1、二维码检测
使用草料二维码生成需要的二维码: 网址如下:https://cli.im/
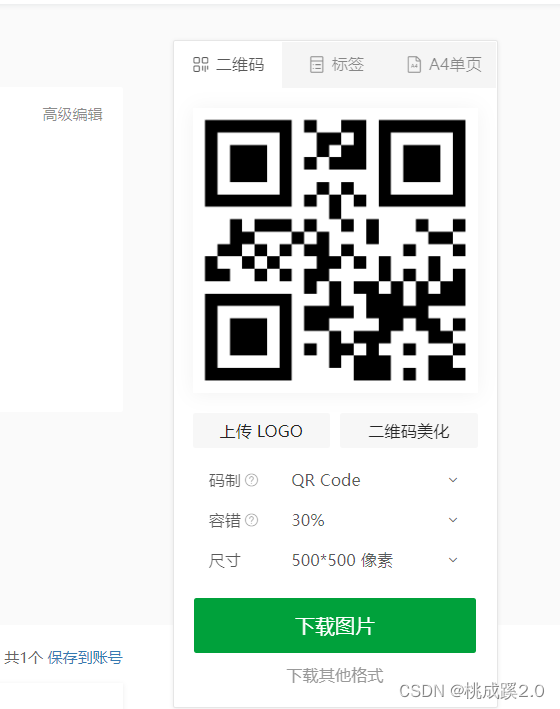
import cv2
import glob
import numpy as np
j=0
detect_obj = cv2.QRCodeDetector()
path = glob.glob('../Resources/QRcode/*.png')
for i in range(len(path)):
img = cv2.imread(path[i])
qr_info, points, qr_img = detect_obj.detectAndDecode(img)
print(path[i])
print('二维码信息:',qr_info)
if qr_info!="":
j=j+1
cv2.drawContours(img, [np.int32(points)], 0, (0, 0, 255), 2)
cv2.imwrite('../Resources/QRcode/'+str(i)+'.jpg', img)
print('检出个数',j,';总数为3个')
print('检测准确率为',j/3 *100,'%')
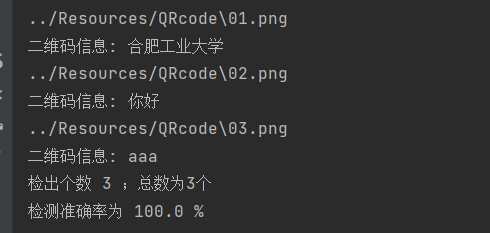
2、模版匹配
使用函数
- cv2.matchTemplate() 模版匹配函数,会匹配所有的模版
- cv2.minMaxLoc() 最大匹配,匹配最大的模版
理解:就是在一张图像里面找模版图像的位置
- 首先准备两张图,一张作为了模版使用,这里我直接选择截图,这样可能准确率高点
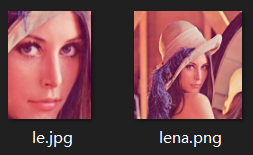
import cv2
img = cv2.imread('../Resources/lena.png', 0)
template = cv2.imread('../Resources/le.jpg', 0)
h, w = template.shape[:2]
res = cv2.matchTemplate(img, template, cv2.TM_CCOEFF)
min_val, max_val, min_loc, max_loc = cv2.minMaxLoc(res)
left_top = max_loc # 左上角
right_bottom = (left_top[0] + w, left_top[1] + h) # 右下角
cv2.rectangle(img, left_top, right_bottom, 255, 2) # 画出矩形位置
cv2.imshow('res',img)
cv2.waitKey(0)
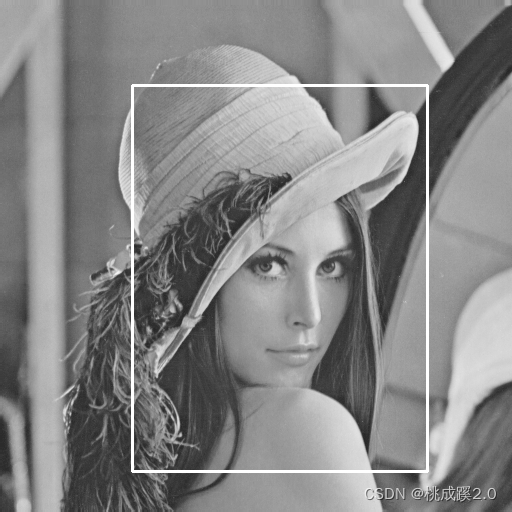
关于匹配方式:
- 平方差匹配 CV_TM_SQDIFF:用两者的平方差来匹配,最好的匹配值为0
- 归一化平方差匹配 CV_TM_SQDIFF_NORMED
- 相关匹配 CV_TM_CCORR:用两者的乘积匹配,数值越大表明匹配程度越好
- 归一化相关匹配 CV_TM_CCORR_NORMED
- 相关系数匹配 CV_TM_CCOEFF:用两者的相关系数匹配,1表示完美的匹配,-1表示最差的匹配
- 归一化相关系数匹配 CV_TM_CCOEFF_NORMED
其中上面的匹配使用的是相关系数匹配
如果要匹配多个物体:
import cv2
import numpy as np
img_origin = cv2.imread('../Resources/apple.jpg')
img = cv2.imread('../Resources/apple.jpg', 0)
template = cv2.imread('../Resources/app.jpg', 0)
h, w = template.shape[:2]
res = cv2.matchTemplate(img,template,cv2.TM_CCOEFF_NORMED)
threshold = 0.66
loc = np.where(res >= threshold) # 返回所有匹配值大于0.8的坐标
for pt in zip(*loc[::-1]): # zip就是把这些搞成一个集合一个个检索
right_bottom = (pt[0]+w,pt[1]+h)
cv2.rectangle(img_origin,pt,right_bottom,[0,0,255],0)
cv2.imshow('res',img_origin)
cv2.waitKey(0)
这里我找了两个苹果,效果属实不行,可能是模版太少角度不行之类的,都识别到第一个苹果了,另外结果和那个置信度是有很大关联的!!!
