卷积神经网络(LeNet)
1. 网络结构
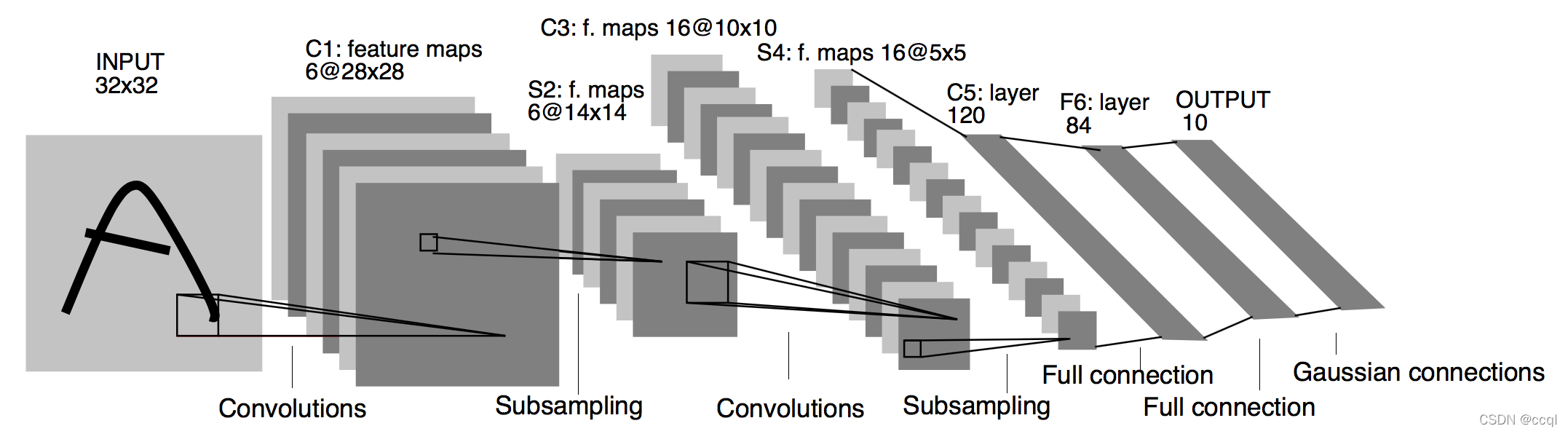
网络结构计算过程:
假设输入形状是
n
h
×
n
w
n_h\times n_w
nh?×nw?,卷积核窗口形状是
k
h
×
h
w
k_h\times h_w
kh?×hw?,在高的两侧一共填充
p
h
p_h
ph?行,在宽的两侧一共填充
p
w
p_w
pw?列,高上步幅为
s
h
s_h
sh?,宽上步幅为
s
w
s_w
sw?,则卷积输出形状计算公式:
?
(
n
h
?
k
h
+
p
h
+
s
h
)
/
s
h
?
×
?
(
n
w
?
k
w
+
p
w
+
s
w
)
/
s
w
?
\lfloor(n_h-k_h+p_h+s_h)/s_h\rfloor\times\lfloor(n_w-k_w+p_w+s_w)/s_w\rfloor
?(nh??kh?+ph?+sh?)/sh??×?(nw??kw?+pw?+sw?)/sw??
