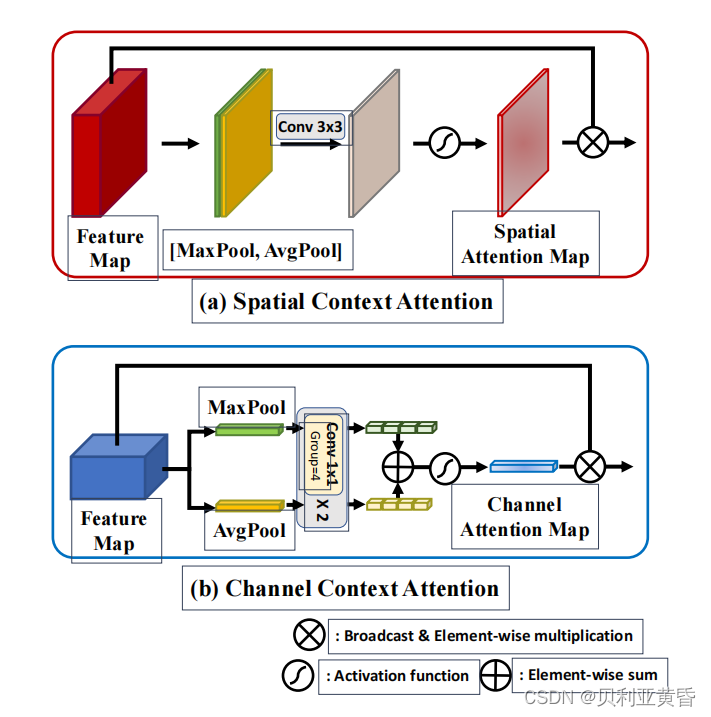
自己在查阅文献的时候,发现了这篇文章(SpaceMeshLab_Spatial_Context_Memoization_And_Meshgrid_Atrous_Convolution_Consensus_For_Semantic_Segmentation),觉得里面提到的SCA和CCA模块很有意思,自己尝试复现出来了,即插即用的模块,用在我的方法中,提点很明显。
import torch
from torchvision import models
import torch.nn as nn
import math
import torch.utils.model_zoo as model_zoo
from torch.nn import functional as F
import warnings
class SCA(torch.nn.Module):
def __init__(self, in_channels, out_channels):
super().__init__()
self.in_channels = in_channels
self.out_channels = out_channels
self.maxpooling1 = nn.AdaptiveMaxPool2d(output_size=(16, 16))
self.avgpooling1 = nn.AdaptiveAvgPool2d(output_size=(16, 16))
self.conv1 = nn.Conv2d(self.in_channels*2, self.in_channels, kernel_size=3, stride=1, padding=1)
self.bn1 = nn.BatchNorm2d(self.in_channels)
self.conv2 = nn.Conv2d(self.in_channels*2, self.out_channels, kernel_size=3, stride=1, padding=1)
self.bn2 = nn.BatchNorm2d(out_channels)
def forward(self, input):
'''
input : B X in_channels X H X W
return
result : B X out_channels X H X W
'''
max_x = self.maxpooling1(input)
avg_x = self.avgpooling1(input)
x = torch.cat((max_x, avg_x), dim=1)
x = F.relu(self.bn1(self.conv1(x)))
result = torch.cat((input, x), dim=1)
result = F.relu(self.bn2(self.conv2(result)))
return result
class CCA(torch.nn.Module):
def __init__(self, in_channels, out_channels):
super().__init__()
self.in_channels = in_channels
self.out_channels = out_channels
self.maxpooling1 = nn.AdaptiveMaxPool2d(output_size=(1, 1))
self.avgpooling1 = nn.AdaptiveAvgPool2d(output_size=(1, 1))
self.conv1 = nn.Conv2d(self.in_channels, self.in_channels, kernel_size=1)
self.bn1 = nn.BatchNorm2d(self.in_channels)
self.conv2 = nn.Conv2d(self.in_channels, self.in_channels, kernel_size=1)
self.bn2 = nn.BatchNorm2d(self.in_channels)
self.conv3 = nn.Conv2d(self.in_channels*2, self.out_channels, kernel_size=1)
self.bn3 = nn.BatchNorm2d(self.out_channels)
def forward(self, input):
'''
input : B X in_channels X H X W
return
result : B X out_channels X H X W
'''
max_x = self.maxpooling1(input)
avg_x = self.avgpooling1(input)
max_x = F.relu(self.bn1(self.conv1(max_x)))
avg_x = F.relu(self.bn2(self.conv2(avg_x)))
encode = torch.cat((max_x, avg_x), dim=1)
encode = F.relu(self.bn3(self.conv3(encode)))
result = torch.mul(input, encode)
return result
|