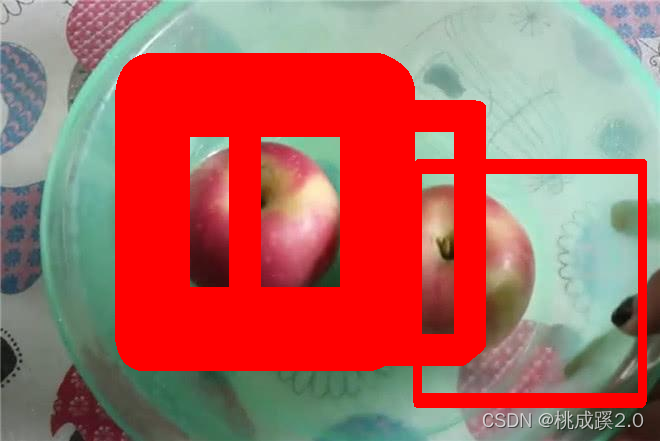
3、霍夫变换提取直线和圆
使用霍夫变换识别出图像中的直线和圆,大概原理就是:同一直线的r,θ相同,同一直线的r,θ相同,而同一曲线的r,θ相交于一点。
- cv2.HoughLines() 检测的二值图,r的精度(越大线越多) θ的精度(越小线越多) 累加数(越小线越多)
- cv2.HoughLinesP() 统计概率霍夫变换,上面的变化计算所有的点,并且不考虑起点和端点,这种进行了优化
- cv2.HoughCircles() 霍夫圆变换
标准霍夫变换
import cv2
import numpy as np
img = cv2.imread('../Resources/xian.jpg')
gray = cv2.cvtColor(img, cv2.COLOR_BGR2GRAY)
edges = cv2.Canny(gray, 50, 150)
lines = cv2.HoughLines(edges, 0.8, np.pi / 180, 90)
for line in lines: # 把所有的线都画出来
rho, theta = line[0]
a = np.cos(theta)
b = np.sin(theta)
x0 = a * rho
y0 = b * rho
x1 = int(x0 + 1000 * (-b))
y1 = int(y0 + 1000 * (a))
x2 = int(x0 - 1000 * (-b))
y2 = int(y0 - 1000 * (a))
cv2.line(img, (x1, y1), (x2, y2), (0, 0, 255),2)
cv2.imshow('res', img)
cv2.waitKey(0)
效果如下:
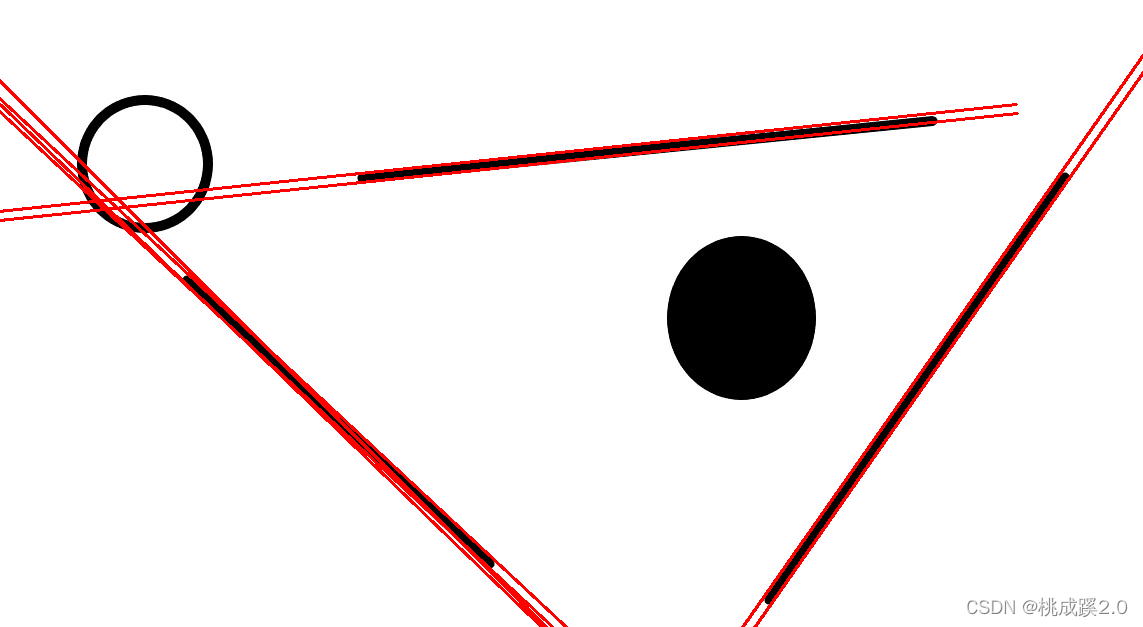
统计概率霍夫变换
import cv2
import numpy as np
