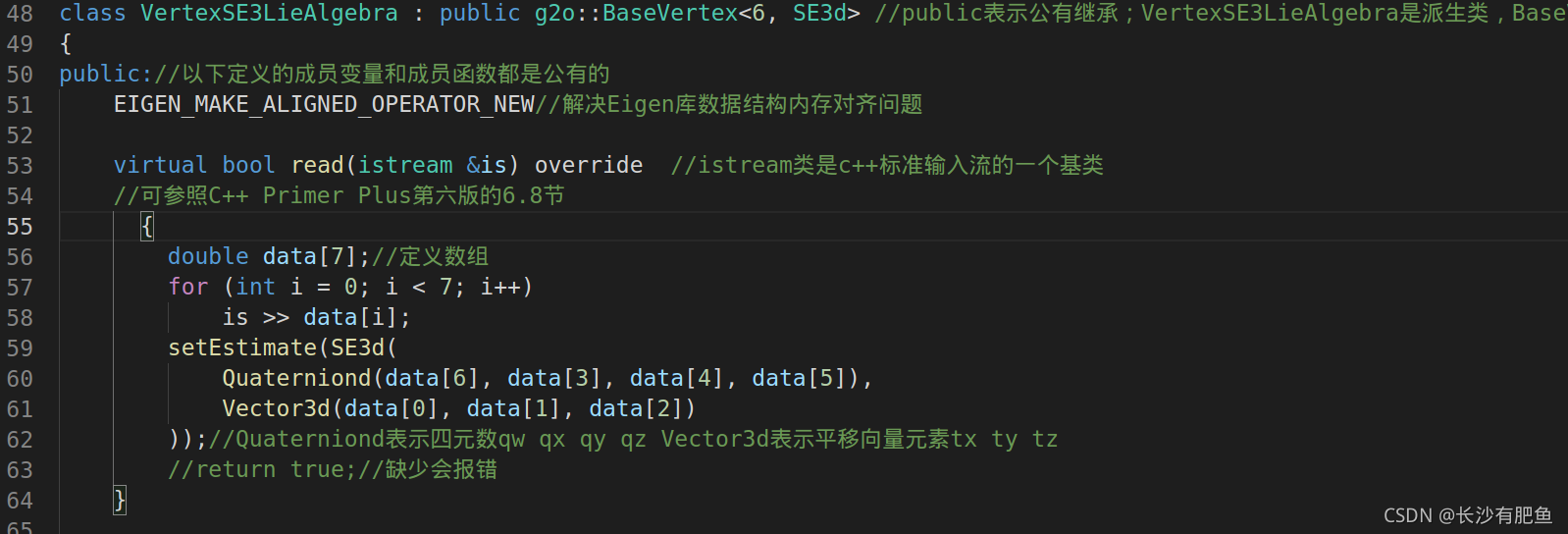
执行结果:
./pose_graph_g2o_lie ../sphere.g2o
read total 2500 vertices, 9799 edges.
optimizing ...
iteration= 0 chi2= 674837160.579970 time= 0.476435 cumTime= 0.476435 edges= 9799 schur= 0 lambda= 6658.554263 levenbergIter= 1
iteration= 1 chi2= 234706314.970484 time= 0.458582 cumTime= 0.935017 edges= 9799 schur= 0 lambda= 2219.518088 levenbergIter= 1
iteration= 2 chi2= 142146174.348537 time= 0.457854 cumTime= 1.39287 edges= 9799 schur= 0 lambda= 739.839363 levenbergIter= 1
iteration= 3 chi2= 83834595.145595 time= 0.454836 cumTime= 1.84771 edges= 9799 schur= 0 lambda= 246.613121 levenbergIter= 1
iteration= 4 chi2= 41878079.903257 time= 0.454826 cumTime= 2.30253 edges= 9799 schur= 0 lambda= 82.204374 levenbergIter= 1
iteration= 5 chi2= 16598628.119946 time= 0.455236 cumTime= 2.75777 edges= 9799 schur= 0 lambda= 27.401458 levenbergIter= 1
iteration= 6 chi2= 6137666.739405 time= 0.461072 cumTime= 3.21884 edges= 9799 schur= 0 lambda= 9.133819 levenbergIter= 1
iteration= 7 chi2= 2182986.250595 time= 0.460546 cumTime= 3.67939 edges= 9799 schur= 0 lambda= 3.044606 levenbergIter= 1
iteration= 8 chi2= 732676.668220 time= 0.459353 cumTime= 4.13874 edges= 9799 schur= 0 lambda= 1.014869 levenbergIter= 1
iteration= 9 chi2= 284457.115176 time= 0.465234 cumTime= 4.60397 edges= 9799 schur= 0 lambda= 0.338290 levenbergIter= 1
