目录
一、前言
二、朴素贝叶斯原理
1.贝叶斯公式:
2.判别模型和生成模型
3.朴素贝叶斯分类器
?4.拉普拉斯修正
5.防溢出策略
6.测试朴素贝叶斯分类器?
6.1构建词向量
6.2 朴素贝叶斯分类训练函数
6.3分类函数
6.4测试函数
三、朴素贝叶斯分类垃圾邮件
1.数据集
?2.代码展示
?3.运行结果
一、前言
对于分类问题,其实谁都不会陌生,日常生活中我们每天都进行着分类过程。例如,当你看到一个人,你的脑子下意识判断他是学生还是社会上的人;你可能经常会走在路上对身旁的朋友说“这个人一看就很有钱、”之类的话,其实这就是一种分类操作。
既然是贝叶斯分类算法,那么分类的数学描述又是什么呢?
从数学角度来说,分类问题可做如下定义:已知集合 和 ,确定映射规则y = f(),使得任意 有且仅有一个 ,使得 成立。
?
其中C叫做类别集合,其中每一个元素是一个类别,而I叫做项集合(特征集合),其中每一个元素是一个待分类项,f叫做分类器。分类算法的任务就是构造分类器f。
二、朴素贝叶斯原理
1.贝叶斯公式:
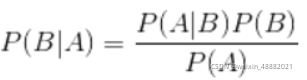
换个表达形式就会明朗很多,如下:?

2.判别模型和生成模型
?判别模型:由数据直接学习决策函数Y=f(X)或者条件概率分布P(Y|X)作为预测的模型,即判别模型。基本思想是有限样本条件下建立判别函数,不考虑样本的产生模型,直接研究预测模型。典型的判别模型包括k近邻,感知级,决策树,支持向量机等。
生成模型:由数据学习联合概率密度分布P(X,Y),然后求出条件概率分布P(Y|X)作为预测的模型,即生成模型:P(Y|X)= P(X,Y)/ P(X)。基本思想是首先建立样本的联合概率概率密度模型P(X,Y),然后再得到后验概率P(Y|X),再利用它进行分类,就像上面说的那样。注意了哦,这里是先求出P(X,Y)才得到P(Y|X)的,然后这个过程还得先求出P(X)。P(X)就是你的训练数据的概率分布。
3.朴素贝叶斯分类器
朴素贝叶斯分类器(Na?ve Bayes Classifier)采用了“属性条件独立性假设”,即每个属性独立地对分类结果发生影响。
为方便公式标记,不妨记
P
(
C
=
c
|
X
=x)
为
P
(
c
|x)
,基于属性条件独立性假设,贝叶斯公式可重写为
?其中d为属性数目,xi为 x 在第i个属性上的取值。
朴素贝叶斯分类器的训练器的训练过程就是基于训练集
D
估计类先验概率
P
(
c
),并为每个属性估计条件概率
。 令
表示训练集D
中第
c
类样本组合的集合,则类先验概率:
?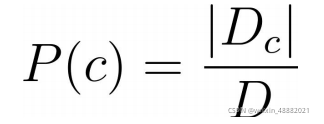

??????????????????????????????????????????? ?