img = cv2.imread('../Resources/xian.jpg')
gray = cv2.cvtColor(img, cv2.COLOR_BGR2GRAY)
edges = cv2.Canny(gray, 50, 150)
# minLineLength:最短长度阈值,比这个长度短的线会被排除 maxLineGap:同一直线两点之间的最大距离
lines = cv2.HoughLinesP(edges, 0.8, np.pi / 180, 90,minLineLength=50, maxLineGap=10)
# 将检测的线画出来
for line in lines:
x1, y1, x2, y2 = line[0]
cv2.line(img, (x1, y1), (x2, y2), (0, 0, 255), 2, lineType=cv2.LINE_AA)
cv2.imshow('res', img)
cv2.waitKey(0)
效果如下:
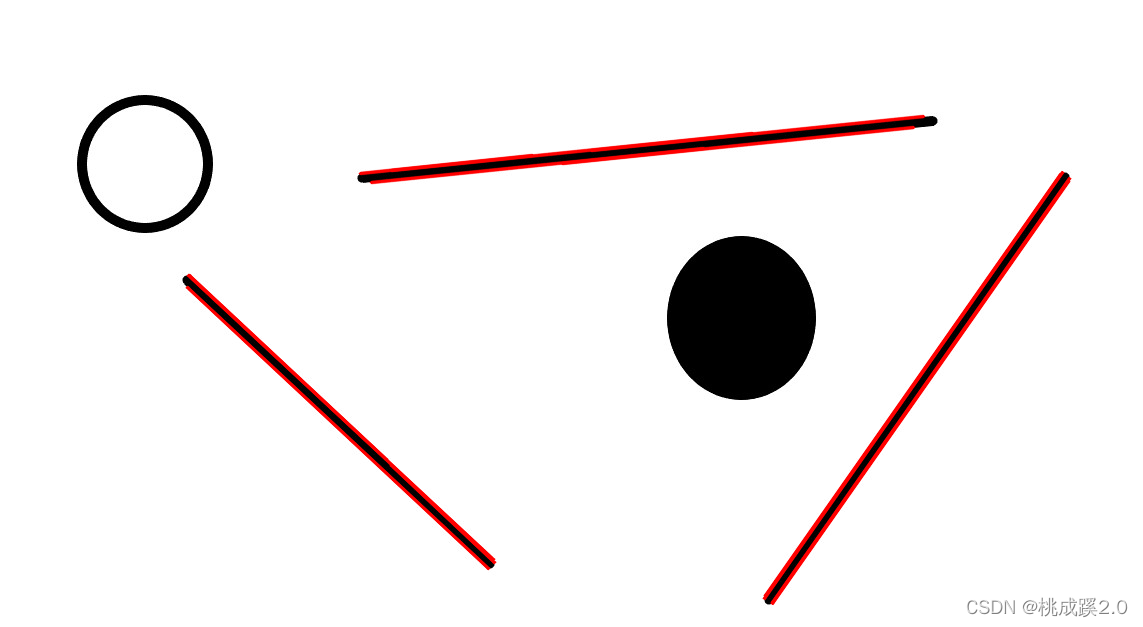
霍夫圆变换,
import cv2
import numpy as np
img = cv2.imread('../Resources/xian.jpg')
gray = cv2.cvtColor(img, cv2.COLOR_BGR2GRAY)
edges = cv2.Canny(gray, 50, 150)
# 2 变换方法,一般使用霍夫梯度法 3 取1表示和原图分辨率一致 4 圆心最短距离 5 累加器数组,和直线变换一样
circles = cv2.HoughCircles(edges, cv2.HOUGH_GRADIENT, 1, 50, param2=30)
circles = np.int0(np.around(circles))
# 将检测的圆画出来
for i in circles[0, :]:
cv2.circle(img, (i[0], i[1]), i[2], (0, 0, 255), 3) # 画出外圆