iteration= 10 chi2= 170796.109734 time= 0.474433 cumTime= 5.07841 edges= 9799 schur= 0 lambda= 0.181974 levenbergIter= 1
iteration= 11 chi2= 145466.315841 time= 0.481857 cumTime= 5.56026 edges= 9799 schur= 0 lambda= 0.060658 levenbergIter= 1
iteration= 12 chi2= 142373.179500 time= 0.506183 cumTime= 6.06645 edges= 9799 schur= 0 lambda= 0.020219 levenbergIter= 1
iteration= 13 chi2= 137485.756901 time= 0.468467 cumTime= 6.53491 edges= 9799 schur= 0 lambda= 0.006740 levenbergIter= 1
iteration= 14 chi2= 131202.175668 time= 0.463868 cumTime= 6.99878 edges= 9799 schur= 0 lambda= 0.002247 levenbergIter= 1
iteration= 15 chi2= 128006.202529 time= 0.463521 cumTime= 7.4623 edges= 9799 schur= 0 lambda= 0.000749 levenbergIter= 1
iteration= 16 chi2= 127587.860945 time= 0.467469 cumTime= 7.92977 edges= 9799 schur= 0 lambda= 0.000250 levenbergIter= 1
iteration= 17 chi2= 127578.599359 time= 0.461724 cumTime= 8.3915 edges= 9799 schur= 0 lambda= 0.000083 levenbergIter= 1
iteration= 18 chi2= 127578.573853 time= 0.459004 cumTime= 8.8505 edges= 9799 schur= 0 lambda= 0.000028 levenbergIter= 1
iteration= 19 chi2= 127578.573840 time= 0.461209 cumTime= 9.31171 edges= 9799 schur= 0 lambda= 0.000018 levenbergIter= 1
iteration= 20 chi2= 127578.573840 time= 0.4623 cumTime= 9.77401 edges= 9799 schur= 0 lambda= 0.000012 levenbergIter= 1
