[论文记录] 2019 - Partners in Crime: Utilizing Arousal-Valence Relationship for Continuous Prediction of Valence in Movies
论文简介
原论文:Partners in Crime: Utilizing Arousal-Valence Relationship for Continuous Prediction of Valence in Movies
利用“唤醒度-效价”的关系进行电影中“效价”的连续值预测
以下仅为作者阅读论文时的记录,学识浅薄,如有错误,欢迎指正。
论文内容
摘要
-
The arousal-valence model is often used in characterizing human emotions. 预备知识:唤醒度-效价模型经常被用于表征人类情感。 -
Arousal is defined as the intensity of emotion, while valence is defined as the polarity of emotion. 唤醒度被定义为情感的强度,而效价被定义为情感的极性。 -
Continuous prediction of valence in entertainment media such as movies is important for applications such as ad placement and personalized recommendations. 应用:对电影等娱乐性媒体中效价的连续值预测对于广告投放和个性化推荐等应用非常重要。 -
While arousal can be effectively predicted using audio-visual information in movies, valence is reported to be more difficult to predict as it also involves understanding the semantics of the movie. 问题:虽然在电影中可以利用视听信息有效预测唤醒度,但效价却更难预测,因为它也涉及到电影中的语义信息理解。 -
In this paper, for improving valence prediction, we utilize the insight from psychology that valence and arousal are interrelated. 依据:本文为了改进效价预测,利用了心理学的观点:效价和唤醒度相互关联。 -
We use Long Short Term Memory networks (LSTMs) to model the temporal context in movies using standard audio features as input. 算法:我们使用长短期记忆网络(LSTMs) 利用标准音频特征作为输入,对电影中时序上下文进行建模。 -
We incorporate arousal-valence interdependence in two ways: 我们用两种方式将唤醒度-效价的关联性进行结合: -
- as a joint loss function to optimize the prediction network;
作为联合损失函数来优化网络; -
- as a geometric constraint simulating the distribution of arousal-valence observed in psychology literature.
作为一种几何约束模拟心理学中观察到的唤醒度-效价分布。 -
Using a joint arousal-valence model, we predict continuous valence for a dataset containing Academy Award winning movies. 利用唤醒度-效价的联合模型,本文在奥斯卡获奖电影数据集上预测了效价的连续值。 -
We report a significant improvement over the state-of-the-art results, with an improved Pearson correlation of 0.69 between the annotation and prediction using the joint model, as compared to a baseline prediction of 0.49 using an independent valence model. 结果:本文的结果比SOTA有显著进步,利用联合模型进行的预测与标注之间的皮尔逊相关系数达到了0.69,而利用独立的效价模型进行预测的基线为0.49。
1 介绍
-
类似于电影这种娱乐媒体可以激发观看者一系列的情感,这种情感在强度(intensity) 和极性(polarity) 两个维度上随时间产生变化; -
情感变化往往与摄影手法有关,例如:音乐强度(music intensity),语言强度(speech intensity),镜头框架(shot framing),构图(composition)和角色运动(character movements); -
静态因素例如:色调(color tones)和环境音(ambient sound),也会影响到场景的情感极性; -
电影情感预测的应用很广泛,例如:
- 投放广告( place advertisements)
