CBOW 模型的学习的实现:给神经网络准备好学习数据。然后求梯度,并逐步更新权重参数。
Trainer类:学习的类。
初始化:类的初始化程序接收神经网络(模型)和优化器(SGD、Momentum、AdaGrad、Adam)
学习:调用 fit() 方法开始学习。参数:x,输入数据;t,监督标签;max_epoch,进行学习的 epoch 数;batch_size,mini-batch 的大小;eval_interval,输出结果(平均损失等)的间隔。 例如设置 eval_interval=20,则每 20 次迭代计算 1 次平均损失, 并将结果输出到界面上;max_grad,梯度的最大范数。 当梯度的范数超过这个值时,缩小梯度。
def fit(self, x, t, max_epoch=10, batch_size=32, max_grad=None, eval_interval=20):
plot方法:画出 fit() 方法记录的损失(按照 eval_interval 评价的平均损失)。
class Trainer:
def __init__(self, model, optimizer):
self.model = model
self.optimizer = optimizer
self.loss_list = []
self.eval_interval = None
self.current_epoch = 0
def fit(self, x, t, max_epoch=10, batch_size=32, max_grad=None, eval_interval=20):
data_size = len(x)
max_iters = data_size // batch_size
self.eval_interval = eval_interval
model, optimizer = self.model, self.optimizer
total_loss = 0
loss_count = 0
start_time = time.time()
for epoch in range(max_epoch):
idx = numpy.random.permutation(numpy.arange(data_size))
x = x[idx]
t = t[idx]
for iters in range(max_iters):
batch_x = x[iters*batch_size:(iters+1)*batch_size]
batch_t = t[iters*batch_size:(iters+1)*batch_size]
loss = model.forward(batch_x, batch_t)
model.backward()
params, grads = remove_duplicate(model.params, model.grads)
if max_grad is not None:
clip_grads(grads, max_grad)
optimizer.update(params, grads)
total_loss += loss
loss_count += 1
if (eval_interval is not None) and (iters % eval_interval) == 0:
avg_loss = total_loss / loss_count
elapsed_time = time.time() - start_time
print('| epoch %d | iter %d / %d | time %d[s] | loss %.2f'
% (self.current_epoch + 1, iters + 1, max_iters, elapsed_time, avg_loss))
self.loss_list.append(float(avg_loss))
total_loss, loss_count = 0, 0
self.current_epoch += 1
def plot(self, ylim=None):
x = numpy.arange(len(self.loss_list))
if ylim is not None:
plt.ylim(*ylim)
plt.plot(x, self.loss_list, label='train')
plt.xlabel('iterations (x' + str(self.eval_interval) + ')')
plt.ylabel('loss')
plt.show()
这里面使用Trainer 类来执行CBOW 模型的学习。
这个model其实存的就是SimpleCBOW的成员变量。
model = SimpleCBOW(vocab_size, hidden_size)
下面是调用Trainer 类:
trainer = Trainer(model, optimizer)
trainer.fit(contexts, target, max_epoch, batch_size)
trainer.plot()
import sys
sys.path.append('..')
from common.trainer import Trainer
from common.optimizer import Adam
from simple_cbow import SimpleCBOW
from common.util import preprocess, create_contexts_target, convert_one_hot
window_size = 1
hidden_size = 5
batch_size = 3
max_epoch = 1000
text = 'You say goodbye and I say hello.'