iteration= 21 chi2= 127578.573840 time= 0.462115 cumTime= 10.2361 edges= 9799 schur= 0 lambda= 0.000008 levenbergIter= 1
iteration= 22 chi2= 127578.573840 time= 0.463833 cumTime= 10.7 edges= 9799 schur= 0 lambda= 0.000005 levenbergIter= 1
iteration= 23 chi2= 127578.573840 time= 0.467892 cumTime= 11.1678 edges= 9799 schur= 0 lambda= 0.000004 levenbergIter= 1
iteration= 24 chi2= 127578.573840 time= 0.923353 cumTime= 12.0912 edges= 9799 schur= 0 lambda= 0.000005 levenbergIter= 2
iteration= 25 chi2= 127578.573840 time= 3.78778 cumTime= 15.879 edges= 9799 schur= 0 lambda= 871.504266 levenbergIter= 8
iteration= 26 chi2= 127578.573840 time= 3.25966 cumTime= 19.1386 edges= 9799 schur= 0 lambda= 1218451276.310652 levenbergIter= 7
iteration= 27 chi2= 127578.573840 time= 1.42473 cumTime= 20.5634 edges= 9799 schur= 0 lambda= 6498406806.990145 levenbergIter= 3
iteration= 28 chi2= 127578.573840 time= 4.88181 cumTime= 25.4452 edges= 9799 schur= 0 lambda= 234129779795701684202635264.000000 levenbergIter= 10
saving optimization results ...
g2o_viewer查看:
g2o_viewer result_lie.g2o
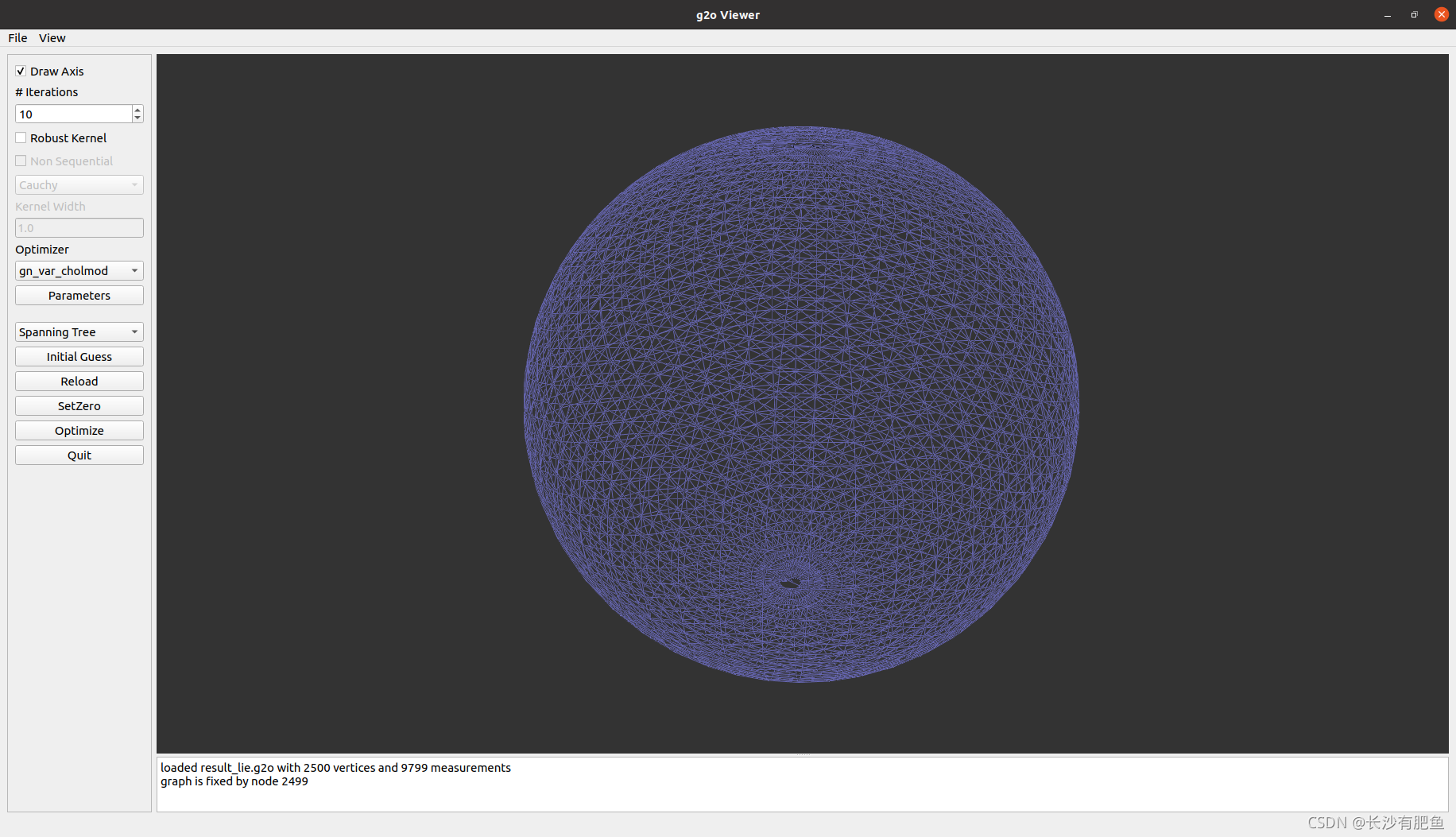
?课后习题:
1. 如果将位姿图中的误差定义为: Δ ξ i , j = ξ i ° ξ j( ? 1) ,推导按照此定义下的左乘扰动雅可比矩阵。
转载于:?视觉SLAM十四讲(第二版)第10讲习题解答 - 知乎
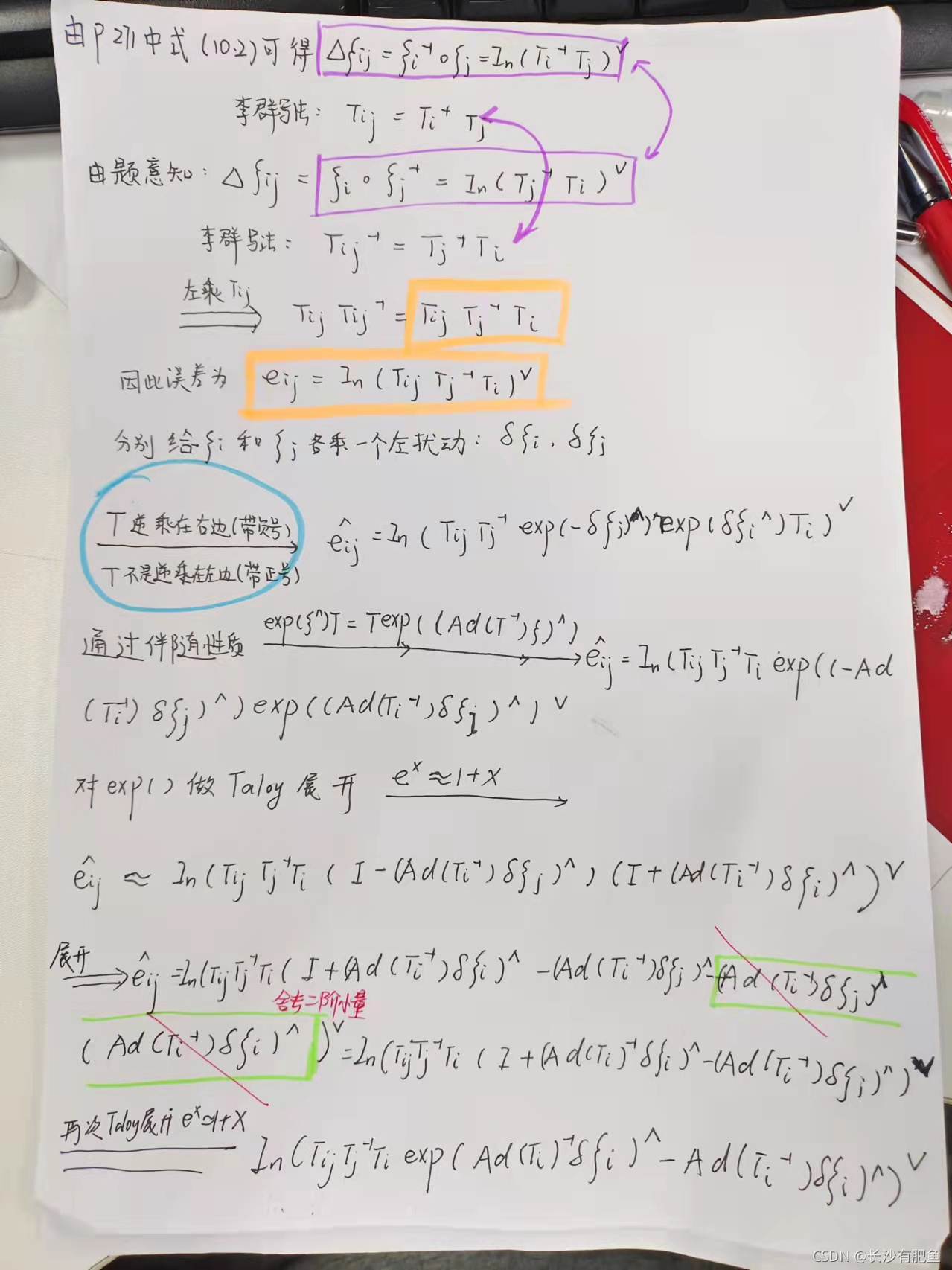
?
