PyTorch深度学习(六):PyTorch进阶与核心(中)
一、torch.nn——PyTorch神经网络的核心
1.torch.nn.Module——神经网络的基类
torch.nn.Module 是所有神经网络的基类,所有搭建神经网络的子类都需要继承 torch.nn.Module,该基类为所有神经网络提供基本的骨架
- 搭建一个神经网络时,我们可以构建一个类,这个类需要继承基类 torch.nn.Module:
class Model(nn.Module) ; - 接着,我们需要重写
__init__() 方法,在 __init__() 方法中我们需要调用 super(Model, self).__init__() 来继承来自基类 torch.nn.Module 中的所有属性; - 其次,每个子类要重写
forward(x) 函数,该函数表示前向传播(加入 PyTorch 计算图)的过程 - 在构建神经网络时,使用
model_name = Model() 进行实例化,同时,在进行前向传播时直接调用 model_name(x) 即可,这是因为 torch.nn.Module 内置的 __call__ 方法中调用了 forward(x) 函数,因此,调用 model_name(x) 时就相当于调用了 forward(x) 函数,以此进行前向传播
import torch.nn as nn
import torch.nn.functional as F
class Model(nn.Module):
def __init__(self):
super(Model, self).__init__()
pass
def forward(self, x):
pass
👇后面介绍的这些方法,都是一个类,都需要实例化后再调用来进行使用👇
2.torch.nn的卷积层
nn.Conv1d(…) 一维卷积 torch.nn.Conv2d(in_channels, out_channels, kernel_size, stride=1, padding=0, dilation=1, groups=1, bias=True, padding_mode=‘zeros’, device=None, dtype=None) 二维卷积(图片) nn.Conv3d(…) 三维卷积 🔺卷积核内部的元素的选取符合一定的分布规律,PyTorch文档中有作出解释 in_channels (int) 输入图像的通道数 out_channels (int) 卷积后输出的通道数 kernel_size (int or tuple) 卷积核大小,如:1×1、3×3、5×5 卷积核,tuple 则可以定义不规则的卷积核 stride (int or tuple) 卷积核移动的步距,default=1 若输入的是 tuple,则 tuple[0] 表示垂直方向的步距;则 tuple[1] 表示水平方向的步距 padding (int, tuple or str, optional) 对输入图像四周填充
0
0
0 像素点的圈数,default=0 默认不填充,填充可使输入输出的宽高不变; 若输入的是 tuple,则 tuple[0] 表示使 H 增大的方向的填充;则 tuple[1] 表示使 W 增大的方向的填充 dilation (int or tuple, optional) 卷积核中两元素的距离大小,该参数用于构造 “空洞卷积”,正常情况下卷积核两元素的距离为
1
1
1,即 default=1;当 default>1 为空洞卷积,表示卷积核两元素的距离更大,default>1 就可以形成空洞 若输入的是 tuple,则 tuple[0] 表示元素在垂直方向上的距离;则 tuple[1] 表示元素在水平方向上的距离 groups (int, optional) “分组卷积” 需要设置的参数,default=1
例如: 基于 nn.Module 构建一个卷积模型,尝试对图像进行卷积操作,通过 tensorboard 对比原图和卷积后的图像,观察它们有什么区别
import torch.nn as nn
import torchvision
from torchvision import transforms
from torch.utils.tensorboard import SummaryWriter
from torch.utils.data import DataLoader
class Model(nn.Module):
def __init__(self):
super(Model, self).__init__()
self.conv1 = nn.Conv2d(3, 6, 3)
def forward(self, x):
return self.conv1(x)
totensor = transforms.ToTensor()
writer = SummaryWriter("logs")
test_set = torchvision.datasets.CIFAR10("./CIFAR10", train=False, transform=totensor, download=True)
test_loader = DataLoader(dataset=test_set, batch_size=64, shuffle=False, num_workers=0, drop_last=False)
convolution_model = Model()
step = 0
for data in test_loader:
images, labels = data
writer.add_images("test_loader", images, step)
output = convolution_model(images)
writer.add_images("After Convolution1", output[:, 0:3, :, :], step)
writer.add_images("After Convolution2", output[:, 3:6, :, :], step)
step = step + 1
由于卷积后的图像为
(
64
,
6
,
30
,
30
)
(64,6,30,30)
(64,6,30,30),通道数为6,无法直接可视化; 但通道数由
3
?
