实现基于logistic/softmax regression的文本分类
-
参考
- 文本分类
- 《神经网络与深度学习》 第2/3章
-
数据集:Classify the sentiment of sentences from the Rotten Tomatoes dataset -
实现要求:NumPy -
需要了解的知识点:
- 文本特征表示:Bag-of-Word,N-gram
- 分类器:logistic/softmax regression,损失函数、(随机)梯度下降、特征选择
- 数据集:训练集/验证集/测试集的划分
-
实验:
- 分析不同的特征、损失函数、学习率对最终分类性能的影响
- shuffle 、batch、mini-batch
-
时间:两周
文本特征表示方法一:Bag of Words
import numpy as np
class BagofWords:
def __init__(self, do_lower_case=False):
self.vocab = {}
self.do_lower_case = do_lower_case
def fit_transform(self, sent_list):
for sent in sent_list:
if self.do_lower_case:
sent = sent.lower()
words = sent.strip().split(" ")
for word in words:
if word not in self.vocab:
self.vocab[word] = len(self.vocab)
vocab_size = len(self.vocab)
bag_of_words_features = np.zeros((len(sent_list), vocab_size))
for idx, sent in enumerate(sent_list):
if self.do_lower_case:
sent = sent.lower()
words = sent.strip().split(" ")
for word in words:
bag_of_words_features[idx][self.vocab[word]] += 1
return bag_of_words_features
文本特征表示方法二:n-gram
import numpy as np
class Ngram:
def __init__(self, ngram, do_lower_case=False):
self.ngram = ngram
self.feature_map = {}
self.do_lower_case = do_lower_case
def fit_transform(self, sent_list):
for gram in self.ngram:
for sent in sent_list:
if self.do_lower_case:
sent = sent.lower()
sent = sent.split(" ")
for i in range(len(sent) - gram + 1):
feature = "_".join(sent[i:i + gram])
if feature not in self.feature_map:
self.feature_map[feature] = len(self.feature_map)
n = len(sent_list)
m = len(self.feature_map)
ngram_feature = np.zeros((n, m))
for idx, sent in enumerate(sent_list):
if self.do_lower_case:
sent = sent.lower()
sent = sent.split(" ")
for gram in self.ngram:
for i in range(len(sent) - gram + 1):
feature = "_".join(sent[i:i + gram])
if feature in self.feature_map:
ngram_feature[idx][self.feature_map[feature]] = 1
return ngram_feature
模型
计算loss function对于系数w的梯度,来源自nndl 
import numpy as np
import pandas as pd
class softmax_regression():
def __init__(self, epochs = 10, learning_rate = 0.01):
self.batch_size = None
self.num_features = None
self.w = None
self.learning_rate = learning_rate
self.num_classes = None
self.epochs = epochs
def fit(self, X, y, learning_rate=0.01, epochs=10, num_classes=5):
'''
:param X: [batch_size, num_features]
:param y: [batch_size, 1]
:param w: [num_classes, num_features]
:return:
'''
self.__init__(epochs, learning_rate=learning_rate)
self.batch_size, self.num_features = X.shape
self.num_classes = num_classes
self.w = np.random.randn(self.num_classes, self.num_features)
y_one_hot = np.zeros((self.batch_size, self.num_classes))
for i in range(self.batch_size):
y_one_hot[i][y[i]] = 1