?
2.使用右乘更新,推导该情况下的雅克比矩阵。
转载于:视觉SLAM十四讲(第二版)第10讲习题解答 - 知乎
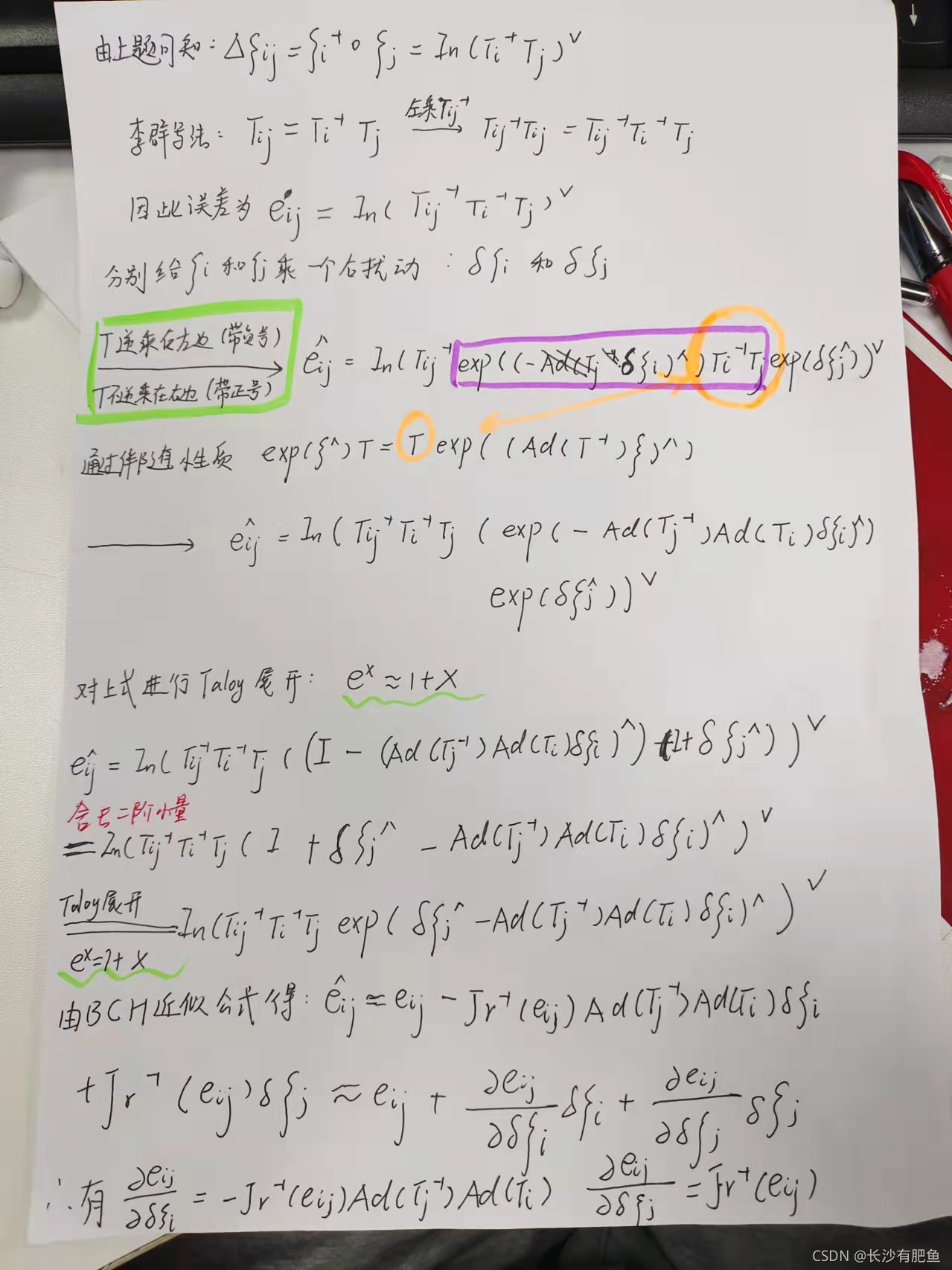 3. 参照g2o 的程序,在Ceres 中实现对“球”位姿图的优化。
转载于:视觉SLAM十四讲(第二版)第10讲习题解答 - 知乎
链接:https://pan.baidu.com/s/1Wi-XTY7Y-x_C_NPbvSpgmA? 提取码:1234
3.cpp
// Ceres Solver - A fast non-linear least squares minimizer
// Copyright 2016 Google Inc. All rights reserved.
// http://ceres-solver.org/
//
// Redistribution and use in source and binary forms, with or without
// modification, are permitted provided that the following conditions are met:
//
// * Redistributions of source code must retain the above copyright notice,
// this list of conditions and the following disclaimer.