6
3\Longrightarrow6
3?6 的变化实际上是两个卷积核分别作用于图像的结果; 因此前
3
3
3 个通道是一个卷积核作用的结果,而后
3
3
3 个通道则是另一个卷积核作用的结果 因此我们可以分别使用 output[:, 0:3, :, :] 和 output[:, 3:6, :, :] 来分别展示两个卷积核的卷积结果
转换结果如图所示: 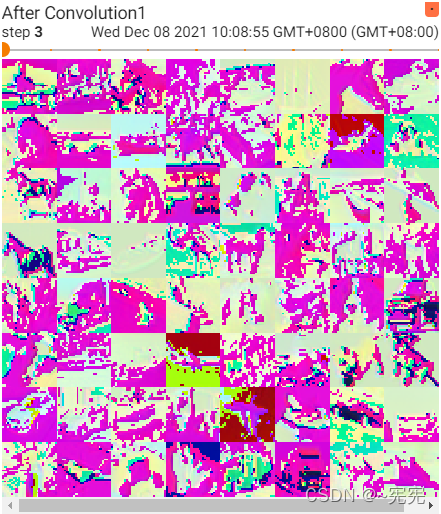 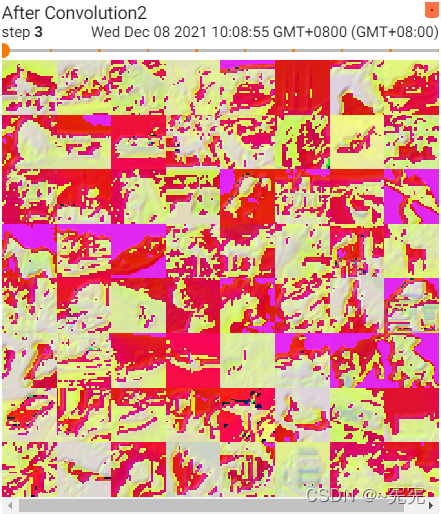
3.torch.nn的池化层
1??最大池化(下采样): nn.MaxPool1d(…) 一维最大池化 torch.nn.MaxPool2d(kernel_size, stride=None, padding=0, dilation=1, return_indices=False, ceil_mode=False) 二维最大池化 nn.MaxPool3d(…) 三维最大池化 kernel_size (int or tuple) 池化窗口的大小 若输入的是 tuple,则 tuple[0] 表示 H;则 tuple[1] 表示 W stride (int or tuple) 池化窗口的步距,default=kernel_size 若输入的是 tuple,则 tuple[0] 表示垂直方向的步距;则 tuple[1] 表示水平方向的步距 padding (int or tuple) 对输入特征图四周填充
0
0
0 像素点的圈数,default=0 默认不填充 若输入的是 tuple,则 tuple[0] 表示使 H 增大的方向的填充;则 tuple[1] 表示使 W 增大的方向的填充 dilation (int or tuple) 池化窗口中两元素的距离大小,和 “空洞卷积” 类似,正常情况下池化窗口两元素的距离为
1
1
1,即 default=1;当 default>1 就构成了空洞窗口 若输入的是 tuple,则 tuple[0] 表示元素在垂直方向上的距离;则 tuple[1] 表示元素在水平方向上的距离 return_indices (bool, optional) 如果为 True,则返回最大值及其所在下标,default=False ceil_mode (bool, optional) 当图像的 W 或 H 无法整除 kernel_size 时,说明池化窗口突出了(池化窗口无法填满),ceil_mode 就决定了剩余的这些无法填满池化窗口的元素是否保留 若 ceil_mode=True,则决定保留这些元素;若 ceil_mode=False 则决定不保留这些元素,default=False 2??平均池化: torch.nn.AvgPool1d(…) 一维平均池化 torch.nn.AvgPool2d(kernel_size, stride=None, padding=0, ceil_mode=False, count_include_pad=True, divisor_override=None) 二维平均池化 torch.nn.AvgPool3d(…) 三维平均池化 kernel_size (int or tuple) 池化窗口的大小 stride (int or tuple) 池化窗口的步距,default=kernel_size padding (int or tuple) 对输入特征图四周填充像素点的圈数,default=0 默认不填充 ceil_mode (bool) 当图像的 W 或 H 无法整除 kernel_size 时,说明池化窗口突出了(池化窗口无法填满),ceil_mode 就决定了剩余的这些无法填满池化窗口的元素是否保留 若 ceil_mode=True,则决定保留这些元素;若 ceil_mode=False 则决定不保留这些元素,default=False count_include_pad (bool) 计算平均值时是否包含
