PyCaret是一个开源、低代码的Python机器学习库,可自动执行机器学习工作流。它是一种端到端的机器学习和模型管理工具,可以以指数方式加快实验周期并提高您的工作效率。
与其他开源机器学习库相比,PyCaret是一个替代的低代码库,可用于仅用几行代码替换数百行代码。这使得实验速度和效率呈指数级增长。喜欢欢迎关注、收藏、点赞。
PyCaret本质上是围绕多个机器学习库和框架(例如 scikit-learn、XGBoost、LightGBM、CatBoost、spaCy、Optuna、Hyperopt、Ray 等的Python包装器。
PyCaret时间序列模块
PyCaret的新时间序列模块现已提供测试版。秉承 PyCaret 的简单性,它与现有的 API 保持一致,并带有很多功能:模型训练和选择(30多种算法)、模型分析、自动超参数调优、实验记录、云部署等。
pip install pycaret-ts-alpha
使用样例
PyCaret的时间序列模块中的工作流程非常简单。它从setup您定义预测范围,然后设置使用函数训练和评估多种算法。
加载数据
import pandas as pd
from pycaret.datasets import get_data
data = get_data('pycaret_downloads')
data['Date'] = pd.to_datetime(data['Date'])
data = data.groupby('Date').sum()
data = data.asfreq('D')
data.head()
data.plot()
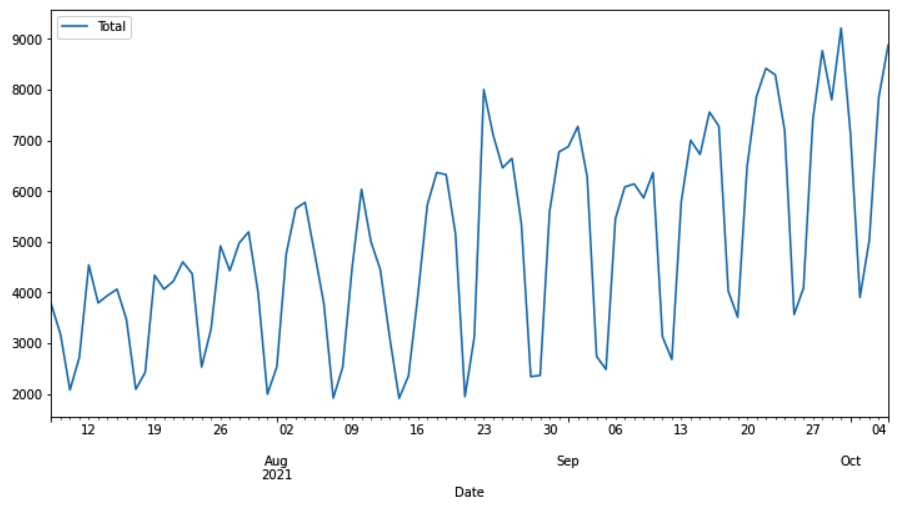
初始化设置
from pycaret.time_series import *
setup(data, fh = 7, fold = 3, session_id = 123)
from pycaret.internal.pycaret_experiment import TimeSeriesExperiment
exp = TimeSeriesExperiment()
exp.setup(data, fh = 7, fold = 3, session_id = 123)
