1. 数值型数据的处理
1.1 标准化&归一化
????????数据标准化是一个常用的数据预处理操作,目的是处理不同规模和量纲的数据,使其缩放到相同的数据区间和范围,以减少规模、特征、分布差异等对模型的影响。
示例代码:
import numpy as np
from sklearn import preprocessing
import matplotlib.pyplot as plt
data = np.loadtxt('../data/excel_data/data6.txt', delimiter='\t') # 读取数据
data
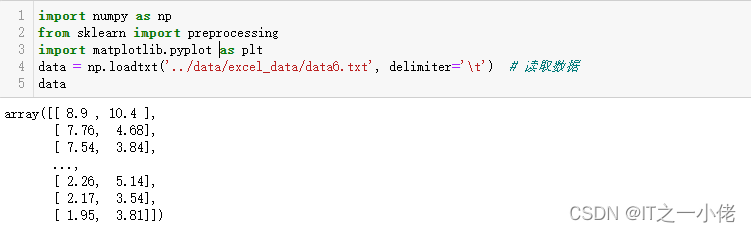
# Z-Score标准化
zscore_scaler = preprocessing.StandardScaler() # 建立StandardScaler对象
data_scale_1 = zscore_scaler.fit_transform(data) # StandardScaler标准化处理
data_scale_1
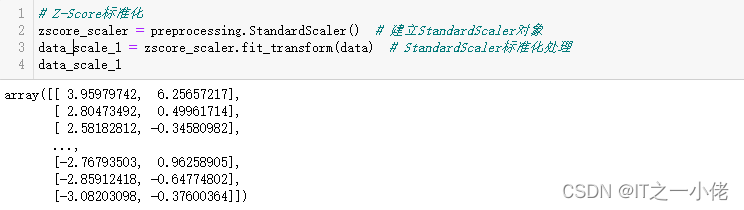
# 归一化Max-Min
minmax_scaler = preprocessing.MinMaxScaler() # 建立MinMaxScaler模型对象
data_scale_2 = minmax_scaler.fit_transform(data) # MinMaxScaler标准化处理
data_scale_2
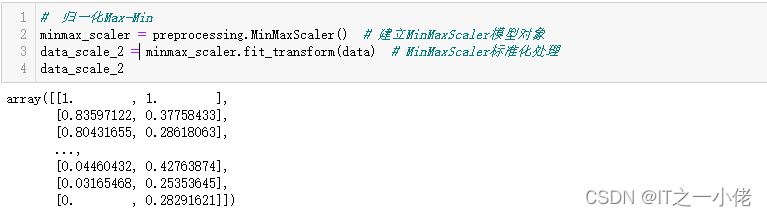
# 展示多网络结果
data_list = [data, data_scale_1, data_scale_2] # 创建数据集列表
color_list = ['black', 'green', 'blue'] # 创建颜色列表
merker_list = ['o', ',','+'] # 创建样式列表
title_list = ['source data', 'zscore_scaler', 'minmax_scaler'] # 创建标题列表
