Pandas(Python Data Analysis Library)是基于NumPy的数据分析模块,它提供了大量标准数据模型和高效操作大型数据集所需的工具,可以说Pandas是使得Python能够成为高效且强大的数据分析环境的重要因素之一。 导入方式:import pandas as pd
? ? Pandas有三种数据结构:Series、DataFrame和Panel。Series类似于一维数组;DataFrame是类似表格的二维数组;Panel可以视为Excel的多表单Sheet
? ? ? Series 是一种一维数组对象,包含了一个值序列,并且包含了数据标签,称为索引(index),可通过索引来访问数组中的数据。
pd.Series(data=None, index=None, dtype=None, name=None, copy=False, fastpath=False)
import pandas as pd
a=pd.Series([5,6,7,8])
print(a)
0 5
1 6
2 7
3 8
dtype: int64
创建Series时指定索引
import pandas as pd
i=["a","b","c","a","b"]
v=[4,5,6,4,6]
t=pd.Series(v,index=i,name="lll")
print(t)
输出:
a 4
b 5
c 6
a 4
b 6
Name: lll, dtype: int64
可以有重复的索引,重复的键值对
尽管创建Series指定了index参数,实际Pandas还是有隐藏的index位置信息的。所以Series有两套描述某条数据的手段:位置和标签
import pandas as pd
val=[2,4,5,6]
idx1=range(10,14)
idx2="hello the cruel world".split()
s0=pd.Series(val)
s1=pd.Series(val,index=idx1)
t=pd.Series(val,index=idx2)
print(s0.index)
print(s1.index)
print(t.index)
print(s0[0])
print(s1[10])
print(t[0],t["hello"])
输出:
RangeIndex(start=0, stop=4, step=1)
RangeIndex(start=10, stop=14, step=1)
Index(['hello', 'the', 'cruel', 'world'], dtype='object')
2
2
2 2
如果数据被存放在一个Python字典中,也可以直接通过这个字典来创建Series。
sdata = {'Ohio': 35000, 'Texas': 71000, 'Oregon': 16000, 'Utah': 5000}
obj3 = pd.Series(sdata)
print(obj3)
Ohio 35000
Texas 71000
Oregon 16000
Utah 5000
dtype: int64
【例4-6】键值和指定的索引不匹配
sdata = {"a" : 100, "b" : 200, "e" : 300}
letter = ["a", "b","c" , "e" ]
obj = pd.Series(sdata, index = letter)
print(obj)
a 100.0
b 200.0
c NaN
e 300.0
dtype: float64
sdata = {'Ohio': 35000, 'Texas': 71000, 'Oregon': 16000, 'Utah': 5000}
obj1 = pd.Series(sdata)
states = ['California', 'Ohio', 'Oregon', 'Texas']
obj2 = pd.Series(sdata, index = states)
print(obj1+obj2)
California NaN
Ohio 70000.0
Oregon 32000.0
Texas 142000.0
Utah NaN
dtype: float64
obj = pd.Series([4,7,-3,2])
obj.index = ['Bob', 'Steve', 'Jeff', 'Ryan']
print(obj)
Bob 4
Steve 7
Jeff -3
Ryan 2
dtype: int64
? ? ? DataFrame是一个表格型的数据结构,它含有一组有序的列,每列可以是不同的值类型(数值、字符串、布尔值等)。 ? ? ? DataFrame既有行索引也有列索引,它可以被看做由Series组成的字典(共用同一个索引)
DataFrame的创建 格式: pd.DataFrame(data=None, index=None, columns=None, dtype=None, copy=False)
data = {
'name':['张三', '李四', '王五', '小明'],
'sex':['female', 'female', 'male', 'male'],
'year':[2001, 2001, 2003, 2002],
'city':['北京', '上海', '广州', '北京']
}
df = pd.DataFrame(data)
print(df)
name sex year city
0 张三 female 2001 北京
1 李四 female 2001 上海
2 王五 male 2003 广州
3 小明 male 2002 北京
df3 = pd.DataFrame(data, columns = ['name', 'sex', 'year', 'city'], index = ['a', 'b', 'c', 'd'])
print(df3)
name sex year city
a 张三 female 2001 北京
b 李四 female 2001 上海
c 王五 male 2003 广州
d 小明 male 2002 北京
函数 | 返回值 | values | 元素 | index | 索引 | columns | 列名 | dtypes | 类型 | size | 元素个数 | ndim | 维度数 | shape | 数据形状(行列数目) |
?? ? ? Pandas的索引对象负责管理轴标签和其他元数据(比如轴名称等)。构建Series或 DataFrame时,所用到的任何数组或其他序列的标签都会被转换成一个Index。
print(df)
print(df.index)
print(df.columns)
name sex year city
a 张三 female 2001 北京
b 李四 female 2001 上海
c 王五 male 2003 广州
d 小明 male 2002 北京
Index(['a', 'b', 'c', 'd'], dtype = 'object')
Index(['name', 'sex', 'year', 'city'], dtype = 'object')
print('name' in df.columns)
print(‘f' in df.index)
True
False
每个索引都有一些方法和属性,它们可用于设置逻辑并回答有关该索引所包含的数据的常见问题。Index的常用方法和属性见表4-1。
方法 | 说明 | append | 连接另一个Index对象,产生一个新的Index | diff | 计算差集,并得到一个Index | intersection | 计算交集 | union | 计算并集 | isin | 计算一个指示各值是否都包含在参数集合中的布尔型数组 | delete | 删除索引i处的元素,并得到新的Index | drop | 删除传入的值,并得到新的Index | insert | 将元素插入到索引i处,并得到新的Index | is_monotonic | 当各元素均大于等于前一个元素时,返回True | is.unique | 当Index没有重复值时,返回True | unique | 计算Index中唯一值的数组 |
?
