引言
weight文件前4-5个int32字节是head信息。单独保存,后面是float32数据,对应bn(gamma beta runing_mean running_var)和conv的权重参数 按照cfg文件定义的网络结构 ,解析cfg文件后,得到module_def, module,索引权重位置。
前提网络熟悉
yolov3输出的网络module_def结构,一共107个
[{'type': 'convolutional', 'batch_normalize': 1, 'filters': 32, 'size': 3, 'stride': 1, 'pad': 1, 'activation': 'leaky'},
{'type': 'convolutional', 'batch_normalize': 1, 'filters': 64, 'size': 3, 'stride': 2, 'pad': 1, 'activation': 'leaky'},
{'type': 'convolutional', 'batch_normalize': 1, 'filters': 32, 'size': 1, 'stride': 1, 'pad': 1, 'activation': 'leaky'},
{'type': 'convolutional', 'batch_normalize': 1, 'filters': 64, 'size': 3, 'stride': 1, 'pad': 1, 'activation': 'leaky'},
{'type': 'shortcut', 'from': [-3], 'activation': 'linear'},
{'type': 'convolutional', 'batch_normalize': 1, 'filters': 128, 'size': 3, 'stride': 2, 'pad': 1, 'activation': 'leaky'},
{'type': 'convolutional', 'batch_normalize': 1, 'filters': 64, 'size': 1, 'stride': 1, 'pad': 1, 'activation': 'leaky'},
{'type': 'convolutional', 'batch_normalize': 1, 'filters': 128, 'size': 3, 'stride': 1, 'pad': 1, 'activation': 'leaky'},
{'type': 'shortcut', 'from': [-3], 'activation': 'linear'},
{'type': 'convolutional', 'batch_normalize': 1, 'filters': 64, 'size': 1, 'stride': 1, 'pad': 1, 'activation': 'leaky'},
{'type': 'convolutional', 'batch_normalize': 1, 'filters': 128, 'size': 3, 'stride': 1, 'pad': 1, 'activation': 'leaky'},
{'type': 'shortcut', 'from': [-3], 'activation': 'linear'},
{'type': 'convolutional', 'batch_normalize': 1, 'filters': 256, 'size': 3, 'stride': 2, 'pad': 1, 'activation': 'leaky'},
{'type': 'convolutional', 'batch_normalize': 1, 'filters': 128, 'size': 1, 'stride': 1, 'pad': 1, 'activation': 'leaky'},
{'type': 'convolutional', 'batch_normalize': 1, 'filters': 256, 'size': 3, 'stride': 1, 'pad': 1, 'activation': 'leaky'},
{'type': 'shortcut', 'from': [-3], 'activation': 'linear'},
{'type': 'convolutional', 'batch_normalize': 1, 'filters': 128, 'size': 1, 'stride': 1, 'pad': 1, 'activation': 'leaky'},
{'type': 'convolutional', 'batch_normalize': 1, 'filters': 256, 'size': 3, 'stride': 1, 'pad': 1, 'activation': 'leaky'},
{'type': 'shortcut', 'from': [-3], 'activation': 'linear'},
{'type': 'convolutional', 'batch_normalize': 1, 'filters': 128, 'size': 1, 'stride': 1, 'pad': 1, 'activation': 'leaky'},
{'type': 'convolutional', 'batch_normalize': 1, 'filters': 256, 'size': 3, 'stride': 1, 'pad': 1, 'activation': 'leaky'},
{'type': 'shortcut', 'from': [-3], 'activation': 'linear'},
{'type': 'convolutional', 'batch_normalize': 1, 'filters': 128, 'size': 1, 'stride': 1, 'pad': 1, 'activation': 'leaky'},
{'type': 'convolutional', 'batch_normalize': 1, 'filters': 256, 'size': 3, 'stride': 1, 'pad': 1, 'activation': 'leaky'},
{'type': 'shortcut', 'from': [-3], 'activation': 'linear'},
{'type': 'convolutional', 'batch_normalize': 1, 'filters': 128, 'size': 1, 'stride': 1, 'pad': 1, 'activation': 'leaky'},
{'type': 'convolutional', 'batch_normalize': 1, 'filters': 256, 'size': 3, 'stride': 1, 'pad': 1, 'activation': 'leaky'},
{'type': 'shortcut', 'from': [-3], 'activation': 'linear'},
{'type': 'convolutional', 'batch_normalize': 1, 'filters': 128, 'size': 1, 'stride': 1, 'pad': 1, 'activation': 'leaky'},
{'type': 'convolutional', 'batch_normalize': 1, 'filters': 256, 'size': 3, 'stride': 1, 'pad': 1, 'activation': 'leaky'},
{'type': 'shortcut', 'from': [-3], 'activation': 'linear'},
{'type': 'convolutional', 'batch_normalize': 1, 'filters': 128, 'size': 1, 'stride': 1, 'pad': 1, 'activation': 'leaky'},
{'type': 'convolutional', 'batch_normalize': 1, 'filters': 256, 'size': 3, 'stride': 1, 'pad': 1, 'activation': 'leaky'},
{'type': 'shortcut', 'from': [-3], 'activation': 'linear'},
{'type': 'convolutional', 'batch_normalize': 1, 'filters': 128, 'size': 1, 'stride': 1, 'pad': 1, 'activation': 'leaky'},
{'type': 'convolutional', 'batch_normalize': 1, 'filters': 256, 'size': 3, 'stride': 1, 'pad': 1, 'activation': 'leaky'},
{'type': 'shortcut', 'from': [-3], 'activation': 'linear'},
{'type': 'convolutional', 'batch_normalize': 1, 'filters': 512, 'size': 3, 'stride': 2, 'pad': 1, 'activation': 'leaky'},
{'type': 'convolutional', 'batch_normalize': 1, 'filters': 256, 'size': 1, 'stride': 1, 'pad': 1, 'activation': 'leaky'},
{'type': 'convolutional', 'batch_normalize': 1, 'filters': 512, 'size': 3, 'stride': 1, 'pad': 1, 'activation': 'leaky'},
{'type': 'shortcut', 'from': [-3], 'activation': 'linear'},