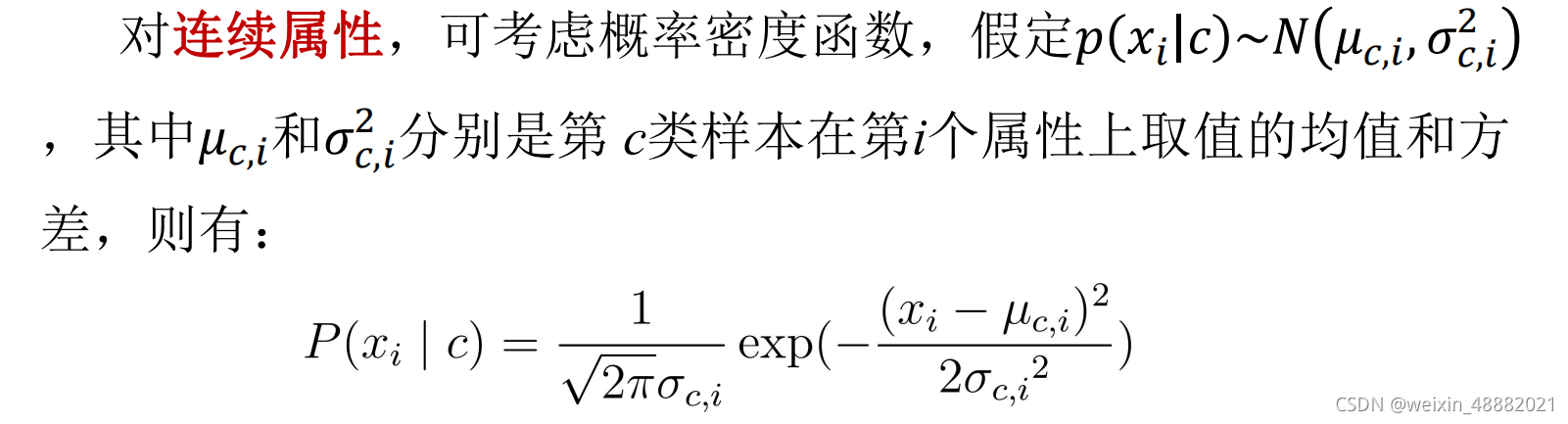
?4.拉普拉斯修正
若某个属性值在训练集中没有与某个类同时出现过,则训练后的模型会出现
over-fitting
现象。比如“敲声
=
清脆”测试例,训练
集中没有该样例,因此连乘式计算的概率值为
0
,无论其他属性上
明显像好瓜,分类结果都是“好瓜
=
否”,这显然不合理。
?
为了避免其他属性携带的信息,被训练集中未出现的属性值“抹去”,在估计概率值时通常要进行“拉普拉斯修正”:
令
N
表示训练集
D
中可能的类别数,Ni
表示第
i
个属性可能的
取值数,则贝叶斯公式可修正为:
?
5.防溢出策略
在条件概率乘法计算过程中,因子一般较小(均是小于的实数)。当属性数量增多时,会导致累乘结果下溢出现象。在代数中有ln(a*b) = ln(a)+ln(b),因此可以把条件概率累乘转换成对数累加。分类结果仅需对比概率的对数假发运算后的值,以确定划分的类别。
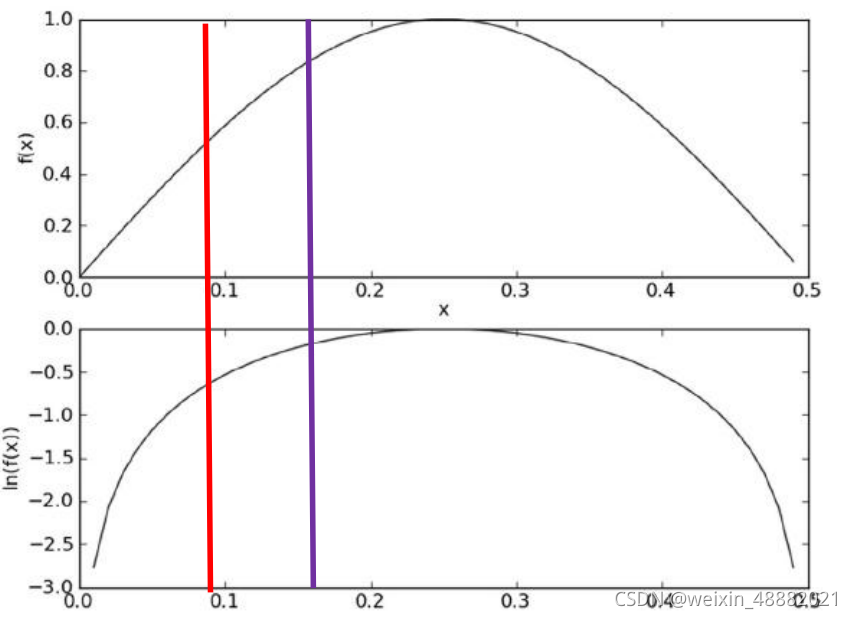
6.测试朴素贝叶斯分类器?