plt.figure(figsize=(13, 3))
for i, data_single in enumerate(data_list): # 循环得到索引和每个数值
plt.subplot(1, 3, i + 1) # 确定子网格
plt.scatter(data_single[:, :-1], data_single[:, -1], s=10, marker=merker_list[i], c=color_list[i]) # 子网格展示散点图
plt.title(title_list[i]) # 设置子网格标题
plt.suptitle("raw data and standardized data") # 设置总标题
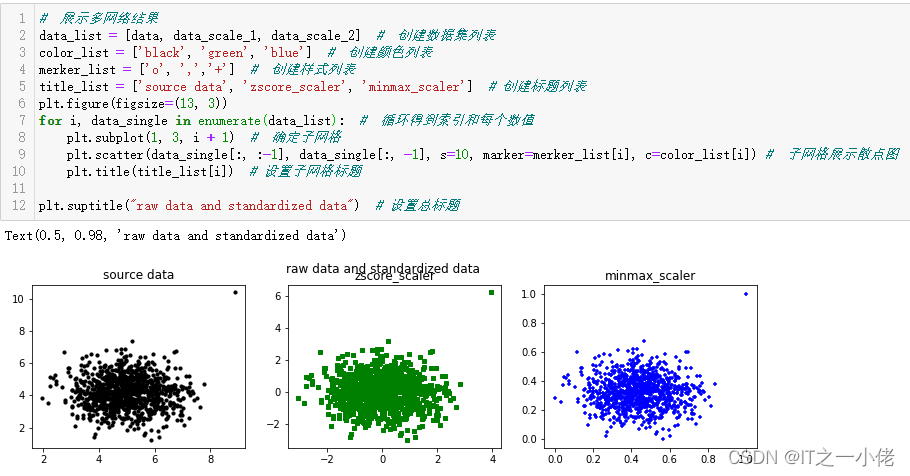
1.2. 离散化/分箱/分桶
import pandas as pd
from sklearn.cluster import KMeans
from sklearn import preprocessing
# 读取数据
df = pd.read_table('../data/excel_data/data7.txt', names=['id', 'amount', 'income', 'datetime', 'age']) # 读取数据文件
df.head(5) # 打印输出前5条数据
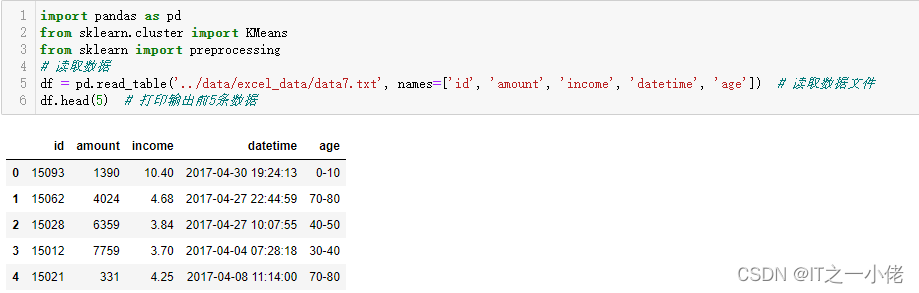
# 针对时间数据的离散化
df['datetime'] = list(map(pd.to_datetime,df['datetime'])) # 将时间转换为datetime格式
df['datetime'] = [i.weekday() for i in df['datetime']]# 离散化为周几
df.head(5) # 打印输出前5条数据
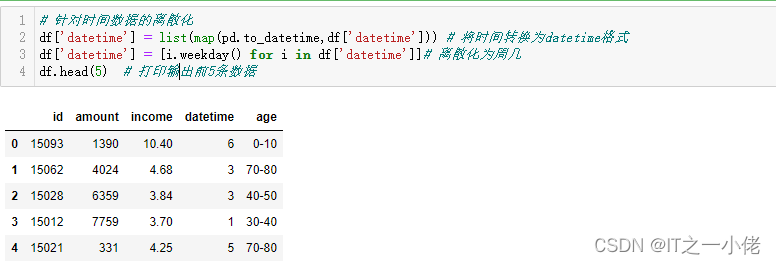
# 针对连续数据的离散化:自定义分箱区间实现离散化
bins = [0, 200, 1000, 5000, 10000] # 自定义区间边界
