本小节通过实例讲述CNN识别垃圾邮件的方法。
1、数据集
将load_one_file函数中open的参数增加encoding='utf-8',如下所示:
def load_one_file(filename):
x=""
with open(filename, encoding='utf-8') as f:
print(filename)
for line in f:
line=line.strip('\n')
line = line.strip('\r')
x+=line
return x
def load_files_from_dir(rootdir):
x=[]
list = os.listdir(rootdir)
for i in range(0, len(list)):
path = os.path.join(rootdir, list[i])
if os.path.isfile(path):
v=load_one_file(path)
x.append(v)
return x
def load_all_files():
ham=[]
spam=[]
for i in range(1,5):
path="../data/enron%d/ham/" % i
print("Load %s" % path)
ham+=load_files_from_dir(path)
path="../data/enron%d/spam/" % i
print("Load %s" % path)
spam+=load_files_from_dir(path)
return ham,spam
会报错?
../data/enron1/spam/0754.2004-04-01.GP.spam.txt
for line in f:
File "C:\ProgramData\Anaconda3\lib\codecs.py", line 322, in decode
(result, consumed) = self._buffer_decode(data, self.errors, final)
UnicodeDecodeError: 'utf-8' codec can't decode byte 0xb7 in position 1400: invalid start byte
Process finished with exit code 1
?可以通过将文件改为utf-8编码解决

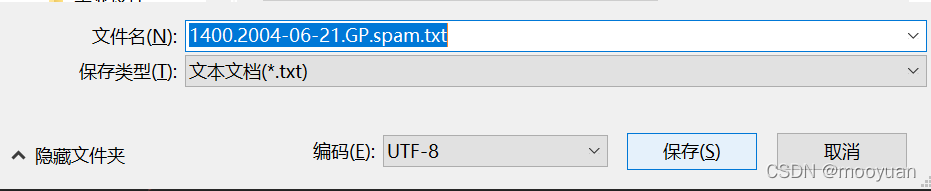
?另外,在实验过程中,发现作者提供的《web安全之机器学习入门》并没有这个data/mail//data/enron%d相关数据集,我这里只是选取了data目录下enron1的数据,并以此做实验。
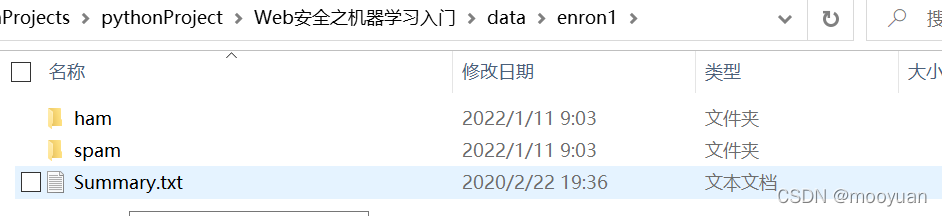
不过我发现在《web安全之深度学习实战》中data目录下有mail这个数据集,可以用这个来做实验。
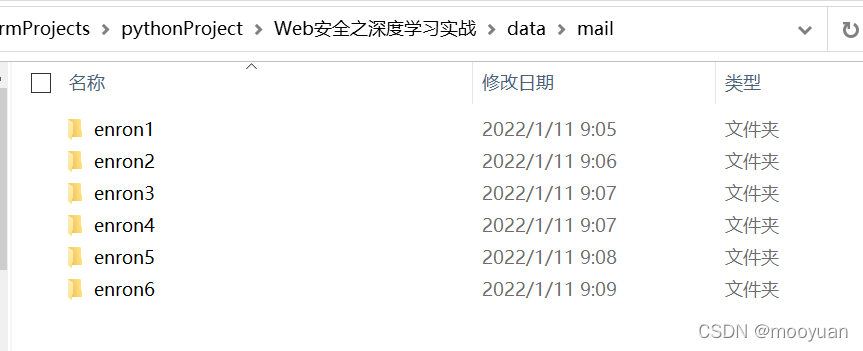
3、词袋特征化
def get_features_by_wordbag():
ham, spam=load_all_files()
x=ham+spam
y=[0]*len(ham)+[1]*len(spam)
vectorizer = CountVectorizer(
decode_error='ignore',
strip_accents='ascii',
max_features=max_features,
stop_words='english',
max_df=1.0,
min_df=1 )
print(vectorizer)
x=vectorizer.fit_transform(x)
x=x.toarray()
return x,y
x,y=get_features_by_wordbag()
x_train, x_test, y_train, y_test = train_test_split(x, y, test_size = 0.4, random_state = 0)
4、TF特征化
def get_features_by_tf():
global max_document_length
x=[]
y=[]
ham, spam=load_all_files()
x=ham+spam
y=[0]*len(ham)+[1]*len(spam)
vp=tflearn.data_utils.VocabularyProcessor(max_document_length=max_document_length,
min_frequency=0,
vocabulary=None,
tokenizer_fn=None)
x=vp.fit_transform(x, unused_y=None)
x=np.array(list(x))
return x,y
print("get_features_by_tf")
x,y=get_features_by_tf()
x_train, x_test, y_train, y_test = train_test_split(x, y, test_size = 0.4, random_state = 0)
5、TF-IDF特征化
def get_features_by_wordbag_tfidf():
ham, spam=load_all_files()
x=ham+spam
y=[0]*len(ham)+[1]*len(spam)
vectorizer = CountVectorizer(binary=True,
decode_error='ignore',
strip_accents='ascii',
