这是知识图谱问答博客的系列二,相比于上一篇博客我的第一次知识图谱问答,区别在于,创建知识图谱的方式不一样、意图识别+槽位提取的方法不同。另外总结与展望干货满满的。
这次要实现的是一个“电影知识图谱”。今天的内容架构与上一篇相似,步骤还是:构建图谱、分析问题类型(分类)、提取槽位、查询图谱。
一、构建图谱?
与上一篇“直接定义Node,或者执行query语句”不同,这次采用将csv文件导入neo4j数据库的方法,然后执行cypher语句。不用读取到系统的栈内存中。
#导入节点 演员信息
cql='''
LOAD CSV WITH HEADERS FROM 'file:///person.csv' AS line
MERGE (p:Person { pid:toInteger(line.pid),birth:line.birth,
death:line.death,name:line.name,
biography:line.biography,
birthplace:line.birthplace})
'''
执行Cypher语句自动导入演员信息节点。首先 在neo4j中的import文件夹下找到对应的CSV文件,然后 MERGE创建新的节点(有则不创建,无则创建),定义好p节点的pid/birth/death等属性,最后 graph.run(cql)实现图谱的创建。
构建图谱的程序如下,
# -*- coding: utf-8 -*-
'''
将csv文件导入neo4j数据库
import文件夹是neo4j默认的数据导入文件夹
所以首先要将data文件夹下所有csv文件拷贝到neo4j数据库的根目录import文件夹下,没有则先创建import文件夹
然后运行此程序
'''
# from neo4j import GraphDatabase
# uri ="neo4j://127.0.0.1:7687"
# driver = GraphDatabase.driver(uri, auth=("neo4j","neo4jneo4j"))
# graph = driver.session()
from py2neo import Graph
graph = Graph(host="localhost",port=7687,user="neo4j",password="neo4jneo4j")
"""
#测试
cql='''
MATCH (p:Person)
where p.name="张柏芝"
return p
'''
#清空数据库
#data = graph.run('MATCH (n) OPTIONAL MATCH (n)-[r]-() DELETE n,r')
data = graph.run(cql)
print(list(data)[0]['p']["biography"])
"""
#导入节点 电影类型 == 注意类型转换
cql='''
LOAD CSV WITH HEADERS FROM "file:///genre.csv" AS line
MERGE (p:Genre{gid:toInteger(line.gid),name:line.gname})
'''
result = graph.run(cql)
print(result,"电影类型 存储成功")
#导入节点 演员信息
cql='''
LOAD CSV WITH HEADERS FROM 'file:///person.csv' AS line
MERGE (p:Person { pid:toInteger(line.pid),birth:line.birth,
death:line.death,name:line.name,
biography:line.biography,
birthplace:line.birthplace})
'''
result = graph.run(cql)
print(result,"演员信息 存储成功")
#导入节点 电影信息
cql='''
LOAD CSV WITH HEADERS FROM "file:///movie.csv" AS line
MERGE (p:Movie{mid:toInteger(line.mid),title:line.title,introduction:line.introduction,
rating:toFloat(line.rating),releasedate:line.releasedate})
'''
result = graph.run(cql)
print(result,"电影信息 存储成功")
#导入关系 actedin 电影是谁参演的 1对多
cql='''
LOAD CSV WITH HEADERS FROM "file:///person_to_movie.csv" AS line
match (from:Person{pid:toInteger(line.pid)}),(to:Movie{mid:toInteger(line.mid)})
merge (from)-[r:actedin{pid:toInteger(line.pid),mid:toInteger(line.mid)}]->(to)
'''
result = graph.run(cql)
print(result,"电影信息<-->演员信息 存储成功")
#导入关系 is 电影是什么类型 == 1对多
cql='''
LOAD CSV WITH HEADERS FROM "file:///movie_to_genre.csv" AS line
match (from:Movie{mid:toInteger(line.mid)}),(to:Genre{gid:toInteger(line.gid)})
merge (from)-[r:is{mid:toInteger(line.mid),gid:toInteger(line.gid)}]->(to)
'''
result = graph.run(cql)
print(result,"电影信息<-->电影类型 存储成功")
注意:执行的时候记住CSV文件有固定的格式,且不能为空,否则提示NULL创建失败。
二、问题分类+槽位提取
目的还是一样,区分问题问什么类型,稳的是哪个实体关键词(关键词槽位)。与第一篇不同,这里步骤分为三步。
步骤 1:对语句进行分词,对词性进行标注。
步骤 2:识别意图,得到问题类型模板
步骤 1:语句分词,词性标注?