df=pd.DataFrame(data)
print(df)
print(df.values)
print(df.columns)
print(df.size)
print(df.ndim)
print(df.shape)
输出:
name sex year city
0 张三 female 2001 北京
1 李四 female 2001 上海
2 王五 male 2003 广州
3 小明 male 2002 北京
[['张三' 'female' 2001 '北京']
['李四' 'female' 2001 '上海']
['王五' 'male' 2003 '广州']
['小明' 'male' 2002 '北京']]
Index(['name', 'sex', 'year', 'city'], dtype='object')
16
2
(4, 4)
索引对象是无法修改的,因此,重新索引是指对索引重新排序而不是重新命名,如果某个索引值不存在的话,会引入缺失值
import pandas as pd
a=pd.Series([2,4,5,6],index=['b','a','d','c'])
print(a)
a.reindex(['b','a','d','c','e'])
print(a)
b 2
a 4
d 5
c 6
dtype: int64
b 2.0
a 4.0
d 5.0
c 6.0
e NaN
dtype: float64
import pandas as pd
a=pd.Series([2,4,5,6],index=['b','a','d','c'])
print(a)
b=a.reindex(['b','a','d','c','e'],fill_value=0)
print(b)
b 2
a 4
d 5
c 6
dtype: int64
b 2
a 4
d 5
c 6
e 0
dtype: int64
对于顺序数据,比如时间序列,重新索引时可能需要进行插值或填值处理,利用参数method选项可以设置: method = ‘ffill’或‘pad’,表示前向值填充 ? ? ? ? ? ? method = ‘bfill’或‘backfill’,表示后向值填充
import pandas as pd
import numpy as np
a=pd.Series(['blue','red','black'],index=[0,2,4])
b=a.reindex(np.arange(6),method='ffill')
print(b)
0 blue
1 blue
2 red
3 red
4 black
5 black
dtype: object
import pandas as pd
import numpy as np
a=pd.Series(['blue','red','black'],index=[0,2,4])
b=a.reindex(np.arange(6),method='backfill')
print(b)
输出:
0 blue
1 red
2 red
3 black
4 black
5 NaN
dtype: object
df4 = pd.DataFrame(np.arange(9).reshape(3,3),
index = ['a','c','d'],columns = ['one','two','four'])
print(df4)
one two four
a 0 1 2
c 3 4 5
d 6 7 8
import pandas as pd
import numpy as np
df4=pd.DataFrame(np.arange(9).reshape(3,3),index=['a','b','c'],columns=['one','two','four'])
df4=df4.reindex(index=['a','b','c','d'],columns=['one','two','three','four'])
print(df4)
one two three four
a 0.0 1.0 NaN 2.0
b 3.0 4.0 NaN 5.0
c 6.0 7.0 NaN 8.0
d NaN NaN NaN NaN
import pandas as pd
import numpy as np
df4=pd.DataFrame(np.arange(9).reshape(3,3),index=['a','b','c'],columns=['one','two','four'])
df4=df4.reindex(index=['a','b','c','d'],columns=['one','two','three','four'],fill_value=2)
print(df4)
one two three four
a 0 1 2 2
b 3 4 2 5
c 6 7 2 8
d 2 2 2 2
传入fill_value = n用n代替缺失值
reindex函数参数
参数 | 使用说明 | index | 用于索引的新序列? | method | 插值(填充)方式 | fill_value | 缺失值替换值 | limit | 最大填充量 | level ??????? copy | 在Multiindex的指定级别上匹配简单索引,否则选取其子集 默认为True,无论如何都复制;如果为False,则新旧相等时就不复制 |
?如果不希望使用默认的行索引,则可以在创建的时候通过Index参数来设置。 在DataFrame数据中,如果希望将列数据作为索引,则可以通过set_index方法来实现。 ?
