A Comparative Study of Statistical and Neural Network Models for PLC Network Traffic Anomaly Detection
一、摘要
在本文中,我们试图通过使用三种不同模型描述的分析网络流量中的异常检测来保护系统和计算机网络免受新的未知攻击。第一类由ARFIMA和Holt-Winters模型组成,它们具有统计依赖性。第二类包括神经网络自回归模型,该模型具有单隐层和滞后输入的特点,用于预测单变量时间序列。
二、介绍
多年来,人们一直使用基于以前隔离和分类的威胁模式的安全系统,称为特征码。防御新的未知攻击的一种手段是作战概念的一个相当彻底的变化。与搜索网络流量中的攻击特征不同,有必要浏览偏离正常流量特征的异常行为。这种方法的优势在于防止迄今为止未知的攻击,特别是针对信息系统的攻击,或仅仅形成所谓的zero-day攻击。 目前深入研究和开发的入侵/攻击检测方法是那些利用网络流量异常现象的方法。一种可能的解决方案是通过统计模型来检测异常行为,该模型描述分析的网络流量。最常用的是自回归模型或指数平滑模型,它们允许对分析的网络流量的特征进行估计。 在本文中,我们建议使用统计模型估计(ARFIMA、Holt-Winters)和神经网络自回归模型来定义给定智能照明网络流量的行为模式。异常检测过程包括正常行为参数(根据测试模型预测)与真实网络流量参数之间的比较。
三、系统建模
下图1为电力线通信(PLC)智能照明网络中提出的异常/攻击检测方法。智能灯网络的PLC流量通过流量集中器获取,流量集中器还充当IP网络的网关和转换器。 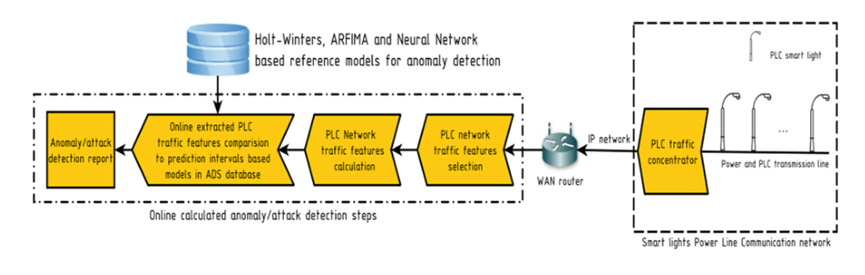
图 1
作为我们方法的第一步,我们提取PLC流量特征,然后计算流量特征,以实现表1所示的功能;下一步,将在线提取的流量特征与基于异常检测系统(ADS)数据库模型中存储的预测间隔的模型进行比较。
我们收集了主要与PLC信号参数有关的流量特征,如PF1–RSSI(PLC节点的接收信号强度指示)、PF2–SNR(信噪比),PF3–PER(每个时间间隔的数据包错误率)或与公共通信介质中使用的传输协议相关的参数,例如:PF5–NNG(每个时间间隔给定PLC节点的邻居数)或PF6–PR:给定PLC节点的数据包重传数。 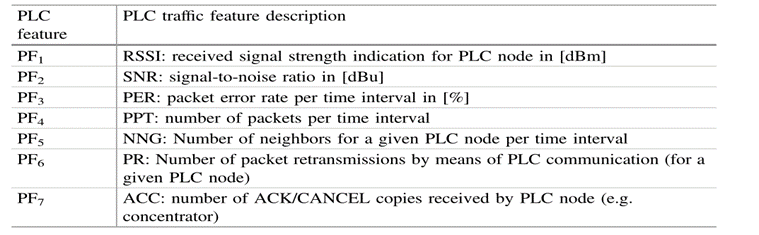
表 1
在提出的异常检测方法的正常工作过程中,我们从表1中提取每个流量特征,并检查在线获得的流量特征值是否在模型设定的预测区间内。如果在线提取的流量特征超出了模型设定的预测时间间隔,则表明可能存在异常或攻击。 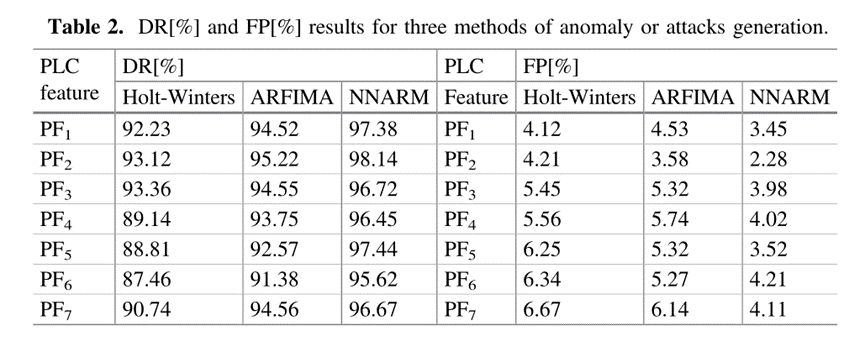
四、结论
本文提出了一种基于PLC传输的智能照明网络异常和攻击检测算法的解决方案。为了检测网络流量异常,在分析的网络参数中使用了真实网络流量和该流量的估计模型之间的差异。我们评估了Holt-winers、ARFIMA统计模型和神经网络解决方案。建立这三种解决方案的模型时,考虑了PLC流量在没有异常和攻击的情况下实现的预测间隔。我们用三种不同的异常和攻击场景测试了我们的解决方案。
1. Esposito, M., Mazzariello, C., Oliviero, F., Romano, S.P., Sansone, C.: Evaluating pattern
recognition techniques in intrusion detection systems. In: Proceedings of the 5th
International Workshop on Pattern Recognition in Information Systems, PRIS 2005, In
