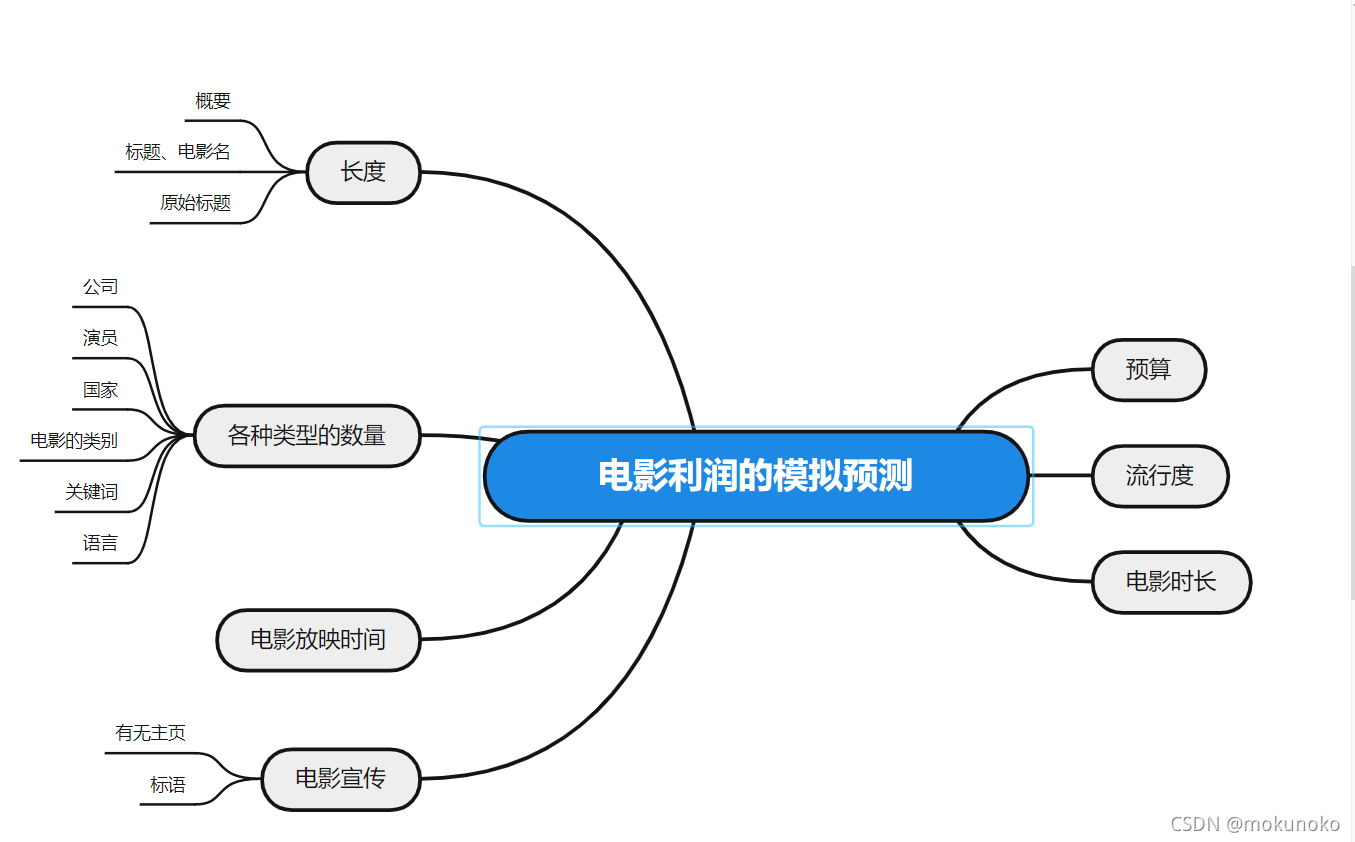
本次实验的涉及要素较多主要分为以上几个比较重要的要素,主要运用到一下模块:
import pandas as pd
import numpy as np
from matplotlib import pyplot as plt
import seaborn as sns
import random
from plotly import tools
import plotly.express as px
from plotly.offline import init_notebook_mode, iplot, plot
import plotly.graph_objs as go
import ast
我们先来看看这组数据的主要元素
df_train.head(1).T(由于列数较多有23列,所以我们先用转置将它倒置,以便于我们能够更加清晰地观察这组数据)
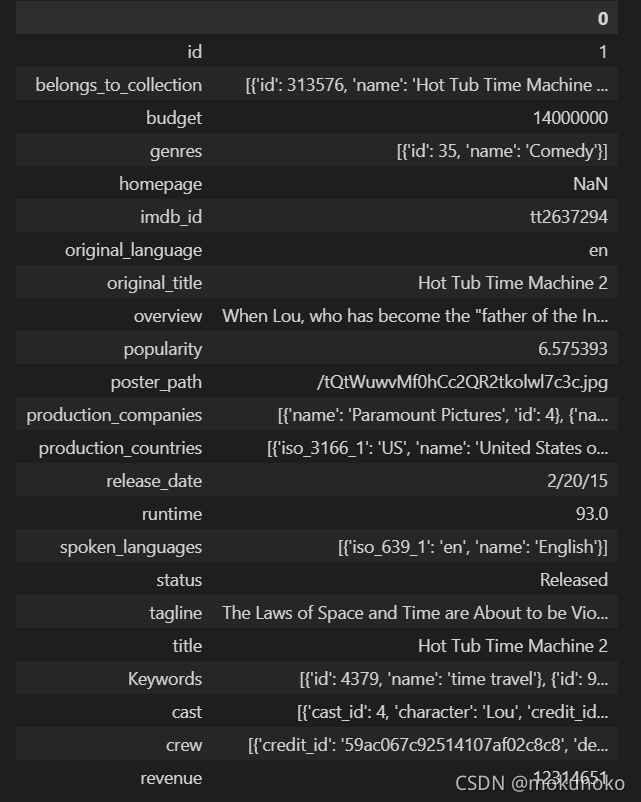
df_train.isna().sum()?
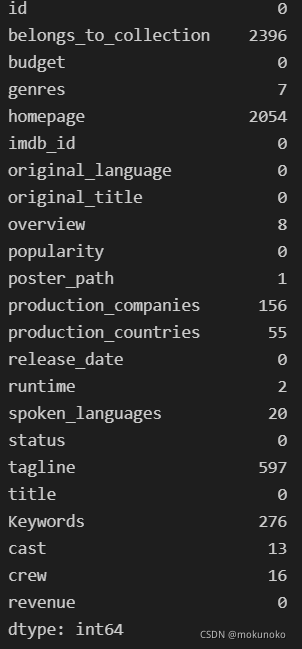
第一步:数据清洗?
1.我们可以很清晰地发现有三个缺失值特别多的地方,分别是(belongs_to_collection,homepage,tagline)而且这三个类型的数据分别属于同一个类型也就是主页,我们在后期可以将之归为一类处理
2.我们发现数据中有很多数据都是json数据,我们是不是应该将之转化为我们更好处理的数据类型以便后续的操作呢
3.剩下的数据nan进行依实际情况进行填补与删除就可以
先处理三个分类的数据,代码如下:(将nan转化为0,如有内容则变为1),并将之合并,category中为1的是有belongs_to_collection,homepage,tagline其中一种类型,为3的则是所有类型都有。
df_train["belongs_to_collection"] = df_train["belongs_to_collection"].isna().apply(lambda x : 0 if x else 1 )
df_train["homepage"] = df_train["homepage"].isna().apply(lambda x : 0 if x else 1)
df_train["tagline"] = df_train["tagline"].isna().apply(lambda x: 0 if x else 1)
df_test["belongs_to_collection"] = df_train["belongs_to_collection"].isna().apply(lambda x : 0 if x else 1 )
df_test["homepage"] = df_train["homepage"].isna().apply(lambda x : 0 if x else 1)
df_test["tagline"] = df_train["tagline"].isna().apply(lambda x: 0 if x else 1)
#将他们合并为一行以便于后面统计
df_train[["belongs_to_collection","homepage","tagline"]].head(20)