df5?=?df1.set_index('city')print(df5)
city name year sex
北京 张三 2001 female
上海 李四 2001 female
广州 王五 2003 male
北京 小明 2002 male
?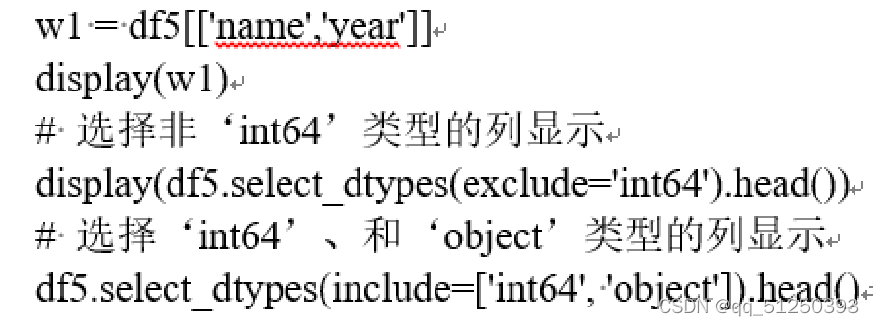
?选取通过DataFrame提供的head和tail方法可以得到多行数据,但是用这两种方法得到的数据都是从开始或者末尾获取连续的数据, 而利用sample可以随机抽取数据并显示。
head() #默认获取前5行
head(n)#获取前n行
tail()#默认获取后5行
head(n)#获取后n行
sample(n)#随机抽取n行显示
sample(frac=0.6) ? ? #随机抽取60%的行
?选取行和列 DataFrame.loc(行索引名称或条件,列索引名称) DataFrame.iloc(行索引位置,列索引位置)
import pandas as pd
import numpy as np
df4=pd.DataFrame(np.arange(9).reshape(3,3),index=['a','b','c'],columns=['one','two','four'])
df4=df4.reindex(index=['a','b','c','d'],columns=['one','two','three','four'],fill_value=2)
print(df4.loc[:,['one','two']])
print(df4.loc[['a','b'],['one','two']])
print(df4.loc[df4['one']>1,['two','three']])
one two
a 0 1
b 3 4
c 6 7
d 2 2
one two
a 0 1
b 3 4
two three
b 4 2
c 7 2
d 2 2
import pandas as pd
import numpy as np
df4=pd.DataFrame(np.arange(9).reshape(3,3),index=['a','b','c'],columns=['one','two','four'])
df4=df4.reindex(index=['a','b','c','d'],columns=['one','two','three','four'],fill_value=2)
print(df4.iloc[:,1])
print(df4.iloc[[1,3]])
print(df4.iloc[[1,3],[1,2]])
out:
a 1
b 4
c 7
d 2
Name: two, dtype: int32
one two three four
b 3 4 2 5
d 2 2 2 2
two three
b 4 2
d 2 2
DataFrame行和列的选取还可以通过Pandas的query方法实现。 用法:
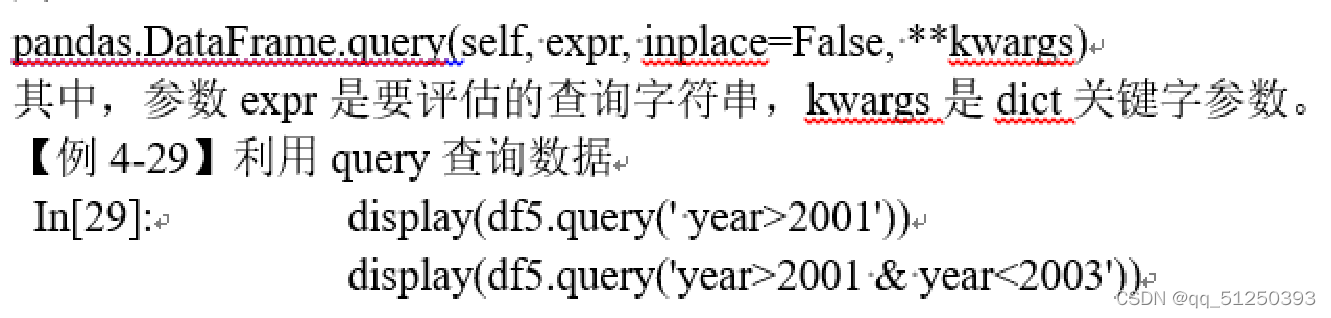
?布尔选择 ? ? 可以对DataFrame中的数据进行布尔方式选择
?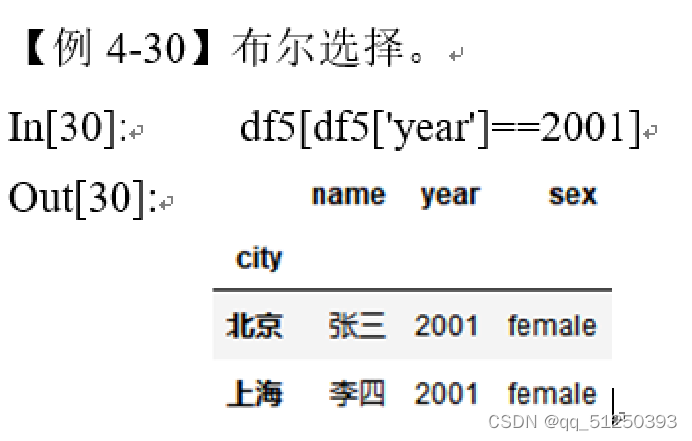
?
import pandas as pd
import numpy as np
df4=pd.DataFrame(np.arange(9).reshape(3,3),index=['a','b','c'],columns=['one','two','four'])
df4=df4.reindex(index=['a','b','c','d'],columns=['one','two','three','four'],fill_value=2)
print(df4['two']==2)
print(df4[df4['two']==2])
out:
a False
b False
c False
d True
Name: two, dtype: bool
one two three four
d 2 2 2 2
