模块图示
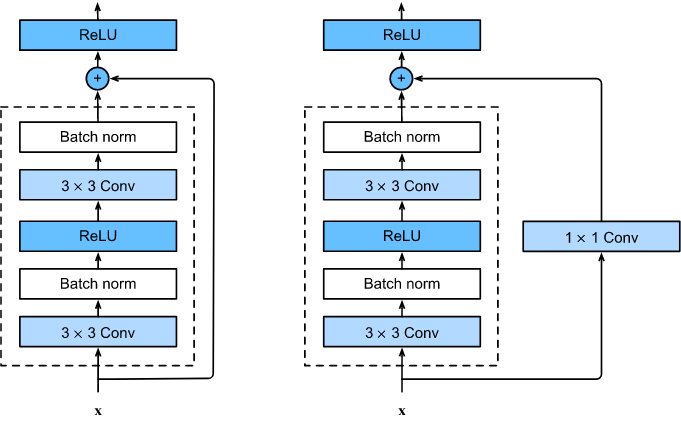
模块介绍
如图左所示,假设输入为x,然后来了一个‘并联’,假设x经过虚线框操作后输出的结果为x1,在汇合的地方输出结果为out,那么out=x+x1。为了使x和x1能够相加,其两者维度需相同,也就是x1的维度要与x相同。
模块作用
解决VGG由于层数过多,网络过深产生的梯度爆炸、过拟合问题
代码实现
下面实现上图左边的Residule block。
import torch
import torch.nn as nn
import torch.nn.functional as F
class ResNet_basic_block(nn.Module):
def __init__(self, in_channels, out_channels):
super().__init__()
self.conv1 = nn.Conv2d(in_channels = in_channels,
out_channels = out_channels,
kernel_size = 3,
padding = 1,
bias = False)
self.bn1 = nn.BatchNorm2d(num_features = out_channels)
self.conv2 = nn.Conv2d(in_channels = out_channels,
out_channels = out_channels,
kernel_size = 3,
padding = 1,
bias = False)
self.bn2 = nn.BatchNorm2d(num_features = out_channels)
def forward(self, x):
residual = x,
out = self.conv1(x)
out = self.bn1(out)
out = F.relu(self.bn1(out), inplace = True)
out = self.conv2(out)
out = self.bn2(out)
return out
模块使用实例
net = ResNet_basic_block(in_channels=3, out_channels=3)
x = torch.rand((1,3,128,128), requires_grad=True)
print(x)
>>>
tensor([[[[0.1748, 0.7122, 0.5482, ..., 0.3072, 0.5013, 0.3448],
[0.9843, 0.9961, 0.2204, ..., 0.7938, 0.0166, 0.4661],
[0.0247, 0.0084, 0.0705, ..., 0.2160, 0.4828, 0.5090],
...,
[0.9613, 0.8825, 0.5579, ..., 0.0887, 0.8651, 0.5624],
[0.9226, 0.5717, 0.7671, ..., 0.9176, 0.2652, 0.0017],
[0.3222, 0.0448, 0.1637, ..., 0.4346, 0.4602, 0.1887]],
[[0.6197, 0.8704, 0.3110, ..., 0.9539, 0.3757, 0.4366],
[0.8575, 0.0412, 0.8464, ..., 0.5786, 0.8352, 0.1744],
[0.0278, 0.0901, 0.1685, ..., 0.1698, 0.1893, 0.8004],
...,
[0.9396, 0.6551, 0.0380, ..., 0.8259, 0.5549, 0.8349],
[0.2380, 0.9816, 0.4802, ..., 0.0942, 0.5014, 0.6619],
[0.2772, 0.9087, 0.0889, ..., 0.3405, 0.0918, 0.7940]]]],
requires_grad=True)
a = net(x)
print(a)
tensor([[[[ 2.6107e+00, 4.9241e+00, 4.4753e+00, ..., 3.0504e+00,
2.5287e+00, 1.6026e+00],
[ 4.4550e-01, -1.5947e+00, -1.3323e+00, ..., -2.5174e+00,
-2.5517e+00, -1.4408e+00],
[ 3.1338e-01, 3.2004e-01, 5.9879e-01, ..., -1.4324e+00,
1.4006e+00, -1.9084e-01],
...,
[ 4.8046e-01, 1.2165e+00, 1.5494e+00, ..., 1.1277e+00,
-1.0431e+00, -8.0495e-01],
[-1.9110e+00, -1.5171e+00, 3.0137e-03, ..., 4.9892e-01,
1.4297e+00, 1.3426e-02],
[-1.1890e+00, -4.4962e-01, -1.2672e+00, ..., -1.8782e+00,
-1.7202e+00, -2.0879e+00]]]], grad_fn=<NativeBatchNormBackward>)
如何在一个CNN中加入Residule block----代码
-------定义网络模型------
class Net(nn.Module):
def __init__(self):
super(Net, self).__init__()
self.conv1 = nn.Conv2d(3, 32, 3)
self.pool = nn.MaxPool2d(2, 2)
self.bn1 = nn.BatchNorm2d(32)
self.conv2 = nn.Conv2d(32, 64, 3)
self.pool = nn.MaxPool2d(2, 2)
self.bn2 = nn.BatchNorm2d(64)
self.conv3 = nn.Conv2d(64, 64, 3)
self.pool = nn.MaxPool2d(2, 2)