df['amount1'] = pd.cut(df['amount'], bins) # 使用边界做离散化
df.head(5) # 打印输出前5条数据
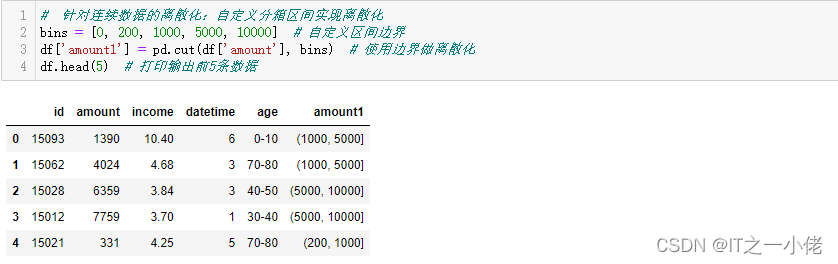
# 针对连续数据的离散化:使用聚类法实现离散化
data = df['amount'] # 获取要聚类的数据,名为amount的列
data_reshape = data.values.reshape((data.shape[0], 1)) # 转换数据形状
model_kmeans = KMeans(n_clusters=4, random_state=0) # 创建KMeans模型并指定要聚类数量
keames_result = model_kmeans.fit_predict(data_reshape) # 建模聚类
df['amount2'] = keames_result # 新离散化的数据合并到原数据框
df.head(5) # 打印输出前5条数据
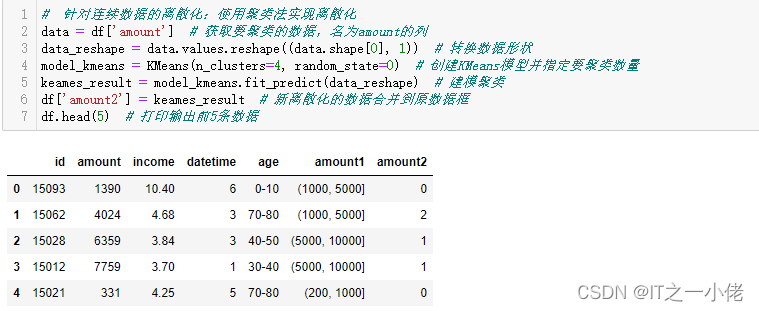
# 针对连续数据的离散化
df['amount3'] = pd.qcut(df['amount'], 4, labels=['bad', 'medium', 'good', 'awesome'])
df = df.drop('amount', 1) # 丢弃名为amount的列
df.head(5) # 打印输出前5条数据
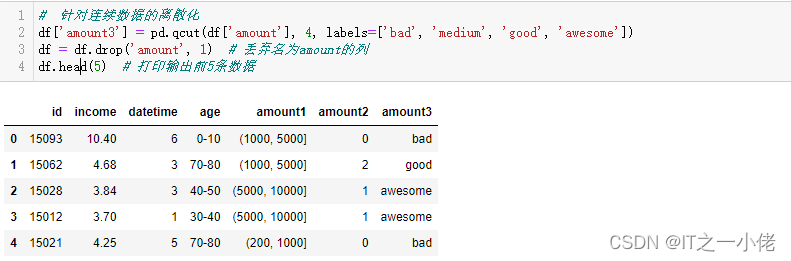
# 针对连续数据的二值化
binarizer_scaler = preprocessing.Binarizer(threshold=df['income'].mean()) # 建立Binarizer模型对象
income_tmp = binarizer_scaler.fit_transform(df[['income']]) # Binarizer标准化转换
income_tmp.resize(df['income'].shape) # 转换数据形状
df['income'] = income_tmp # Binarizer标准化转换