# 对问题进行词性标注
def question_posseg(self):
jieba.load_userdict("./data/userdict.txt")
clean_question = re.sub("[\s+\.\!\/_,$%^*(+\"\')]+|[+——()?【】“”!,。?、~@#¥%……&*()]+","",self.raw_question)
self.clean_question=clean_question
question_seged=jieba.posseg.cut(str(clean_question))
result=[]
question_word, question_flag = [], []
for w in question_seged:
temp_word=f"{w.word}/{w.flag}"
result.append(temp_word)
# 预处理问题
word, flag = w.word,w.flag
question_word.append(str(word).strip())
question_flag.append(str(flag).strip())
assert len(question_flag) == len(question_word)
self.question_word = question_word
self.question_flag = question_flag
# print("question_word:",question_word)
# print("question_flag:",question_flag)
print("result:",result)
return result
中间加载一个userdict的分词库,通过jieba加载用于分词。其中userdict如下介绍
ヒロイン危機一髪 15 nm 赏金猎人 15 nm 13.7十亿年 15 nm 羊与钢的森林 15 nm 粉红色高跟鞋 15 nm 希亚:勇敢的心 15 nm 越过舍伍德森林 15 nm EXO showcase 15 nm 黑道勇士 15 nm 深入敌后2:邪恶轴心 15 nm 银与金 15 nm 杀死一个人 15 nm
如,赏金猎人是词库,15是固定值不用关注,nm是对应的分词属性标注。这样子可以直接通过分词提取对应实体。【这也是一个新的应用点】
本质上还是标注大量的实体样本,从文本中匹配,只不过在分词过程中,完成了这个匹配。
步骤 2:识别问题类型及模板
下面去识别语句的意图,也就是问题类型分类,对应到问题模板中去。这里分类采用学习的方法,对问题类型进行提取文本特征、学习分类。【这是一个创新点】
其过程为:1.对问题抽象处理;2.训练文本分类模型;3.进行分类预测。以“周润发演过多少部”为例。
对问题抽象处理:依赖分词词性标注的成果['周润发/nr', '演/v', '过/ug', '多少/m', '部/n'],将问题进行抽象为含词性的信息,抽象问题为: nr演过多少部(周润发实体抽象化)
训练文本分类:针对提供的训练样本进行问题的分类,这里的训练样本都是抽象问题!!!!
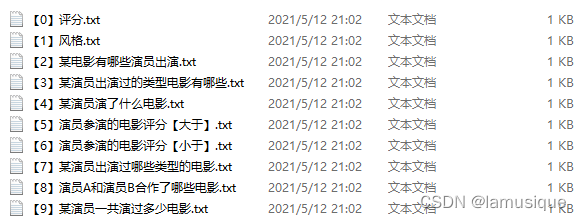 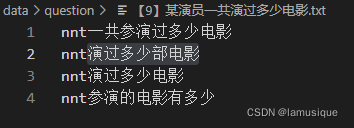
?提取TF-IDF特征,训练多项式贝叶斯分类模型(常用文本词频特征模型),完成模型的训练?
# 获取训练数据
def read_train_data(self):
train_x=[]
train_y=[]
file_list=getfilelist("./data/question/")
# 遍历所有文件
for one_file in file_list:
# 获取文件名中的数字
num = re.sub(r'\D', "", one_file)
# 如果该文件名有数字,则读取该文件
if str(num).strip()!="":
# 设置当前文件下的数据标签
label_num=int(num)
# 读取文件内容
with(open(one_file,"r",encoding="utf-8")) as fr:
data_list=fr.readlines()
for one_line in data_list:
word_list=list(jieba.cut(str(one_line).strip()))
# 将这一行加入结果集
train_x.append(" ".join(word_list))
train_y.append(label_num)
print(train_x,train_y)
return train_x,train_y
# 训练并测试模型-NB
def train_model_NB(self):
X_train, y_train = self.train_x, self.train_y
self.tv = TfidfVectorizer()
train_data = self.tv.fit_transform(X_train).toarray()
clf = MultinomialNB(alpha=0.01)
clf.fit(train_data, y_train)
return clf
# 预测
def predict(self,question):
question=[" ".join(list(jieba.cut(question)))]
test_data=self.tv.transform(question).toarray()
y_predict = self.model.predict(test_data)[0]
print("question type:",y_predict)
return y_predict
进行分类预测:对抽象问题[nr演过多少部],进行预测类型。得到模板编号(最重要的在这)
def get_question_template(self):
# 抽象问题
for item in ['nr','nm','ng']:
while (item in self.question_flag):
ix=self.question_flag.index(item)
self.question_word[ix]=item
self.question_flag[ix]=item+"ed"
# 将问题转化字符串
str_question="".join(self.question_word)
print("抽象问题为:",str_question)
# 通过分类器获取问题模板编号
question_template_num=self.classify_model.predict(str_question)
print("使用模板编号:",question_template_num)
根据模板编号,得到对应的问题模板。【其实这步有没有无所谓】
question type: 9 使用模板编号: 9 问题模板: nnt 电影数量
question_template=self.question_mode_dict[question_template_num]