self.bn3 = nn.BatchNorm2d(64)
self.conv3 = nn.Conv2d(64, 64, 3)
self.drop1d = nn.Dropout(0.2)
self.bn4 = nn.BatchNorm2d(64)
self.fc1 = nn.Linear(64 * 14 * 14, 1024)
self.fc2 = nn.Linear(1024, 256)
self.fc3 = nn.Linear(256, 4)
def forward(self, x):
x = self.pool(F.relu(self.conv1(x)))
x = self.bn1(x)
x = self.pool(F.relu(self.conv2(x)))
x = self.bn2(x)
x = self.pool(F.relu(self.conv3(x)))
x = self.bn3(x)
x = x.view(-1, x.size(1) * x.size(2) * x.size(3))
x = F.relu(self.fc1(x))
x = self.drop1d(x)
x = F.relu(self.fc2(x))
x = self.drop1d(x)
x = self.fc3(x)
return x
建立了一个卷积–池化–卷积–池化–卷积–池化–全连接–全连接–全连接(输出为4分类)
Model = Net()
x = torch.randn(32, 3, 128, 128)
model(x)
>>>
tensor([[ 2.4648, -0.1044, -0.0207, ..., -1.5143, -0.0301, 0.4112],
[-1.1457, 1.1705, -1.1644, ..., -0.6782, 1.5006, 0.6034],
[ 1.8320, 2.3075, 0.9986, ..., -0.7777, -0.5291, 0.7829],
...,
[-0.4622, -0.6317, 0.4479, ..., 2.8768, -0.5076, 2.9432],
[-0.9910, -0.6667, 0.5861, ..., -0.1901, -2.6266, 0.2797],
[-1.4598, -0.4183, -1.2018, ..., -2.0129, 1.1534, 1.2424]],
grad_fn=<AddmmBackward>)
现在问题是如何在普通的CNN网络中添加Residule block
class Net(nn.Module):
def __init__(self):
super(Net, self).__init__()
self.conv1 = nn.Conv2d(3, 32, 3)
self.pool = nn.MaxPool2d(2, 2)
self.bn1 = nn.BatchNorm2d(32)
self.res = ResNet_basic_block(in_channels=32, out_channels=32)
self.conv2 = nn.Conv2d(32, 64, 3)
self.pool = nn.MaxPool2d(2, 2)
self.bn2 = nn.BatchNorm2d(64)
self.conv3 = nn.Conv2d(64, 64, 3)
self.pool = nn.MaxPool2d(2, 2)
self.bn3 = nn.BatchNorm2d(64)
self.conv3 = nn.Conv2d(64, 64, 3)
self.drop1d = nn.Dropout(0.2)
self.bn4 = nn.BatchNorm2d(64)
self.fc1 = nn.Linear(64 * 14 * 14, 1024)
self.fc2 = nn.Linear(1024, 256)
self.fc3 = nn.Linear(256, 4)
def forward(self, x):
x = self.pool(F.relu(self.conv1(x)))
x = self.bn1(x)
x = self.res(x)
x = self.pool(F.relu(self.conv2(x)))
x = self.bn2(x)
x = self.pool(F.relu(self.conv3(x)))
x = self.bn3(x)
x = x.view(-1, x.size(1) * x.size(2) * x.size(3))
x = F.relu(self.fc1(x))
x = self.drop1d(x)
x = F.relu(self.fc2(x))
x = self.drop1d(x)
x = self.fc3(x)
return x
Model = Net()
x = torch.randn(32, 3, 128, 128)
model(x)
>>>
tensor([[ 1.5100e+00, -7.4613e-01, 8.7256e-01, ..., 3.0170e+00,
-3.0221e-01, -1.8396e+00],
[-6.5097e-01, -9.8755e-03, -1.5788e-01, ..., 1.6645e+00,
9.5299e-01, 8.2736e-01],
[-1.8710e+00, 2.0923e-01, 4.7972e-01, ..., 1.8698e-01,
1.8506e-01, 1.6153e-04],
...,
[ 5.4644e+00, 1.8698e+00, -7.3383e-01, ..., 6.9908e-01,
-1.3000e+00, 1.7883e+00],
[-6.2972e-02, -2.3328e+00, -2.5254e-01, ..., 1.5666e+00,
8.7195e-01, -2.8013e-01],
[-1.5590e+00, -2.7793e+00, -1.5177e+00, ..., -1.2423e+00,
-4.3381e-01, -6.5499e-01]], grad_fn=<AddmmBackward>)
模型输出结果了,大工完成
欢迎关注gzh:故障诊断与python学习
|