df.head(5) # 打印输出前5条数据
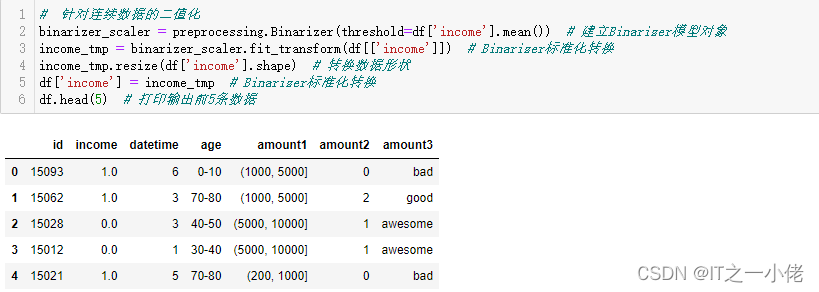
2. 分类数据的处理
举例:
- 性别中的男和女 [0,1] [1,0]
- 颜色中的红、黄和蓝
- 用户的价值度分为高、中、低
- 学历分为博士、硕士、学士、大专、高中
处理方法:
- 将字符串表示的分类特征转换成数值类型,一般用one-hot编码表示,方便建模处理
示例代码:
import pandas as pd # 导入pandas库
from sklearn.preprocessing import OneHotEncoder # 导入库
# 生成数据
df = pd.DataFrame({'id': [3566841, 6541227, 3512441],
'sex': ['male', 'Female', 'Female'],
'level': ['high', 'low', 'middle'],
'score': [1, 2, 3]})
df # 打印输出原始数据框
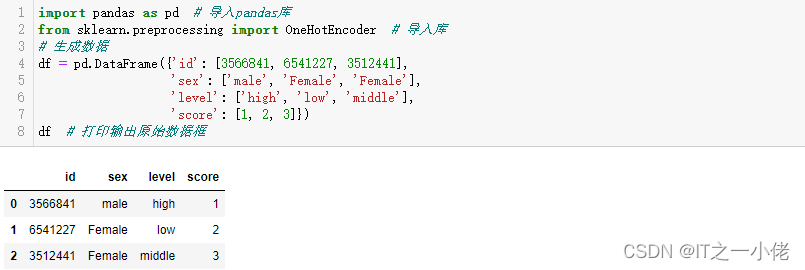
# 使用sklearn进行标志转换
# 拆分ID和数据列
id_data = df[['id']] # 获得ID列
raw_convert_data = df.iloc[:, 1:] # 指定要转换的列
raw_convert_data
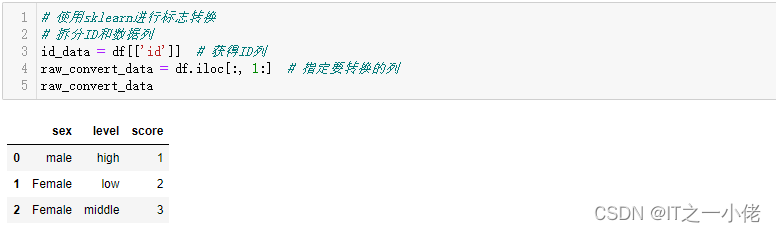
# 将数值型分类向量转换为标志变量
model_enc = OneHotEncoder() # 建立标志转换模型对象(也称为哑编码对象)
df_new2 = model_enc.fit_transform(raw_convert_data).toarray() # 标志转换
df_new2
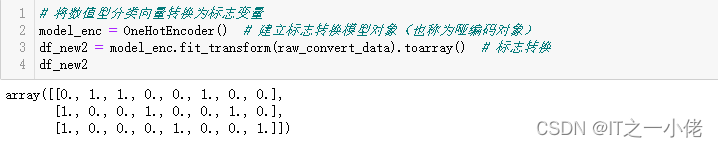
# 合并数据
df_all = pd.concat((id_data, pd.DataFrame(df_new2)), axis=1) # 重新组合为数据框
df_all # 打印输出转换后的数据框