利用25封侮辱性邮件和25封非侮辱性文件对朴素贝叶斯分类器进行测试,其中49封作为训练数据,随机抽取10个作为测试集。
6.1构建词向量
'''
函数说明:创建实验样本
:return: 进行词条切分后的文档集合;类别标签的集合(侮辱性和非侮辱性)
'''
def loadDataSet():
postingList=[['my', 'dog', 'has', 'flea', 'problems', 'help', 'please'],
['maybe', 'not', 'take', 'him', 'to', 'dog', 'park', 'stupid'],
['my', 'dalmation', 'is', 'so', 'cute', 'I', 'love', 'him'],
['stop', 'posting', 'stupid', 'worthless', 'garbage'],
['mr', 'licks', 'ate', 'my', 'steak', 'how', 'to', 'stop', 'him'],
['quit', 'buying', 'worthless', 'dog', 'food', 'stupid']]
classVec = [0,1,0,1,0,1] #1表示侮辱性文字,0代表正常言论
return postingList,classVec
#创建一个包含在所有文档中出现的不重复词的列表
def createVocabList(dataSet):
vocabSet = set([]) #创建空的集合
for document in dataSet:
vocabSet = vocabSet | set(document) #求两个集合的并集
return list(vocabSet)
#根据vocabList词汇表,将每个inputSet词条向量化,向量的每个值为1或0,分别表示该词有或者没有出现在词汇表中
#输入变量:词汇表,某个文档
def setOfWords2Vec(vocabList, inputSet):
#创建一个其中所含元素都为0的向量
returnVec = [0]*len(vocabList)
for word in inputSet:
if word in vocabList:
returnVec[vocabList.index(word)] = 1
else:
print("the word: %s is not in my Vocabulary!" % word)
return returnVec
#朴素贝叶斯词袋模型
def bagOfWords2VecMN(vocabList, inputSet):
returnVec = [0]*len(vocabList)
for word in inputSet:
if word in vocabList:
#每个词在词袋中可以出现多次。出现则累加
returnVec[vocabList.index(word)] += 1
return returnVec
6.2 朴素贝叶斯分类训练函数
#朴素贝叶斯分类器训练函数
'''
函数说明:朴素贝叶斯分类器训练函数
:param trainMatrix: 文档矩阵
:param trainCategory: 文档类别标签向量
:return: 非侮辱类的条件概率数组,侮辱类的条件概率数组,文档属于侮辱类的概率
'''
def trainNB0(trainMatrix,trainCategory):
numTrainDocs = len(trainMatrix) #训练集的数量,如6个元素
#print("数量为:",numTrainDocs)
numWords = len(trainMatrix[0]) #每个词条向量的长度,如每一个都是32维
#print("长度为:", numWords)
#sum(trainCategory)表示将标签向量中的(0,1)相加,即得到1的个数(也就是侮辱性文档数目)
#标签中“1”表示侮辱,“0”表示非侮辱,所以是统计文档属于侮辱类的概率
pAbusive = sum(trainCategory)/float(numTrainDocs)
#zeros()创建的数组,其元素值均为0
#p0Num = zeros(numWords)
#p1Num = zeros(numWords)
#p0Denom = 0.0
#p1Denom = 0.0
#ones()函数可以创建任意维度和元素个数的数组,其元素值均为1
#创建numpy.ones数组,词条出现数初始化为1,拉普拉斯平滑方法(为了防止与0相乘)
p0Num = ones(numWords)
p1Num = ones(numWords)
#分母初始化为2,拉普拉斯平滑方法
p0Denom = 2.0
p1Denom = 2.0
for i in range(numTrainDocs):
if trainCategory[i] ==1:
#统计属于侮辱类的条件概率所需的数据,即P(w0/1),P(w1/1)......