loss_history = []
for i in range(epochs):
loss = 0
probs = X.dot(self.w.T)
probs = softmax(probs)
for i in range(self.batch_size):
loss -= np.log(probs[i][y[i]])
weight_update = np.zeros_like(self.w)
for i in range(self.batch_size):
weight_update += X[i].reshape(1, self.num_features).T.dot((y_one_hot[i] - probs[i]).reshape(1, self.num_classes)).T
self.w += weight_update * self.learning_rate / self.batch_size
loss /= self.batch_size
loss_history.append(loss)
if i % 10 == 0:
print("epoch {} loss {}".format(i, loss))
return loss_history
def predict(self, X):
prob = softmax(X.dot(self.w.T))
return prob.argmax(axis=1)
def score(self, X, y):
pred = self.predict(X)
return np.sum(pred.reshape(y.shape) == y) / y.shape[0]
def sigmoid(z):
return 1 / (1 + np.exp(-z))
def softmax(z):
z -= np.max(z, axis=1, keepdims=True)
z = np.exp(z)
z /= np.sum(z, axis=1, keepdims=True)
return z
主函数
import numpy as np
import pandas as pd
from BagofWords import BagofWords
from Ngram import Ngram
from sklearn.model_selection import train_test_split
from model import softmax_regression
import matplotlib.pyplot as plt
if __name__ == '__main__':
train_df = pd.read_csv('./data/train.tsv', sep='\t')
X_data, y_data = train_df["Phrase"].values, train_df["Sentiment"].values
just_test = 0
if just_test == 1:
X_data = X_data[:10000]
y_data = y_data[:10000]
y = np.array(y_data).reshape((-1, 1))
bag_of_words = BagofWords()
Ngram = Ngram(ngram=(1, 2))
X_Bow = bag_of_words.fit_transform(X_data)
X_Gram = Ngram.fit_transform(X_data)
X_train_Bow, X_test_Bow, y_train_Bow, y_test_Bow = train_test_split(X_Bow, y, test_size=0.2, random_state=42, stratify=y)
X_train_Gram, X_test_Gram, y_train_Gram, y_test_Gram = train_test_split(X_Gram, y, test_size=0.2, random_state=42, stratify=y)
epochs = 100
bow_learning_rate = 1
gram_learning_rate = 1
model1 = softmax_regression()
history = model1.fit(X_train_Bow, y_train_Bow, epochs=epochs, learning_rate=bow_learning_rate)
plt.title('Bag of words')
plt.plot(np.arange(len(history)), np.array(history))
plt.show()
print("Bow train {} test {}".format(model1.score(X_train_Bow, y_train_Bow), model1.score(X_test_Bow, y_test_Bow)))
model2 = softmax_regression()
history = model2.fit(X_train_Gram, y_train_Gram, epochs=epochs, learning_rate=gram_learning_rate)
plt.title('N-Gram')
plt.plot(np.arange(len(history)), np.array(history))
plt.show()
print("Gram train {} test {}".format(model2.score(X_train_Gram, y_train_Gram),
model2.score(X_test_Gram, y_test_Gram)))
training data = 20000 epochs = 100
实验结果:
Bow train 0.25275 test 0.2545
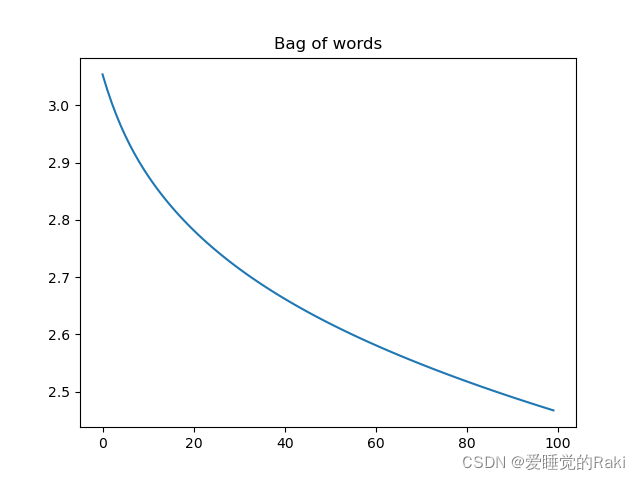
Gram train 0.2644375 test 0.262
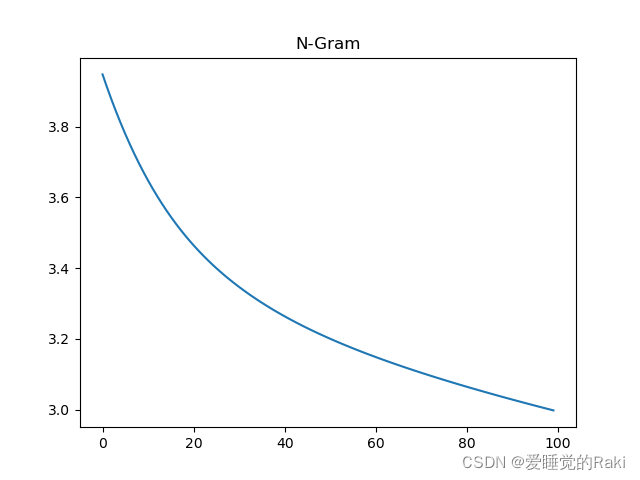
|