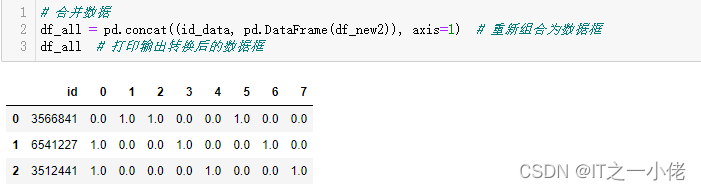
# 使用pandas的get_dummuies ,此方法只会对非数值类型的数据做转换
df_new3 = pd.get_dummies(raw_convert_data)
df_all2 = pd.concat((id_data, df_new3), axis=1) # 重新组合为数据框
df_all2 # 打印输出转换后的数据框
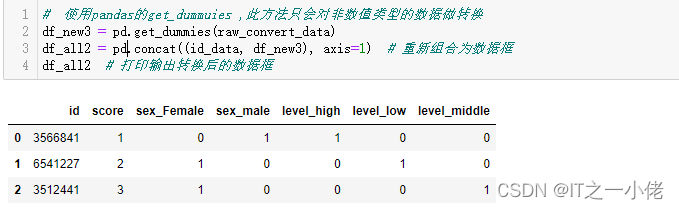
?3. 时间类型数据的处理
????????数据中包含日期时间类型的数据可以通过pandas的 to_datetime 转换成datetime类型,方便提取各种时间信息。
示例代码:
import pandas as pd
car_sales = pd.read_csv('../data/excel_data/car_data.csv')
car_sales.head()
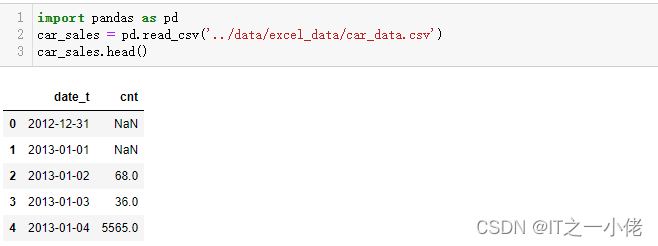
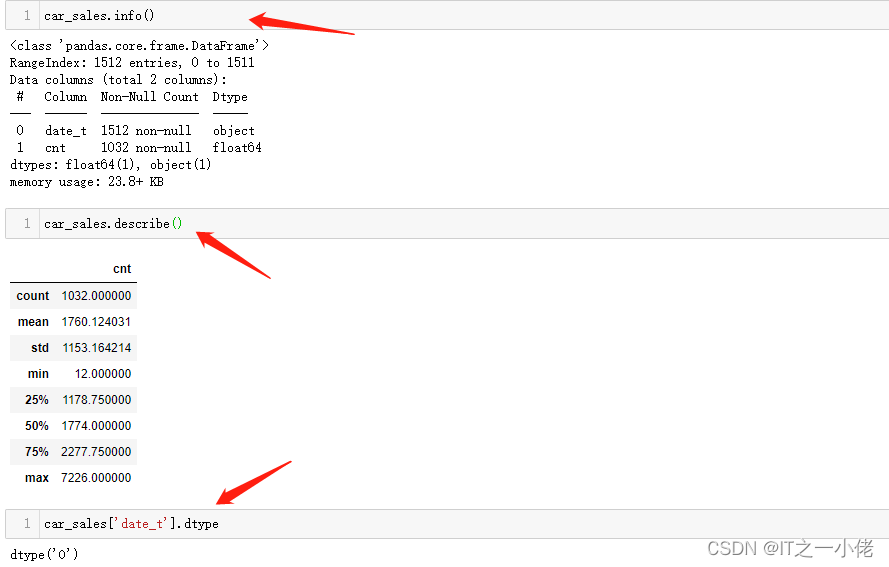
car_sales.loc[:,'date'] = pd.to_datetime(car_sales['date_t'])
car_sales
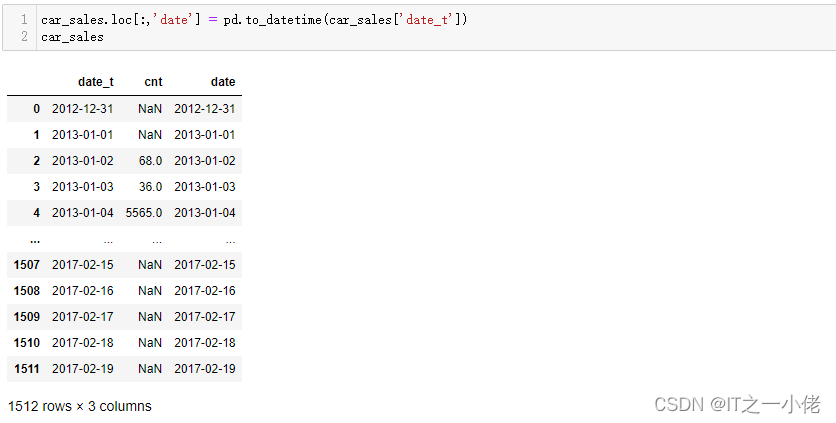
?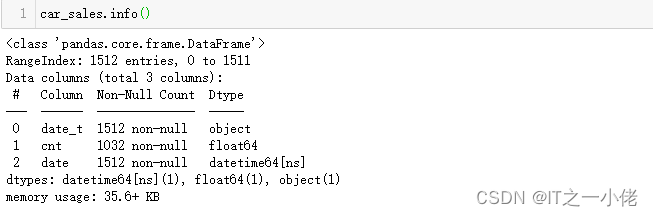
# 取出关键时间信息
# 取出几月份
car_sales.loc[:,'month'] = car_sales['date'].dt.month
# 取出来是几号
car_sales.loc[:,'dom'] = car_sales['date'].dt.day
# 取出一年当中的第几天
car_sales.loc[:,'doy'] = car_sales['date'].dt.dayofyear
# 取出星期几
car_sales.loc[:,'dow'] = car_sales['date'].dt.dayofweek
car_sales.head()