// * Redistributions in binary form must reproduce the above copyright notice,
// this list of conditions and the following disclaimer in the documentation
// and/or other materials provided with the distribution.
// * Neither the name of Google Inc. nor the names of its contributors may be
// used to endorse or promote products derived from this software without
// specific prior written permission.
//
// THIS SOFTWARE IS PROVIDED BY THE COPYRIGHT HOLDERS AND CONTRIBUTORS "AS IS"
// AND ANY EXPRESS OR IMPLIED WARRANTIES, INCLUDING, BUT NOT LIMITED TO, THE
// IMPLIED WARRANTIES OF MERCHANTABILITY AND FITNESS FOR A PARTICULAR PURPOSE
// ARE DISCLAIMED. IN NO EVENT SHALL THE COPYRIGHT OWNER OR CONTRIBUTORS BE
// LIABLE FOR ANY DIRECT, INDIRECT, INCIDENTAL, SPECIAL, EXEMPLARY, OR
// CONSEQUENTIAL DAMAGES (INCLUDING, BUT NOT LIMITED TO, PROCUREMENT OF
// SUBSTITUTE GOODS OR SERVICES; LOSS OF USE, DATA, OR PROFITS; OR BUSINESS
// INTERRUPTION) HOWEVER CAUSED AND ON ANY THEORY OF LIABILITY, WHETHER IN
// CONTRACT, STRICT LIABILITY, OR TORT (INCLUDING NEGLIGENCE OR OTHERWISE)
// ARISING IN ANY WAY OUT OF THE USE OF THIS SOFTWARE, EVEN IF ADVISED OF THE
// POSSIBILITY OF SUCH DAMAGE.
//
// Author: vitus@google.com (Michael Vitus)
#include <iostream>
#include <fstream>
#include <string>
#include "ceres/ceres.h"
#include "common/read_g2o.h"
#include "gflags/gflags.h"
#include "glog/logging.h"
#include "common/pose_graph_3d_error_term.h"
#include "common/types.h"
DEFINE_string(input, "sphere.g2o", "The pose graph definition filename in g2o format.");
namespace ceres {
namespace examples {
// Constructs the nonlinear least squares optimization problem from the pose
// graph constraints.
void BuildOptimizationProblem(const VectorOfConstraints& constraints,
MapOfPoses* poses, ceres::Problem* problem) {
CHECK(poses != NULL);
CHECK(problem != NULL);
if (constraints.empty()) {
LOG(INFO) << "No constraints, no problem to optimize.";
return;
}
ceres::LossFunction* loss_function = NULL;
ceres::LocalParameterization* quaternion_local_parameterization =
new EigenQuaternionParameterization;
for (VectorOfConstraints::const_iterator constraints_iter =
constraints.begin();
constraints_iter != constraints.end(); ++constraints_iter) {
const Constraint3d& constraint = *constraints_iter;
MapOfPoses::iterator pose_begin_iter = poses->find(constraint.id_begin);
CHECK(pose_begin_iter != poses->end())
<< "Pose with ID: " << constraint.id_begin << " not found.";
MapOfPoses::iterator pose_end_iter = poses->find(constraint.id_end);
CHECK(pose_end_iter != poses->end())
<< "Pose with ID: " << constraint.id_end << " not found.";
const Eigen::Matrix<double, 6, 6> sqrt_information =
constraint.information.llt().matrixL();
// Ceres will take ownership of the pointer.
ceres::CostFunction* cost_function =
PoseGraph3dErrorTerm::Create(constraint.t_be, sqrt_information);
problem->AddResidualBlock(cost_function, loss_function,
pose_begin_iter->second.p.data(),
pose_begin_iter->second.q.coeffs().data(),
pose_end_iter->second.p.data(),
pose_end_iter->second.q.coeffs().data());
problem->SetParameterization(pose_begin_iter->second.q.coeffs().data(),
quaternion_local_parameterization);
problem->SetParameterization(pose_end_iter->second.q.coeffs().data(),
quaternion_local_parameterization);
}
// The pose graph optimization problem has six DOFs that are not fully
// constrained. This is typically referred to as gauge freedom. You can apply
// a rigid body transformation to all the nodes and the optimization problem
// will still have the exact same cost. The Levenberg-Marquardt algorithm has
// internal damping which mitigates this issue, but it is better to properly
// constrain the gauge freedom. This can be done by setting one of the poses
// as constant so the optimizer cannot change it.
MapOfPoses::iterator pose_start_iter = poses->begin();
CHECK(pose_start_iter != poses->end()) << "There are no poses.";
problem->SetParameterBlockConstant(pose_start_iter->second.p.data());
problem->SetParameterBlockConstant(pose_start_iter->second.q.coeffs().data());
}
// Returns true if the solve was successful.
bool SolveOptimizationProblem(ceres::Problem* problem) {
CHECK(problem != NULL);
ceres::Solver::Options options;
options.max_num_iterations = 200;
options.linear_solver_type = ceres::SPARSE_NORMAL_CHOLESKY;
options.minimizer_progress_to_stdout = true;
ceres::Solver::Summary summary;
ceres::Solve(options, problem, &summary);
std::cout << summary.FullReport() << '\n';
return summary.IsSolutionUsable();
}
// Output the poses to the file with format: id x y z q_x q_y q_z q_w.