max_features=max_features,
stop_words='english',
max_df=1.0,
min_df=1 )
print(vectorizer)
x=vectorizer.fit_transform(x)
x=x.toarray()
transformer = TfidfTransformer(smooth_idf=False)
print(transformer)
tfidf = transformer.fit_transform(x)
x = tfidf.toarray()
return x,y
print("get_features_by_tfidf")
x,y=get_features_by_wordbag_tfidf()
x_train, x_test, y_train, y_test = train_test_split(x, y, test_size = 0.4, random_state = 0)
6、构建朴素贝叶斯NB模型
def do_nb_wordbag(x_train, x_test, y_train, y_test):
print("NB and wordbag")
gnb = GaussianNB()
gnb.fit(x_train,y_train)
y_pred=gnb.predict(x_test)
print(metrics.accuracy_score(y_test, y_pred))
print(metrics.confusion_matrix(y_test, y_pred))
7.构建SVM模型
def do_svm_wordbag(x_train, x_test, y_train, y_test):
print("SVM and wordbag")
clf = svm.SVC()
clf.fit(x_train, y_train)
y_pred = clf.predict(x_test)
print(metrics.accuracy_score(y_test, y_pred))
print(metrics.confusion_matrix(y_test, y_pred))
8、构建DNN
def do_dnn_wordbag(x_train, x_test, y_train, y_testY):
print("DNN and wordbag")
# Building deep neural network
clf = MLPClassifier(solver='lbfgs',
alpha=1e-5,
hidden_layer_sizes = (5, 2),
random_state = 1)
print(clf)
clf.fit(x_train, y_train)
y_pred = clf.predict(x_test)
print(metrics.accuracy_score(y_test, y_pred))
print(metrics.confusion_matrix(y_test, y_pred))
9、构建RNN
def do_rnn_wordbag(trainX, testX, trainY, testY):
global max_document_length
print("RNN and wordbag")
trainX = pad_sequences(trainX, maxlen=max_document_length, value=0.)
testX = pad_sequences(testX, maxlen=max_document_length, value=0.)
# Converting labels to binary vectors
trainY = to_categorical(trainY, nb_classes=2)
testY = to_categorical(testY, nb_classes=2)
# Network building
net = tflearn.input_data([None, max_document_length])
net = tflearn.embedding(net, input_dim=10240000, output_dim=128)
net = tflearn.lstm(net, 128, dropout=0.8)
net = tflearn.fully_connected(net, 2, activation='softmax')
net = tflearn.regression(net, optimizer='adam', learning_rate=0.001,
loss='categorical_crossentropy')
# Training
model = tflearn.DNN(net, tensorboard_verbose=0)
model.fit(trainX, trainY, validation_set=(testX, testY), show_metric=True,
batch_size=10,run_id="spm-run",n_epoch=5)
10、构建CNN?
def do_cnn_wordbag(trainX, testX, trainY, testY):
global max_document_length
print("CNN and tf")
trainX = pad_sequences(trainX, maxlen=max_document_length, value=0.)
testX = pad_sequences(testX, maxlen=max_document_length, value=0.)