{'type': 'convolutional', 'batch_normalize': 1, 'filters': 256, 'size': 1, 'stride': 1, 'pad': 1, 'activation': 'leaky'},
{'type': 'convolutional', 'batch_normalize': 1, 'filters': 512, 'size': 3, 'stride': 1, 'pad': 1, 'activation': 'leaky'},
{'type': 'shortcut', 'from': [-3], 'activation': 'linear'},
{'type': 'convolutional', 'batch_normalize': 1, 'filters': 256, 'size': 1, 'stride': 1, 'pad': 1, 'activation': 'leaky'},
{'type': 'convolutional', 'batch_normalize': 1, 'filters': 512, 'size': 3, 'stride': 1, 'pad': 1, 'activation': 'leaky'},
{'type': 'shortcut', 'from': [-3], 'activation': 'linear'},
{'type': 'convolutional', 'batch_normalize': 1, 'filters': 256, 'size': 1, 'stride': 1, 'pad': 1, 'activation': 'leaky'},
{'type': 'convolutional', 'batch_normalize': 1, 'filters': 512, 'size': 3, 'stride': 1, 'pad': 1, 'activation': 'leaky'},
{'type': 'shortcut', 'from': [-3], 'activation': 'linear'},
{'type': 'convolutional', 'batch_normalize': 1, 'filters': 256, 'size': 1, 'stride': 1, 'pad': 1, 'activation': 'leaky'},
{'type': 'convolutional', 'batch_normalize': 1, 'filters': 512, 'size': 3, 'stride': 1, 'pad': 1, 'activation': 'leaky'},
{'type': 'shortcut', 'from': [-3], 'activation': 'linear'},
{'type': 'convolutional', 'batch_normalize': 1, 'filters': 256, 'size': 1, 'stride': 1, 'pad': 1, 'activation': 'leaky'},
{'type': 'convolutional', 'batch_normalize': 1, 'filters': 512, 'size': 3, 'stride': 1, 'pad': 1, 'activation': 'leaky'},
{'type': 'shortcut', 'from': [-3], 'activation': 'linear'},
{'type': 'convolutional', 'batch_normalize': 1, 'filters': 256, 'size': 1, 'stride': 1, 'pad': 1, 'activation': 'leaky'},
{'type': 'convolutional', 'batch_normalize': 1, 'filters': 512, 'size': 3, 'stride': 1, 'pad': 1, 'activation': 'leaky'},
{'type': 'shortcut', 'from': [-3], 'activation': 'linear'},
{'type': 'convolutional', 'batch_normalize': 1, 'filters': 256, 'size': 1, 'stride': 1, 'pad': 1, 'activation': 'leaky'},
{'type': 'convolutional', 'batch_normalize': 1, 'filters': 512, 'size': 3, 'stride': 1, 'pad': 1, 'activation': 'leaky'},
{'type': 'shortcut', 'from': [-3], 'activation': 'linear'},
{'type': 'convolutional', 'batch_normalize': 1, 'filters': 1024, 'size': 3, 'stride': 2, 'pad': 1, 'activation': 'leaky'},
{'type': 'convolutional', 'batch_normalize': 1, 'filters': 512, 'size': 1, 'stride': 1, 'pad': 1, 'activation': 'leaky'},
{'type': 'convolutional', 'batch_normalize': 1, 'filters': 1024, 'size': 3, 'stride': 1, 'pad': 1, 'activation': 'leaky'},
{'type': 'shortcut', 'from': [-3], 'activation': 'linear'},
{'type': 'convolutional', 'batch_normalize': 1, 'filters': 512, 'size': 1, 'stride': 1, 'pad': 1, 'activation': 'leaky'},
{'type': 'convolutional', 'batch_normalize': 1, 'filters': 1024, 'size': 3, 'stride': 1, 'pad': 1, 'activation': 'leaky'},
{'type': 'shortcut', 'from': [-3], 'activation': 'linear'},
{'type': 'convolutional', 'batch_normalize': 1, 'filters': 512, 'size': 1, 'stride': 1, 'pad': 1, 'activation': 'leaky'},
{'type': 'convolutional', 'batch_normalize': 1, 'filters': 1024, 'size': 3, 'stride': 1, 'pad': 1, 'activation': 'leaky'},
{'type': 'shortcut', 'from': [-3], 'activation': 'linear'},
{'type': 'convolutional', 'batch_normalize': 1, 'filters': 512, 'size': 1, 'stride': 1, 'pad': 1, 'activation': 'leaky'},
{'type': 'convolutional', 'batch_normalize': 1, 'filters': 1024, 'size': 3, 'stride': 1, 'pad': 1, 'activation': 'leaky'},
{'type': 'shortcut', 'from': [-3], 'activation': 'linear'},
{'type': 'convolutional', 'batch_normalize': 1, 'filters': 512, 'size': 1, 'stride': 1, 'pad': 1, 'activation': 'leaky'},
{'type': 'convolutional', 'batch_normalize': 1, 'size': 3, 'stride': 1, 'pad': 1, 'filters': 1024, 'activation': 'leaky'},
{'type': 'convolutional', 'batch_normalize': 1, 'filters': 512, 'size': 1, 'stride': 1, 'pad': 1, 'activation': 'leaky'},
{'type': 'convolutional', 'batch_normalize': 1, 'size': 3, 'stride': 1, 'pad': 1, 'filters': 1024, 'activation': 'leaky'},
{'type': 'convolutional', 'batch_normalize': 1, 'filters': 512, 'size': 1, 'stride': 1, 'pad': 1, 'activation': 'leaky'},
{'type': 'convolutional', 'batch_normalize': 1, 'size': 3, 'stride': 1, 'pad': 1, 'filters': 1024, 'activation': 'leaky'},
{'type': 'convolutional', 'batch_normalize': 0, 'size': 1, 'stride': 1, 'pad': 1, 'filters': 24, 'activation': 'linear'},
{'type': 'yolo', 'mask': [6, 7, 8], 'anchors': array([[ 10., 13.],
[ 16., 30.],
[ 33., 23.],
[ 30., 61.],
[ 62., 45.],
[ 59., 119.],
[116., 90.],
[156., 198.],
