optimtool测试文档
Github项目源码:https://github.com/linjing-lab/optimtool
GitCode项目源码:https://gitcode.net/linjing_zyq/optimtool
pip install optimtool
1. 无约束优化算法性能对比
前五个参数完全一致,其中第四个参数是绘图接口,默认绘制单个算法的迭代过程;第五个参数是输出函数迭代值接口,默认为不输出。
method :用于传递线搜索方式
- from optimtool.unconstrain import gradient_descent
方法 | 函数参数 | 调用示例 |
---|
解方程得到精确解法(solve) | solve(funcs, args, x_0, draw=True, output_f=False, epsilon=1e-10, k=0) | gradient_descent.solve(funcs, args, x_0) | 基于Grippo非单调线搜索的梯度下降法 | barzilar_borwein(funcs, args, x_0, draw=True, output_f=False, method="grippo", M=20, c1=0.6, beta=0.6, alpha=1, epsilon=1e-10, k=0) | gradient_descent.barzilar_borwein(funcs, args, x_0, method=“grippo”) | 基于ZhangHanger非单调线搜索的梯度下降法 | barzilar_borwein(funcs, args, x_0, draw=True, output_f=False, method="ZhangHanger", M=20, c1=0.6, beta=0.6, alpha=1, epsilon=1e-10, k=0) | gradient_descent.barzilar_borwein(funcs, args, x_0, method=“ZhangHanger”) | 基于最速下降法的梯度下降法 | steepest(funcs, args, x_0, draw=True, output_f=False, method="wolfe", epsilon=1e-10, k=0) | gradient_descent.steepest(funcs, args, x_0) |
- from optimtool.unconstrain import newton
方法 | 函数参数 | 调用示例 |
---|
经典牛顿法 | classic(funcs, args, x_0, draw=True, output_f=False, epsilon=1e-10, k=0) | newton.classic(funcs, args, x_0) | 基于armijo线搜索方法的修正牛顿法 | modified(funcs, args, x_0, draw=True, output_f=False, method="armijo", m=20, epsilon=1e-10, k=0) | newton.modified(funcs, args, x_0, method=“armijo”) | 基于goldstein线搜索方法的修正牛顿法 | modified(funcs, args, x_0, draw=True, output_f=False, method="goldstein", m=20, epsilon=1e-10, k=0) | newton.modified(funcs, args, x_0, method=“goldstein”) | 基于wolfe线搜索方法的修正牛顿法 | modified(funcs, args, x_0, draw=True, output_f=False, method="wolfe", m=20, epsilon=1e-10, k=0) | newton.modified(funcs, args, x_0, method=“wolfe”) | 基于armijo线搜索方法的非精确牛顿法 | CG(funcs, args, x_0, draw=True, output_f=False, method="armijo", epsilon=1e-6, k=0) | newton.CG(funcs, args, x_0, method=“armijo”) | 基于goldstein线搜索方法的非精确牛顿法 | CG(funcs, args, x_0, draw=True, output_f=False, method="goldstein", epsilon=1e-6, k=0) | newton.CG(funcs, args, x_0, method=“goldstein”) | 基于wolfe线搜索方法的非精确牛顿法 | CG(funcs, args, x_0, draw=True, output_f=False, method="wolfe", epsilon=1e-6, k=0) | newton.CG(funcs, args, x_0, method=“wolfe”) |
- from optimtool.unconstrain import newton_quasi