p1Num += trainMatrix[i] #数组相加
#print("p1Num:",p1Num)
p1Denom += sum(trainMatrix[i]) #sum():将trainMatrix[i]中所有元素相加
#print("p1Denom:",p1Denom)
else:
#统计属于非侮辱类的条件概率所需的数据,即P(w0/0),P(w1/0)......
p0Num += trainMatrix[i]
p0Denom +=sum(trainMatrix[i])
#print("p0Denom:",p0Denom)
p1Vect = log(p1Num/p1Denom) #p1Num中的每一项取对数
p0Vect = log(p0Num/p0Denom) #非侮辱性邮件中单词出现的概率
return p0Vect,p1Vect,pAbusive
6.3分类函数
#朴素贝叶斯分类函数
'''
函数说明:朴素贝叶斯分类函数
:param vec2Classify: 要分类的向量
:param p0Vec: 非侮辱类的条件概率数组
:param p1Vec: 侮辱类的条件概率数组
:param pClass1: 文档属于侮辱类的概率
:return: 0->表示非侮辱类文档;1->表示侮辱类文档
'''
def classifyNB(vec2Classify,p0Vec,p1Vec,pClass1):
#两个向量对应元素相乘,然后求和
p1 = sum(vec2Classify * p1Vec) +log(pClass1)
p0 = sum(vec2Classify * p0Vec) +log(1-pClass1)
if p1>p0:
return 1
else:
return 0
6.4测试函数
#利用单条数据测试
def testingNB():
listOPosts,listClasses = loadDataSet()
# 创建一个包含在所有文档中出现的不重复词的列表
myVocabList = createVocabList(listOPosts)
trainMat=[]
for postinDoc in listOPosts:
trainMat.append(setOfWords2Vec(myVocabList,postinDoc))
p0V,p1V,pAb = trainNB0(array(trainMat),array(listClasses))
testEntry=['love','my','dalmation']
thisDoc = array(setOfWords2Vec(myVocabList,testEntry))
print(testEntry,'分类结果为:',classifyNB(thisDoc,p0V,p1V,pAb))
testEntry = ['stupid','garbage']
thisDoc = array(setOfWords2Vec(myVocabList, testEntry))
print(testEntry, '分类结果为:', classifyNB(thisDoc, p0V, p1V, pAb))
#文件解析函数
def textParse(bigString): #input is big string, #output is word list
import re #正则表达式工具
#分割数据,其分隔符是除单词、数字外任意的字符串
listOfTokens = re.split(r'\W*', bigString)
#单词全部转小写,过滤没用的短字符串
return [tok.lower() for tok in listOfTokens if len(tok) > 2]
#垃圾邮件测试函数
def spamTest():
docList = [] #存放每个邮件的单词向量
classList = [] #存放邮件对应的标签
fullText = []
for i in range(1, 26):
#读取侮辱类(spam中存储)邮件,并生成单词向量
wordList = textParse(open('./email/spam/%d.txt' % i).read())
docList.append(wordList) #将单词向量存放到docList中
fullText.extend(wordList)
classList.append(1) #存放对应的类标签,侮辱类为1
# 读取非侮辱类(ham中存储)邮件,并生成单词向量
wordList = textParse(open('./email/ham/%d.txt' % i).read())