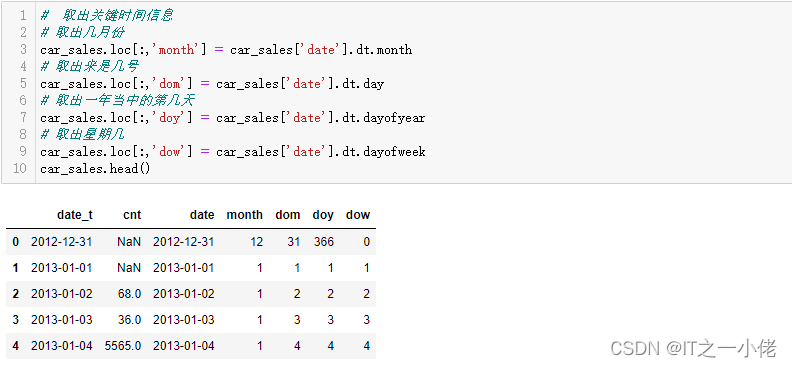
4.? 样本类别分布不均衡数据处理
示例代码:
import pandas as pd
from imblearn.over_sampling import SMOTE # 过抽样处理库SMOTE
from imblearn.under_sampling import RandomUnderSampler # 欠抽样处理库RandomUnderSampler
# 导入数据文件
df = pd.read_table('../data/excel_data/data2.txt', sep='\t', names=['col1', 'col2', 'col3', 'col4', 'col5', 'label']) # 读取数据文件
x, y = df.iloc[:, :-1],df.iloc[:, -1] # 切片,得到输入x,标签y
groupby_data_orgianl = df.groupby('label').count() # 对label做分类汇总
groupby_data_orgianl # 打印输出原始数据集样本分类分布
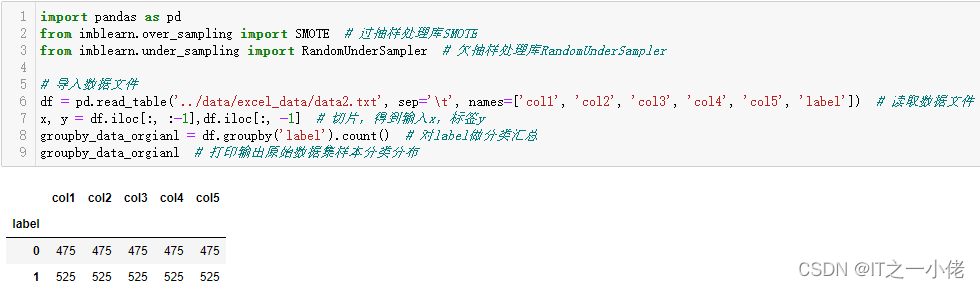
# 使用SMOTE方法进行过抽样处理
model_smote = SMOTE() # 建立SMOTE模型对象
x_smote_resampled, y_smote_resampled = model_smote.fit_resample(x, y) # 输入数据并作过抽样处理
x_smote_resampled = pd.DataFrame(x_smote_resampled, columns=['col1', 'col2', 'col3', 'col4', 'col5']) # 将数据转换为数据框并命名列名
y_smote_resampled = pd.DataFrame(y_smote_resampled, columns=['label']) # 将数据转换为数据框并命名列名
smote_resampled = pd.concat([x_smote_resampled, y_smote_resampled], axis=1) # 按列合并数据框