corpus, word_to_id, id_to_word = preprocess(text)
vocab_size = len(word_to_id)
contexts, target = create_contexts_target(corpus, window_size)
target = convert_one_hot(target, vocab_size)
contexts = convert_one_hot(contexts, vocab_size)
model = SimpleCBOW(vocab_size, hidden_size)
optimizer = Adam()
trainer = Trainer(model, optimizer)
trainer.fit(contexts, target, max_epoch, batch_size)
trainer.plot()
word_vecs = model.word_vecs
for word_id, word in id_to_word.items():
print(word, word_vecs[word_id])
结果:
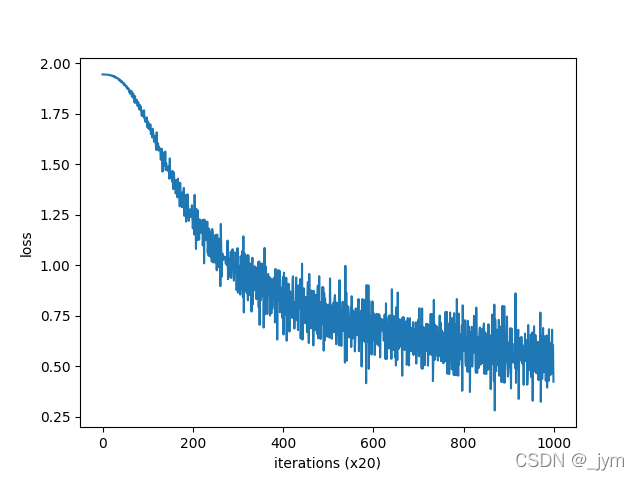
SimpleCBOW类里面成员变量有下面这个:权重矩阵W_in就是单词的分布式表示。
# 将单词的分布式表示设置为成员变量
self.word_vecs = W_in
那就可以看看单词的分布式表示。
word_vecs = model.word_vecs
for word_id, word in id_to_word.items():
print(word, word_vecs[word_id])
结果如下:可见,单词表示为了密集向量
you [-0.9987413 1.0136298 -1.4921554 0.97300434 1.0181936 ]
say [ 1.161595 -1.1513934 -0.25779223 -1.1773298 -1.1531342 ]
goodbye [-0.88470864 0.9155085 -0.30859873 0.9318609 0.9092796 ]
and [ 0.7929211 -0.8148116 -1.8787507 -0.7845257 -0.8028278]
i [-0.8925459 0.95505357 -0.29667985 0.90895575 0.90703803]
hello [-1.0259517 0.97562104 -1.5057516 0.96239203 1.0297285 ]
. [ 1.2134467 -1.1766206 1.6439314 -1.1993438 -1.1676227]
这里面为啥是5个数,其实还是在于权重矩阵W。在SimpleCBOW类里面W_in大小是跟单词数目和hidden_size有关的。
V, H = vocab_size, hidden_size
W_in = 0.01 * np.random.randn(V, H).astype('f')
在使用Trainer 类来执行CBOW 模型的学习时,设置的hidden_size = 5,所以最后单词就表示成包含五个数的向量了。
CBOW模型的学习:调整权重,以使预测准确。也就是说,上下文是 you 和 goodbye,正确解标签应该是 say,那么如果网络具有良好的权重,对应正确解的神经元(say)的得分应该更高。
对神经网络进行学习,其实是用了Softmax 函数和交叉熵误差。使用 Softmax 函数将得分转化为概率,再求这些概率和监督标签之间的交叉熵误差,并将其作为损失进行学习。推理的 CBOW 模型加上 Softmax 层和 Cross Entropy Error 层,就可以得到损失。
输入侧和输出侧的权重都可以被视为单词的分布式表示,这里面只使用输入侧的权重作为单词的分布式表示。
最后把之前写的CBOW模型类放上来:
class SimpleCBOW:
def __init__(self, vocab_size, hidden_size):
V, H = vocab_size, hidden_size
W_in = 0.01 * np.random.randn(V, H).astype('f')
W_out = 0.01 * np.random.randn(H, V).astype('f')
self.in_layer0 = MatMul(W_in)
self.in_layer1 = MatMul(W_in)
self.out_layer = MatMul(W_out)
self.loss_layer = SoftmaxWithLoss()
layers = [self.in_layer0, self.in_layer1, self.out_layer]
self.params, self.grads = [], []
for layer in layers:
self.params += layer.params
self.grads += layer.grads
self.word_vecs = W_in
def forward(self, contexts, target):
h0 = self.in_layer0.forward(contexts[:, 0])
h1 = self.in_layer1.forward(contexts[:, 1])
h = (h0 + h1) * 0.5
score = self.out_layer.forward(h)
loss = self.loss_layer.forward(score, target)
return loss
def backward(self, dout=1):
ds = self.loss_layer.backward(dout)
da = self.out_layer.backward(ds)
da *= 0.5
self.in_layer1.backward(da)
self.in_layer0.backward(da)
return None
|