0
0
0 填充?count_include_pad=True 时包含
0
0
0 填充,default=True divisor_override 计算平均值时的除数,如果指定,则平均池化的除数为该参数,否则为池化窗口面积 3??最大池化的逆(上采样): nn.MaxUnpool1d(…) 一维上采样 nn.MaxUnpool2d(…) 二维上采样 nn.MaxUnpool3d(…) 三维上采样 … …
例如: 基于 nn.Module 构建一个 卷积+池化 模型,尝试对图像进行操作,通过 tensorboard 对比原图和输出图像,观察它们有什么区别
import torch.nn as nn
import torchvision
from torchvision import transforms
from torch.utils.tensorboard import SummaryWriter
from torch.utils.data import DataLoader
class Model(nn.Module):
def __init__(self):
super(Model, self).__init__()
self.conv1 = nn.Conv2d(3, 6, 3)
self.maxpool1 = nn.MaxPool2d(3)
def forward(self, x):
x = self.conv1(x)
y = self.maxpool1(x)
return y
totensor = transforms.ToTensor()
writer = SummaryWriter("logs")
test_set = torchvision.datasets.CIFAR10("./CIFAR10", train=False, transform=totensor, download=True)
test_loader = DataLoader(dataset=test_set, batch_size=64, shuffle=False, num_workers=0, drop_last=False)
model = Model()
step = 0
for data in test_loader:
images, labels = data
writer.add_images("test_loader", images, step)
output = model(images)
writer.add_images("After Convolution and MaxPooling 1", output[:, 0:3, :, :], step)
writer.add_images("After Convolution and MaxPooling 2", output[:, 3:6, :, :], step)
step = step + 1
可以看到,经过卷积+池化后的图像明显比只经过卷积的图像更模糊了,但仍然能够看到一些特征 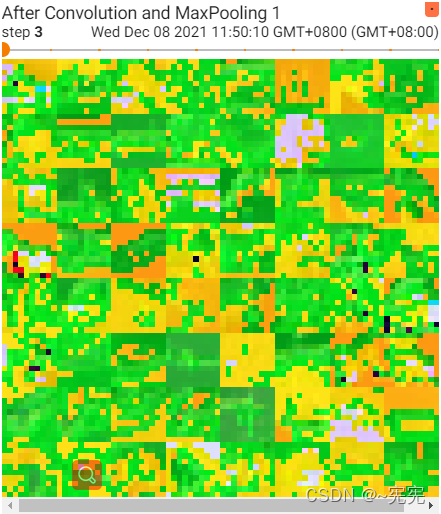
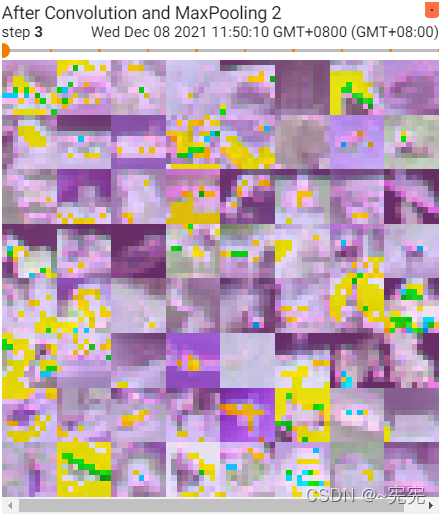
4.torch.nn的非线性激活函数
torch.nn.Sigmoid(x) Sigmoid激活函数 torch.nn.Tanh() Tanh激活函数 torch.nn.ReLU(inplace=False) ReLU激活函数 inplace() 是否原地替换,inplace=True 表示原地替换,default=False torch.nn.Softmax(dim=None) Softmax函数 dim (int) Softmax 将会沿着 dim 指定的维度进行计算,因此沿着 dim 的每个切片的总和为