groupby_data_smote = smote_resampled.groupby('label').count() # 对label做分类汇总
groupby_data_smote # 打印输出经过SMOTE处理后的数据集样本分类分布
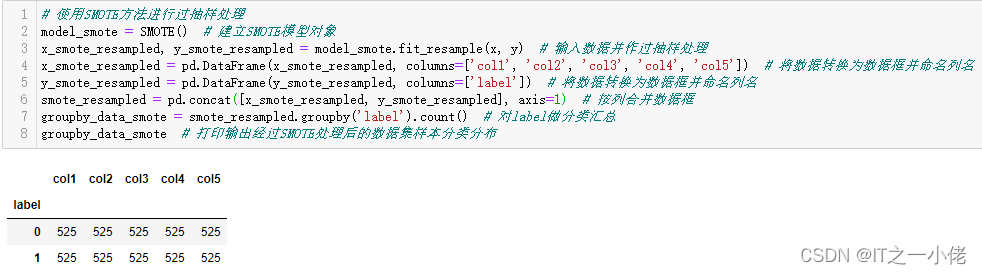
# 使用RandomUnderSampler方法进行欠抽样处理
model_RandomUnderSampler = RandomUnderSampler() # 建立RandomUnderSampler模型对象
x_RandomUnderSampler_resampled, y_RandomUnderSampler_resampled = model_RandomUnderSampler.fit_resample(x, y) # 输入数据并作欠抽样处理
x_RandomUnderSampler_resampled = pd.DataFrame(x_RandomUnderSampler_resampled,
columns=['col1', 'col2', 'col3', 'col4', 'col5']) # 将数据转换为数据框并命名列名
y_RandomUnderSampler_resampled = pd.DataFrame(y_RandomUnderSampler_resampled,
columns=['label']) # 将数据转换为数据框并命名列名
RandomUnderSampler_resampled = pd.concat([x_RandomUnderSampler_resampled, y_RandomUnderSampler_resampled],axis=1) # 按列合并数据框
groupby_data_RandomUnderSampler = RandomUnderSampler_resampled.groupby('label').count() # 对label做分类汇总
groupby_data_RandomUnderSampler # 打印输出经过RandomUnderSampler处理后的数据集样本分类分布