[373., 326.]]), 'classes': 3, 'num': 9, 'jitter': '.3', 'ignore_thresh': '.5', 'truth_thresh': 1, 'random': 1},
{'type': 'route', 'layers': [-4]},
{'type': 'convolutional', 'batch_normalize': 1, 'filters': 256, 'size': 1, 'stride': 1, 'pad': 1, 'activation': 'leaky'},
{'type': 'upsample', 'stride': 2},
{'type': 'route', 'layers': [-1, 61]},
{'type': 'convolutional', 'batch_normalize': 1, 'filters': 256, 'size': 1, 'stride': 1, 'pad': 1, 'activation': 'leaky'},
{'type': 'convolutional', 'batch_normalize': 1, 'size': 3, 'stride': 1, 'pad': 1, 'filters': 512, 'activation': 'leaky'},
{'type': 'convolutional', 'batch_normalize': 1, 'filters': 256, 'size': 1, 'stride': 1, 'pad': 1, 'activation': 'leaky'},
{'type': 'convolutional', 'batch_normalize': 1, 'size': 3, 'stride': 1, 'pad': 1, 'filters': 512, 'activation': 'leaky'},
{'type': 'convolutional', 'batch_normalize': 1, 'filters': 256, 'size': 1, 'stride': 1, 'pad': 1, 'activation': 'leaky'},
{'type': 'convolutional', 'batch_normalize': 1, 'size': 3, 'stride': 1, 'pad': 1, 'filters': 512, 'activation': 'leaky'},
{'type': 'convolutional', 'batch_normalize': 0, 'size': 1, 'stride': 1, 'pad': 1, 'filters': 24, 'activation': 'linear'},
{'type': 'yolo', 'mask': [3, 4, 5], 'anchors': array([[ 10., 13.],
[ 16., 30.],
[ 33., 23.],
[ 30., 61.],
[ 62., 45.],
[ 59., 119.],
[116., 90.],
[156., 198.],
[373., 326.]]), 'classes': 3, 'num': 9, 'jitter': '.3', 'ignore_thresh': '.5', 'truth_thresh': 1, 'random': 1},
{'type': 'route', 'layers': [-4]},
{'type': 'convolutional', 'batch_normalize': 1, 'filters': 128, 'size': 1, 'stride': 1, 'pad': 1, 'activation': 'leaky'},
{'type': 'upsample', 'stride': 2},
{'type': 'route', 'layers': [-1, 36]},
{'type': 'convolutional', 'batch_normalize': 1, 'filters': 128, 'size': 1, 'stride': 1, 'pad': 1, 'activation': 'leaky'},
{'type': 'convolutional', 'batch_normalize': 1, 'size': 3, 'stride': 1, 'pad': 1, 'filters': 256, 'activation': 'leaky'},
{'type': 'convolutional', 'batch_normalize': 1, 'filters': 128, 'size': 1, 'stride': 1, 'pad': 1, 'activation': 'leaky'},
{'type': 'convolutional', 'batch_normalize': 1, 'size': 3, 'stride': 1, 'pad': 1, 'filters': 256, 'activation': 'leaky'},
{'type': 'convolutional', 'batch_normalize': 1, 'filters': 128, 'size': 1, 'stride': 1, 'pad': 1, 'activation': 'leaky'},
{'type': 'convolutional', 'batch_normalize': 1, 'size': 3, 'stride': 1, 'pad': 1, 'filters': 256, 'activation': 'leaky'},
{'type': 'convolutional', 'batch_normalize': 0, 'size': 1, 'stride': 1, 'pad': 1, 'filters': 24, 'activation': 'linear'},
{'type': 'yolo', 'mask': [0, 1, 2], 'anchors': array([[ 10., 13.],
[ 16., 30.],
[ 33., 23.],
[ 30., 61.],
[ 62., 45.],
[ 59., 119.],
[116., 90.],
[156., 198.],
[373., 326.]]), 'classes': 3, 'num': 9, 'jitter': '.3', 'ignore_thresh': '.5', 'truth_thresh': 1, 'random': 1}]
对应的model结构 0-106
Darknet(
(module_list): ModuleList(
(0): Sequential(
(Conv2d): Conv2d(3, 32, kernel_size=(3, 3), stride=(1, 1), padding=(1, 1), bias=False)
(BatchNorm2d): BatchNorm2d(32, eps=1e-05, momentum=0.1, affine=True, track_running_stats=True)
(activation): LeakyReLU(negative_slope=0.1)
)
(1): Sequential(
(Conv2d): Conv2d(32, 64, kernel_size=(3, 3), stride=(2, 2), padding=(1, 1), bias=False)
(BatchNorm2d): BatchNorm2d(64, eps=1e-05, momentum=0.1, affine=True, track_running_stats=True)
(activation): LeakyReLU(negative_slope=0.1)
)
(2): Sequential(
(Conv2d): Conv2d(64, 32, kernel_size=(1, 1), stride=(1, 1), bias=False)
(BatchNorm2d): BatchNorm2d(32, eps=1e-05, momentum=0.1, affine=True, track_running_stats=True)
(activation): LeakyReLU(negative_slope=0.1)
)
(3): Sequential(
(Conv2d): Conv2d(32, 64, kernel_size=(3, 3), stride=(1, 1), padding=(1, 1), bias=False)
(BatchNorm2d): BatchNorm2d(64, eps=1e-05, momentum=0.1, affine=True, track_running_stats=True)
(activation): LeakyReLU(negative_slope=0.1)
)
(4): WeightedFeatureFusion()
(5): Sequential(
(Conv2d): Conv2d(64, 128, kernel_size=(3, 3), stride=(2, 2), padding=(1, 1), bias=False)
(BatchNorm2d): BatchNorm2d(128, eps=1e-05, momentum=0.1, affine=True, track_running_stats=True)
(activation): LeakyReLU(negative_slope=0.1)
)
(6): Sequential(
(Conv2d): Conv2d(128, 64, kernel_size=(1, 1), stride=(1, 1), bias=False)
(BatchNorm2d): BatchNorm2d(64, eps=1e-05, momentum=0.1, affine=True, track_running_stats=True)
(activation): LeakyReLU(negative_slope=0.1)
)
(7): Sequential(
(Conv2d): Conv2d(64, 128, kernel_size=(3, 3), stride=(1, 1), padding=(1, 1), bias=False)