bool OutputPoses(const std::string& filename, const MapOfPoses& poses) {
std::fstream outfile;
outfile.open(filename.c_str(), std::istream::out);
if (!outfile) {
LOG(ERROR) << "Error opening the file: " << filename;
return false;
}
for (std::map<int, Pose3d, std::less<int>,
Eigen::aligned_allocator<std::pair<const int, Pose3d> > >::
const_iterator poses_iter = poses.begin();
poses_iter != poses.end(); ++poses_iter) {
const std::map<int, Pose3d, std::less<int>,
Eigen::aligned_allocator<std::pair<const int, Pose3d> > >::
value_type& pair = *poses_iter;
outfile << pair.first << " " << pair.second.p.transpose() << " "
<< pair.second.q.x() << " " << pair.second.q.y() << " "
<< pair.second.q.z() << " " << pair.second.q.w() << '\n';
}
return true;
}
bool WriteG2oFile(const std::string& filename,
const MapOfPoses& poses,
const VectorOfConstraints& constraints){
std::ofstream outfile(filename.c_str());
if (!outfile) {
LOG(ERROR) << "Error opening the file: " << filename;
return false;
}
for (std::map<int, Pose3d, std::less<int>,
Eigen::aligned_allocator<std::pair<const int, Pose3d> > >::
const_iterator poses_iter = poses.begin();
poses_iter != poses.end(); ++poses_iter) {
const std::map<int, Pose3d, std::less<int>,
Eigen::aligned_allocator<std::pair<const int, Pose3d> > >::
value_type& pair = *poses_iter;
outfile << pair.second.name() << " " << pair.first << " "
<< pair.second.p.transpose() << " "
<< pair.second.q.x() << " " << pair.second.q.y() << " "
<< pair.second.q.z() << " " << pair.second.q.w() << '\n';
}
for (VectorOfConstraints::const_iterator constraints_iter =
constraints.begin(); constraints_iter != constraints.end();
++constraints_iter){
const Constraint3d& constraint = *constraints_iter;
Pose3d pose_r = constraint.t_be;
outfile << constraint.name() << " " << constraint.id_begin
<< " " << constraint.id_end << " "
<< pose_r.p.transpose() << " "
<< pose_r.q.x() << " " << pose_r.q.y() << " "
<< pose_r.q.z() << " " << pose_r.q.w() << " ";
const Eigen::Matrix<double, 6, 6> sqrt_information =
constraint.information.llt().matrixL();
for (size_t i = 0 ; i < 6 ; ++i ){
for (size_t j = i ; j < 6 ; ++j){
outfile << sqrt_information(i,j) << " ";
}
}
outfile << "\n";
}
//outfile.close();
return true;
}
} // namespace examples
} // namespace ceres
int main(int argc, char** argv) {
google::InitGoogleLogging(argv[0]);
gflags::ParseCommandLineFlags(&argc, &argv, true);
CHECK(FLAGS_input != "") << "Need to specify the filename to read.";
ceres::examples::MapOfPoses poses;
ceres::examples::VectorOfConstraints constraints;
CHECK(ceres::examples::ReadG2oFile(FLAGS_input, &poses, &constraints))
<< "Error reading the file: " << FLAGS_input;
std::cout << "Number of poses: " << poses.size() << '\n';
