数据集:乳腺癌数据集(from sklearn.datasets import load_breast_cancer)。
(1)将样本集划分为70%的训练集,30%作为测试集,分别用逻辑回归算法和KNN算法(需要先对数据进行标准化)建模(不指定参数),输出其测试结果的混淆矩阵,计算其准确率、查全率和假正率。
(2)利用搜索网格,分别确定逻辑回归及KNN模型的最优参数。 KNN算法的主要参数提示: ①n_neighbors(最近邻个数) 取值一般为奇数。 ②algorithm(用于计算最近邻的算法) 取值有‘auto’, ‘ball_tree’, ‘kd_tree’, ‘brute’等,默认为‘auto’。注意:算法选择不影响KNN的最终结果,只影响模型的性能(计算的快慢程度)。 ③p(Minkowski距离的指标参数) 默认取p=2,即欧氏距离。而p=1为曼哈顿距离。如果需要使用非明氏距离的其它指标,应修改metric参数的值。 ④weights(权重) 预测中使用的权重函数。可能的取值:‘uniform’:统一权重,即每个邻域中的所有点均被加权。 ‘distance’:权重点与其距离的倒数,在这种情况下,查询点的近邻比远处的近邻具有更大的影响力。
(3)对整个数据集使用K折交叉验证方式(k=2,3,4,5,6,7,8,9,10),分别用逻辑回归和KNN建模(用上一步确定的最优参数),绘图对比两种模型在k取不同值下的的分类准确率。
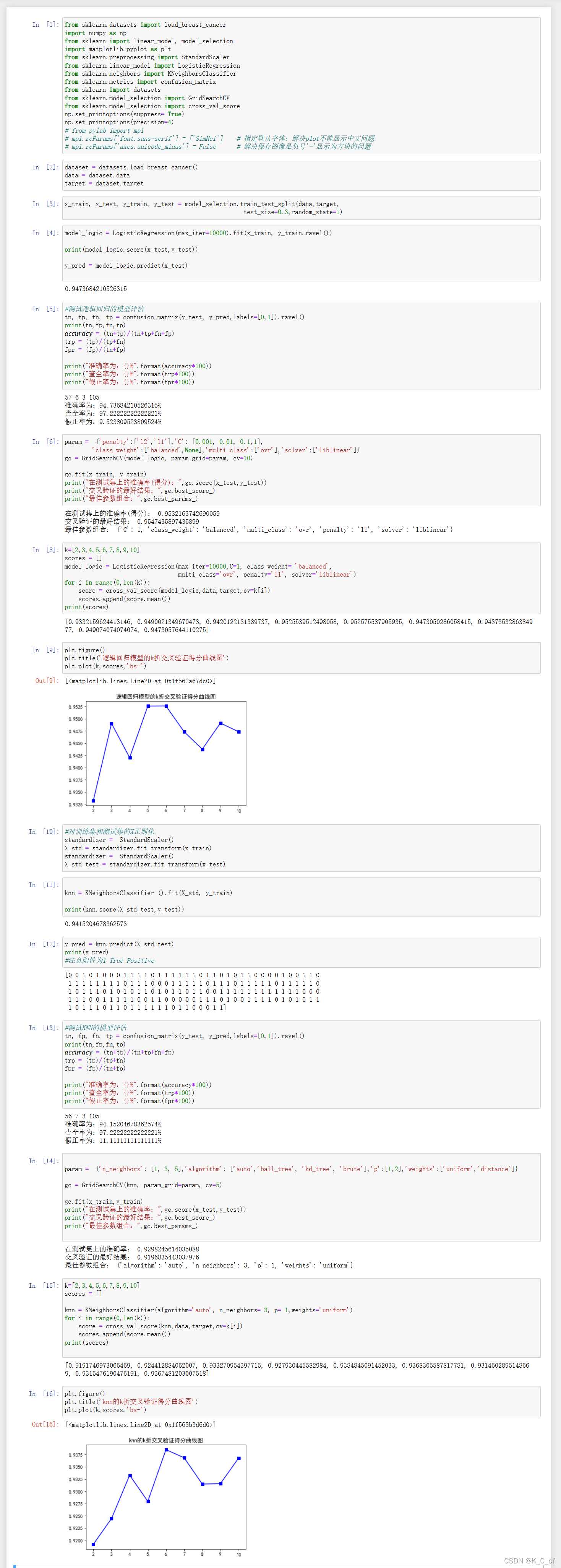
from sklearn.datasets import load_breast_cancer
import numpy as np
from sklearn import linear_model, model_selection
import matplotlib.pyplot as plt
from sklearn.preprocessing import StandardScaler
from sklearn.linear_model import LogisticRegression
from sklearn.neighbors import KNeighborsClassifier
from sklearn.metrics import confusion_matrix
from sklearn import datasets
from sklearn.model_selection import GridSearchCV
from sklearn.model_selection import cross_val_score
np.set_printoptions(suppress= True)
np.set_printoptions(precision=4)
dataset = datasets.load_breast_cancer()
data = dataset.data
target = dataset.target
x_train, x_test, y_train, y_test = model_selection.train_test_split(data,target,
test_size=0.3,random_state=1)
model_logic = LogisticRegression(max_iter=10000).fit(x_train, y_train.ravel())
print(model_logic.score(x_test,y_test))
y_pred = model_logic.predict(x_test)
tn, fp, fn, tp = confusion_matrix(y_test, y_pred,labels=[0,1]).ravel()
print(tn,fp,fn,tp)
𝑎𝑐𝑐𝑢𝑟𝑎𝑐𝑦 = (tn+tp)/(tn+tp+fn+fp)
trp = (tp)/(tp+fn)
fpr = (fp)/(tn+fp)
print("准确率为:{}%".format(accuracy*100))
print("查全率为:{}%".format(trp*100))
print("假正率为:{}%".format(fpr*100))
param = {'penalty':['l2','l1'],'C': [0.001, 0.01, 0.1,1],
