??本文是PyTorch入门的第二篇文章,后续将会持续更新,作为PyTorch系列文章。 ??本文将会介绍如何使用PyTorch来搭建简单的MLP(Multi-layer Perceptron,多层感知机)模型,来实现二分类及多分类任务。
数据集介绍
?? 二分类数据集为ionosphere.csv (电离层数据集),是UCI机器学习数据集中的经典二分类数据集。它一共有351个观测值,34个自变量,1个因变量(类别),类别取值为g (good)和b (bad)。在ionosphere.csv 文件中,共351行,前34列作为自变量(输入的X),最后一列作为类别值(输出的y)。 ? ? 多分类数据集为iris.csv (鸢尾花数据集),是UCI机器学习数据集中的经典多分类数据集。它一共有150个观测值,4个自变量(萼片长度,萼片宽度,花瓣长度,花瓣宽度),1个因变量(类别),类别取值为Iris-setosa ,Iris-versicolour ,Iris-virginica 。在iris.csv 文件中,共150行,前4列作为自变量(输入的X),最后一列作为类别值(输出的y)。前几行数据如下图: 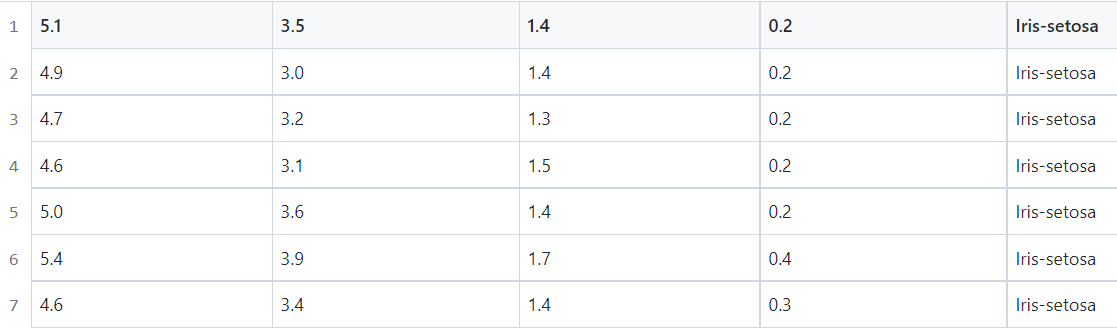
分类模型流程
??使用PyTorch构建神经网络模型来解决分类问题的基本流程如下:
加载数据集
划分数据集
构建模型
选择损失函数及优化器
模型训练
模型预测
其中加载数据集 和划分数据集 为数据处理部分,构建模型 和选择损失函数及优化器 为创建模型部分,模型训练 的目标是选择合适的优化器及训练步长使得损失函数的值很小,模型预测 是在模型测试集或新数据上的预测。
二分类模型
??使用PyTorch构建MLP模型来实现二分类任务,模型结果图如下:
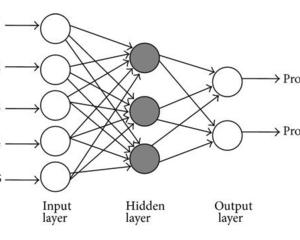 实现MLP模型的Python代码如下:
from numpy import vstack
from pandas import read_csv
from sklearn.preprocessing import LabelEncoder
from sklearn.metrics import accuracy_score
from torch import Tensor
from torch.optim import SGD
from torch.utils.data import Dataset, DataLoader, random_split
from torch.nn import Linear, ReLU, Sigmoid, Module, BCELoss
from torch.nn.init import kaiming_uniform_, xavier_uniform_
class CSVDataset(Dataset):
def __init__(self, path):
df = read_csv(path, header=None)
self.X = df.values[:, :-1]
self.y = df.values[:, -1]
self.X = self.X.astype('float32')
self.y = LabelEncoder().fit_transform(self.y)
self.y = self.y.astype('float32')
self.y = self.y.reshape((len(self.y), 1))
def __len__(self):
return len(self.X)
def __getitem__(self, idx):
return [self.X[idx], self.y[idx]]
def get_splits(self, n_test=0.3):
test_size = round(n_test * len(self.X))
train_size = len(self.X) - test_size
return random_split(self, [train_size, test_size])
class MLP(Module):
def __init__(self, n_inputs):
super(MLP, self).__init__()
self.hidden1 = Linear(n_inputs, 10)
kaiming_uniform_(self.hidden1.weight, nonlinearity='relu')
self.act1 = ReLU()
self.hidden2 = Linear(10, 8)
kaiming_uniform_(self.hidden2.weight, nonlinearity='relu')
self.act2 = ReLU()
self.hidden3 = Linear(8, 1)
xavier_uniform_(self.hidden3.weight)
self.act3 = Sigmoid()
def forward(self, X):
X = self.hidden1(X)
X = self.act1(X)
X = self.hidden2(X)
X = self.act2(X)
X = self.hidden3(X)
X = self.act3(X)
return X
def prepare_data(path):