# Converting labels to binary vectors
trainY = to_categorical(trainY, nb_classes=2)
testY = to_categorical(testY, nb_classes=2)
# Building convolutional network
network = input_data(shape=[None,max_document_length], name='input')
network = tflearn.embedding(network, input_dim=1000000, output_dim=128)
branch1 = conv_1d(network, 128, 3, padding='valid', activation='relu', regularizer="L2")
branch2 = conv_1d(network, 128, 4, padding='valid', activation='relu', regularizer="L2")
branch3 = conv_1d(network, 128, 5, padding='valid', activation='relu', regularizer="L2")
network = merge([branch1, branch2, branch3], mode='concat', axis=1)
network = tf.expand_dims(network, 2)
network = global_max_pool(network)
network = dropout(network, 0.8)
network = fully_connected(network, 2, activation='softmax')
network = regression(network, optimizer='adam', learning_rate=0.001,
loss='categorical_crossentropy', name='target')
# Training
model = tflearn.DNN(network, tensorboard_verbose=0)
model.fit(trainX, trainY,
n_epoch=5, shuffle=True, validation_set=(testX, testY),
show_metric=True, batch_size=100,run_id="spam")
11、完整代码
基于python3运行环境的完整代码
from sklearn.feature_extraction.text import CountVectorizer
import os
from sklearn.naive_bayes import GaussianNB
from sklearn.model_selection import train_test_split
from sklearn import metrics
import matplotlib.pyplot as plt
import numpy as np
from sklearn import svm
from sklearn.feature_extraction.text import TfidfTransformer
import tensorflow as tf
import tflearn
from tflearn.layers.core import input_data, dropout, fully_connected
from tflearn.layers.conv import conv_1d, global_max_pool
from tflearn.layers.conv import conv_2d, max_pool_2d
from tflearn.layers.merge_ops import merge
from tflearn.layers.estimator import regression
from tflearn.data_utils import to_categorical, pad_sequences
from sklearn.neural_network import MLPClassifier
from tflearn.layers.normalization import local_response_normalization
from tensorflow.contrib import learn
max_features=500
max_document_length=1024
def load_one_file(filename):
x=""
with open(filename, encoding='utf-8') as f:
#print(filename)
for line in f:
line=line.strip('\n')
line = line.strip('\r')
x+=line
return x
def load_files_from_dir(rootdir):
x=[]
list = os.listdir(rootdir)
for i in range(0, len(list)):
path = os.path.join(rootdir, list[i])
if os.path.isfile(path):
v=load_one_file(path)
x.append(v)
return x
def load_all_files():
ham=[]
spam=[]
for i in range(1,2):
path="../data/enron%d/ham/" % i
print("Load %s" % path)
ham+=load_files_from_dir(path)
path="../data/enron%d/spam/" % i
print("Load %s" % path)
spam+=load_files_from_dir(path)
return ham,spam
def get_features_by_wordbag():
ham, spam=load_all_files()
x=ham+spam
y=[0]*len(ham)+[1]*len(spam)
vectorizer = CountVectorizer(
decode_error='ignore',
strip_accents='ascii',
max_features=max_features,
stop_words='english',
max_df=1.0,
min_df=1 )
print(vectorizer)
x=vectorizer.fit_transform(x)
x=x.toarray()
return x,y
def show_diffrent_max_features():
global max_features
a=[]
b=[]
for i in range(1000,20000,2000):
max_features=i
print("max_features=%d" % i)
x, y = get_features_by_wordbag()
x_train, x_test, y_train, y_test = train_test_split(x, y, test_size=0.4, random_state=0)
gnb = GaussianNB()
gnb.fit(x_train, y_train)
y_pred = gnb.predict(x_test)
score=metrics.accuracy_score(y_test, y_pred)
a.append(max_features)
b.append(score)
plt.plot(a, b, 'r')
plt.xlabel("max_features")
plt.ylabel("metrics.accuracy_score")
plt.title("metrics.accuracy_score VS max_features")
plt.legend()
plt.show()
def do_nb_wordbag(x_train, x_test, y_train, y_test):
print("NB and wordbag")
gnb = GaussianNB()
gnb.fit(x_train,y_train)
y_pred=gnb.predict(x_test)
print(metrics.accuracy_score(y_test, y_pred))
print(metrics.confusion_matrix(y_test, y_pred))
def do_svm_wordbag(x_train, x_test, y_train, y_test):
print("SVM and wordbag")
clf = svm.SVC()
clf.fit(x_train, y_train)
y_pred = clf.predict(x_test)
print(metrics.accuracy_score(y_test, y_pred))
print(metrics.confusion_matrix(y_test, y_pred))
def get_features_by_wordbag_tfidf():
ham, spam=load_all_files()
x=ham+spam
y=[0]*len(ham)+[1]*len(spam)
vectorizer = CountVectorizer(binary=True,
decode_error='ignore',
strip_accents='ascii',
max_features=max_features,
stop_words='english',
max_df=1.0,
min_df=1 )
print(vectorizer)
x=vectorizer.fit_transform(x)
x=x.toarray()
transformer = TfidfTransformer(smooth_idf=False)
print(transformer)
tfidf = transformer.fit_transform(x)
x = tfidf.toarray()
return x,y
def do_cnn_wordbag(trainX, testX, trainY, testY):
global max_document_length
print("CNN and tf")
trainX = pad_sequences(trainX, maxlen=max_document_length, value=0.)
testX = pad_sequences(testX, maxlen=max_document_length, value=0.)