方法 | 函数参数 | 调用示例 |
---|
基于BFGS方法更新海瑟矩阵的拟牛顿法 | bfgs(funcs, args, x_0, draw=True, output_f=False, method="wolfe", m=20, epsilon=1e-10, k=0) | newton_quasi.bfgs(funcs, args, x_0) | 基于DFP方法更新海瑟矩阵的拟牛顿法 | dfp(funcs, args, x_0, draw=True, output_f=False, method="wolfe", m=20, epsilon=1e-4, k=0) | newton_quasi.dfp(funcs, args, x_0) | 基于有限内存BFGS方法更新海瑟矩阵的拟牛顿法 | L_BFGS(funcs, args, x_0, draw=True, output_f=False, method="wolfe", m=6, epsilon=1e-10, k=0) | newton_quasi.L_BFGS(funcs, args, x_0) |
- from optimtool.unconstrain import trust_region
方法 | 函数参数 | 调用示例 |
---|
基于截断共轭梯度法的信赖域算法 | steihaug_CG(funcs, args, x_0, draw=True, output_f=False, m=100, r0=1, rmax=2, eta=0.2, p1=0.4, p2=0.6, gamma1=0.5, gamma2=1.5, epsilon=1e-6, k=0) | trust_region.steihaug_CG(funcs, args, x_0) |
import sympy as sp
import matplotlib.pyplot as plt
import optimtool as oo
f, x1, x2, x3, x4 = sp.symbols("f x1 x2 x3 x4")
f = (x1 - 1)**2 + (x2 - 1)**2 + (x3 - 1)**2 + (x1**2 + x2**2 + x3**2 + x4**2 - 0.25)**2
funcs = sp.Matrix([f])
args = sp.Matrix([x1, x2, x3, x4])
x_0 = (1, 2, 3, 4)
f_list = []
title = ["gradient_descent_barzilar_borwein", "newton_CG", "newton_quasi_L_BFGS", "trust_region_steihaug_CG"]
colorlist = ["maroon", "teal", "slateblue", "orange"]
_, _, f = oo.unconstrain.gradient_descent.barzilar_borwein(funcs, args, x_0, False, True)
f_list.append(f)
_, _, f = oo.unconstrain.newton.CG(funcs, args, x_0, False, True)
f_list.append(f)
_, _, f = oo.unconstrain.newton_quasi.L_BFGS(funcs, args, x_0, False, True)
f_list.append(f)
_, _, f = oo.unconstrain.trust_region.steihaug_CG(funcs, args, x_0, False, True)
f_list.append(f)
handle = []
for j, z in zip(colorlist, f_list):
ln, = plt.plot([i for i in range(len(z))], z, c=j, marker='o', linestyle='dashed')
handle.append(ln)
plt.xlabel("$Iteration \ times \ (k)$")
plt.ylabel("$Objective \ function \ value: \ f(x_k)$")
plt.legend(handle, title)
plt.title("Performance Comparison")
plt.show()
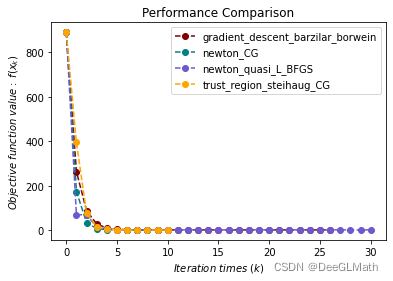
2. 非线性最小二乘问题
- from optimtool.unconstrain import nonlinear_least_square
method :用于传递线搜索方法
方法 | 函数参数 | 调用示例 |
---|
基于高斯牛顿法的非线性最小二乘问题解法 | gauss_newton(funcr, args, x_0, draw=True, output_f=False, method="wolfe", epsilon=1e-10, k=0) | nonlinear_least_square.gauss_newton(funcr, args, x_0) | 基于levenberg_marquardt的非线性最小二乘问题解法 | levenberg_marquardt(funcr, args, x_0, draw=True, output_f=False, m=100, lamk=1, eta=0.2, p1=0.4, p2=0.9, gamma1=0.7, gamma2=1.3, epsilon=1e-10, k=0) | nonlinear_least_square.levenberg_marquardt(funcr, args, x_0) |