(BatchNorm2d): BatchNorm2d(128, eps=1e-05, momentum=0.1, affine=True, track_running_stats=True)
(activation): LeakyReLU(negative_slope=0.1)
)
(8): WeightedFeatureFusion()
(9): Sequential(
(Conv2d): Conv2d(128, 64, kernel_size=(1, 1), stride=(1, 1), bias=False)
(BatchNorm2d): BatchNorm2d(64, eps=1e-05, momentum=0.1, affine=True, track_running_stats=True)
(activation): LeakyReLU(negative_slope=0.1)
)
(10): Sequential(
(Conv2d): Conv2d(64, 128, kernel_size=(3, 3), stride=(1, 1), padding=(1, 1), bias=False)
(BatchNorm2d): BatchNorm2d(128, eps=1e-05, momentum=0.1, affine=True, track_running_stats=True)
(activation): LeakyReLU(negative_slope=0.1)
)
(11): WeightedFeatureFusion()
(12): Sequential(
(Conv2d): Conv2d(128, 256, kernel_size=(3, 3), stride=(2, 2), padding=(1, 1), bias=False)
(BatchNorm2d): BatchNorm2d(256, eps=1e-05, momentum=0.1, affine=True, track_running_stats=True)
(activation): LeakyReLU(negative_slope=0.1)
)
(13): Sequential(
(Conv2d): Conv2d(256, 128, kernel_size=(1, 1), stride=(1, 1), bias=False)
(BatchNorm2d): BatchNorm2d(128, eps=1e-05, momentum=0.1, affine=True, track_running_stats=True)
(activation): LeakyReLU(negative_slope=0.1)
)
(14): Sequential(
(Conv2d): Conv2d(128, 256, kernel_size=(3, 3), stride=(1, 1), padding=(1, 1), bias=False)
(BatchNorm2d): BatchNorm2d(256, eps=1e-05, momentum=0.1, affine=True, track_running_stats=True)
(activation): LeakyReLU(negative_slope=0.1)
)
(15): WeightedFeatureFusion()
(16): Sequential(
(Conv2d): Conv2d(256, 128, kernel_size=(1, 1), stride=(1, 1), bias=False)
(BatchNorm2d): BatchNorm2d(128, eps=1e-05, momentum=0.1, affine=True, track_running_stats=True)
(activation): LeakyReLU(negative_slope=0.1)
)
(17): Sequential(
(Conv2d): Conv2d(128, 256, kernel_size=(3, 3), stride=(1, 1), padding=(1, 1), bias=False)
(BatchNorm2d): BatchNorm2d(256, eps=1e-05, momentum=0.1, affine=True, track_running_stats=True)
(activation): LeakyReLU(negative_slope=0.1)
)
(18): WeightedFeatureFusion()
(19): Sequential(
(Conv2d): Conv2d(256, 128, kernel_size=(1, 1), stride=(1, 1), bias=False)
(BatchNorm2d): BatchNorm2d(128, eps=1e-05, momentum=0.1, affine=True, track_running_stats=True)
(activation): LeakyReLU(negative_slope=0.1)
)
(20): Sequential(
(Conv2d): Conv2d(128, 256, kernel_size=(3, 3), stride=(1, 1), padding=(1, 1), bias=False)
(BatchNorm2d): BatchNorm2d(256, eps=1e-05, momentum=0.1, affine=True, track_running_stats=True)
(activation): LeakyReLU(negative_slope=0.1)
)
(21): WeightedFeatureFusion()
(22): Sequential(
(Conv2d): Conv2d(256, 128, kernel_size=(1, 1), stride=(1, 1), bias=False)
(BatchNorm2d): BatchNorm2d(128, eps=1e-05, momentum=0.1, affine=True, track_running_stats=True)
(activation): LeakyReLU(negative_slope=0.1)
)
(23): Sequential(
(Conv2d): Conv2d(128, 256, kernel_size=(3, 3), stride=(1, 1), padding=(1, 1), bias=False)
(BatchNorm2d): BatchNorm2d(256, eps=1e-05, momentum=0.1, affine=True, track_running_stats=True)
(activation): LeakyReLU(negative_slope=0.1)
)
(24): WeightedFeatureFusion()
(25): Sequential(
(Conv2d): Conv2d(256, 128, kernel_size=(1, 1), stride=(1, 1), bias=False)
(BatchNorm2d): BatchNorm2d(128, eps=1e-05, momentum=0.1, affine=True, track_running_stats=True)
(activation): LeakyReLU(negative_slope=0.1)
)
(26): Sequential(
(Conv2d): Conv2d(128, 256, kernel_size=(3, 3), stride=(1, 1), padding=(1, 1), bias=False)
(BatchNorm2d): BatchNorm2d(256, eps=1e-05, momentum=0.1, affine=True, track_running_stats=True)
(activation): LeakyReLU(negative_slope=0.1)
)
(27): WeightedFeatureFusion()
(28): Sequential(
(Conv2d): Conv2d(256, 128, kernel_size=(1, 1), stride=(1, 1), bias=False)
(BatchNorm2d): BatchNorm2d(128, eps=1e-05, momentum=0.1, affine=True, track_running_stats=True)
(activation): LeakyReLU(negative_slope=0.1)
)
(29): Sequential(
(Conv2d): Conv2d(128, 256, kernel_size=(3, 3), stride=(1, 1), padding=(1, 1), bias=False)
(BatchNorm2d): BatchNorm2d(256, eps=1e-05, momentum=0.1, affine=True, track_running_stats=True)
(activation): LeakyReLU(negative_slope=0.1)
)
(30): WeightedFeatureFusion()
(31): Sequential(
(Conv2d): Conv2d(256, 128, kernel_size=(1, 1), stride=(1, 1), bias=False)
(BatchNorm2d): BatchNorm2d(128, eps=1e-05, momentum=0.1, affine=True, track_running_stats=True)
(activation): LeakyReLU(negative_slope=0.1)
)
(32): Sequential(
(Conv2d): Conv2d(128, 256, kernel_size=(3, 3), stride=(1, 1), padding=(1, 1), bias=False)
(BatchNorm2d): BatchNorm2d(256, eps=1e-05, momentum=0.1, affine=True, track_running_stats=True)