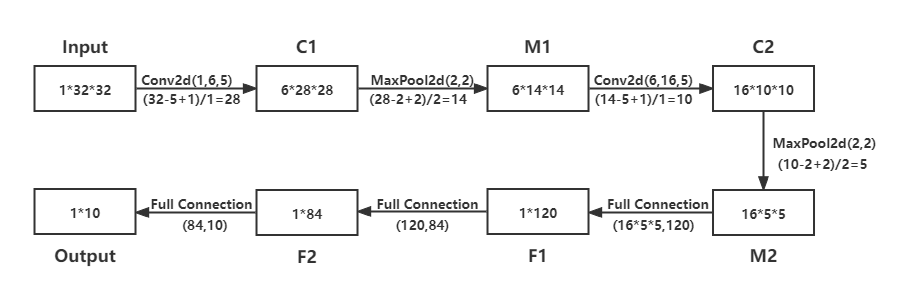
2. 网络结构说明
LeNet分为卷积层块和全连接层块两个部分。
卷积层块里的基本单位是卷积层后接最大池化层:卷积层用来识别图像里的空间模式,如线条和物体局部,之后的最大池化层则用来降低卷积层对位置的敏感性。卷积层块由两个这样的基本单位重复堆叠构成。在卷积层块中,每个卷积层都使用
5
×
5
5\times 5
5×5的窗口,并在输出上使用sigmoid激活函数。第一个卷积层输出通道数为6,第二个卷积层输出通道数则增加到16。这是因为第二个卷积层比第一个卷积层的输入的高和宽要小,所以增加输出通道使两个卷积层的参数尺寸类似。卷积层块的两个最大池化层的窗口形状均为
2
×
2
2\times 2
2×2,且步幅为2。由于池化窗口与步幅形状相同,池化窗口在输入上每次滑动所覆盖的区域互不重叠。
3. 代码实现
3.1 模型建立
import time
import torch
import torchvision
import torchvision.transforms as transforms
from torch import nn, optim
device = torch.device('cuda' if torch.cuda.is_available() else 'cpu')
class LeNet(nn.Module):
def __init__(self):
super(LeNet, self).__init__()
self.conv = nn.Sequential(
nn.Conv2d(1, 6, 5),
nn.Sigmoid(),
nn.MaxPool2d(2, 2),
nn.Conv2d(6, 16, 5),
nn.Sigmoid(),
nn.MaxPool2d(2, 2)
)
self.fc = nn.Sequential(
nn.Linear(16*4*4, 120),
nn.Sigmoid(),
nn.Linear(120, 84),
nn.Sigmoid(),
nn.Linear(84, 10)
)
def forward(self, img):
feature = self.conv(img)
output = self.fc(feature.view(img.shape[0], -1))
return output
net = LeNet()
print(net)
3.2 数据集加载
batch_size = 256
mnist_train = torchvision.datasets.FashionMNIST(root='~/Datasets/FashionMNIST', train=True, download=True, transform=transforms.ToTensor())
mnist_test = torchvision.datasets.FashionMNIST(root='~/Datasets/FashionMNIST', train=False, download=True, transform=transforms.ToTensor())
train_iter = torch.utils.data.DataLoader(mnist_train, batch_size=batch_size, shuffle=True)
test_iter = torch.utils.data.DataLoader(mnist_test, batch_size=batch_size, shuffle=False)
3.3 定义评估函数
def evaluate_accuracy(data_iter, net, device=None):
if device is None and isinstance(net, torch.nn.Module):
device = list(net.parameters())[0].device
acc_sum, n = 0.0, 0
with torch.no_grad():
for X, y in data_iter:
if isinstance(net, torch.nn.Module):
net.eval()
acc_sum += (net(X.to(device)).argmax(dim=1) == y.to(device)).float().sum().cpu().item()
net.train()
else:
if('is_training' in net.__code__.co_varnames):
acc_sum += (net(X, is_training=False).argmax(dim=1) == y).float().sum().item()
else:
acc_sum += (net(X).argmax(dim=1) == y).float().sum().item()
n += y.shape[0]
return acc_sum / n
3.4 模型训练
def train_ch5(net, train_iter, test_iter, batch_size, optimizer, device, num_epochs):
net = net.to(device)
print("training on ", device)
loss = torch.nn.CrossEntropyLoss()
for epoch in range(num_epochs):
train_l_sum, train_acc_sum, n, batch_count, start = 0.0, 0.0, 0, 0, time.time()
for X, y in train_iter:
X = X.to(device)
y = y.to(device)
y_hat = net(X)
l = loss(y_hat, y)
optimizer.zero_grad()
l.backward()
optimizer.step()
train_l_sum += l.cpu().item()
train_acc_sum += (y_hat.argmax(dim=1) == y).sum().cpu().item()
n += y.shape[0]
batch_count += 1
test_acc = evaluate_accuracy(test_iter, net)
print('epoch %d, loss %.4f, train acc %.3f, test acc %.3f, time %.1f sec'
% (epoch + 1, train_l_sum / batch_count, train_acc_sum / n, test_acc, time.time() - start))
lr, num_epochs = 0.001, 5
optimizer = torch.optim.Adam(net.parameters(), lr=lr)
train_ch5(net, train_iter, test_iter, batch_size, optimizer, device, num_epochs)
4. 问题发现及解决
Q:lenet模型传播函数的output = self.fc(feature.view(img.shape[0], -1))这句代码的理解?
A:卷积层计块算出来后将数据扁平化再输入全连接层块进行运算,img的形状为[256, 1, 28, 28],feature的尺寸为[256, 1, 16, 4, 4],经过这句代码运算后feature.view(img.shape[0], -1)张量的尺寸为[256, 256]。也就是说,全连接层的输入形状将变成二维,其中第一维是小批量中的样本,第二维是每个样本变平后的向量表示,且向量长度为通道、高和宽的乘积。
|