docList.append(wordList) #将单词向量存放到docList中
fullText.extend(wordList)
classList.append(0) #存放对应的类标签,非侮辱类为0
#由所有的单词向量生成词库
# xx = len(docList)
# yy = list(range(xx))
# print(xx,yy)
vocabList = createVocabList(docList)
trainSet = list(range(50)) #产生0-49的50个数字
testIndex = [] #存放测试数据的下标
for i in range(10):
#从0-49之间随机生成一个下标
randIndex = int(random.uniform(0, len(trainSet)))
testIndex.append(trainSet[randIndex]) #提取对应的数据作为测试数据
del(trainSet[randIndex]) #删除对应的数据,避免下次再选中
trainDataSet = [] #存放训练数据(用于词集方法)
trainClasses = [] #存放训练数据标签(用于词集方法)
trainDataSet1 = [] #存放训练数据(用于词袋方法)
trainClasses1 = [] #存放训练数据标签(用于词袋方法)
for docIndex in trainSet:
#提取训练数据(词集方法)
trainDataSet.append(setOfWords2Vec(vocabList, docList[docIndex]))
#提取训练数据标签
trainClasses.append(classList[docIndex])
#提取训练数据(词袋方法)
trainDataSet1.append(bagOfWords2VecMN(vocabList, docList[docIndex]))
trainClasses1.append(classList[docIndex])
#开始训练
p0V, p1V, pSpam = trainNB0(array(trainDataSet), array(trainClasses))
errorCount = 0 #统计测试时分类错误的数据个数
p0V_1, p1V_1, pSpam1 = trainNB0(array(trainDataSet1), array(trainClasses1))
errorCount1 = 0
#开始测试分类器
for Index in testIndex: # classify the remaining items
#print("classification:", Index)
wordVector = setOfWords2Vec(vocabList, docList[Index]) #数据预处理
# 测试分类器,如果分类不正确,错误个数加1
if classifyNB(array(wordVector), p0V, p1V, pSpam) != classList[Index]:
errorCount += 1
wordVector1 = bagOfWords2VecMN(vocabList, docList[Index]) #数据预处理
if classifyNB(array(wordVector1), p0V_1, p1V_1, pSpam1) != classList[Index]:
errorCount1 += 1
#输出分类错误率
print('词集方法(set)的错误率: ', float(errorCount) / len(testIndex))
print('词库方法(bag)的错误率: ', float(errorCount1) / len(testIndex))
测试结果:

?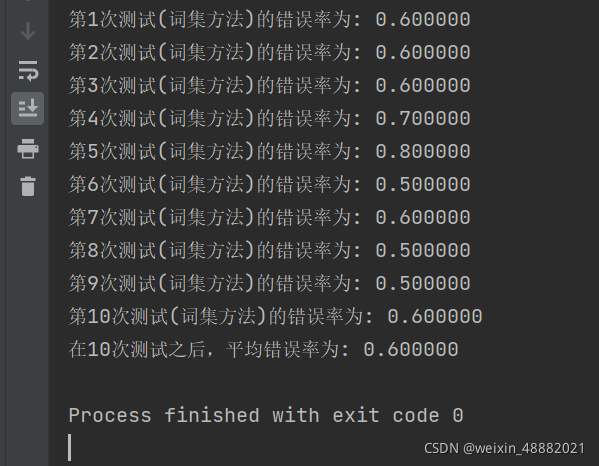
?可见错误率为百分之60
三、朴素贝叶斯分类垃圾邮件
1.数据集
数据集包括两部分,训练数据ham.data(1523条)和spam.data(1232条),测试数据ham.data(250条)和spam.data(501条)。
数据集从github中下载,想要下载的朋友可以点击下面这个链接
update gitignore, provide 50 training and test emails in spam and ham?