dataset = CSVDataset(path)
train, test = dataset.get_splits()
train_dl = DataLoader(train, batch_size=32, shuffle=True)
test_dl = DataLoader(test, batch_size=1024, shuffle=False)
return train_dl, test_dl
def train_model(train_dl, model):
criterion = BCELoss()
optimizer = SGD(model.parameters(), lr=0.01, momentum=0.9)
for epoch in range(100):
for i, (inputs, targets) in enumerate(train_dl):
optimizer.zero_grad()
yhat = model(inputs)
loss = criterion(yhat, targets)
loss.backward()
print("epoch: {}, batch: {}, loss: {}".format(epoch, i, loss.data))
optimizer.step()
def evaluate_model(test_dl, model):
predictions, actuals = [], []
for i, (inputs, targets) in enumerate(test_dl):
yhat = model(inputs)
yhat = yhat.detach().numpy()
actual = targets.numpy()
actual = actual.reshape((len(actual), 1))
yhat = yhat.round()
predictions.append(yhat)
actuals.append(actual)
predictions, actuals = vstack(predictions), vstack(actuals)
acc = accuracy_score(actuals, predictions)
return acc
def predict(row, model):
row = Tensor([row])
yhat = model(row)
yhat = yhat.detach().numpy()
return yhat
path = './data/ionosphere.csv'
train_dl, test_dl = prepare_data(path)
print(len(train_dl.dataset), len(test_dl.dataset))
model = MLP(34)
print(model)
train_model(train_dl, model)
acc = evaluate_model(test_dl, model)
print('Accuracy: %.3f' % acc)
row = [1, 0, 0.99539, -0.05889, 0.85243, 0.02306, 0.83398, -0.37708, 1, 0.03760, 0.85243, -0.17755, 0.59755, -0.44945,
0.60536, -0.38223, 0.84356, -0.38542, 0.58212, -0.32192, 0.56971, -0.29674, 0.36946, -0.47357, 0.56811, -0.51171,
0.41078, -0.46168, 0.21266, -0.34090, 0.42267, -0.54487, 0.18641, -0.45300]
yhat = predict(row, model)
print('Predicted: %.3f (class=%d)' % (yhat, yhat.round()))
在上面代码中,CSVDataset类为csv数据集加载类,处理成模型适合的数据格式,并划分训练集和测试集比例为7:3。MLP类为MLP模型,模型输出层采用Sigmoid函数,损失函数采用BCELoss,优化器采用SGD,共训练100次。evaluate_model函数是模型在测试集上的表现,predict函数为在新数据上的预测结果。MLP模型的PyTorch输出如下:
MLP(
(hidden1): Linear(in_features=34, out_features=10, bias=True)
(act1): ReLU()
(hidden2): Linear(in_features=10, out_features=8, bias=True)
(act2): ReLU()
(hidden3): Linear(in_features=8, out_features=1, bias=True)
(act3): Sigmoid()
)
? 运行上述代码,输出结果如下:
epoch: 0, batch: 0, loss: 0.7491992712020874
epoch: 0, batch: 1, loss: 0.750106692314148
epoch: 0, batch: 2, loss: 0.7033759355545044
......