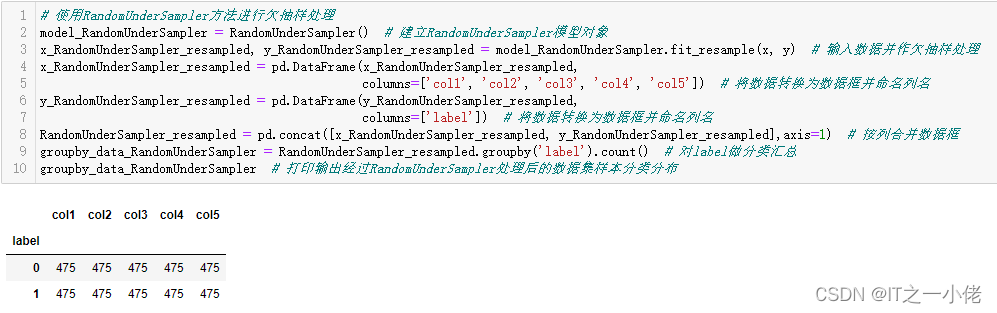
5.? 数据抽样
示例代码:
import random # 导入标准库
import numpy as np # 导入第三方库
data = np.loadtxt('../data/excel_data/data3.txt') # 导入普通数据文件
shuffle_index = np.random.choice(np.arange(data.shape[0]),2000,True)#随机生成200个行号
data_sample = data[shuffle_index] #从原始数据中取出200个行号对应的数据
print(len(data_sample)) # 打印输出抽样样本量
data_sample[:2] # 打印输出前2条数据
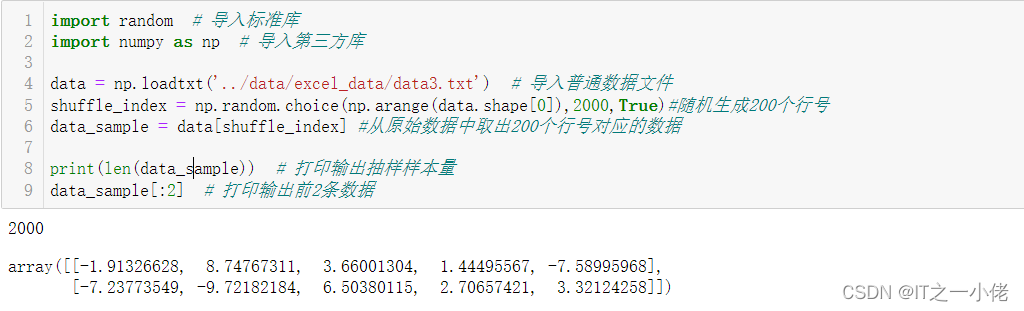
# 等距抽样
data = np.loadtxt('../data/excel_data/data3.txt') # 导入普通数据文件
sample_count = 2000 # 指定抽样数量
record_count = data.shape[0] # 获取最大样本量
width = record_count / sample_count # 计算抽样间距
data_sample = [] # 初始化空白列表,用来存放抽样结果数据
i = 0 # 自增计数以得到对应索引值
while len(data_sample) <= sample_count and i * width <= record_count - 1: # 当样本量小于等于指定抽样数量并且矩阵索引在有效范围内时
data_sample.append(data[int(i * width)]) # 新增样本
i += 1 # 自增长
print(len(data_sample)) # 打印输出样本数量
data_sample[:2] # 打印输出前2条数据
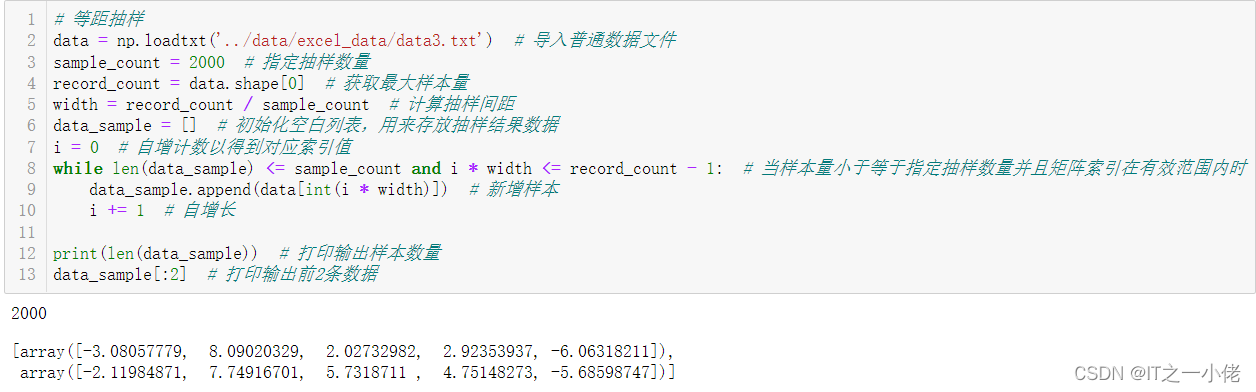
|