部分数据展示:
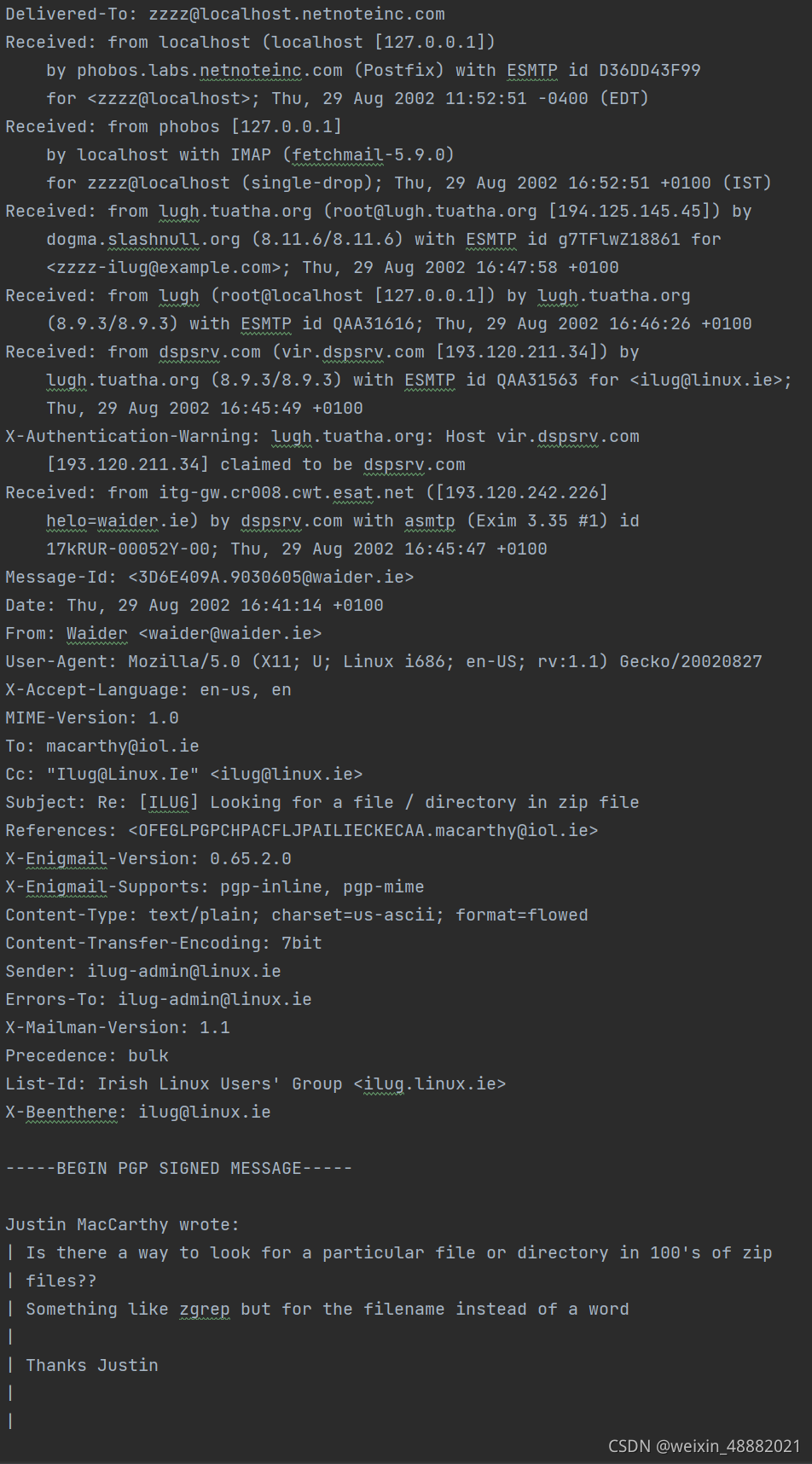
?2.代码展示
import math
import os
import re
from collections import Counter
class Spamfilter:
"""A naive Bayesian spam filter"""
def __init__(self, training_dir):
""" inits Spamfilter with training data
:param training_dir: path of training directory with subdirectories
'/ham' and '/spam'
"""
print("Training filter with known ham ...")
self.ham_table = dict(Counter(dir_tokens(training_dir + "ham/")))
print("Training filter with known spam...")