std::cout << "Number of constraints: " << constraints.size() << '\n';
// CHECK(ceres::examples::OutputPoses("poses_original.txt", poses))
// << "Error outputting to poses_original.txt";
ceres::Problem problem;
ceres::examples::BuildOptimizationProblem(constraints, &poses, &problem);
CHECK(ceres::examples::SolveOptimizationProblem(&problem))
<< "The solve was not successful, exiting.";
// CHECK(ceres::examples::OutputPoses("poses_optimized.txt", poses))
// << "Error outputting to poses_original.txt";
CHECK(ceres::examples::WriteG2oFile("result_Ceres.g2o", poses, constraints))
<< "Error outputting to result_Ceres.g2o";
return 0;
}
CMakeLists.txt
cmake_minimum_required(VERSION 2.8)
project(pose_graph)
set(CMAKE_BUILD_TYPE "Release")
set(CMAKE_CXX_FLAGS "-std=c++14 -O3")
list(APPEND CMAKE_MODULE_PATH ${PROJECT_SOURCE_DIR}/cmake_modules)
# Eigen
include_directories("/usr/include/eigen3")
# sophus
find_package(Sophus REQUIRED)
include_directories(${Sophus_INCLUDE_DIRS})
find_package(Ceres REQUIRED)
include_directories(${CERES_INCLUDE_DIRS})
find_package(Gflags REQUIRED)
include_directories(${GFLAGS_INCLUDE_DIRS})
add_executable(pose_graph_3d 3.cpp)
target_link_libraries(pose_graph_3d ceres ${GFLAGS_LIBRARIES})
# add_executable(test test.cpp)
# target_link_libraries(test
# ${CERES_LIBRARIES}
# ${CHOLMOD_LIBRARIES}
# ${Sophus_LIBRARIES}
# )
error解决:
F1129 21:08:44.134934 13817 3.cpp:208] Check failed: ceres::examples::ReadG2oFile(FLAGS_input, &poses, &constraints) Error reading the file: sphere.g2o
*** Check failure stack trace: ***
@ 0x7fb2007dd1c3 google::LogMessage::Fail()
@ 0x7fb2007e225b google::LogMessage::SendToLog()
@ 0x7fb2007dcebf google::LogMessage::Flush()
@ 0x7fb2007dd6ef google::LogMessageFatal::~LogMessageFatal()
@ 0x55803e6f93db main
@ 0x7fb1fe1700b3 __libc_start_main
@ 0x55803e6f972e _start
Aborted (core dumped)
将sphere.g2o 放到build文件夹下面。
执行结果:
./pose_graph_3d
Number of poses: 2500
Number of constraints: 9799
iter cost cost_change |gradient| |step| tr_ratio tr_radius ls_iter iter_time total_time
0 4.772379e+09 0.00e+00 1.58e+06 0.00e+00 0.00e+00 1.00e+04 0 2.36e-02 3.33e-02
1 2.262672e+08 4.55e+09 3.60e+05 9.61e+02 9.53e-01 3.00e+04 1 2.58e-01 2.91e-01
2 1.270530e+06 2.25e+08 2.36e+04 3.42e+02 9.95e-01 9.00e+04 1 1.60e+00 1.89e+00
3 7.829917e+04 1.19e+06 1.16e+03 1.02e+02 1.00e+00 2.70e+05 1 1.98e+00 3.87e+00
4 7.441786e+04 3.88e+03 2.80e+02 6.98e+01 1.00e+00 8.10e+05 1 6.23e-01 4.49e+00
5 7.364500e+04 7.73e+02 3.72e+01 7.94e+01 9.71e-01 2.43e+06 1 1.35e+00 5.84e+00
6 7.299000e+04 6.55e+02 1.06e+02 2.08e+02 4.40e-01 2.43e+06 1 9.08e-01 6.75e+00
7 7.144054e+04 1.55e+03 8.08e+01 1.90e+02 7.58e-01 2.81e+06 1 3.21e-01 7.07e+00