cv2.circle(img, (i[0], i[1]), 2, (0, 0, 255), 4) # 画出圆心
cv2.imshow('res', img)
cv2.waitKey(0)
效果如下:
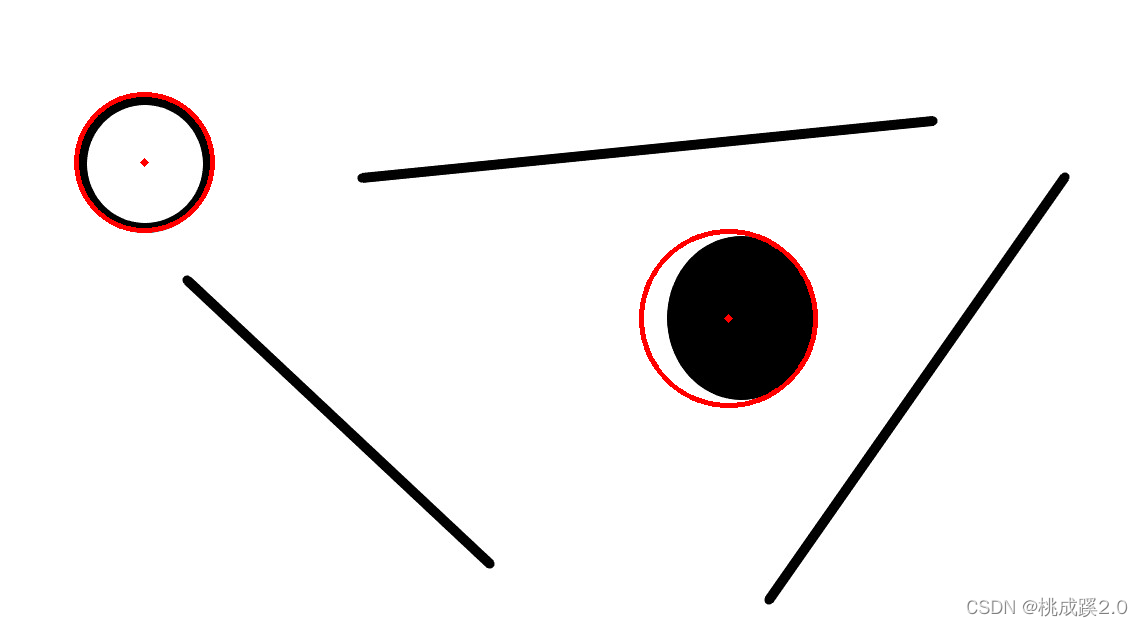
4、人脸识别
import cv2
faceCascade= cv2.CascadeClassifier("../Resources/haarcascade_frontalface_default.xml")
cap = cv2.VideoCapture(0)
while True:
success,img = cap.read()
# 使用级联分类函数进行处理
# 图像,最小的检测框 检测可信度,越大越难识别,越小越不准
faces = faceCascade.detectMultiScale(img,1.1,5)
for (x,y,w,h) in faces:
cv2.rectangle(img,(x,y),(x+w,y+h),(255,0,0),2)
cv2.imshow("Result", img)
if cv2.waitKey(1) & 0xFF == ord('q'):
break
cap.release()
cv2.destroyAllWindows()
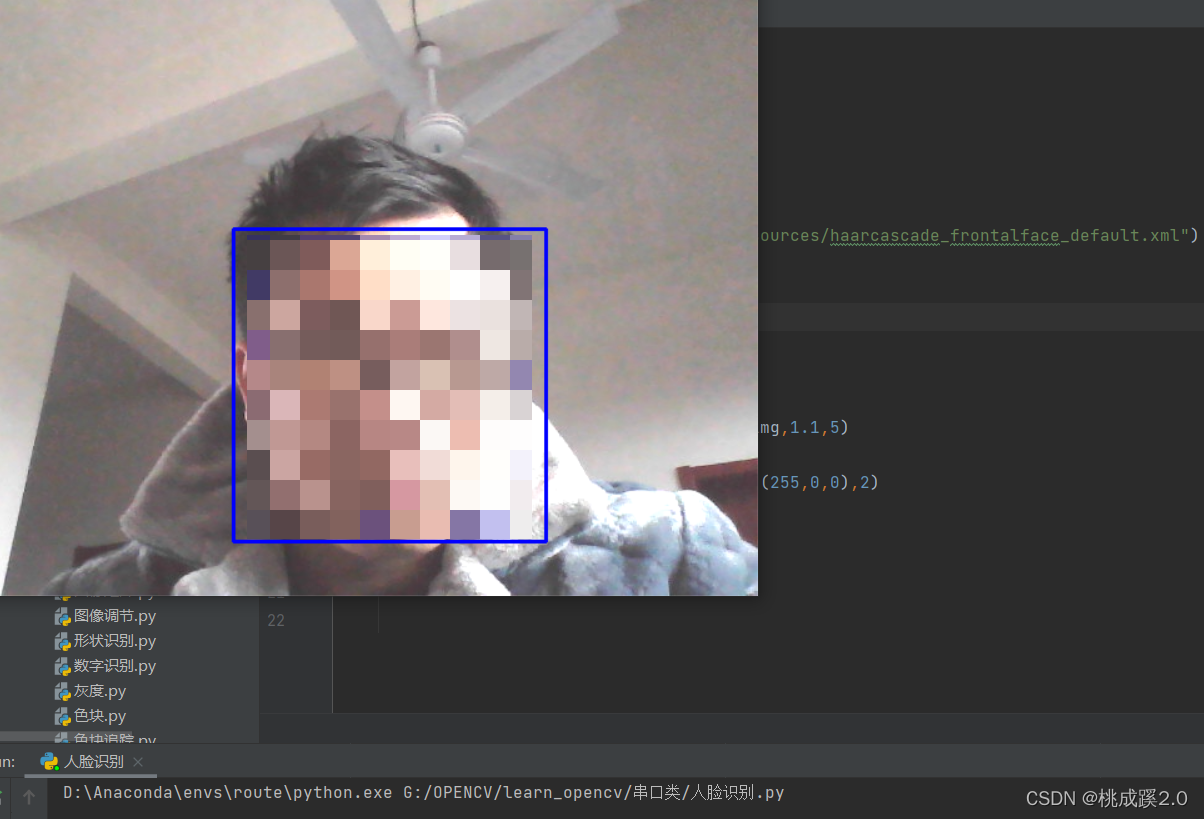
5、巡线
思路如下
- 二值化图像,在阈值范围内即可
- 寻找所有符合阈值的点
- 使用拟合函数进行拟合这里选择了一次函数
- 之后得到拟合函数,利用阈值中最左和最右的点配合拟合函数吧图像绘制出来
import cv2
import numpy as np
img = cv2.imread('../Resources/222.png') #180 390 482 125
mask=cv2.inRange(img,0,10) #二值化
kernel=np.ones((3,3),np.uint8)
