首先,在对图像进行奇异值分解之前,我们应当明白SVD的原理。 在矩阵原理这门课里,我们曾经学过奇异值分解,其中讲到
奇异值分解可以将一个比较复杂的矩阵用更小更简单的几个子矩阵的相乘来表示,这些小矩阵描述的是矩阵的重要的特性。
在这里,我推荐对奇异值分解原理不了解,或者忘得差不多的朋友先去看看这篇文章,讲的非常详细,一定能让你回想起在课堂上学的东西。
https://zhuanlan.zhihu.com/p/29846048
而本篇博客,主要讲如何使用SVD对图像进行压缩的代码的实现。
clc
close all
clear all
path='D:\xx\xx.xx';
I=imread(path);
I=im2double(I);
red = I(:,:,1);
green = I(:,:,2);
blue = I(:,:,3);
Ig=rgb2gray(I);
FirSV=1;
SecfSV=10;
%进行压缩
cRed = svdCompress(red,FirSV,SecfSV);
cGreen = svdCompress(green,FirSV,SecfSV);
cBlue = svdCompress(blue,FirSV,SecfSV);
img1(:,:,1) = cRed;
img1(:,:,2) = cGreen;
img1(:,:,3) = cBlue;
imshow(im2uint8(img1));
function [compressedImage] = svdCompress(rawGrayImage,fir,sec)
% get the size of primitive image
[rowCount,~] = size(rawGrayImage);
% we can get U,S,V directly in matlab
[U,S,V] = svd(rawGrayImage);
% big idea here,select the number of K maximum singular values
% use 0 to denote that we discard the remained small singular values
S1 = S(:,fir:sec);
V1=V(:,fir:sec);
%compressedImage=u*s*v'
compressedImage = U*S1*V1';
end
结果1: 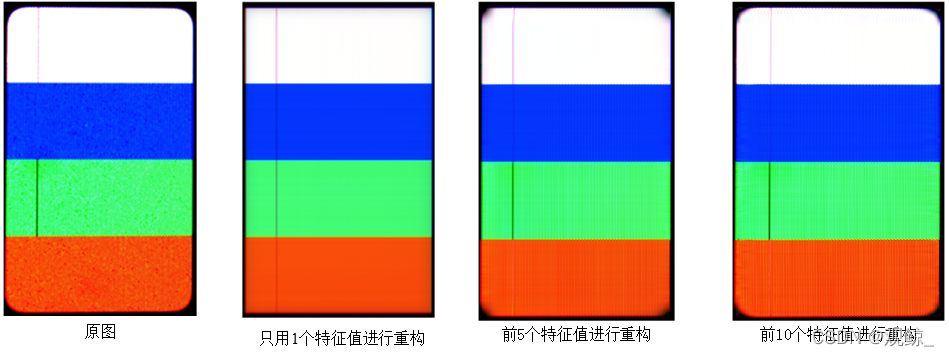 原图: 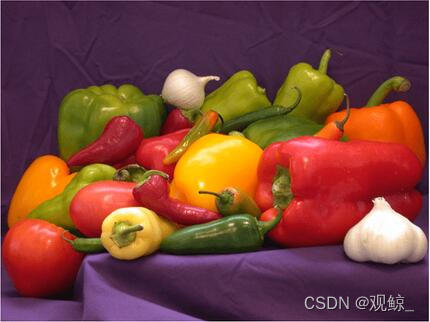 只使用第一个奇异值:
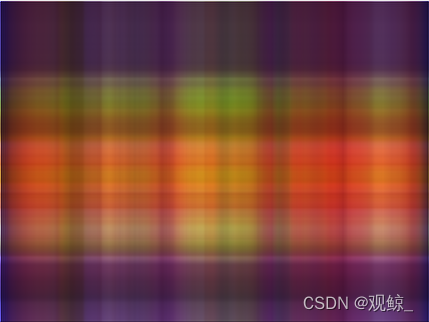 使用前3个奇异值: 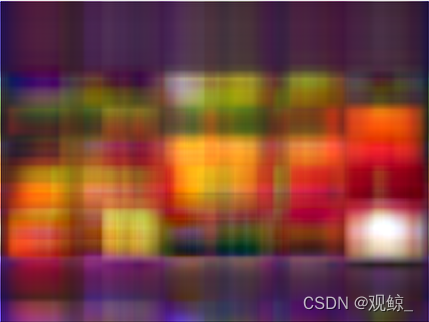 前10个奇异值: 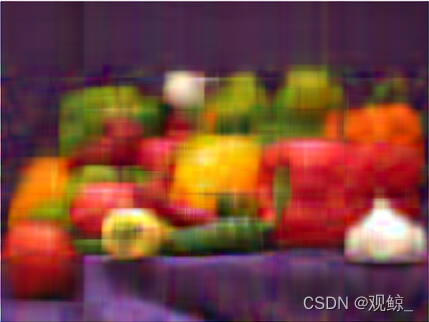 前30个奇异值: 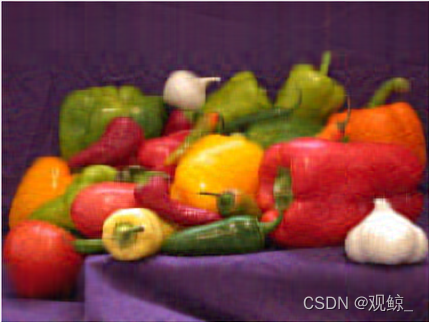 前80个奇异值: 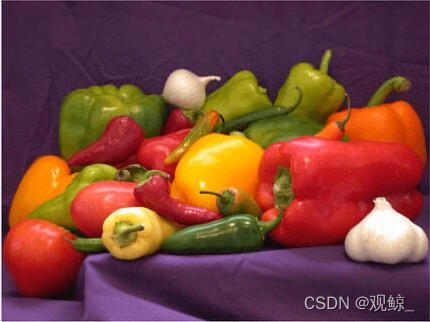
|