import sympy as sp
import matplotlib.pyplot as plt
import optimtool as oo
r1, r2, x1, x2 = sp.symbols("r1 r2 x1 x2")
r1 = x1**3 - 2*x2**2 - 1
r2 = 2*x1 + x2 - 2
funcr = sp.Matrix([r1, r2])
args = sp.Matrix([x1, x2])
x_0 = (2, 2)
f_list = []
title = ["gauss_newton", "levenberg_marquardt"]
colorlist = ["maroon", "teal"]
_, _, f = oo.unconstrain.nonlinear_least_square.gauss_newton(funcr, args, x_0, False, True)
f_list.append(f)
_, _, f = oo.unconstrain.nonlinear_least_square.levenberg_marquardt(funcr, args, x_0, False, True)
f_list.append(f)
handle = []
for j, z in zip(colorlist, f_list):
ln, = plt.plot([i for i in range(len(z))], z, c=j, marker='o', linestyle='dashed')
handle.append(ln)
plt.xlabel("$Iteration \ times \ (k)$")
plt.ylabel("$Objective \ function \ value: \ f(x_k)$")
plt.legend(handle, title)
plt.title("Performance Comparison")
plt.show()
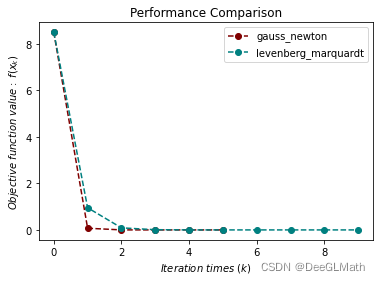
3. 等式约束优化测试
- from optimtool.constrain import equal
无约束内核默认采用wolfe线搜索方法
方法 | 函数参数 | 调用示例 |
---|
二次罚函数法 | penalty_quadratic(funcs, args, cons, x_0, draw=True, output_f=False, method="gradient_descent", sigma=10, p=2, epsilon=1e-4, k=0) | equal.penalty_quadratic(funcs, args, cons, x_0) | 增广拉格朗日法 | lagrange_augmented(funcs, args, cons, x_0, draw=True, output_f=False, method="gradient_descent", lamk=6, sigma=10, p=2, etak=1e-4, epsilon=1e-6, k=0) | equal.lagrange_augmented(funcs, args, cons, x_0) |
import numpy as np
import sympy as sp
import matplotlib.pyplot as plt
import optimtool as oo
f, x1, x2 = sp.symbols("f x1 x2")
f = x1 + np.sqrt(3) * x2
c1 = x1**2 + x2**2 - 1
funcs = sp.Matrix([f])
cons = sp.Matrix([c1])
args = sp.Matrix([x1, x2])
x_0 = (-1, -1)
f_list = []
title = ["penalty_quadratic", "lagrange_augmented"]
colorlist = ["maroon", "teal"]
_, _, f = oo.constrain.equal.penalty_quadratic(funcs, args, cons, x_0, False, True)
f_list.append(f)
_, _, f = oo.constrain.equal.lagrange_augmented(funcs, args, cons, x_0, False, True)
f_list.append(f)
handle = []
for j, z in zip(colorlist, f_list):
ln, = plt.plot([i for i in range(len(z))], z, c=j, marker='o', linestyle='dashed')
handle.append(ln)