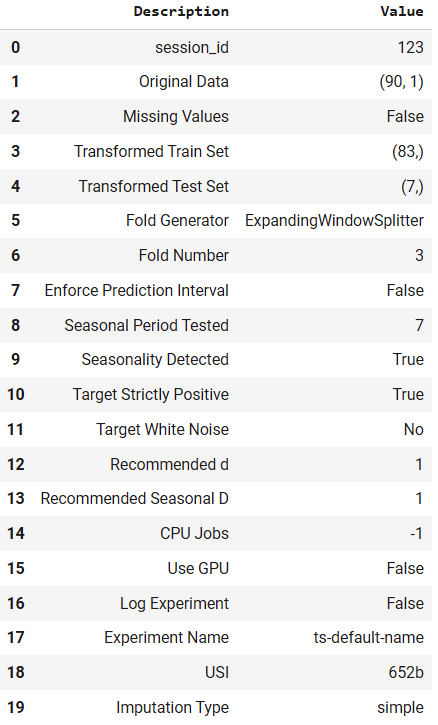
统计测试
check_stats()
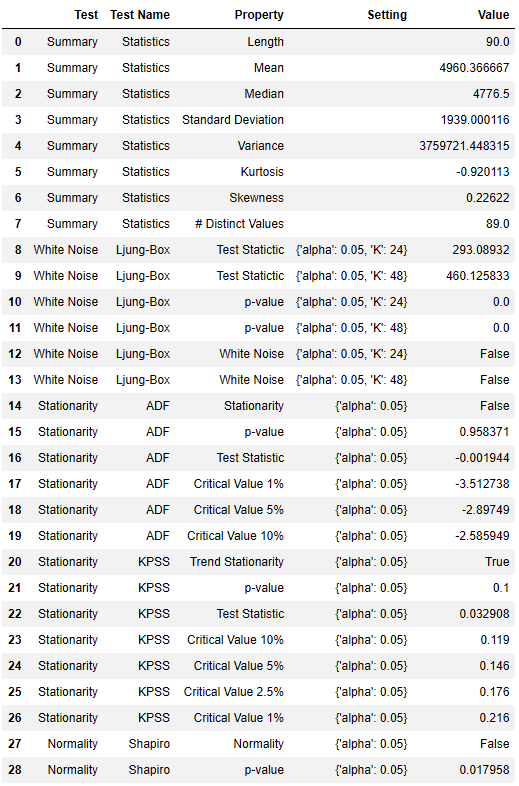
探索性数据分析
plot_model(plot = 'ts')
exp.plot_model(plot = 'ts')
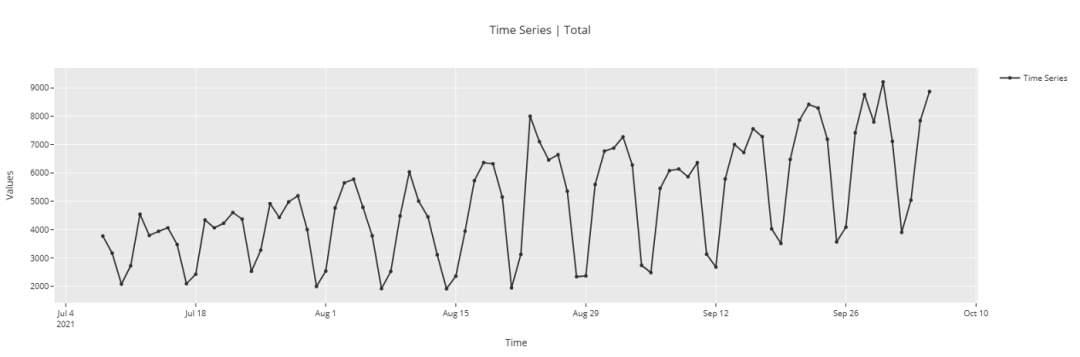
plot_model(plot = 'cv')
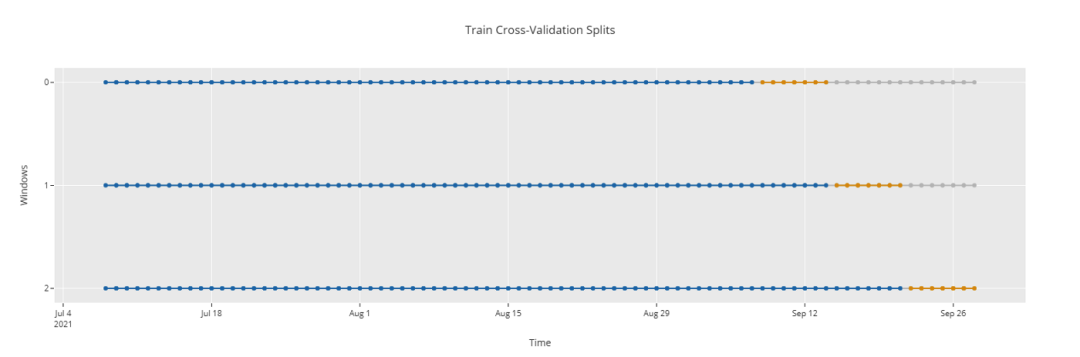
plot_model(plot = 'acf')
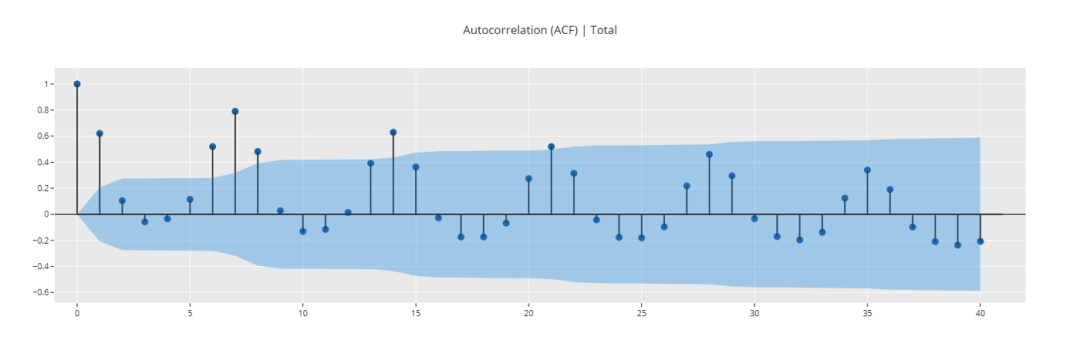
plot_model(plot = 'diagnostics')
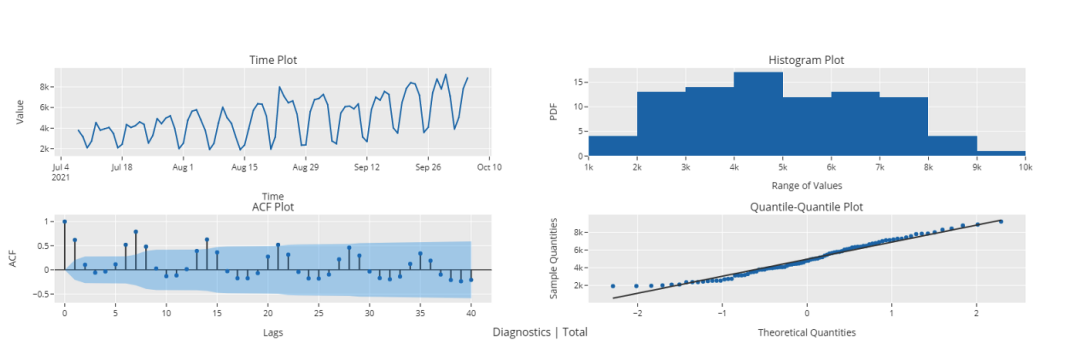
plot_model(plot = 'decomp_stl')
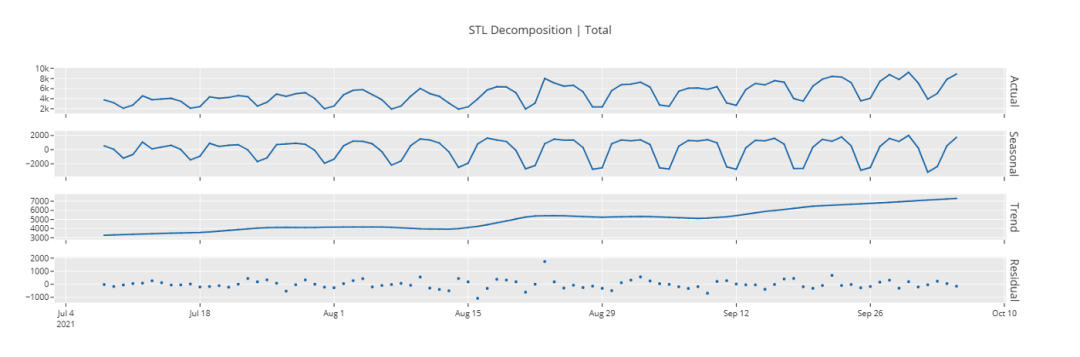