erode=cv2.morphologyEx(mask,cv2.MORPH_ERODE,kernel)# 腐蚀去除噪点
def liner(img,result):
n = [len(img[:,0]),len(img[0,:])] # 高宽 480 640
(x_point,y_point)=np.nonzero(img) # 返回非0元素的值
if len(x_point)<2:
return 0
f1 = np.polyfit(x_point, y_point,1) # 使用一次函数拟合
p1 = np.poly1d(f1) # 得到拟合函数,用来后面画图用
point1 = (int(p1(x_point[0])),x_point[0])
point2 = (int(p1(x_point[len(x_point) - 1])),x_point[len(x_point) - 1])
print(point2,point1)
result = cv2.line(result, (int(n[1]/2),0) , (int(n[1]/2),n[0]), (0, 0, 255),2) # 画中心线
result=cv2.line(result,point1,point2,(0,255,0),2) # 画需要的线
cv2.imshow('inside',result)
liner(mask,img)
cv2.waitKey(0)
cv2.destroyAllWindows()
效果如下: 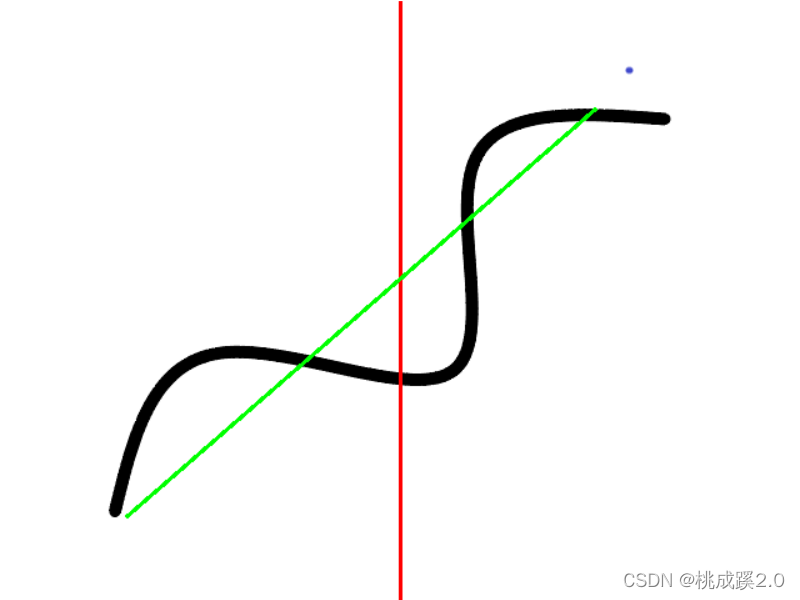
6、形状检测
先造一点形状出来
在window的画图应用里面就可以找到这个了,我其他一些比较规则的图像也是这么出来的
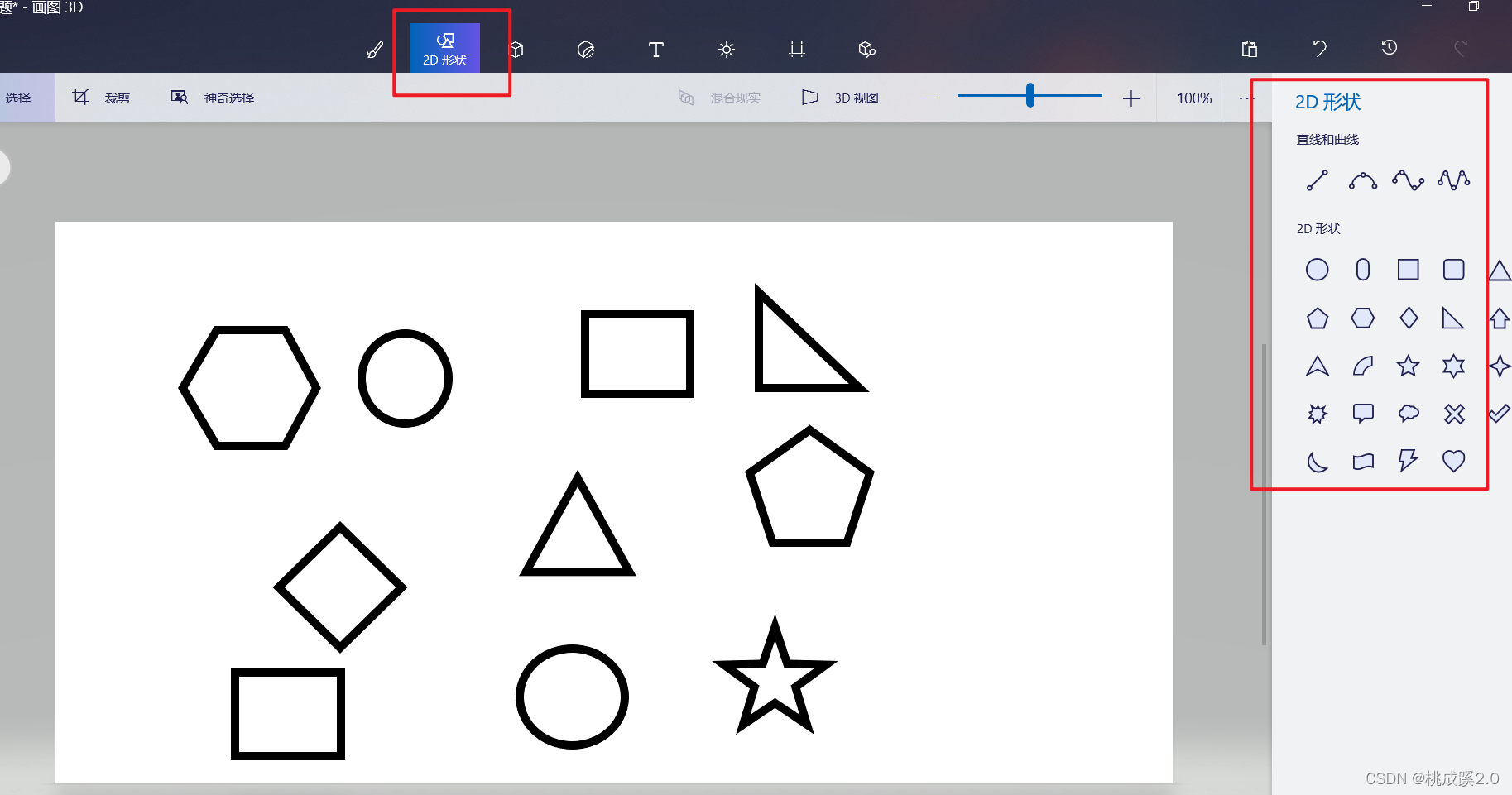
提取轮廓
mport cv2
import numpy as np
img = cv2.imread('../Resources/xing.jpg')