plt.xlabel("$Iteration \ times \ (k)$")
plt.ylabel("$Objective \ function \ value: \ f(x_k)$")
plt.legend(handle, title)
plt.title("Performance Comparison")
plt.show()
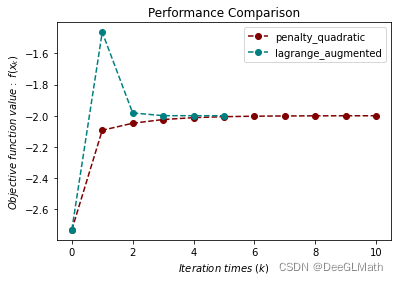
4. 不等式约束优化测试
- from optimtool.constrain import unequal
无约束内核默认采用wolfe线搜索方法
方法 | 函数参数 | 调用示例 |
---|
二次罚函数法 | penalty_quadratic(funcs, args, cons, x_0, draw=True, output_f=False, method="gradient_descent", sigma=1, p=0.4, epsilon=1e-10, k=0) | unequal.penalty_quadratic(funcs, args, cons, x_0) | 内点(分式)罚函数法 | penalty_interior_fraction(funcs, args, cons, x_0, draw=True, output_f=False, method="gradient_descent", sigma=12, p=0.6, epsilon=1e-6, k=0) | unequal.penalty_interior_fraction(funcs, args, cons, x_0) | 拉格朗日法(本质上为不存在等式约束) | lagrange_augmented(funcs, args, cons, x_0, draw=True, output_f=False, method="gradient_descent", muk=10, sigma=8, alpha=0.2, beta=0.7, p=2, eta=1e-1, epsilon=1e-4, k=0) | unequal.lagrange_augmented(funcs, args, cons, x_0) |
import sympy as sp
import matplotlib.pyplot as plt
import optimtool as oo
f, x1, x2 = sp.symbols("f x1 x2")
f = x1**2 + (x2 - 2)**2
c1 = 1 - x1
c2 = 2 - x2
funcs = sp.Matrix([f])
cons = sp.Matrix([c1, c2])
args = sp.Matrix([x1, x2])
x_0 = (2, 3)
f_list = []
title = ["penalty_quadratic", "penalty_interior_fraction"]
colorlist = ["maroon", "teal"]
_, _, f = oo.constrain.unequal.penalty_quadratic(funcs, args, cons, x_0, False, True, method="newton", sigma=10, epsilon=1e-6)
f_list.append(f)
_, _, f = oo.constrain.unequal.penalty_interior_fraction(funcs, args, cons, x_0, False, True, method="newton")
f_list.append(f)
handle = []
for j, z in zip(colorlist, f_list):
ln, = plt.plot([i for i in range(len(z))], z, c=j, marker='o', linestyle='dashed')
handle.append(ln)
plt.xlabel("$Iteration \ times \ (k)$")
plt.ylabel("$Objective \ function \ value: \ f(x_k)$")
plt.legend(handle, title)
plt.title("Performance Comparison")
plt.show()
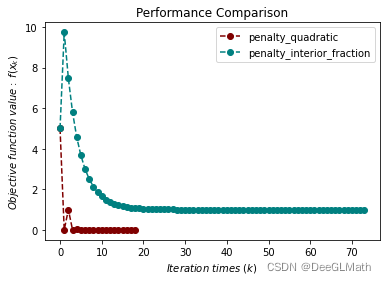
单独测试拉格朗日方法 :
import sympy as sp
import optimtool as oo
f1 = sp.symbols("f1")
x1, x2, x3, x4 = sp.symbols("x1 x2 x3 x4")
f1 = x1**2 + x2**2 + 2*x3**3 + x4**2 - 5*x1 - 5*x2 - 21*x3 + 7*x4
c1 = 8 - x1 + x2 - x3 + x4 - x1**2 - x2**2 - x3**2 - x4**2
c2 = 10 + x1 + x4 - x1**2 - 2*x2**2 - x3**2 - 2*x4**2
c3 = 5 - 2*x1 + x2 + x4 - 2*x1**2 - x2**2 - x3**2