1
1
1
5.torch.nn的正则化层
torch.nn.BatchNorm2d(num_features, eps=1e-05, momentum=0.1, affine=True, track_running_stats=True, device=None, dtype=None) 正则化 论文地址:Batch Normalization: Accelerating Deep Network Training by Reducing Internal Covariate Shift num_features (int) 通道数的数量,即输入
(
N
,
C
,
H
,
W
)
(N,C,H,W)
(N,C,H,W) 中的
C
C
C eps (float) 正则化公式中为保证数值稳定性而加在分母上加的
?
\epsilon
? 则,Default=
1
×
1
0
?
5
\tt 1×10^{-5}
1×10?5 momentum 用于 running_mean 和 running_var 计算的值,default=0.1 affine (bool) 若 affine=True,该模块具有可学习的仿射参数,Default=True track_running_stats (bool) 若 track_running_stats=True,该模块跟踪运行平均值 running_mean 和方差 running_var,若 track_running_stats=False,该模块不跟踪此类统计,并初始化统计缓冲区 running_mean 和running_var 为 None,当这些缓冲区为 None 时,训练和测试两种模式中该模块总是使用批处理统计信息,default=True
正则化层的类较多,这里不再作详细的说明,详情查阅 PyTorch 官网文档或相关论文
6.torch.nn的线性层
object = torch.nn.Linear(in_features, out_features, bias=True, device=None, dtype=None) 神经网络中的一层,即单层感知机 in_features (int) 输入特征数量 out_features (int) 输出特征数量 bias(bool) 是否学习偏差,若 bias=True,则该层具有偏差
b
(
l
)
b^{(l)}
b(l),若 bias=False 则不具有偏差,default=True 具有的属性: object.weight 该层的权重,初值的选取符合一定的分布 object.bias 该层的偏差,初值的选取符合一定的分布
7.torch.nn的Dropout层
torch.nn.Dropout(p=0.5, inplace=False) 在训练过程中(训练模式),根据伯努利分布,将输入张量中的某些元素以概率 p 随机失活(归零),是一种防止过拟合的手段 p (float) 一个元素失活的概率,default=0.5 inplace (bool) 是否原地替换,inplace=True 表示原地替换,default=False
二、案例演示:搭建CIFAR10分类网络
1.网络结构
层级 | 形状 |
---|
I
n
p
u
t
s
\tt Inputs
Inputs |
(
3
,
32
,
32
)
(3,32,32)
(3,32,32) |
C
o
n
v
o
l
u
t
i
o
n
(
5
×
5
)
\tt Convolution(5×5)
Convolution(5×5) |
(
3
,
32
,
32
)
?