epoch: 99, batch: 5, loss: 0.020291464403271675
epoch: 99, batch: 6, loss: 0.02309396117925644
epoch: 99, batch: 7, loss: 0.0278386902064085
Accuracy: 0.924
Predicted: 0.989 (class=1)
可以看到,该MLP模型的最终训练loss值为0.02784,在测试集上的Accuracy为0.924,在新数据上预测完全正确。
多分类模型
? 接着我们来创建MLP模型实现iris数据集的三分类任务,Python代码如下:
from numpy import vstack
from numpy import argmax
from pandas import read_csv
from sklearn.preprocessing import LabelEncoder, LabelBinarizer
from sklearn.metrics import accuracy_score
from torch import Tensor
from torch.optim import SGD, Adam
from torch.utils.data import Dataset, DataLoader, random_split
from torch.nn import Linear, ReLU, Softmax, Module, CrossEntropyLoss
from torch.nn.init import kaiming_uniform_, xavier_uniform_
class CSVDataset(Dataset):
def __init__(self, path):
df = read_csv(path, header=None)
self.X = df.values[:, :-1]
self.y = df.values[:, -1]
self.X = self.X.astype('float32')
self.y = LabelEncoder().fit_transform(self.y)
def __len__(self):
return len(self.X)
def __getitem__(self, idx):
return [self.X[idx], self.y[idx]]
def get_splits(self, n_test=0.3):
test_size = round(n_test * len(self.X))
train_size = len(self.X) - test_size
return random_split(self, [train_size, test_size])
class MLP(Module):
def __init__(self, n_inputs):
super(MLP, self).__init__()
self.hidden1 = Linear(n_inputs, 5)
kaiming_uniform_(self.hidden1.weight, nonlinearity='relu')
self.act1 = ReLU()
self.hidden2 = Linear(5, 6)
kaiming_uniform_(self.hidden2.weight, nonlinearity='relu')
self.act2 = ReLU()
self.hidden3 = Linear(6, 3)
xavier_uniform_(self.hidden3.weight)
self.act3 = Softmax(dim=1)
def forward(self, X):
X = self.hidden1(X)
X = self.act1(X)
X = self.hidden2(X)
X = self.act2(X)
X = self.hidden3(X)
X = self.act3(X)
return X
def prepare_data(path):
dataset = CSVDataset(path)
train, test = dataset.get_splits()
train_dl = DataLoader(train, batch_size=1, shuffle=True)
test_dl = DataLoader(test, batch_size=1024, shuffle=False)
return train_dl, test_dl
def train_model(train_dl, model):
criterion = CrossEntropyLoss()
optimizer = Adam(model.parameters())
for epoch in range(100):
for i, (inputs, targets) in enumerate(train_dl):
targets = targets.long()
optimizer.zero_grad()
yhat = model(inputs)
loss = criterion(yhat, targets)
loss.backward()
print("epoch: {}, batch: {}, loss: {}".format(epoch, i, loss.data))
optimizer.step()
def evaluate_model(test_dl, model):
predictions, actuals = [], []
for i, (inputs, targets) in enumerate(test_dl):
yhat = model(inputs)
yhat = yhat.detach().numpy()
actual = targets.numpy()
yhat = argmax(yhat, axis=1)
actual = actual.reshape((len(actual), 1))
yhat = yhat.reshape((len(yhat), 1))
predictions.append(yhat)
actuals.append(actual)
predictions, actuals = vstack(predictions), vstack(actuals)
acc = accuracy_score(actuals, predictions)
return acc
def predict(row, model):
row = Tensor([row])
yhat = model(row)
yhat = yhat.detach().numpy()
return yhat
path = './data/iris.csv'
train_dl, test_dl = prepare_data(path)
print(len(train_dl.dataset), len(test_dl.dataset))
model = MLP(4)
print(model)
train_model(train_dl, model)
acc = evaluate_model(test_dl, model)
print('Accuracy: %.3f' % acc)
row = [5.1, 3.5, 1.4, 0.2]
yhat = predict(row, model)
print('Predicted: %s (class=%d)' % (yhat, argmax(yhat)))
可以看到,多分类代码与二分类代码大同小异,在加载数据集、模型结构、模型训练(训练batch值取1)代码上略有不同。运行上述代码,输出结果如下:
105 45
MLP(
(hidden1): Linear(in_features=4, out_features=5, bias=True)
(act1): ReLU()
(hidden2): Linear(in_features=5, out_features=6, bias=True)
(act2): ReLU()
(hidden3): Linear(in_features=6, out_features=3, bias=True)
(act3): Softmax(dim=1)
)
epoch: 0, batch: 0, loss: 1.4808106422424316
epoch: 0, batch: 1, loss: 1.4769641160964966
epoch: 0, batch: 2, loss: 0.654313325881958
......
epoch: 99, batch: 102, loss: 0.5514447093009949
epoch: 99, batch: 103, loss: 0.620153546333313
epoch: 99, batch: 104, loss: 0.5514482855796814
Accuracy: 0.933
Predicted: [[9.9999809e-01 1.8837408e-06 2.4509615e-19]] (class=0)
可以看到,该MLP模型的最终训练loss值为0.5514,在测试集上的Accuracy为0.933,在新数据上预测完全正确。
总结
??本文介绍的模型代码已开源,Github地址为:https://github.com/percent4/PyTorch_Learning。后续将持续介绍PyTorch内容,欢迎大家关注~
|