'class_weight':['balanced',None],'multi_class':['ovr'],'solver':['liblinear']}
gc = GridSearchCV(model_logic, param_grid=param, cv=10)
gc.fit(x_train, y_train)
print("在测试集上的准确率(得分):",gc.score(x_test,y_test))
print("交叉验证的最好结果:",gc.best_score_)
print("最佳参数组合:",gc.best_params_)
k=[2,3,4,5,6,7,8,9,10]
scores = []
model_logic = LogisticRegression(max_iter=10000,C=1, class_weight= 'balanced',
multi_class='ovr', penalty='l1', solver='liblinear')
for i in range(0,len(k)):
score = cross_val_score(model_logic,data,target,cv=k[i])
scores.append(score.mean())
print(scores)
plt.figure()
plt.title('逻辑回归模型的k折交叉验证得分曲线图')
plt.plot(k,scores,'bs-')
standardizer = StandardScaler()
X_std = standardizer.fit_transform(x_train)
standardizer = StandardScaler()
X_std_test = standardizer.fit_transform(x_test)
knn = KNeighborsClassifier ().fit(X_std, y_train)
print(knn.score(X_std_test,y_test))
y_pred = knn.predict(X_std_test)
print(y_pred)
tn, fp, fn, tp = confusion_matrix(y_test, y_pred,labels=[0,1]).ravel()
print(tn,fp,fn,tp)
𝑎𝑐𝑐𝑢𝑟𝑎𝑐𝑦 = (tn+tp)/(tn+tp+fn+fp)
trp = (tp)/(tp+fn)
fpr = (fp)/(tn+fp)
print("准确率为:{}%".format(accuracy*100))
print("查全率为:{}%".format(trp*100))
print("假正率为:{}%".format(fpr*100))
param = {'n_neighbors': [1, 3, 5],'algorithm': ['auto','ball_tree', 'kd_tree', 'brute'],'p':[1,2],'weights':['uniform','distance']}
gc = GridSearchCV(knn, param_grid=param, cv=5)
gc.fit(x_train,y_train)
print("在测试集上的准确率:",gc.score(x_test,y_test))
print("交叉验证的最好结果:",gc.best_score_)
print("最佳参数组合:",gc.best_params_)
k=[2,3,4,5,6,7,8,9,10]
scores = []
knn = KNeighborsClassifier(algorithm='auto', n_neighbors= 3, p= 1,weights='uniform')
for i in range(0,len(k)):
score = cross_val_score(knn,data,target,cv=k[i])
scores.append(score.mean())
print(scores)
plt.figure()
plt.title('knn的k折交叉验证得分曲线图')
plt.plot(k,scores,'bs-')
注意,对于画曲线图中文乱码问题: from pylab import mpl mpl.rcParams[‘font.sans-serif’] = [‘SimHei’]
对于knn的k折交叉验证和网格搜索,应该也需要对测试的数据进行标准化。 因版本问题导致的参数设置,特别是算法选择的参数无法设置
|