imgContour = img.copy()# 备份一站用来标记的。不破坏元图像
imgGray = cv2.cvtColor(img,cv2.COLOR_BGR2GRAY) #灰度
imgBlur = cv2.GaussianBlur(imgGray,(7,7),1) #阈值
imgCanny = cv2.Canny(imgBlur,50,50) # 边缘
# 轮廓数,轮廓索引
contours,hierarchy = cv2.findContours(imgCanny,cv2.RETR_EXTERNAL,cv2.CHAIN_APPROX_NONE) #提取轮廓,选择保存所有轮廓点
# 下面遍历对每一个轮廓进行处理
for cnt in contours:
cv2.drawContours(imgContour,cnt,-1,(255,0,0),2)
cv2.imshow('res',imgContour)
cv2.waitKey(0)
cv2.destroyAllWindow()
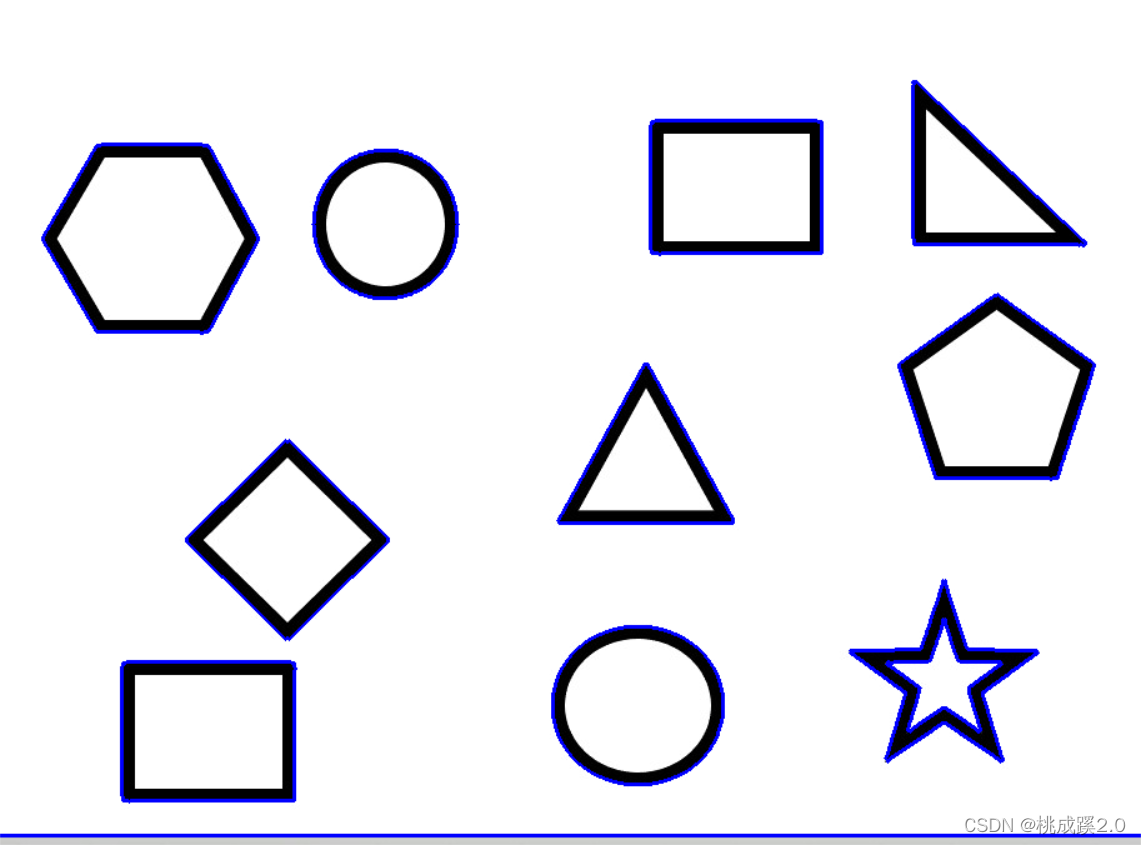
下面开始处理轮廓,主要是根据轮廓折线的数量,因此我们就很有必要处理下这个折线
import cv2
import numpy as np
img = cv2.imread('../Resources/xing.jpg')
imgContour = img.copy()# 备份一站用来标记的。不破坏元图像
imgGray = cv2.cvtColor(img,cv2.COLOR_BGR2GRAY) #灰度
imgBlur = cv2.GaussianBlur(imgGray,(7,7),1) #阈值
imgCanny = cv2.Canny(imgBlur,50,50) # 边缘
# 轮廓数,轮廓索引
contours,hierarchy = cv2.findContours(imgCanny,cv2.RETR_EXTERNAL,cv2.CHAIN_APPROX_NONE) #提取轮廓,选择保存所有轮廓点