# Converting labels to binary vectors
trainY = to_categorical(trainY, nb_classes=2)
testY = to_categorical(testY, nb_classes=2)
# Building convolutional network
network = input_data(shape=[None,max_document_length], name='input')
network = tflearn.embedding(network, input_dim=1000000, output_dim=128)
branch1 = conv_1d(network, 128, 3, padding='valid', activation='relu', regularizer="L2")
branch2 = conv_1d(network, 128, 4, padding='valid', activation='relu', regularizer="L2")
branch3 = conv_1d(network, 128, 5, padding='valid', activation='relu', regularizer="L2")
network = merge([branch1, branch2, branch3], mode='concat', axis=1)
network = tf.expand_dims(network, 2)
network = global_max_pool(network)
network = dropout(network, 0.8)
network = fully_connected(network, 2, activation='softmax')
network = regression(network, optimizer='adam', learning_rate=0.001,
loss='categorical_crossentropy', name='target')
# Training
model = tflearn.DNN(network, tensorboard_verbose=0)
model.fit(trainX, trainY,
n_epoch=5, shuffle=True, validation_set=(testX, testY),
show_metric=True, batch_size=100,run_id="spam")
def do_rnn_wordbag(trainX, testX, trainY, testY):
global max_document_length
print("RNN and wordbag")
trainX = pad_sequences(trainX, maxlen=max_document_length, value=0.)
testX = pad_sequences(testX, maxlen=max_document_length, value=0.)
# Converting labels to binary vectors
trainY = to_categorical(trainY, nb_classes=2)
testY = to_categorical(testY, nb_classes=2)
# Network building
net = tflearn.input_data([None, max_document_length])
net = tflearn.embedding(net, input_dim=10240000, output_dim=128)
net = tflearn.lstm(net, 128, dropout=0.8)
net = tflearn.fully_connected(net, 2, activation='softmax')
net = tflearn.regression(net, optimizer='adam', learning_rate=0.001,
loss='categorical_crossentropy')
# Training
model = tflearn.DNN(net, tensorboard_verbose=0)
model.fit(trainX, trainY, validation_set=(testX, testY), show_metric=True,
batch_size=10,run_id="spm-run",n_epoch=5)
def do_dnn_wordbag(x_train, x_test, y_train, y_testY):
print("DNN and wordbag")
# Building deep neural network
clf = MLPClassifier(solver='lbfgs',
alpha=1e-5,
hidden_layer_sizes = (5, 2),
random_state = 1)
print(clf)
clf.fit(x_train, y_train)
y_pred = clf.predict(x_test)
print(metrics.accuracy_score(y_test, y_pred))
print(metrics.confusion_matrix(y_test, y_pred))
def get_features_by_tf():
global max_document_length
x=[]
y=[]
ham, spam=load_all_files()
x=ham+spam
y=[0]*len(ham)+[1]*len(spam)
vp=tflearn.data_utils.VocabularyProcessor(max_document_length=max_document_length,
min_frequency=0,
vocabulary=None,
tokenizer_fn=None)
x=vp.fit_transform(x, unused_y=None)
x=np.array(list(x))
return x,y
if __name__ == "__main__":
print("Hello spam-mail")
#print "get_features_by_wordbag"
#x,y=get_features_by_wordbag()
#x_train, x_test, y_train, y_test = train_test_split(x, y, test_size = 0.4, random_state = 0)
#print "get_features_by_wordbag_tfidf"
#x,y=get_features_by_wordbag_tfidf()
#x_train, x_test, y_train, y_test = train_test_split(x, y, test_size = 0.4, random_state = 0)
#NB
#do_nb_wordbag(x_train, x_test, y_train, y_test)
#show_diffrent_max_features()
#SVM
#do_svm_wordbag(x_train, x_test, y_train, y_test)
#DNN
#do_dnn_wordbag(x_train, x_test, y_train, y_test)
print("get_features_by_tf")
x,y=get_features_by_tf()
x_train, x_test, y_train, y_test = train_test_split(x, y, test_size = 0.4, random_state = 0)
#CNN
do_cnn_wordbag(x_train, x_test, y_train, y_test)
#RNN
#do_rnn_wordbag(x_train, x_test, y_train, y_test)
12、运行结果
| Adam | epoch: 005 | loss: 0.09914 - acc: 0.9897 -- iter: 3000/3103
Training Step: 159 | total loss: 0.09456 | time: 70.005s
| Adam | epoch: 005 | loss: 0.09456 - acc: 0.9907 -- iter: 3100/3103
Training Step: 160 | total loss: 0.09103 | time: 79.386s
| Adam | epoch: 005 | loss: 0.09103 - acc: 0.9907 | val_loss: 0.12595 - val_acc: 0.9657 -- iter: 3103/3103
--
Process finished with exit code 0
其实大家可以对比每种算法的效果,自己也可以多写几个算法调试。
|