(activation): LeakyReLU(negative_slope=0.1)
)
(33): WeightedFeatureFusion()
(34): Sequential(
(Conv2d): Conv2d(256, 128, kernel_size=(1, 1), stride=(1, 1), bias=False)
(BatchNorm2d): BatchNorm2d(128, eps=1e-05, momentum=0.1, affine=True, track_running_stats=True)
(activation): LeakyReLU(negative_slope=0.1)
)
(35): Sequential(
(Conv2d): Conv2d(128, 256, kernel_size=(3, 3), stride=(1, 1), padding=(1, 1), bias=False)
(BatchNorm2d): BatchNorm2d(256, eps=1e-05, momentum=0.1, affine=True, track_running_stats=True)
(activation): LeakyReLU(negative_slope=0.1)
)
(36): WeightedFeatureFusion()
(37): Sequential(
(Conv2d): Conv2d(256, 512, kernel_size=(3, 3), stride=(2, 2), padding=(1, 1), bias=False)
(BatchNorm2d): BatchNorm2d(512, eps=1e-05, momentum=0.1, affine=True, track_running_stats=True)
(activation): LeakyReLU(negative_slope=0.1)
)
(38): Sequential(
(Conv2d): Conv2d(512, 256, kernel_size=(1, 1), stride=(1, 1), bias=False)
(BatchNorm2d): BatchNorm2d(256, eps=1e-05, momentum=0.1, affine=True, track_running_stats=True)
(activation): LeakyReLU(negative_slope=0.1)
)
(39): Sequential(
(Conv2d): Conv2d(256, 512, kernel_size=(3, 3), stride=(1, 1), padding=(1, 1), bias=False)
(BatchNorm2d): BatchNorm2d(512, eps=1e-05, momentum=0.1, affine=True, track_running_stats=True)
(activation): LeakyReLU(negative_slope=0.1)
)
(40): WeightedFeatureFusion()
(41): Sequential(
(Conv2d): Conv2d(512, 256, kernel_size=(1, 1), stride=(1, 1), bias=False)
(BatchNorm2d): BatchNorm2d(256, eps=1e-05, momentum=0.1, affine=True, track_running_stats=True)
(activation): LeakyReLU(negative_slope=0.1)
)
(42): Sequential(
(Conv2d): Conv2d(256, 512, kernel_size=(3, 3), stride=(1, 1), padding=(1, 1), bias=False)
(BatchNorm2d): BatchNorm2d(512, eps=1e-05, momentum=0.1, affine=True, track_running_stats=True)
(activation): LeakyReLU(negative_slope=0.1)
)
(43): WeightedFeatureFusion()
(44): Sequential(
(Conv2d): Conv2d(512, 256, kernel_size=(1, 1), stride=(1, 1), bias=False)
(BatchNorm2d): BatchNorm2d(256, eps=1e-05, momentum=0.1, affine=True, track_running_stats=True)
(activation): LeakyReLU(negative_slope=0.1)
)
(45): Sequential(
(Conv2d): Conv2d(256, 512, kernel_size=(3, 3), stride=(1, 1), padding=(1, 1), bias=False)
(BatchNorm2d): BatchNorm2d(512, eps=1e-05, momentum=0.1, affine=True, track_running_stats=True)
(activation): LeakyReLU(negative_slope=0.1)
)
(46): WeightedFeatureFusion()
(47): Sequential(
(Conv2d): Conv2d(512, 256, kernel_size=(1, 1), stride=(1, 1), bias=False)
(BatchNorm2d): BatchNorm2d(256, eps=1e-05, momentum=0.1, affine=True, track_running_stats=True)
(activation): LeakyReLU(negative_slope=0.1)
)
(48): Sequential(
(Conv2d): Conv2d(256, 512, kernel_size=(3, 3), stride=(1, 1), padding=(1, 1), bias=False)
(BatchNorm2d): BatchNorm2d(512, eps=1e-05, momentum=0.1, affine=True, track_running_stats=True)
(activation): LeakyReLU(negative_slope=0.1)
)
(49): WeightedFeatureFusion()
(50): Sequential(
(Conv2d): Conv2d(512, 256, kernel_size=(1, 1), stride=(1, 1), bias=False)
(BatchNorm2d): BatchNorm2d(256, eps=1e-05, momentum=0.1, affine=True, track_running_stats=True)
(activation): LeakyReLU(negative_slope=0.1)
)
(51): Sequential(
(Conv2d): Conv2d(256, 512, kernel_size=(3, 3), stride=(1, 1), padding=(1, 1), bias=False)
(BatchNorm2d): BatchNorm2d(512, eps=1e-05, momentum=0.1, affine=True, track_running_stats=True)
(activation): LeakyReLU(negative_slope=0.1)
)
(52): WeightedFeatureFusion()
(53): Sequential(
(Conv2d): Conv2d(512, 256, kernel_size=(1, 1), stride=(1, 1), bias=False)
(BatchNorm2d): BatchNorm2d(256, eps=1e-05, momentum=0.1, affine=True, track_running_stats=True)
(activation): LeakyReLU(negative_slope=0.1)
)
(54): Sequential(
(Conv2d): Conv2d(256, 512, kernel_size=(3, 3), stride=(1, 1), padding=(1, 1), bias=False)
(BatchNorm2d): BatchNorm2d(512, eps=1e-05, momentum=0.1, affine=True, track_running_stats=True)
(activation): LeakyReLU(negative_slope=0.1)
)
(55): WeightedFeatureFusion()
(56): Sequential(
(Conv2d): Conv2d(512, 256, kernel_size=(1, 1), stride=(1, 1), bias=False)
(BatchNorm2d): BatchNorm2d(256, eps=1e-05, momentum=0.1, affine=True, track_running_stats=True)
(activation): LeakyReLU(negative_slope=0.1)
)
(57): Sequential(
(Conv2d): Conv2d(256, 512, kernel_size=(3, 3), stride=(1, 1), padding=(1, 1), bias=False)
(BatchNorm2d): BatchNorm2d(512, eps=1e-05, momentum=0.1, affine=True, track_running_stats=True)
(activation): LeakyReLU(negative_slope=0.1)
)
(58): WeightedFeatureFusion()
(59): Sequential(