8 7.034563e+04 1.09e+03 8.70e+01 2.00e+02 6.60e-01 2.91e+06 1 2.42e+00 9.49e+00
9 6.921258e+04 1.13e+03 7.66e+01 1.88e+02 7.26e-01 3.20e+06 1 1.30e+00 1.08e+01
10 6.829885e+04 9.14e+02 7.58e+01 1.88e+02 6.87e-01 3.38e+06 1 2.48e+00 1.33e+01
11 6.744817e+04 8.51e+02 6.86e+01 1.78e+02 7.16e-01 3.67e+06 1 7.07e-01 1.40e+01
12 6.673782e+04 7.10e+02 6.49e+01 1.73e+02 7.03e-01 3.94e+06 1 7.00e-01 1.47e+01
13 6.611361e+04 6.24e+02 5.87e+01 1.64e+02 7.19e-01 4.30e+06 1 7.58e-01 1.54e+01
14 6.559464e+04 5.19e+02 5.38e+01 1.57e+02 7.17e-01 4.68e+06 1 2.98e+00 1.84e+01
15 6.515719e+04 4.37e+02 4.81e+01 1.49e+02 7.28e-01 5.17e+06 1 3.68e-01 1.88e+01
16 6.480192e+04 3.55e+02 4.30e+01 1.41e+02 7.31e-01 5.74e+06 1 6.70e-01 1.94e+01
17 6.451483e+04 2.87e+02 3.76e+01 1.31e+02 7.42e-01 6.47e+06 1 9.80e-01 2.04e+01
18 6.429085e+04 2.24e+02 3.26e+01 1.22e+02 7.49e-01 7.38e+06 1 1.91e+00 2.23e+01
19 6.411960e+04 1.71e+02 2.75e+01 1.13e+02 7.61e-01 8.60e+06 1 4.05e+00 2.64e+01
20 6.399434e+04 1.25e+02 2.28e+01 1.03e+02 7.72e-01 1.02e+07 1 1.61e+00 2.80e+01
21 6.390644e+04 8.79e+01 1.82e+01 9.17e+01 7.88e-01 1.27e+07 1 1.80e+00 2.98e+01
22 6.384895e+04 5.75e+01 1.40e+01 8.03e+01 8.05e-01 1.64e+07 1 1.37e+00 3.12e+01
23 6.381446e+04 3.45e+01 9.93e+00 6.78e+01 8.30e-01 2.30e+07 1 2.78e+00 3.39e+01
24 6.379654e+04 1.79e+01 6.39e+00 5.45e+01 8.59e-01 3.65e+07 1 6.49e-01 3.46e+01
25 6.378909e+04 7.44e+00 3.37e+00 3.96e+01 9.01e-01 7.51e+07 1 3.93e+00 3.85e+01
26 6.378709e+04 2.01e+00 2.00e+00 2.37e+01 9.50e-01 2.25e+08 1 2.59e+00 4.11e+01
27 6.378687e+04 2.22e-01 1.97e+00 8.56e+00 9.92e-01 6.76e+08 1 1.66e+00 4.28e+01
Solver Summary (v 2.0.0-eigen-(3.4.0)-lapack-suitesparse-(5.7.1)-cxsparse-(3.2.0)-eigensparse-no_openmp)
Original Reduced
Parameter blocks 5000 4998
Parameters 17500 17493
Effective parameters 15000 14994
Residual blocks 9799 9799
Residuals 58794 58794
Minimizer TRUST_REGION
Sparse linear algebra library SUITE_SPARSE
Trust region strategy LEVENBERG_MARQUARDT
Given Used
Linear solver SPARSE_NORMAL_CHOLESKY SPARSE_NORMAL_CHOLESKY
Threads 1 1
Linear solver ordering AUTOMATIC 4998
Cost:
Initial 4.772379e+09
Final 6.378687e+04
Change 4.772315e+09
Minimizer iterations 28
Successful steps 28
Unsuccessful steps 0
Time (in seconds):
Preprocessor 0.009657
Residual only evaluation 0.046179 (28)
Jacobian & residual evaluation 0.777356 (28)
Linear solver 42.494283 (28)
Minimizer 43.423383
Postprocessor 0.000665
Total 43.433705
Termination: CONVERGENCE (Function tolerance reached. |cost_change|/cost: 5.519336e-08 <= 1.000000e-06)
g2o_viewer查看:
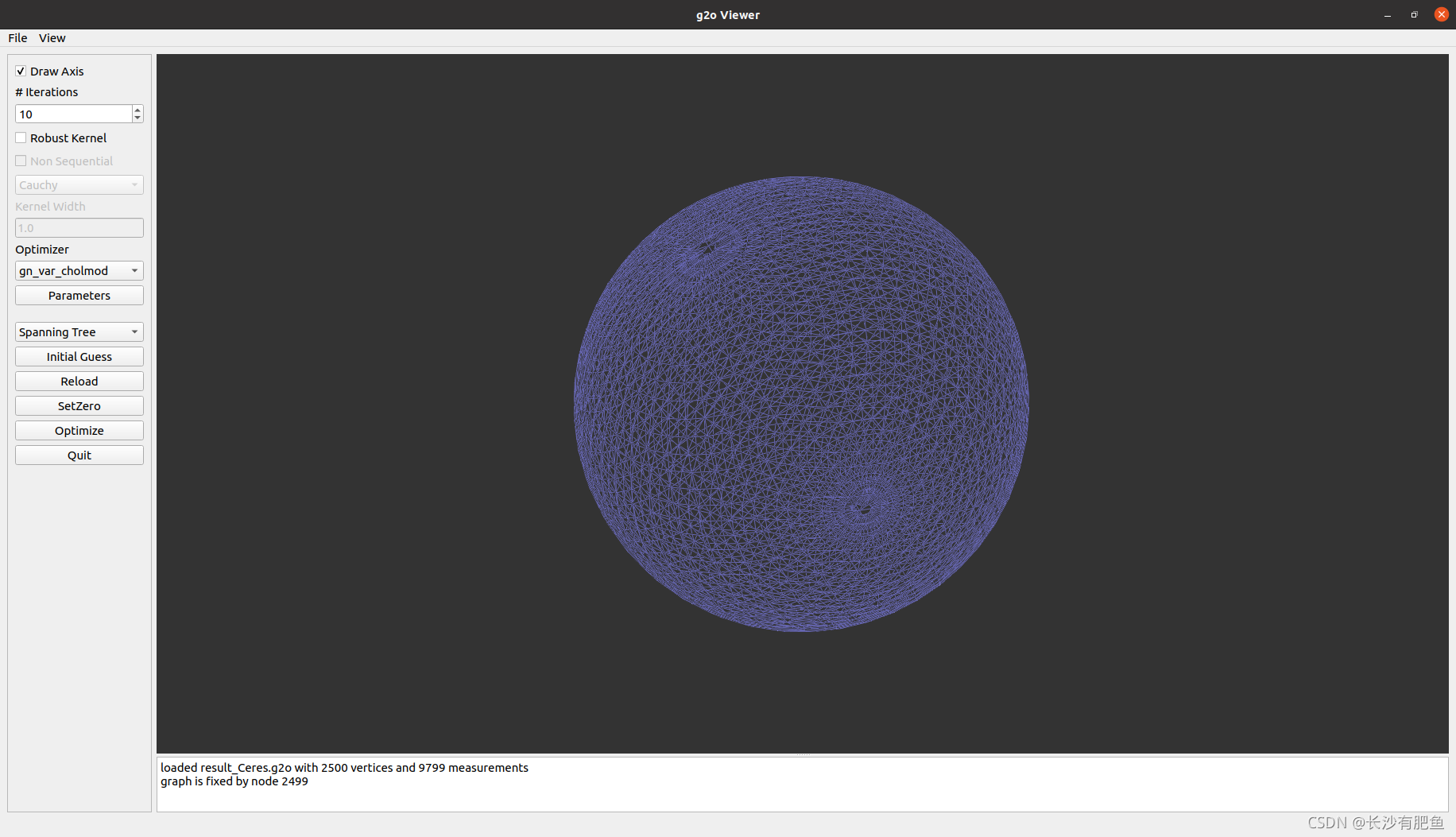 4. 对“球”中的信息按照时间排序,分别喂给g2o 和gtsam 优化,比较它们的性能差异。
可参考这篇文章:论文精读 | slam中姿态估计的图优化方法比较 - 知乎
5. *阅读iSAM相关论文,理解它是如何实现增量式优化的。
?**********
|