CYadati, K., Katti, H., Kankanhalli, M.: Cavva: Computational affective video-in-video advertising. IEEE Transactions on Multimedia 16(1), 15–23 (2014) - 内容推荐(content recommendation)
Canini, L., Benini, S., Leonardi, R.: Affective recommendation of movies based on selected connotative features. IEEE Transactions on Circuits and Systems for Video Technology 23(4), 636–647 (2013) - 内容索引( content indexing)
Zhang, S., Huang, Q., Jiang, S., Gao, W., Tian, Q.: Affective visualization and retrieval for music video. IEEE Transactions on Multimedia 12(6), 510–522 (2010) -
提出影视情感可以映射到唤醒度(Arousal)-效价(Valence) 空间中,唤醒度表示情感的强度,效价表示情感极性(正向、负向、中性),如下图所示,不同场景激发的情绪被映射到2D空间中对应的位置,整体展现出一个抛物线的轮廓;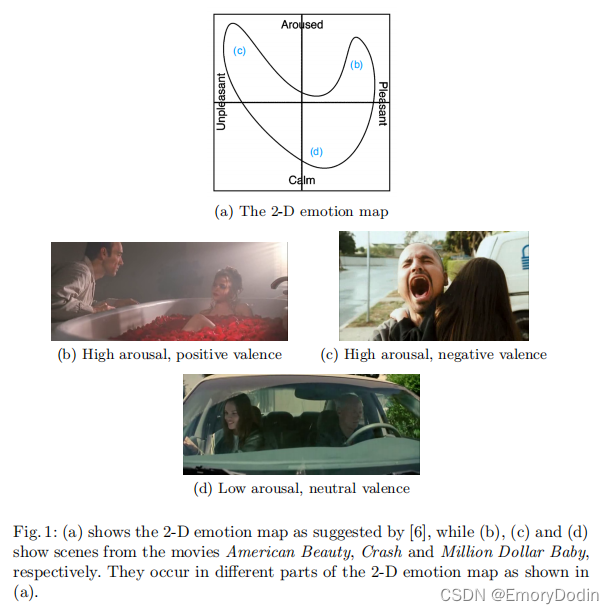 -
这项任务很具有挑战性,因为电影动态融合了听觉、视觉、文本(语义)多种模态的信息,一些相关工作如下:
- 核方法和深度学习预测30个短电影的VA值
Baveye, Y., Chamaret, C., Dellandr′ea, E., Chen, L.: Affective video content analysis: A multidisciplinary insight. IEEE Transactions on Affective Computing (2017) - 手工视听特征预测12部30分钟奥斯卡获奖电影片段的VA值
Malandrakis, N., Potamianos, A., Evangelopoulos, G., Zlatintsi, A.: A supervised approach to movie emotion tracking. In: Acoustics, Speech and Signal Processing (ICASSP), 2011 IEEE International Conference on. pp. 2376–2379. IEEE (2011) - 混合专家模型(Mixture-of-Experts ,MoE) 来改进视听模型的融合
Goyal, A., Kumar, N., Guha, T., Narayanan, S.S.: A multimodal mixture-of-experts model for dynamic emotion prediction in movies. In: Acoustics, Speech and Signal Processing (ICASSP), 2016 IEEE International Conference on. pp. 2822–2826. IEEE (2016) - 长短时记忆网络(Long Short Term Memory networks ,LSTMs) 捕获视听信息的上下文
Sivaprasad, S., Joshi, T., Agrawal, R., Pedanekar, N.: Multimodal continuous prediction of emotions in movies using long short-term memory networks. In: Proceedings of the 2018 ACM on International Conference on Multimedia Retrieval. pp. 413–419. ACM (2018) -
观察发现效价(Valence) 往往比唤醒度(Arousal)的预测效果更差,因为Valence预测需要更多高阶语义信息,例如:一场打斗应该有负面的含义,但如果主角赢了就是正向的情感;花园中明亮的景象应该有正向的含义,但对话可能更偏向负向情感; -
上述工作都是将Valence与Arousal分开建模的,下述文献提议将二者联合建模,用LSTM在200个5-30s的短视频上预测VA值: Zhang, L., Zhang, J.: Synchronous prediction of arousal and valence using lstm network for affective video content analysis. In: 2017 13th International Conference on Natural Computation, Fuzzy Systems and Knowledge Discovery (ICNC-FSKD). pp. 727–732. IEEE (2017) -
本文使用COGNIMUSE数据集,因为本文认为电影的情感预测在实际应用中很必要,该数据集标注的Valence与Arousal相关性较高(0.62),本文希望利用Arousal的信息来预测Valence,如果能够利用认知心理学的观点,也就是Arousal和Valence通常在一个抛物线范围内(如上图a),也许可以进一步提升Valence的预测;
|