(Conv2d): Conv2d(512, 256, kernel_size=(1, 1), stride=(1, 1), bias=False)
(BatchNorm2d): BatchNorm2d(256, eps=1e-05, momentum=0.1, affine=True, track_running_stats=True)
(activation): LeakyReLU(negative_slope=0.1)
)
(60): Sequential(
(Conv2d): Conv2d(256, 512, kernel_size=(3, 3), stride=(1, 1), padding=(1, 1), bias=False)
(BatchNorm2d): BatchNorm2d(512, eps=1e-05, momentum=0.1, affine=True, track_running_stats=True)
(activation): LeakyReLU(negative_slope=0.1)
)
(61): WeightedFeatureFusion()
(62): Sequential(
(Conv2d): Conv2d(512, 1024, kernel_size=(3, 3), stride=(2, 2), padding=(1, 1), bias=False)
(BatchNorm2d): BatchNorm2d(1024, eps=1e-05, momentum=0.1, affine=True, track_running_stats=True)
(activation): LeakyReLU(negative_slope=0.1)
)
(63): Sequential(
(Conv2d): Conv2d(1024, 512, kernel_size=(1, 1), stride=(1, 1), bias=False)
(BatchNorm2d): BatchNorm2d(512, eps=1e-05, momentum=0.1, affine=True, track_running_stats=True)
(activation): LeakyReLU(negative_slope=0.1)
)
(64): Sequential(
(Conv2d): Conv2d(512, 1024, kernel_size=(3, 3), stride=(1, 1), padding=(1, 1), bias=False)
(BatchNorm2d): BatchNorm2d(1024, eps=1e-05, momentum=0.1, affine=True, track_running_stats=True)
(activation): LeakyReLU(negative_slope=0.1)
)
(65): WeightedFeatureFusion()
(66): Sequential(
(Conv2d): Conv2d(1024, 512, kernel_size=(1, 1), stride=(1, 1), bias=False)
(BatchNorm2d): BatchNorm2d(512, eps=1e-05, momentum=0.1, affine=True, track_running_stats=True)
(activation): LeakyReLU(negative_slope=0.1)
)
(67): Sequential(
(Conv2d): Conv2d(512, 1024, kernel_size=(3, 3), stride=(1, 1), padding=(1, 1), bias=False)
(BatchNorm2d): BatchNorm2d(1024, eps=1e-05, momentum=0.1, affine=True, track_running_stats=True)
(activation): LeakyReLU(negative_slope=0.1)
)
(68): WeightedFeatureFusion()
(69): Sequential(
(Conv2d): Conv2d(1024, 512, kernel_size=(1, 1), stride=(1, 1), bias=False)
(BatchNorm2d): BatchNorm2d(512, eps=1e-05, momentum=0.1, affine=True, track_running_stats=True)
(activation): LeakyReLU(negative_slope=0.1)
)
(70): Sequential(
(Conv2d): Conv2d(512, 1024, kernel_size=(3, 3), stride=(1, 1), padding=(1, 1), bias=False)
(BatchNorm2d): BatchNorm2d(1024, eps=1e-05, momentum=0.1, affine=True, track_running_stats=True)
(activation): LeakyReLU(negative_slope=0.1)
)
(71): WeightedFeatureFusion()
(72): Sequential(
(Conv2d): Conv2d(1024, 512, kernel_size=(1, 1), stride=(1, 1), bias=False)
(BatchNorm2d): BatchNorm2d(512, eps=1e-05, momentum=0.1, affine=True, track_running_stats=True)
(activation): LeakyReLU(negative_slope=0.1)
)
(73): Sequential(
(Conv2d): Conv2d(512, 1024, kernel_size=(3, 3), stride=(1, 1), padding=(1, 1), bias=False)
(BatchNorm2d): BatchNorm2d(1024, eps=1e-05, momentum=0.1, affine=True, track_running_stats=True)
(activation): LeakyReLU(negative_slope=0.1)
)
(74): WeightedFeatureFusion()
(75): Sequential(
(Conv2d): Conv2d(1024, 512, kernel_size=(1, 1), stride=(1, 1), bias=False)
(BatchNorm2d): BatchNorm2d(512, eps=1e-05, momentum=0.1, affine=True, track_running_stats=True)
(activation): LeakyReLU(negative_slope=0.1)
)
(76): Sequential(
(Conv2d): Conv2d(512, 1024, kernel_size=(3, 3), stride=(1, 1), padding=(1, 1), bias=False)
(BatchNorm2d): BatchNorm2d(1024, eps=1e-05, momentum=0.1, affine=True, track_running_stats=True)
(activation): LeakyReLU(negative_slope=0.1)
)
(77): Sequential(
(Conv2d): Conv2d(1024, 512, kernel_size=(1, 1), stride=(1, 1), bias=False)
(BatchNorm2d): BatchNorm2d(512, eps=1e-05, momentum=0.1, affine=True, track_running_stats=True)
(activation): LeakyReLU(negative_slope=0.1)
)
(78): Sequential(
(Conv2d): Conv2d(512, 1024, kernel_size=(3, 3), stride=(1, 1), padding=(1, 1), bias=False)
(BatchNorm2d): BatchNorm2d(1024, eps=1e-05, momentum=0.1, affine=True, track_running_stats=True)
(activation): LeakyReLU(negative_slope=0.1)
)
(79): Sequential(
(Conv2d): Conv2d(1024, 512, kernel_size=(1, 1), stride=(1, 1), bias=False)
(BatchNorm2d): BatchNorm2d(512, eps=1e-05, momentum=0.1, affine=True, track_running_stats=True)
(activation): LeakyReLU(negative_slope=0.1)
)
(80): Sequential(
(Conv2d): Conv2d(512, 1024, kernel_size=(3, 3), stride=(1, 1), padding=(1, 1), bias=False)
(BatchNorm2d): BatchNorm2d(1024, eps=1e-05, momentum=0.1, affine=True, track_running_stats=True)
(activation): LeakyReLU(negative_slope=0.1)
)
(81): Sequential(
(Conv2d): Conv2d(1024, 24, kernel_size=(1, 1), stride=(1, 1))
)
(82): YOLOLayer()
(83): FeatureConcat()
(84): Sequential(
(Conv2d): Conv2d(512, 256, kernel_size=(1, 1), stride=(1, 1), bias=False)