df_train["category"] = df_train.belongs_to_collection + df_train.homepage + df_train.tagline
df_test["category"] = df_test.belongs_to_collection + df_test.homepage + df_test.tagline
#drop掉合并前的那三列
df_train = df_train.drop(columns=["belongs_to_collection","homepage","tagline"])
df_test = df_test.drop(columns=["belongs_to_collection","homepage","tagline"])
?2.处理json数据,定义如下列表,将字符串转化为列表再从列表中一个个取出字典,并将名为“name”的键取出放进name_list,代码如下
#定义一个转化的方法将json转化成我们需要的列表
def transform_json(json_str):
name_list = []
dics = ast.literal_eval(json_str)
for dic in dics:
name = dic["name"]
name_list.append(name)
return name_list
#有nan的要处理稚只能扔掉或填补如果需要处理有nan的那一段数据的话实在不行就先处理非nan的
json_columns = ["genres","production_companies","production_countries","spoken_languages","Keywords","cast","crew"]
for column in json_columns:
df_train[column] = df_train.loc[df_train[column].notnull(),column].apply(lambda x:transform_json(x) if x else [])
df_test[column] = df_test.loc[df_test[column].notnull(),column].apply(lambda x:transform_json(x) if x else [])
#将nan填补为【】,非nan保留列表
json_columns = ["genres","production_companies","production_countries","spoken_languages","Keywords","cast","crew"]
for column in json_columns:
df_train[column] = df_train[column].apply(lambda x : x if isinstance(x,list) else [] )#如果种类为列表则保留,非字典则填补一个空列表【】
df_test[column] = df_test[column].apply(lambda x: x if isinstance(x,list) else [])
3.处理剩下的nan数据:
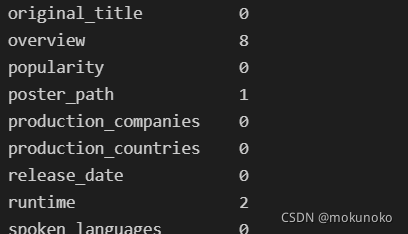
?