(
32
,
32
,
32
)
(3,32,32)\Longrightarrow(32,32,32)
(3,32,32)?(32,32,32) |
M
a
x
P
o
o
l
i
n
g
(
2
×
2
)
\tt MaxPooling(2×2)
MaxPooling(2×2) |
(
32
,
32
,
32
)
?
(
32
,
16
,
16
)
(32,32,32)\Longrightarrow(32,16,16)
(32,32,32)?(32,16,16) |
C
o
n
v
o
l
u
t
i
o
n
(
5
×
5
)
\tt Convolution(5×5)
Convolution(5×5) |
(
32
,
16
,
16
)
?
(
32
,
16
,
16
)
(32,16,16)\Longrightarrow(32,16,16)
(32,16,16)?(32,16,16) |
M
a
x
P
o
o
l
i
n
g
(
2
×
2
)
\tt MaxPooling(2×2)
MaxPooling(2×2) |
(
32
,
16
,
16
)
?
(
32
,
8
,
8
)
(32,16,16)\Longrightarrow(32,8,8)
(32,16,16)?(32,8,8) |
C
o
n
v
o
l
u
t
i
o
n
(
5
×
5
)
\tt Convolution(5×5)
Convolution(5×5) |
(
32
,
8
,
8
)
?
(
64
,
8
,
8
)
(32,8,8)\Longrightarrow(64,8,8)
(32,8,8)?(64,8,8) |
M
a
x
P
o
o
l
i
n
g
(
2
×
2
)
\tt MaxPooling(2×2)
MaxPooling(2×2) |
(
64
,
8
,
8
)
?
(
64
,
4
,
4
)
(64,8,8)\Longrightarrow(64,4,4)
(64,8,8)?(64,4,4) |
F
l
a
t
t
e
n
\tt Flatten
Flatten |
(
64
,
4
,
4
)
?
(
1
,
1
,
1024
)
(64,4,4)\Longrightarrow(1,1,1024)
(64,4,4)?(1,1,1024) |
F
u
l
l
y
?
c
o
n
n
e
c
t
e
d
\tt Fully \ connected
Fully?connected |
(
1
,
1
,
1024
)
?
(
64
)
(1,1,1024)\Longrightarrow(64)
(1,1,1024)?(64) |
R
e
L
U
\tt ReLU
ReLU |
(
64
)
?
(
64
)
(64)\Longrightarrow(64)
(64)?(64) |
F
u
l
l
y
?
c
o
n
n
e
c
t
e
d
\tt Fully \ connected
Fully?connected |
(
64
)
?
(
10
)
(64)\Longrightarrow(10)
(64)?(10) |
S
o
f
t
m
a
x
\tt Softmax
Softmax |
(
10
)
?
(
10
)
(10)\Longrightarrow(10)
(10)?(10) |
注意:现在已不使用
5
×
5
5×5
5×5 的卷积核了,而是使用两个
3
×
3
3×3
3×3 的卷积核来代替一个
5
×
5
5×5
5×5 的卷积核,从而减少参数量,或者使用两个
1
×
1
1×1
1×1 的卷积核和一个
3
×
3
3×3
3×3 的卷积核,此处仅当练习使用
2.构建模型
import torch
import torch.nn as nn
import torchvision
from torchvision import transforms
from torch.utils.tensorboard import SummaryWriter
from torch.utils.data import DataLoader
class Model(nn.Module):
def __init__(self):
super(Model, self).__init__()
self.conv1 = nn.Conv2d(3, 32, 5, padding=2)
self.maxpool1 = nn.MaxPool2d(2)
self.conv2 = nn.Conv2d(32, 32, 5, padding=2)