# 下面遍历对每一个轮廓进行处理
for cnt in contours:
cv2.drawContours(imgContour,cnt,-1,(0,255,0),3)
# 开始处理轮廓
per = cv2.arcLength(cnt,True) #计算轮廓长度
# 1是源图像的某个轮廓,2(epsilon)是一个距离值,表示多边形的轮廓接近实际轮廓的程度,值越小,越精确;参数3表示是否闭合。
num_per = cv2.approxPolyDP(cnt,0.01*per,True) #近似函数
# 做一个外接圆
x,y,w,h = cv2.boundingRect(num_per)
cv2.rectangle(imgContour,(x,y),(x+w,y+h),(0,0,255),2)
obj = len(num_per) #边长
if obj == 3:
text = 'sanjiao'
elif obj == 4:
text = 'rec'
elif obj == 5:
text = 'wubian'
elif obj == 6:
text = 'liubian'
else:
text = 'roll'
cv2.putText(imgContour,text,(x+(w
cv2.imshow('res',imgContour)
cv2.waitKey(0)
cv2.destroyAllWindow()
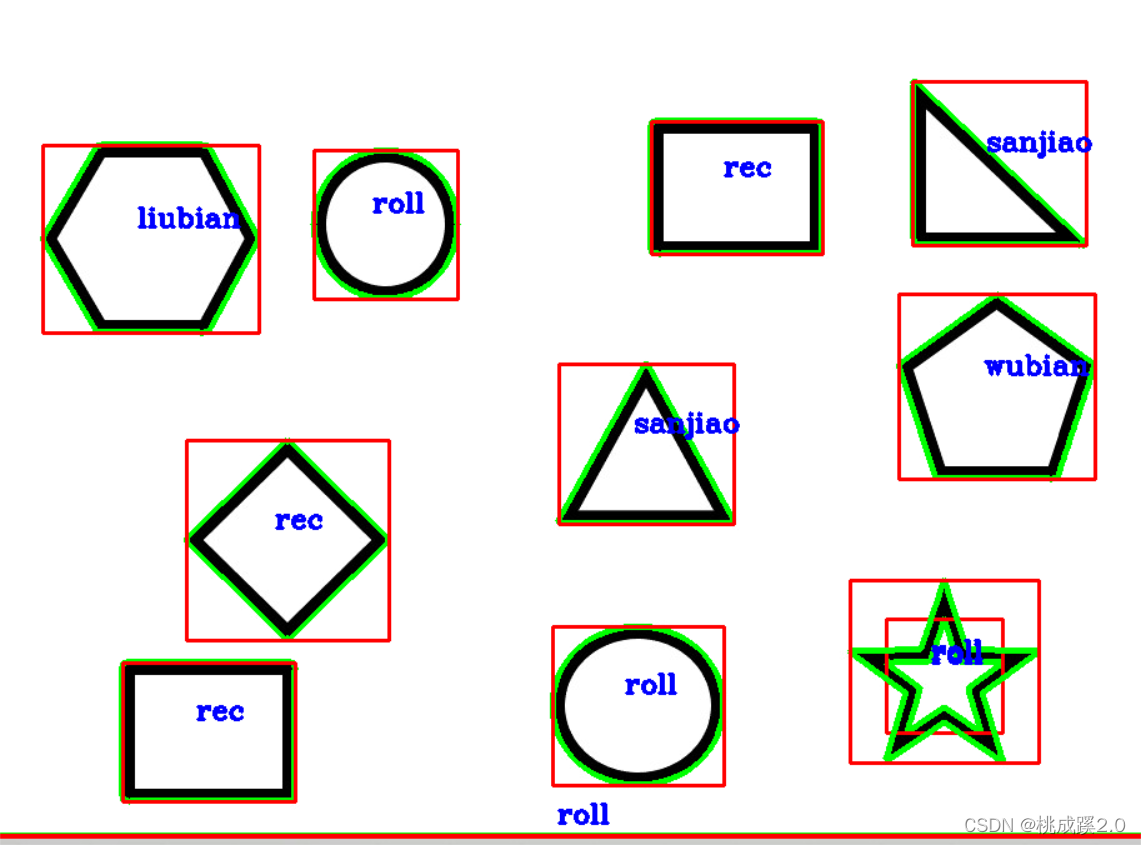
7、手势识别
采用Mediapipe
MediaPipe是一款由Google开发并开源的数据流处理机器学习应用开发框架。它是一个基于图的数据处理管线,用于构建使用了多种形式的数据源,如视频、音频、传感器数据以及任何时间序列数据。 MediaPipe是跨平台的,可以运行在嵌入式平台(树莓派等),移动设备(iOS和Android),工作站和服务器上,并支持移动端GPU加速。 使用MediaPipe,可以将机器学习任务构建为一个图形的模块表示的数据流管道,可以包括推理模型和流媒体处理功能。