(BatchNorm2d): BatchNorm2d(256, eps=1e-05, momentum=0.1, affine=True, track_running_stats=True)
(activation): LeakyReLU(negative_slope=0.1)
)
(85): Upsample(scale_factor=2.0, mode=nearest)
(86): FeatureConcat()
(87): Sequential(
(Conv2d): Conv2d(768, 256, kernel_size=(1, 1), stride=(1, 1), bias=False)
(BatchNorm2d): BatchNorm2d(256, eps=1e-05, momentum=0.1, affine=True, track_running_stats=True)
(activation): LeakyReLU(negative_slope=0.1)
)
(88): Sequential(
(Conv2d): Conv2d(256, 512, kernel_size=(3, 3), stride=(1, 1), padding=(1, 1), bias=False)
(BatchNorm2d): BatchNorm2d(512, eps=1e-05, momentum=0.1, affine=True, track_running_stats=True)
(activation): LeakyReLU(negative_slope=0.1)
)
(89): Sequential(
(Conv2d): Conv2d(512, 256, kernel_size=(1, 1), stride=(1, 1), bias=False)
(BatchNorm2d): BatchNorm2d(256, eps=1e-05, momentum=0.1, affine=True, track_running_stats=True)
(activation): LeakyReLU(negative_slope=0.1)
)
(90): Sequential(
(Conv2d): Conv2d(256, 512, kernel_size=(3, 3), stride=(1, 1), padding=(1, 1), bias=False)
(BatchNorm2d): BatchNorm2d(512, eps=1e-05, momentum=0.1, affine=True, track_running_stats=True)
(activation): LeakyReLU(negative_slope=0.1)
)
(91): Sequential(
(Conv2d): Conv2d(512, 256, kernel_size=(1, 1), stride=(1, 1), bias=False)
(BatchNorm2d): BatchNorm2d(256, eps=1e-05, momentum=0.1, affine=True, track_running_stats=True)
(activation): LeakyReLU(negative_slope=0.1)
)
(92): Sequential(
(Conv2d): Conv2d(256, 512, kernel_size=(3, 3), stride=(1, 1), padding=(1, 1), bias=False)
(BatchNorm2d): BatchNorm2d(512, eps=1e-05, momentum=0.1, affine=True, track_running_stats=True)
(activation): LeakyReLU(negative_slope=0.1)
)
(93): Sequential(
(Conv2d): Conv2d(512, 24, kernel_size=(1, 1), stride=(1, 1))
)
(94): YOLOLayer()
(95): FeatureConcat()
(96): Sequential(
(Conv2d): Conv2d(256, 128, kernel_size=(1, 1), stride=(1, 1), bias=False)
(BatchNorm2d): BatchNorm2d(128, eps=1e-05, momentum=0.1, affine=True, track_running_stats=True)
(activation): LeakyReLU(negative_slope=0.1)
)
(97): Upsample(scale_factor=2.0, mode=nearest)
(98): FeatureConcat()
(99): Sequential(
(Conv2d): Conv2d(384, 128, kernel_size=(1, 1), stride=(1, 1), bias=False)
(BatchNorm2d): BatchNorm2d(128, eps=1e-05, momentum=0.1, affine=True, track_running_stats=True)
(activation): LeakyReLU(negative_slope=0.1)
)
(100): Sequential(
(Conv2d): Conv2d(128, 256, kernel_size=(3, 3), stride=(1, 1), padding=(1, 1), bias=False)
(BatchNorm2d): BatchNorm2d(256, eps=1e-05, momentum=0.1, affine=True, track_running_stats=True)
(activation): LeakyReLU(negative_slope=0.1)
)
(101): Sequential(
(Conv2d): Conv2d(256, 128, kernel_size=(1, 1), stride=(1, 1), bias=False)
(BatchNorm2d): BatchNorm2d(128, eps=1e-05, momentum=0.1, affine=True, track_running_stats=True)
(activation): LeakyReLU(negative_slope=0.1)
)
(102): Sequential(
(Conv2d): Conv2d(128, 256, kernel_size=(3, 3), stride=(1, 1), padding=(1, 1), bias=False)
(BatchNorm2d): BatchNorm2d(256, eps=1e-05, momentum=0.1, affine=True, track_running_stats=True)
(activation): LeakyReLU(negative_slope=0.1)
)
(103): Sequential(
(Conv2d): Conv2d(256, 128, kernel_size=(1, 1), stride=(1, 1), bias=False)
(BatchNorm2d): BatchNorm2d(128, eps=1e-05, momentum=0.1, affine=True, track_running_stats=True)
(activation): LeakyReLU(negative_slope=0.1)
)
(104): Sequential(
(Conv2d): Conv2d(128, 256, kernel_size=(3, 3), stride=(1, 1), padding=(1, 1), bias=False)
(BatchNorm2d): BatchNorm2d(256, eps=1e-05, momentum=0.1, affine=True, track_running_stats=True)
(activation): LeakyReLU(negative_slope=0.1)
)
(105): Sequential(
(Conv2d): Conv2d(256, 24, kernel_size=(1, 1), stride=(1, 1))
)
(106): YOLOLayer()
)
)
转换代码
项目下载
在u版的yolo目录下运行(包含所需的model) 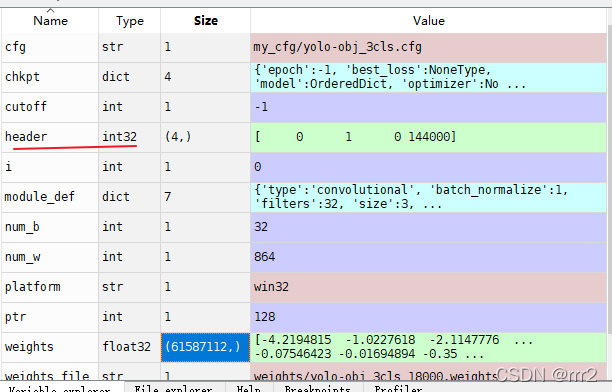
weights2pt转换代码
以yolov3 yolov3-spp为例
import torch
#from models import Darknet, load_darknet_weights
from models import Darknet
import numpy as np
import os
def load_darknet_weights(self, weights, cutoff=-1):
# Parses and loads the weights stored in 'weights'
# cutoff: save layers between 0 and cutoff (if cutoff = -1 all are saved)
weights_file = weights.split(os.sep)[-1]
# Try to download weights if not available locally
# if not os.path.isfile(weights):
# try:
# os.system('wget https://pjreddie.com/media/files/' + weights_file + ' -O ' + weights)
# except IOError:
# print(weights + ' not found.\nTry https://drive.google.com/drive/folders/1uxgUBemJVw9wZsdpboYbzUN4bcRhsuAI')
# Establish cutoffs
if weights_file == 'darknet53.conv.74':