conjunction with ICEIS 2005, Miami, FL, USA, pp. 144–153, May 2005
2. Chondola, V., Banerjee, A., Kumar, V.: Anomaly Detection: a Survey. ACM Comput. Surv.
41(3), 1–72 (2009)
3. Jackson, K.: Intrusion Detection Systems (IDS). Product Survey. Los Alamos National
Library, LA-UR-99-3883 (1999)
4. Lim, S.Y., Jones, A.: Network anomaly detection system: the state of art of network
behavior analysis. In: Proceedings of the 2008 International Conference on Convergence and
Hybrid Information Technology, pp. 459–465 (2008)
5. Patcha, A., Park, J.M.: An overview of anomaly detection techniques: existing solutions and
latest technological trends. Comput. Netw.51(12), 3448–3470 (2007)
6. Wei, L., Ghorbani, A.: Network anomaly detection based on wavelet analysis. In: EURASIP
Journal on Advances in Signal Processing, vol. 2009 (2009)
7. Lakhina, A., Crovella, M., Diot, C.H.: Characterization of network-wide anomalies in traffic
flows. In: Proceedings of the 4th ACM SIGCOMM Conference on Internet Measurement,
pp. 201–206 (2004)
8. Yaacob, A., Tan, I., Chien, S., Tan, H.: Arima based network anomaly detection. In:
Proceedings of the 2nd International Conference on Communication Software and
Networks IEEE, pp. 205–209, (2010)
9. Zhou, Z.G., Tang, P.: Improving time series anomaly detection based on exponentially
weighted moving average (EWMA) of season-trend model residuals. In: Proceedings of the
2016 IEEE International Geoscience and Remote Sensing Symposium (IGARSS), pp. 3414–
3417 (2016)
10. Amini, M., Jalili, R., Shahriari, H.R.: RT-UNNID: a practical solution to real-time network-
based intrusion detection using unsupervised neural networks. Comput. Secur.25, 459–468
(2006)
11. Azkuna, I.: Smart Cities Study: International study on the situation of ICT, innovation and
Knowledge in cities, The Committee of Digital and Knowledge‐based Cities of UCLG,
Bilbao (2012)
12. Mitchell, W.J.: Intelligent cities. Universitat Oberta de Catalunya (UOC) Papers: E-Journal on
the Knowledge Society, no. 5. (2007).https://www.uoc.edu/uocpapers/5/dt/eng/mitchell.pdf
13. Wu, Y., Shi, Ch., Zhang, X., Yang, W.: Design of new intelligent street light control system.
In: 8th IEEE International Conferences on Control and Automation (ICCA), pp. 1423–1427
(2010)
14. Abouzakhar, N.: Critical infrastructure cybersecurity: a review of recent threats and
violations. In: 12th European Conference on Cyber Warfare and Security, pp. 1–10 (2013)
15. Elmaghraby, A.S., Losavio, M.M.: Cyber security challenges in smart cities: Safety, security
and privacy. J. Adv. Res.5(4), 491–497 (2014)
16. Kiedrowski, P.: Toward more efficient and more secure last mile smart metering and smart
lighting communication systems with the use of PLC/RF hybrid technology. Int. J. Distrib.
Sens. Netw.2015, 1–9 (2015)
17. Holt, C.C.: Forecasting seasonals and trends by exponentially weighted moving averages,
ONR Memorandum, vol. 52, Pittsburgh, PA: Carnegie Institute of Technology. Available
from the Engineering Library, University of Texas at Austin (1957)
18. Winters, P.R.: Forecasting sales by exponentially weighted moving averages. Manage. Sci.
6, 324–342 (1960)
19. Archibald, B.C.: Parameter Space of the Holt-Winters’Model. Int. J. Forecast.6, 199–209
(1990)
20. Gardner, E.S.: Exponential smoothing: the state of the art Part II. Int. J. Forecast.22, 637–
666 (2006)
21. Granger, C.W.J., Joyeux, R.: An introduction to long-memory time series models and
fractional differencing. J. Time Ser. Anal.1, 1 5–29 (1980)
22. Hosking, J.R.M.: Fractional differencing. Biometrika68, 165–176 (1981)
23. Box, G., Jenkins, G., Reinsel, G.: Time series analysis. Holden-day San Francisco (1970)
24. Crato, N., Ray, B.K.: Model selection and forecasting for long-range dependent processes.
J. Forecast.15, 107–125 (1996)
25. Cogollo, M.R., Velasquez, J.D.: Are neural networks able to forecast nonlinear time series
with moving average components? IEEE Lat. Am. Trans.13(7), 2292–2300 (2015)
26. Zhang, G.P., Patuwo, B.E., Hu, M.Y.: A simulation study of artificial neural networks for
nonlinear time series forecasting. Comput. Oper. Res.28, 381–396 (2001)
|