import pandas as pd
import numpy as np
df4=pd.DataFrame(np.arange(9).reshape(3,3),index=['a','b','c'],columns=['one','two','four'])
df4=df4.reindex(index=['a','b','c','d'],columns=['one','two','three','four'],fill_value=2)
data={'one':11,'two':12,'three':13,'four':14}
print(df4.append(data,ignore_index=True))
data={'one':11,'two':12,'three':13}
print(df4.append(data,ignore_index=True))
out:
one two three four
0 0 1 2 2
1 3 4 2 5
2 6 7 2 8
3 2 2 2 2
4 11 12 13 14
one two three four
0 0.0 1.0 2.0 2.0
1 3.0 4.0 2.0 5.0
2 6.0 7.0 2.0 8.0
3 2.0 2.0 2.0 2.0
4 11.0 12.0 13.0 NaN
print(df4.append(data,ignore_index=False))
报错TypeError: Can only append a dict if ignore_index=True
增加列时,只需为要增加的列赋值即可创建一个新的列。若要指定新增列的位置,可以用insert函数。
import pandas as pd
import numpy as np
df4=pd.DataFrame(np.arange(9).reshape(3,3),index=['a','b','c'],columns=['one','two','four'])
df4=df4.reindex(index=['a','b','c','d'],columns=['one','two','three','four'],fill_value=2)
data={'one':11,'two':12,'three':13,'four':14}
df4['five']=[22,23,24,25]
df4.insert(1,'NN',['001','002','003','004'])
print(df4)
out:
one NN two three four five
a 0 001 1 2 2 22
b 3 002 4 2 5 23
c 6 003 7 2 8 24
d 2 004 2 2 2 25
2. 删除数据 ? ? 删除数据直接用drop方法,通过axis参数确定是删除的是行还是列。默认数据删除不修改原数据,需要在原数据删除行列需要设置参数inplace = True。
import pandas as pd
import numpy as np
df4=pd.DataFrame(np.arange(9).reshape(3,3),index=['a','b','c'],columns=['one','two','four'])
df4=df4.reindex(index=['a','b','c','d'],columns=['one','two','three','four'],fill_value=2)
data={'one':11,'two':12,'three':13,'four':14}
print(df4.drop('four',axis=1))
print(df4.drop('a'))#默认axis=0
out:
one two three
a 0 1 2
b 3 4 2
c 6 7 2
d 2 2 2
one two three four
b 3 4 2 5
c 6 7 2 8
d 2 2 2 2
3. 修改数据 ? ? 修改数据时直接对选择的数据赋值即可。 ? ? 需要注意的是,数据修改是直接对DataFrame数据修改,操作无法撤销,因此更改数据时要做好数据备份。
|