cons_unequal1 = sp.Matrix([c1, c2, c3])
funcs1 = sp.Matrix([f1])
args1 = sp.Matrix([x1, x2, x3, x4])
x_1 = (0, 0, 0, 0)
x_0, _, f = oo.constrain.unequal.lagrange_augmented(funcs1, args1, cons_unequal1, x_1, output_f=True, method="trust_region", sigma=1, muk=1, p=1.2)
for i in range(len(x_0)):
x_0[i] = round(x_0[i], 2)
print("\n最终收敛点:", x_0, "\n目标函数值:", f[-1])
result :
最终收敛点: [ 2.5 2.5 1.87 -3.5 ]
目标函数值: -50.94151192711454
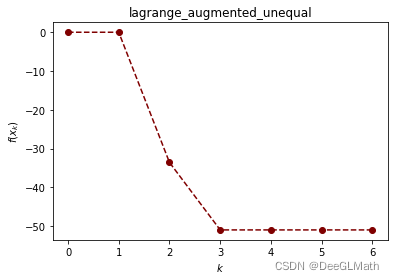
5. 混合等式约束测试
- from optimtool.constrain import mixequal
无约束内核默认采用wolfe线搜索方法
方法 | 函数参数 | 调用示例 |
---|
二次罚函数法 | penalty_quadratic(funcs, args, cons_equal, cons_unequal, x_0, draw=True, output_f=False, method="gradient_descent", sigma=1, p=0.6, epsilon=1e-10, k=0) | mixequal.penalty_quadratic(funcs, args, cons_equal, cons_unequal, x_0) | L1罚函数法 | penalty_L1(funcs, args, cons_equal, cons_unequal, x_0, draw=True, output_f=False, method="gradient_descent", sigma=1, p=0.6, epsilon=1e-10, k=0) | mixequal.penalty_L1(funcs, args, cons_equal, cons_unequal, x_0) | 增广拉格朗日函数法 | lagrange_augmented(funcs, args, cons_equal, cons_unequal, x_0, draw=True, output_f=False, method="gradient_descent", lamk=6, muk=10, sigma=8, alpha=0.5, beta=0.7, p=2, eta=1e-3, epsilon=1e-4, k=0) | mixequal.lagrange_augmented(funcs, args, cons_equal, cons_unequal, x_0) |
import sympy as sp
import matplotlib.pyplot as plt
import optimtool as oo
f, x1, x2 = sp.symbols("f x1 x2")
f = (x1 - 2)**2 + (x2 - 1)**2
c1 = x1 - 2*x2
c2 = 0.25*x1**2 - x2**2 - 1
funcs = sp.Matrix([f])
cons_equal = sp.Matrix([c1])
cons_unequal = sp.Matrix([c2])
args = sp.Matrix([x1, x2])
x_0 = (0.5, 1)
f_list = []
title = ["penalty_quadratic", "penalty_L1", "lagrange_augmented"]
colorlist = ["maroon", "teal", "orange"]
_, _, f = oo.constrain.mixequal.penalty_quadratic(funcs, args, cons_equal, cons_unequal, x_0, False, True)
f_list.append(f)
_, _, f = oo.constrain.mixequal.penalty_L1(funcs, args, cons_equal, cons_unequal, x_0, False, True)
f_list.append(f)
_, _, f = oo.constrain.mixequal.lagrange_augmented(funcs, args, cons_equal, cons_unequal, x_0, False, True)
f_list.append(f)
handle = []
for j, z in zip(colorlist, f_list):
ln, = plt.plot([i for i in range(len(z))], z, c=j, marker='o', linestyle='dashed')
handle.append(ln)
plt.xlabel("$Iteration \ times \ (k)$")
plt.ylabel("$Objective \ function \ value: \ f(x_k)$")