self.spam_table = dict(Counter(dir_tokens(training_dir + "spam/")))
self.uniq_h_toks = len(self.ham_table)
self.uniq_s_toks = len(self.spam_table)
self.total_h_toks = sum(self.ham_table.values())
self.total_s_toks = sum(self.spam_table.values())
self.tok_arr = sorted(
list(self.ham_table.keys()) + list(self.spam_table.keys())
)
self.freq_tab = self.create_frequency_table()
self.file_count = 0
self.count_spam = 0
self.count_ham = 0
self.spam_list = []
self.ham_list = []
def create_frequency_table(self):
""" Generates token frequency table from training emails
:return: dict{k,v}: spam/ham frequencies
k = (str)token, v = {spam_freq: , ham_freq:, prob_spam:, prob_ham:}
"""
freq_table = {}
for tok in self.tok_arr:
entry = {}
s_freq = self.spam_table.get(tok, 0)
entry["spam_freq"] = s_freq
h_freq = self.ham_table.get(tok, 0)
entry["ham_freq"] = h_freq
s_prob = (s_freq + 1 / float(self.uniq_s_toks)) / (self.total_s_toks + 1)
entry["prob_spam"] = s_prob
h_prob = (h_freq + 1 / float(self.uniq_h_toks)) / (self.total_h_toks + 1)
entry["prob_ham"] = h_prob
freq_table[tok] = entry
return freq_table
def prob_spam(self, token):
"""calculates the probability that 'token' is found in spam emails
:param token: (str)
:return: (float) probability 'token' is spam based on training emails
"""
val = self.freq_tab.get(token)
if val is not None:
return val["prob_spam"]
return (1.0 / self.uniq_s_toks) / (self.total_s_toks + 1)
def prob_ham(self, token):
"""calculates the probability that 'token' is found in ham emails
:param token: (str)
:return: (float) probability 'token' is ham based on training emails
"""
val = self.freq_tab.get(token)
if val is not None:
return val["prob_ham"]
return (1.0 / self.uniq_h_toks) / (self.total_h_toks + 1)
def prob_msg_spam(self, filepath):
"""Calculates the probability that a message is spam
:param filepath: (str) path of email
:return: (float) probability message is spam
"""
toks = file_tokens(filepath)
sm = 0
for tok in toks:
sm += math.log10(self.prob_spam(tok))
return sm
def prob_msg_ham(self, filepath):
"""Calculates the probability that a message is ham
:param filepath: (str) path of email
:return: (float) probability message is ham
"""
toks = file_tokens(filepath)
sm = 0
for tok in toks:
sm += math.log10(self.prob_ham(tok))
return sm
def classify(self, filepath):
"""classifies a file as spam or ham based on training data
:param filepath:
:return: (boolean) True->spam, False->ham
"""
self.file_count += 1
if self.prob_msg_spam(filepath) > self.prob_msg_ham(filepath):
self.count_spam += 1
self.spam_list.append(filepath)
return True
else:
self.count_ham += 1
self.ham_list.append(filepath)
return False
def classify_all(self, dir_path, known_type="spam"):
"""Classifies all emails in a testing directory and maintains count of errors
:param dir_path: path of testing directory
:param known_type: str: the known type of testing directory
"""
self.ham_list = []
self.spam_list = []
self.file_count = 0
self.count_spam = 0
self.count_ham = 0
print("\nClassifying all emails found in directory: ./" + dir_path)
try:
for f in os.listdir(dir_path):
self.classify(dir_path + f)
if known_type == "spam":
correct = self.count_spam / float(self.file_count)
else:
correct = self.count_ham / float(self.file_count)
print("Total spam:{:8d}".format(self.count_spam))
print("Total ham: {:8d}".format(self.count_ham))
print("Correctly classified: {:6.2f}%".format(correct * 100))
except FileNotFoundError as e:
print("ERROR: classify_all() failed " + str(e))
def clean_table(self, min_freq):
"""Removes entries from frequency table if they are deemed poor indicators.