#把overview里面空缺的字符串用“”填补而nan的对象用dropna的方法去掉!!
df_train["overview"] = df_train["overview"].fillna("")
df_train = df_train.dropna()
df_test["overview"] = df_test["overview"].fillna("")
df_test = df_test.dropna()
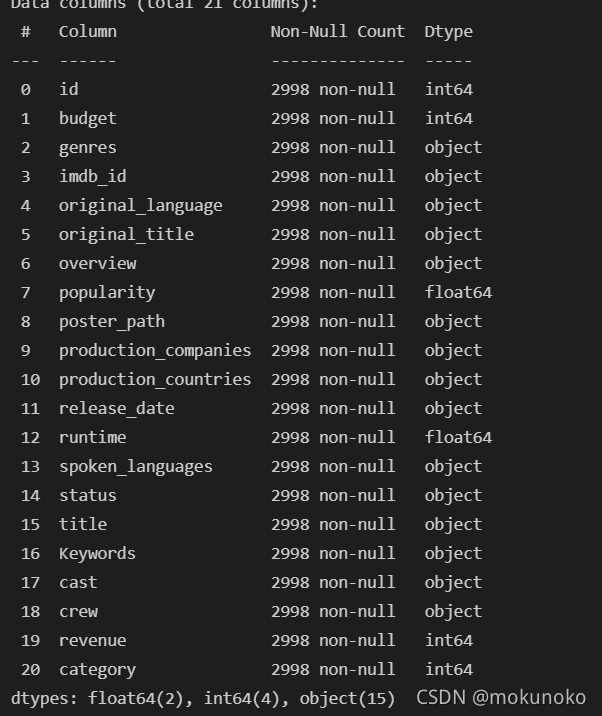
?
?第二步:数据分析(做出有相关性的类型以达到做模型的目的)
我们主要是挖掘出利润和不同自变量之间的关系所以创建一个新的dataframe;
df_compared_revenue = df_train[["revenue","popularity","runtime","budget"]]
1.流行度和利润之间的关系
#流行度和利润的关系
# fig = px.scatter(df_compared_revenue,x = "popularity",y = "revenue",color = "revenue",color_continuous_scale=px.colors.sequential.Bluered)
# fig.update_layout(title ="流行度和利润对比图")
# fig.show()
sns.set()
sns.scatterplot(df_compared_revenue[df_compared_revenue.popularity<50].popularity,df_compared_revenue[df_compared_revenue.popularity<50].revenue)
我们发现大于50的过于离散化了,所以取小于50流行度的进行分析,可视化结果如下:
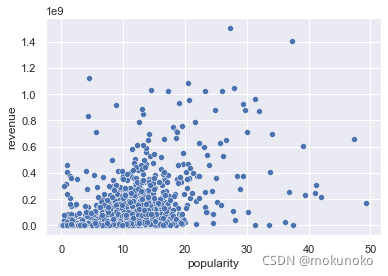
?通过以上的观察我们可以得到从流行度5到20这个过程中,我们可以发现流行度越高,普遍的他的利润越高,这是我们可以观察到的,而在20之后流行度的,由于数据比较分散不好统计,总的来说还是正相关的关系
2.电影时长和利润之间的关系:
由于大部分数据都是大于50分钟以上的电影,所以我们取大于50分钟的电影进行分析
sns.scatterplot(df_compared_revenue[df_compared_revenue.runtime >50].runtime,df_compared_revenue.revenue)
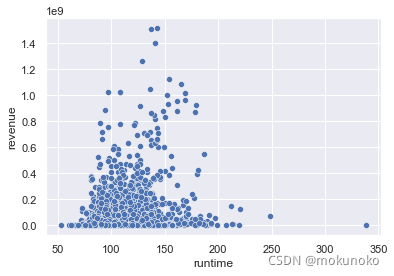