模型训练与选择
best = compare_models()
best = exp.compare_models()
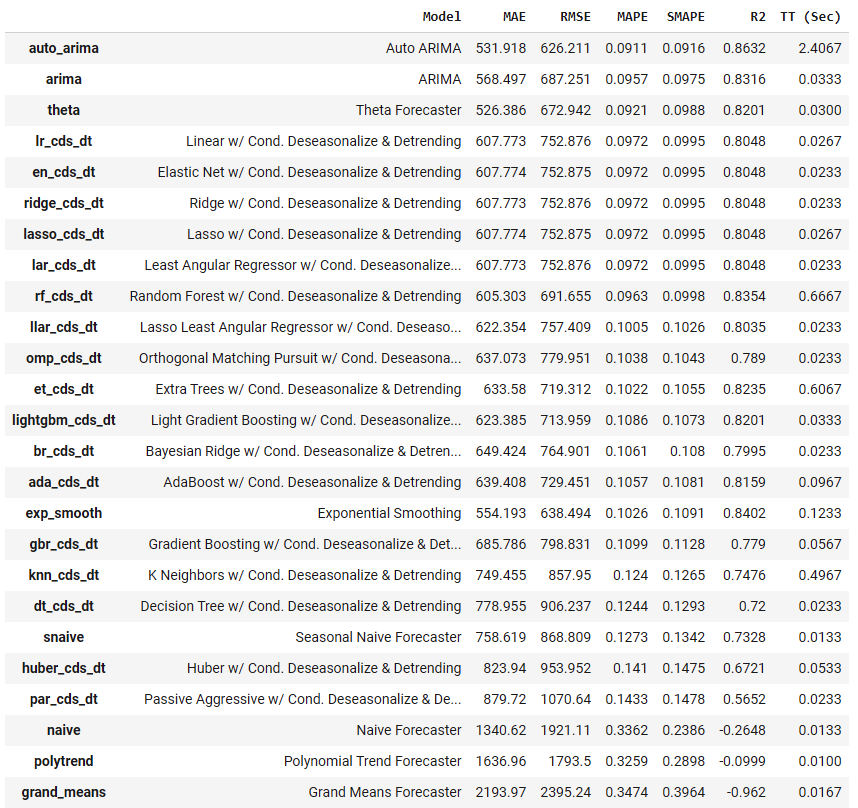
prophet = create_model('prophet')
print(prophet)
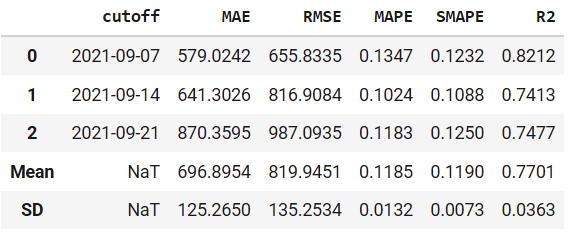
tuned_prophet = tune_model(prophet)
print(tuned_prophet)
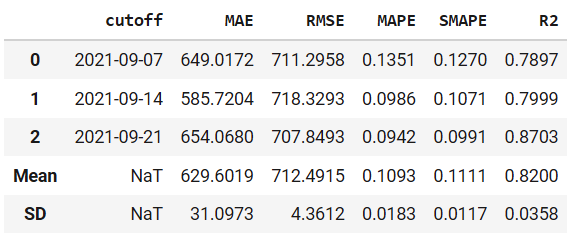
plot_model(best, plot = 'forecast')
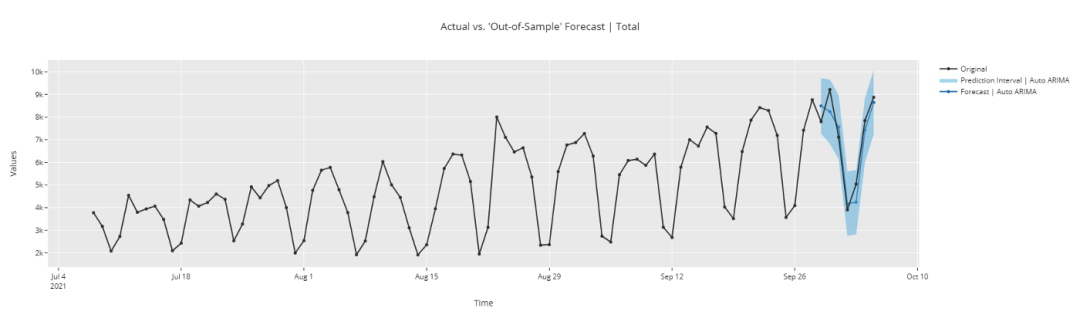
plot_model(best, plot = 'forecast', data_kwargs = {'fh' : 30})
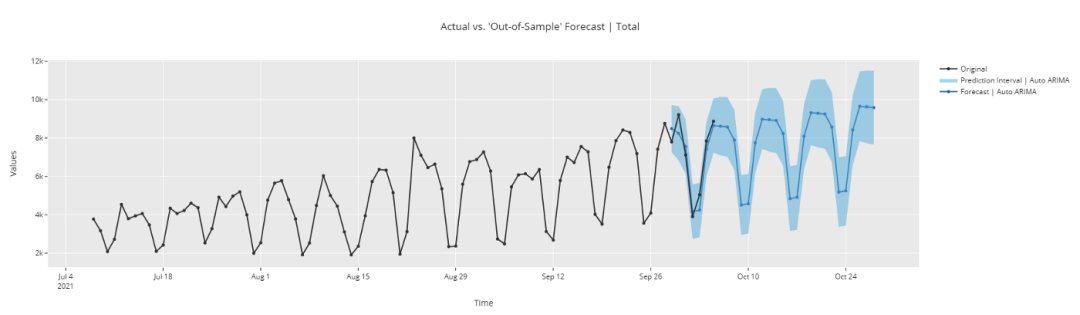