plt.legend(handle, title)
plt.title("Performance Comparison")
plt.show()
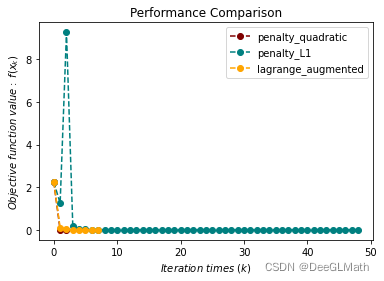
6. Lasso问题测试
- from optimtool.example import Lasso
方法 | 函数参数 | 调用示例 |
---|
梯度下降法 | gradient_descent(A, b, mu, args, x_0, draw=True, output_f=False, delta=10, alp=1e-3, epsilon=1e-2, k=0) | Lasso.gradient_descent(A, b, mu, args, x_0,) | 次梯度算法 | subgradient(A, b, mu, args, x_0, draw=True, output_f=False, alphak=2e-2, epsilon=1e-3, k=0) | Lasso.subgradient(A, b, mu, args, x_0,) |
import numpy as np
import sympy as sp
import matplotlib.pyplot as plt
import optimtool as oo
import scipy.sparse as ss
f, A, b, mu = sp.symbols("f A b mu")
x = sp.symbols('x1:9')
m = 4
n = 8
u = (ss.rand(n, 1, 0.1)).toarray()
A = np.random.randn(m, n)
b = A.dot(u)
mu = 1e-2
args = sp.Matrix(x)
x_0 = tuple([1 for i in range(8)])
f_list = []
title = ["gradient_descent", "subgradient"]
colorlist = ["maroon", "teal"]
_, _, f = oo.example.Lasso.gradient_descent(A, b, mu, args, x_0, False, True, epsilon=1e-4)
f_list.append(f)
_, _, f = oo.example.Lasso.subgradient(A, b, mu, args, x_0, False, True)
f_list.append(f)
handle = []
for j, z in zip(colorlist, f_list):
ln, = plt.plot([i for i in range(len(z))], z, c=j, marker='o', linestyle='dashed')
handle.append(ln)
plt.xlabel("$Iteration \ times \ (k)$")
plt.ylabel("$Objective \ function \ value: \ f(x_k)$")
plt.legend(handle, title)
plt.title("Performance Comparison")
plt.show()
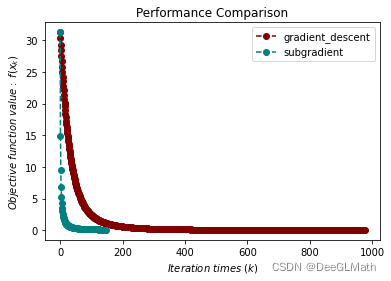
7. WanYuan问题测试
- from optimtool.example import WanYuan
方法 | 函数参数 | 调用示例 |
---|
构造7个残差函数并采用高斯牛顿法 | gauss_newton(m, n, a, b, c, x3, y3, x_0, draw=False, eps=1e-10) | WanYuan.gauss_newton(1, 2, 0.2, -1.4, 2.2, 2**(1/2), 0, (0, -1, -2.5, -0.5, 2.5, -0.05), draw=True) |
问题描述 :
给定直线方程的斜率(
m
m
m)与截距(
n
n
n),给定一元二次方程的二次项系数(
a
a
a)、一次项系数(
b
b
b)、常数(
c
c
c),给定一个过定点的圆(
x
3
x_3
x3?,
y
3
y_3
y3???),要求这个过定点的圆与直线(
x
1
x_1
x1?,
y
1
y_1
y1?)和抛物线(
x
2
x_2
x2?,
y
2
y_2
y2?)相切的切点以及该圆的圆心(
x
0
x_0
x0?,
y
0
y_0
y0?)。
code :
import sympy as sp
import matplotlib.pyplot as plt
import optimtool as oo
m = 1
n = 2
a = 0.2
b = -1.4
c = 2.2
x3 = 2*(1/2)
y3 = 0
x_0 = (0, -1, -2.5, -0.5, 2.5, -0.05)
oo.example.WanYuan.gauss_newton(1, 2, 0.2, -1.4, 2.2, 2**(1/2), 0, (0, -1, -2.5, -0.5, 2.5, -0.05), draw=True)
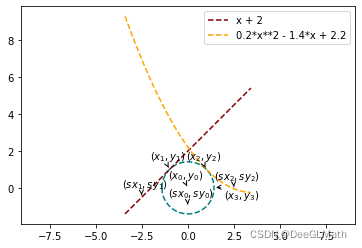
|