cutoff = 75
elif weights_file == 'yolov3-tiny.conv.15':
cutoff = 15
# Open the weights file
with open(weights, 'rb') as f:
header = np.fromfile(f, dtype=np.int32, count=4) #yolov3是4 yolov3-spp以上是5 First five are header values
# Needed to write header when saving weights
self.header_info = header
self.seen = header[3] # number of images seen during training
weights = np.fromfile(f, dtype=np.float32) # The rest are weights
ptr = 0
for i, (module_def, module) in enumerate(zip(self.module_defs[:cutoff], self.module_list[:cutoff])):
if module_def['type'] == 'convolutional':
conv_layer = module[0]
if module_def['batch_normalize']:
# Load BN bias, weights, running mean and running variance
bn_layer = module[1]
num_b = bn_layer.bias.numel() # Number of biases
# Bias
bn_b = torch.from_numpy(weights[ptr:ptr + num_b]).view_as(bn_layer.bias)
bn_layer.bias.data.copy_(bn_b)
ptr += num_b
# Weight
bn_w = torch.from_numpy(weights[ptr:ptr + num_b]).view_as(bn_layer.weight)
bn_layer.weight.data.copy_(bn_w)
ptr += num_b
# Running Mean
bn_rm = torch.from_numpy(weights[ptr:ptr + num_b]).view_as(bn_layer.running_mean)
bn_layer.running_mean.data.copy_(bn_rm)
ptr += num_b
# Running Var
bn_rv = torch.from_numpy(weights[ptr:ptr + num_b]).view_as(bn_layer.running_var)
bn_layer.running_var.data.copy_(bn_rv)
ptr += num_b
else:
# Load conv. bias
num_b = conv_layer.bias.numel()
conv_b = torch.from_numpy(weights[ptr:ptr + num_b]).view_as(conv_layer.bias)
conv_layer.bias.data.copy_(conv_b)
ptr += num_b
# Load conv. weights
num_w = conv_layer.weight.numel()
conv_w = torch.from_numpy(weights[ptr:ptr + num_w]).view_as(conv_layer.weight)
conv_layer.weight.data.copy_(conv_w)
ptr += num_w
return cutoff
device = torch.device("cuda:0" if torch.cuda.is_available() else "cpu")
cfg = 'my_cfg/yolo-obj_3cls.cfg'
weights = 'weights/yolo-obj_3cls_18000.weights'
model = Darknet(cfg).to(device)
load_darknet_weights(model, weights)
chkpt = {'epoch': -1, 'best_loss': None, 'model': model.state_dict(), 'optimizer': None}
torch.save(chkpt, 'weights/yolo-obj_3cls.pt')
pt2weights
from models import * 注意:新建的pt2weights.py与models.py在同一目录下
cfg = 'my_cfg/yolo-obj_3cls.cfg'
model=Darknet(cfg) #训练时候用的cfg 改成自己.cfg的地址
checkpoint = torch.load("weights/yolo-obj_3cls.pt",map_location='cpu')#导入训练好的.pt文件 改成自己的.pt文件地址
model.load_state_dict(checkpoint['model'])
save_weights(model,path='weights/bt2weights_obj_3cls.weights',cutoff=-1)#生成.weights文件 改成自己的地址
checkpint内容
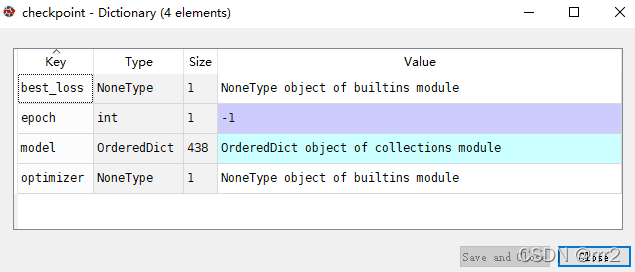
{'epoch': -1, 'best_loss': None, 'model': OrderedDict([
('module_list.0.conv_0.weight', tensor([[[[ 1.22904e-01, 1.03453e-01, 8.72016e-02],
[ 1.00648e-01, 7.50468e-02, 9.15262e-02],
[-6.72630e-03, -2.11742e-02, 2.15247e-02]], [[-3.27195e-02, 1.09584e-01, 1.08887e-01]
,.....[-2.40979e-02, 6.60297e-02, 5.63852e-02],[-4.11258e-02, -1.59853e-02, -2.48185e-02]]]])),
('module_list.0.batch_norm_0.weight', tensor([ 2.81073, 0.97984, 1.30070, 0.61605, -1.12916, 2.66054, 0.73914, 0.63000, 0.75663, -0.47399, 0.97683, 0.94648, 1.12235, 1.35054, 2.16467, 1.36777, 0.93587, 1.80257, 1.42253, 1.00391, 1.39467, -1.76530, -0.76111, -0.79642, 0.63124, 1.64442, 1.34087, 0.86794, 2.53687, 2.07082, 0.58116, 1.69326])),
('module_list.0.batch_norm_0.bias', tensor([-4.21948, -1.02276, -2.11478, 1.32232, -0.79700, -3.23063, -0.32061, 0.02714, 1.69935, 0.75224, -0.87066, 3.32068, 2.53358, -2.02973, -3.66029, -1.94644, 3.56482, -1.57652, -1.98882, -0.84602, -2.13664, -2.77446, 1.45947, 0.27386, 1.32830, -2.41020, 2.64488, -1.26660, -2.85382, -2.76217, -0.07457, -2.27203])),
('module_list.0.batch_norm_0.running_mean', tensor([ 3.47822e-01, -1.38476e-01, 1.28535e-01, 1.99359e-01, -1.43450e-01, 1.93027e-01, -1.26285e-01, 5.53209e-02, -2.10289e-01, -2.40412e-01, -8.84716e-02, -2.61738e-05, -2.29773e-02, 9.82087e-02, 2.56201e-01, 1.20729e-01, -1.49580e-01, -1.24053e-01, 1.10301e-01, -6.28857e-02, -1.15364e-01, 1.67073e-01, -2.27964e-01, -4.17056e-01, -4.56573e-02, 1.28036e-01, 1.88810e-02, -1.37764e-02, -2.29639e-01, -3.33596e-01, -1.09311e-01, 2.01629e-01])),
('module_list.0.batch_norm_0.running_var', tensor([0.07550, 0.01245, 0.01136, 0.02681, 0.01321, 0.02595, 0.01172, 0.02844, 0.03290, 0.03974, 0.00646, 0.00892, 0.01218, 0.00612, 0.04389, 0.00941, 0.02433, 0.01332, 0.00934, 0.00453, 0.00895, 0.01764, 0.03760, 0.11741, 0.03504, 0.01099, 0.01229, 0.00182, 0.03769, 0.07087, 0.01180, 0.02624])), ('module_list.0.batch_norm_0.num_batches_tracked', tensor(0)),
......
|