时长太短或者太长都不利于我们利润的增长,适当的时长对于利润来说是呈正相关的
3.电影预算与利润的关系:
sns.scatterplot(df_compared_revenue.budget,df_compared_revenue.revenue,color = "red")
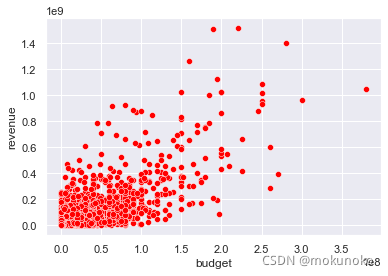
?过多的预算相对于利润来说是呈现正相关的关系及在预算增加的过程中收入相对应得也会增加,当然现实生活中预算不可能随意增加
4.放映时间与利润的关系:
df_train["release_date"] = pd.to_datetime(df_train.release_date)
#小于2016的数据会比较准确,因为2016年以后的数据有缺失
df_train.release_date.dt.year.value_counts().sort_values(ascending = False)[:50]
df_time_revenue = df_train[["revenue","release_date"]]
df_time_revenue = df_time_revenue[df_time_revenue["release_date"]<="2016" ]
df_time_revenue = df_time_revenue.set_index("release_date")
df_time_revenue = df_time_revenue.resample("Y").sum()
df_time_revenue
fig =px.line(df_time_revenue,x = df_time_revenue.index,y="revenue")
fig.show()
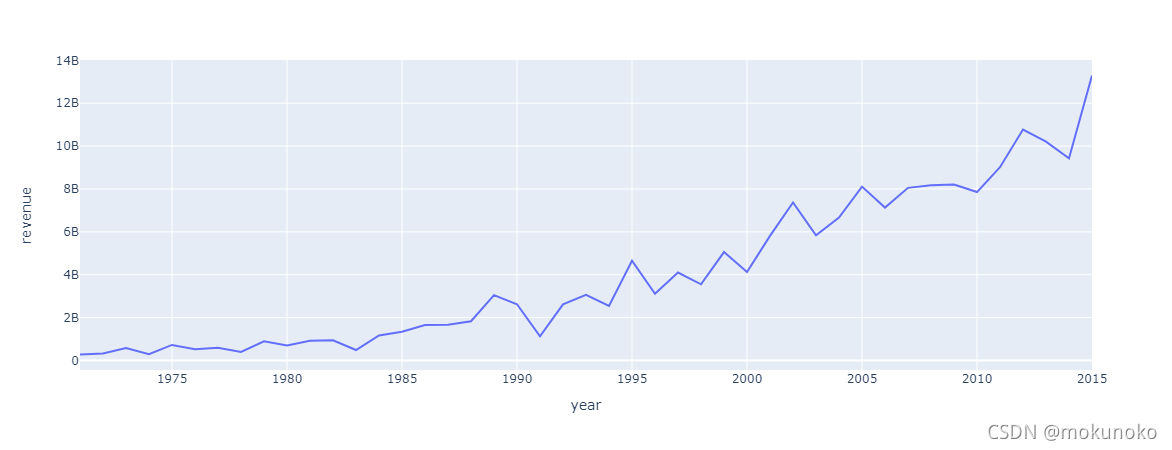
?人们的经济在变好,电影的消费也在蒸蒸日上,放映时间和利润也呈现正相关的关系
5.json数据的种类多少与利润的关系:
df_json_revenue = df_train[["genres","production_companies","production_countries","spoken_languages","Keywords","cast","crew","revenue"]]
一·种类多少与利润的关系:
sns.scatterplot(df_json_revenue.genres.apply(len),df_json_revenue.revenue)
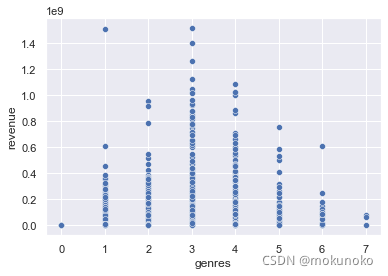
我们可以在图中很清晰地发现种类在3左右的利润将会达到峰值?