plot_model(best, plot = 'insample')
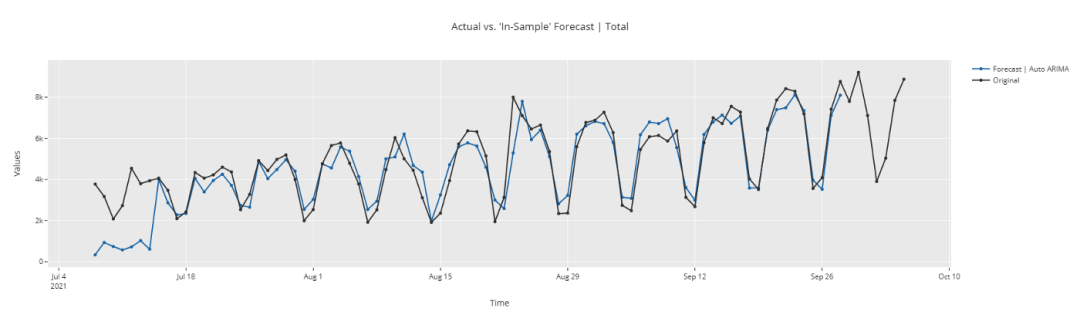
plot_model(best, plot = 'residuals')
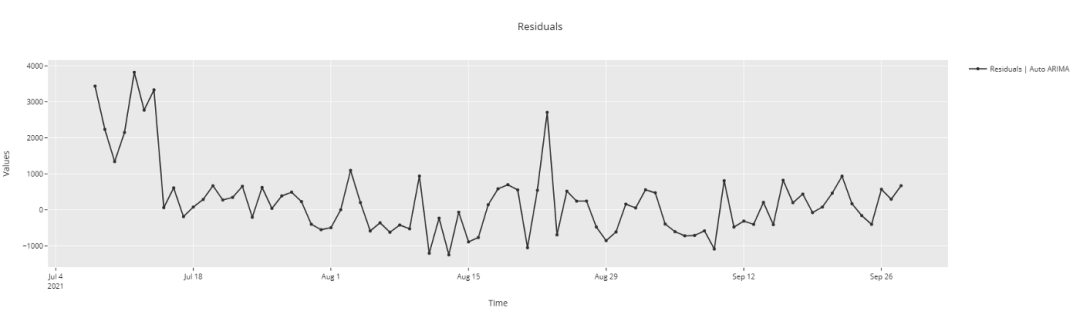
测试部署
final_best = finalize_model(best)
predict_model(final_best, fh = 90)
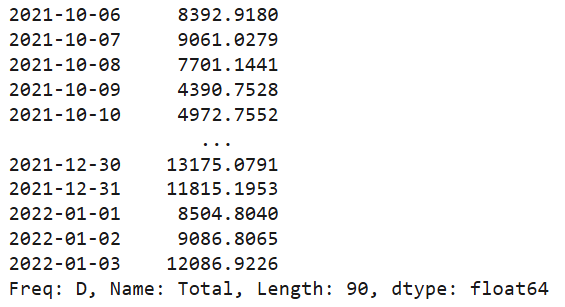
save_model(final_best, 'my_best_model')
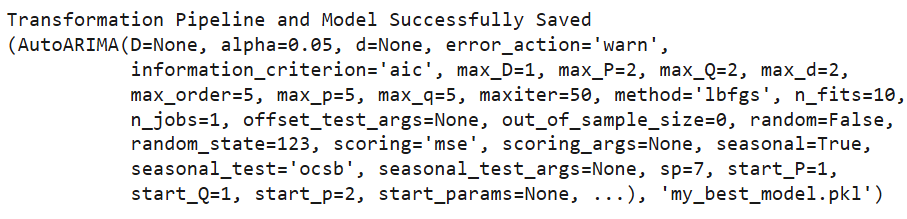
参考资料
pycaret时序文档:https://pycaret.readthedocs.io/en/time_series/api/time_series.html
pycaret时序规划:https://github.com/pycaret/pycaret/issues/1648
|