好说完了废话介绍,下面说下用途:
安装一下这个库:
pip install mediapipe
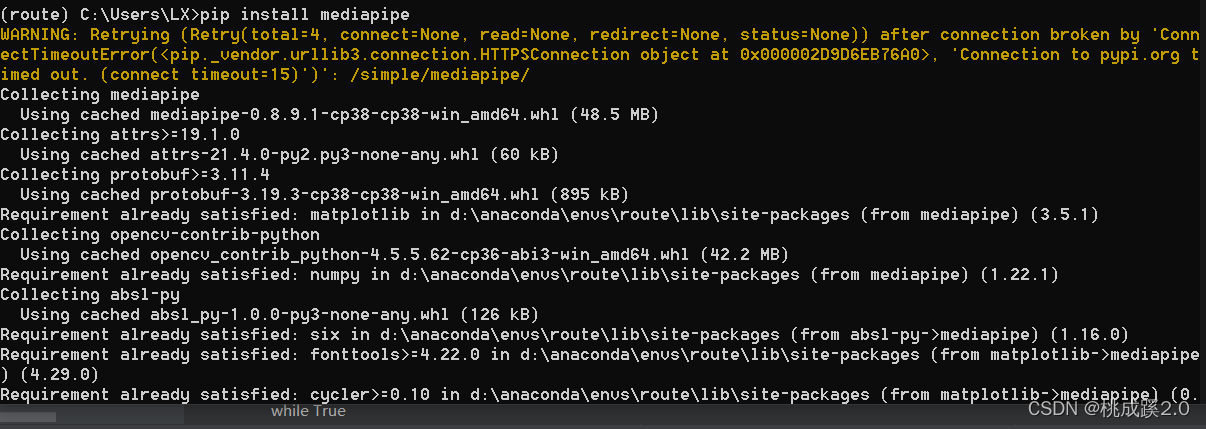
21个手关节坐标如下
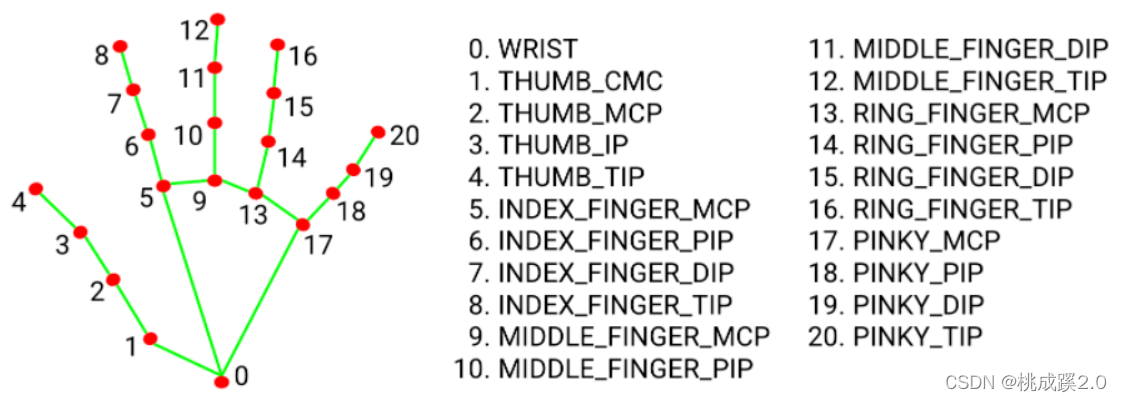
import cv2
import mediapipe as mp
cap = cv2.VideoCapture(0)
mpHands = mp.solutions.hands
hands = mpHands.Hands()
mpDraw = mp.solutions.drawing_utils
while True:
success, img = cap.read()
# imgRGB = cv2.cvtColor(img, cv2.COLOR_BGR2RGB)
results = hands.process(img) #直接调用hands
if results.multi_hand_landmarks: #检测手关键的模型
for hand in results.multi_hand_landmarks:
for id, lm in enumerate(hand.landmark):
h, w, c = img.shape
cx, cy = int(lm.x * w), int(lm.y * h)
print(id, cx, cy)
cv2.circle(img, (cx, cy), 5, (0, 255, 0), cv2.FILLED)
mpDraw.draw_landmarks(img, hand, mpHands.HAND_CONNECTIONS) # 连接关键点函数
cv2.imshow("res", img)
cv2.waitKey(1)
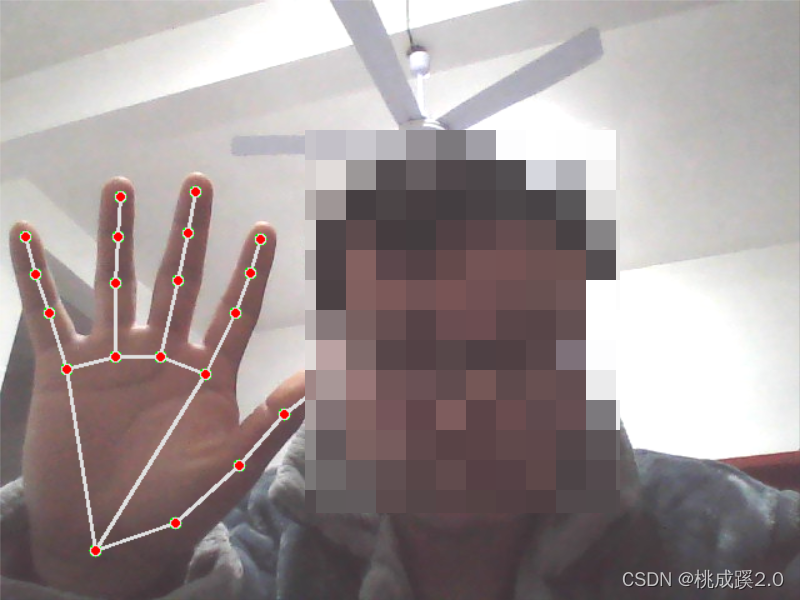
|