or if combined spam/ham frequency is below 'min_freq'
:param min_freq: if total token count below threshold, delete from table
"""
rm_keys = []
for k, v in self.freq_tab.items():
if (
v["spam_freq"] + v["ham_freq"] < min_freq
or 0.45 < (v["prob_spam"] / (v["prob_spam"] + v["prob_ham"])) < 0.55
):
rm_keys.append(k)
for k in rm_keys:
print("deleting " + str(k) + " from freq table in clean()")
del self.freq_tab[k]
def print_table_info(self):
""" Print training info:
- unique tokens in ham and spam, number of emails in training set"""
print("\n=======================================")
print("TRAINING AND FREQUENCY TABLE INFO")
print("=======================================")
print("Unique tokens in spam messages:{:8d}".format(len(self.spam_table)))
print("Unique tokens in ham messages: {:8d}".format(len(self.ham_table)))
print("Unique tokens in ALL messages: {:8d}".format(len(self.freq_tab)))
print("Num spam e-mails:{:22d}".format(len(os.listdir("emails/testing/spam/"))))
print("Num ham e-mails: {:22d}".format(len(os.listdir("emails/testing/ham/"))))
def tokens(text, tok_size=3):
""" Returns a list of all substrings contained in 'text' of size 'tok_size'
:param text: (string) text to tokenize
:param tok_size: length of substrings
:return: (list) tokens of 'text'
"""
return [text[i : i + tok_size] for i in range(len(text) - tok_size + 1)]
def clean_split(in_str):
""" Removes all non-alphanum chars and splits string at whitespace, downcase
:param in_str: (str) target string
:return: (list) cleaned strings
"""
return re.sub(r"[^\s\w]|_", "", in_str).lower().split()
def file_tokens(filepath):
""" tokenizes all strings contained in 'filepath' after removing \
all non-alphanum chars and splitting strings at whitespace
:param filepath: path of target file
:return: list of tokens
"""
toks = []
try:
with open(filepath, encoding="utf8", errors="ignore") as fp:
for line in fp:
words = clean_split(line)
toks.extend(words)
except FileNotFoundError as e:
print("Error:" + str(e))
return [x for x in toks if len(x) < 10]
def dir_tokens(dir_path):
""" tokenizes all files contained in 'dir_path'
:param dir_path: directory containing files to be tokenized
:return: list of tokens
"""
dir_toks = []
try:
filenames = os.listdir(dir_path)
for f in filenames:
dir_toks.extend(file_tokens(dir_path + f))
except FileNotFoundError as e:
print("Error:" + str(e))
return dir_toks
if __name__ == "__main__":
spamfilter = Spamfilter("emails/training/")
spamfilter.print_table_info()
spamfilter.classify_all("emails/testing/spam/", "spam")
spamfilter.classify_all("emails/testing/ham/", "ham")
?3.运行结果

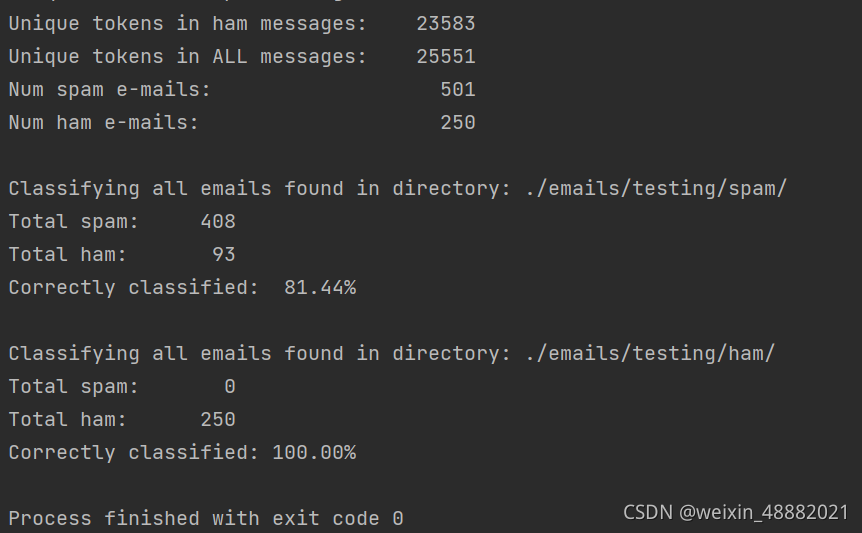
|