print("问题模板:",question_template)
question_template_id_str=str(question_template_num)+"\t"+question_template
return question_template_id_str
注意:问题模板是构建出问题的通用范式,但是无法用于图谱的查询。所以这步有没有无所谓,只是去把意图识别这件事的输出闭环了,得到一个问题模板。
0:nm 评分 1:nm 类型 2:nm 演员列表 3:nnt ng 电影作品 4:nnt 电影作品 5:nnt 参演评分 大于 x 6:nnt 参演评分 小于 x 7:nnt 电影类型 8:nnt nnr 合作 电影列表 9:nnt 电影数量
槽位提取在词性标注中已经做完了。
? ? ? ? # 对问题进行词性标注
? ? ? ? self.pos_quesiton=self.question_posseg()
? ? ? ? # 得到问题的模板
? ? ? ? self.question_template_id_str=self.get_question_template()
问题类型 question_template_num;槽位实体:self.pos_quesiton
三、查询图谱
根据问题类型,槽位实体进行图谱查询。
# 根据问题模板的具体类容,构造cql语句,并查询
def query_template(self):
# 调用问题模板类中的获取答案的方法
try:
answer=self.questiontemplate.get_question_answer(self.pos_quesiton,self.question_template_id_str)
except:
answer="不好意思,您的问题我查询不到!"
return answer
其中get_question_answer函数如下。本质上用的还是问题编号template_id,
def get_question_answer(self,question,template):
# 如果问题模板的格式不正确则结束
assert len(str(template).strip().split("\t"))==2
template_id,template_str=int(str(template).strip().split("\t")[0]),str(template).strip().split("\t")[1]
self.template_id=template_id
self.template_str2list=str(template_str).split()
# 预处理问题
question_word,question_flag=[],[]
for one in question:
word, flag = one.split("/")
question_word.append(str(word).strip())
question_flag.append(str(flag).strip())
assert len(question_flag)==len(question_word)
self.question_word=question_word
self.question_flag=question_flag
self.raw_question=question
# 根据问题模板来做对应的处理,获取答案
answer=self.q_template_dict[template_id]()
return answer
其中q_template_dict对应9种查询处理模板,根据不同的问题类型编号实现调用,其如下定义。
self.q_template_dict={
0:self.get_movie_rating,
1:self.get_movie_type,
2:self.get_movie_actor_list,
3:self.get_actor_act_type_movie,
4:self.get_actor_act_movie_list,
5:self.get_movie_rating_bigger,
6:self.get_movie_rating_smaller,
7:self.get_actor_movie_type,
8:self.get_cooperation_movie_list,
9:self.get_actor_movie_num,
}
对图谱查询答复结果进行拼接。举个例子。get_movie_type对电影类型进行查询,
首先,对实体槽位进行解析,即对pos_quesiton进行解析,参考get_movie_name函数,定位到nm的位置index,提取到该词作为槽位实体;
然后,结合该槽位实体名称,拼装为cql,得到neo4j的数据库查询结果;
最后,对查询结果进行拼装返回查询结果。
# 1:nm 类型
def get_movie_type(self):
movie_name = self.get_movie_name()
cql = f"match(m:Movies)-[r:genres]->(b) where m.name='{movie_name}' return b.name"
print(cql)
answer = self.graph.run(cql)
answer_set=set(answer)
answer_list=list(answer_set)
answer="、".join(answer_list)
final_answer = movie_name + "是" + str(answer) + "等类型的电影!"
return final_answer
# 获取电影名字
def get_movie_name(self):
## 获取nm在原问题中的下标
tag_index = self.question_flag.index("nm")
## 获取电影名称
movie_name = self.question_word[tag_index]
return movie_name
这样就完成了一套知识图谱的问答系统。最后主函数如下,
import sys
from process_question import Question
# 创建问题处理对象,这样模型就可以常驻内存
que=Question()
# Restore
def enablePrint():
sys.stdout = sys.__stdout__
enablePrint()
result=que.question_process("周润发演过多少部")
print(result)
四、总结与展望
1、可以将知识图谱问答系统进行对外的封装服务,采用FLASK/Django等完成
2、文本分类的方法可以改进,但是将问题抽象,作为训练样本的方法是正确的。
3、系统最大的关键在于词性标注,本质上还是标注大量的实体样本,从文本中匹配,只不过分词过程中,完成了这个匹配。未来这个地方要改进,词性标注的作用是识别电影相关实体,也就是槽位提取,后面是不是可以采用BIO实体识别的方法进行槽位提取。
后续会再出新的想法,将这个系列做下去。
|