self.maxpool2 = nn.MaxPool2d(2)
self.conv3 = nn.Conv2d(32, 64, 5, padding=2)
self.maxpool3 = nn.MaxPool2d(2)
self.flatten = nn.Flatten()
self.linear1 = nn.Linear(1024, 64)
self.relu1 = nn.ReLU()
self.linear2 = nn.Linear(64, 10)
self.softmax1 = nn.Softmax()
def forward(self, x):
x = self.conv1(x)
x = self.maxpool1(x)
x = self.conv2(x)
x = self.maxpool2(x)
x = self.conv3(x)
x = self.maxpool3(x)
x = self.flatten(x)
x = self.linear1(x)
x = self.relu(x)
x = self.linear2(x)
y = self.softmax(x)
return y
3.torch.nn.Sequential——网络的容器
torch.nn.Sequential 是一个网络的容器,在构造网络时,可以按网络的传递顺序来将 torch.nn 各层添加到容器中,Sequential 的forward() 方法接受任何输入并将其转发给 Sequential 容器内的第一个模块,然后,它将随后每个模块的输出按顺序 “链接” 到输入,最后返回最后一个模块的输出
容器可以将多个层存放在一起,增强代码复用性与高效性
torch.nn.Sequential(*args) 构造一个容器,可将若干个 torch.nn 对象添加到容器中 *args 若干个顺序 torch.nn 对象 此外,容器 Sequential 还可以嵌套 Sequential 容器,灵活运用可以使编码更高效
4.利用torch.nn.Sequential简化网络
import torch
import torch.nn as nn
import torchvision
from torchvision import transforms
from torch.utils.tensorboard import SummaryWriter
from torch.utils.data import DataLoader
class Model(nn.Module):
def __init__(self):
super(Model, self).__init__()
self.model1 = nn.Sequential(
nn.Conv2d(3, 32, 5, padding=2),
nn.MaxPool2d(2),
nn.Conv2d(32, 32, 5, padding=2),
nn.MaxPool2d(2),
nn.Conv2d(32, 64, 5, padding=2),
nn.MaxPool2d(2),
nn.Flatten(),
nn.Linear(1024, 64),
nn.ReLU(),
nn.Linear(64, 10),
nn.Softmax(),
)
def forward(self, x):
y = self.model1(x)
return y
5.利用tensorboard可视化网络
add_graph(model, input_to_model=None, verbose=False, use_strict_trace=True) 向 tensorboard 日志中添加一个网络可视化图 model 实例化后的 PyTorch 模型 input_to_model (torch.Tensor or list of torch.Tensor) 要输入模型的张量或张量元组,可以是
(
N
,
C
,
H
,
W
)
(N,C,H,W)
(N,C,H,W) verbose (bool) 是否在控制台打印图像结构,若 verbose=True,则打印在控制台,default=False
writer = SummaryWriter("logs")
model = Model()
input = torch.ones((64, 3, 32, 32))
writer.add_graph(model, input)
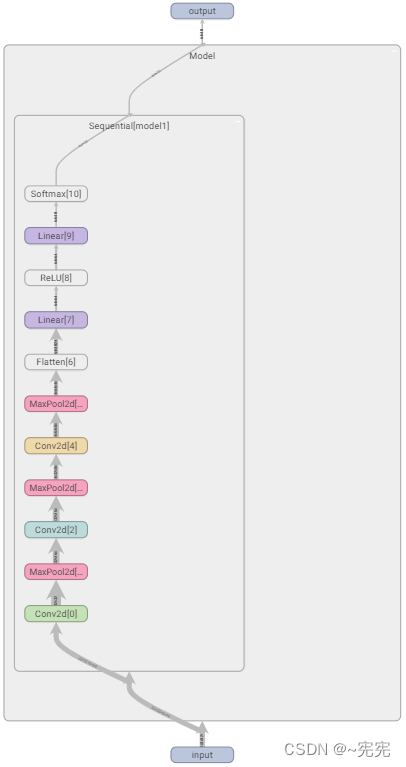
参考资料: [1]PyTorch深度学习快速入门教程(绝对通俗易懂!)【小土堆】 [2]PyTorch官网文档
|