二·参与制作的国家多少与利润的关系:
sns.scatterplot(df_json_revenue.production_companies.apply(len),df_json_revenue.revenue)
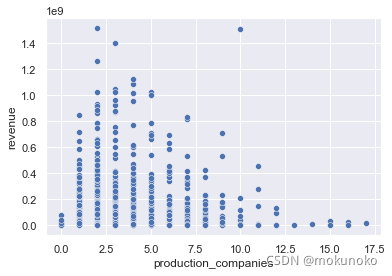
类别在三个的大部分电影的收入都会较高,国家制造在2.5到5个国家制作的话会到达峰值
?
第三步:特征工程
特征工程1.列表特征2、字符串特征 3.日期特征?
1.列表特征:
for column in json_columns:
df_train[column+ "_count"] = df_train[column].apply(len)
df_test[column+ "_count"] = df_test[column].apply(len)
计算出列表种类的个数
2.字符串特征
#处理字符串特征
str_list = ["original_title","overview","title"]
for str_obj in str_list:
df_train[str_obj+"_len"] = df_train[str_obj].apply(len)
df_test[str_obj+ "_len"] = df_test[str_obj].apply(len)
3.日期特征
#处理日期特征
df_train["day"] = df_train["release_date"].apply(lambda x: int(str(x).split("-")[2].split(" ")[0]))
df_train["month"] = df_train["release_date"].apply(lambda x: int(str(x).split("-")[1]))
df_train["year"] = df_train["release_date"].apply(lambda x: int(str(x).split("-")[0]))
df_train["dayofweek"] = df_train["release_date"].dt.dayofweek
df_test["release_date"] = pd.to_datetime(df_test.release_date)
df_test["day"] = df_test["release_date"].apply(lambda x: int(str(x).split("-")[2].split(" ")[0]))
df_test["month"] = df_test["release_date"].apply(lambda x: int(str(x).split("-")[1]))
df_test["year"] = df_test["release_date"].apply(lambda x: int(str(x).split("-")[0]))
df_test["dayofweek"] = df_test["release_date"].dt.dayofweek
第四步:构建模型
model_list = ["budget", "popularity", "runtime","category", 'genres_count', 'production_companies_count',
'production_countries_count', 'Keywords_count',
'spoken_languages_count', 'cast_count', 'crew_count',
'original_title_len', 'overview_len', 'title_len', "year","month","day", "dayofweek"]
x = df_train[model_list]
y = df_train["revenue"]
将我们所需的自变量和因变量准备好之后就可以准备好模型构建:
from xgboost import XGBRegressor
xgb = XGBRegressor(n_estimators= 2500,max_denth = 27,random_size = 0)
xgb.fit(x,y)
xgb.score(x,y)
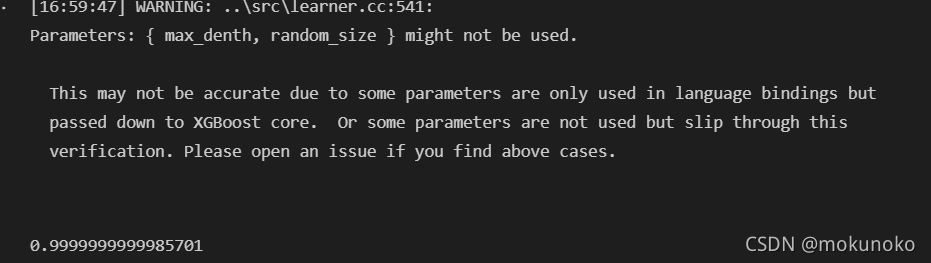
?
?拟合程度0.99完美拉!!
接下来就是预测我们的结果: ?
df_test["predict_revenue"] = xgb.predict(df_test[model_list])
df_test["predict_revenue"]
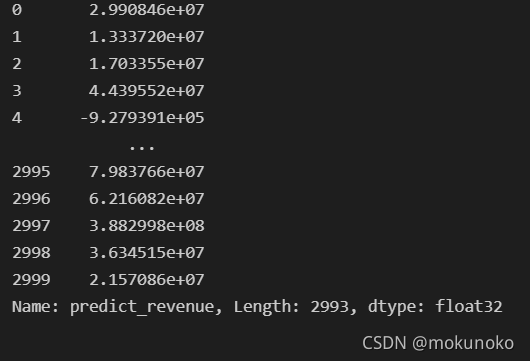
?预测结果如上图所示,本次实验结束!
|