前言
??卷积神经网络取得了突破性进展,效果也非常理想,但是卷积神经网络的学习过程难以从理论上难以解释,因此被不少人诟病。因此可视化其的学习过程十分重要,DeepDream 模型的目的也正是如此。DeepDearm 模型在2015年由谷歌提出,理论基础是2013年所提出的《Visualizing and Understanding Convolutional Neural Networks》,该文章提出了使用梯度上升的方法可视化网络每一层的特征,即用一张噪声图像输入网络,反向更新的时候不更新网络权重,而是更新初始图像的像素值,以这种训练图像的方式可视化网络。深度学习领域奠基性的经典教材《深度学习》的封面就是使用 DeepDream 模型生成的。 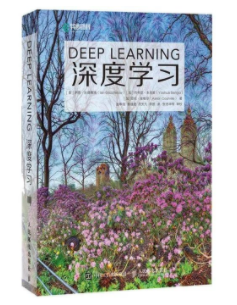
1 DeepDream原理
??DeepDream 为了说明CNN学习到的各特征的意义,将采用放大处理的方式。具体来说就是使用梯度上升的方法可视化网络每一层的特征,即用一张噪声图像输入网络,但反向更新的时候不更新网络权重,而是更新初始图像的像素值,以这种“训练图像”的方式可视化网络。此外输入图像也可以是一些正常的图片,这样的话就是生成背景图像之类的。 ??DeepDream 如何放大图像特征?比如:有 一个网络学习了分类猫和狗的任务,给这个网络一张云的图像,这朵云可能比较像狗,那么机器提取的特征可能也会像狗。假设对应一个特征最后输入概率为[0.6,0.4],0.6表示为狗的概率,0.4则表示为猫的概率,那么采用
L
2
L_2
L2?范数可以很好达到放大特征的效果。对于这样一个特征,
L
2
=
x
1
2
+
x
2
2
L_2 = x_1^{2} + x_2^{2}
L2?=x12?+x22?,若
x
1
x_1
x1? 越大,
x
2
x_2
x2?越小,则
L
2
L_2
L2?越大,所以只需要最大化
L
2
L_2
L2?就能保证当
x
1
x_1
x1? >
x
1
x_1
x1?的时候, 迭代的轮数越多
x
1
x_1
x1?越大,
x
2
x_2
x2?越小,所以图像就会越来越像狗。每次迭代相当 于计算
L
2
L_2
L2?范数,然后用梯度上升的方法调整图像。优化的就不再是优化权重参数,而是特征值或像素点,因此,构建损失函数时,不使用通常的交叉熵,而是最大化特征值的L2范数。使图像经过网络之后提取的特征更像网络隐含的特征。具体实现的时候还要通过多尺度、随机 移动等方法获取比较好的结果。
2 DeepDream算法流程
??使用基本图像,它输入到预训练的CNN。并正向传播到特定层。 ??为了更好地理解该层学到了什么,我们需要最大化通过该层激活值。这里要解释一下什么是激活值,激活值表示属于某类的概率大小,比如说二分类问题中,用[0,1]表示两类的标签,我们规定当神经网络的输出大于0就被分类到1(100% 被激活),小于0就分到0(没有被激活),所以在此情况下激活值只有100%或者0%,但是我们在平常的多分类任务中希望它可以是0%~100%的任意值。激活值越大,激活程度越高,对于分类,也就意味着它属于这一类的概率越大。DeepDream 以该层输出为梯度,然后在输入图像上完成渐变上升,以最大化该层的激活值。不过,光这样做并不能产生好的图像。为了提高训练质量,需要使用一些技术使得到的图像更好。通常可以进行高斯模糊以使图像更平滑,使用多尺度(又称为八度)的图像进行计算。先连续缩小输入图像,然后,再逐步放大,并将结果合并为一个图像输出。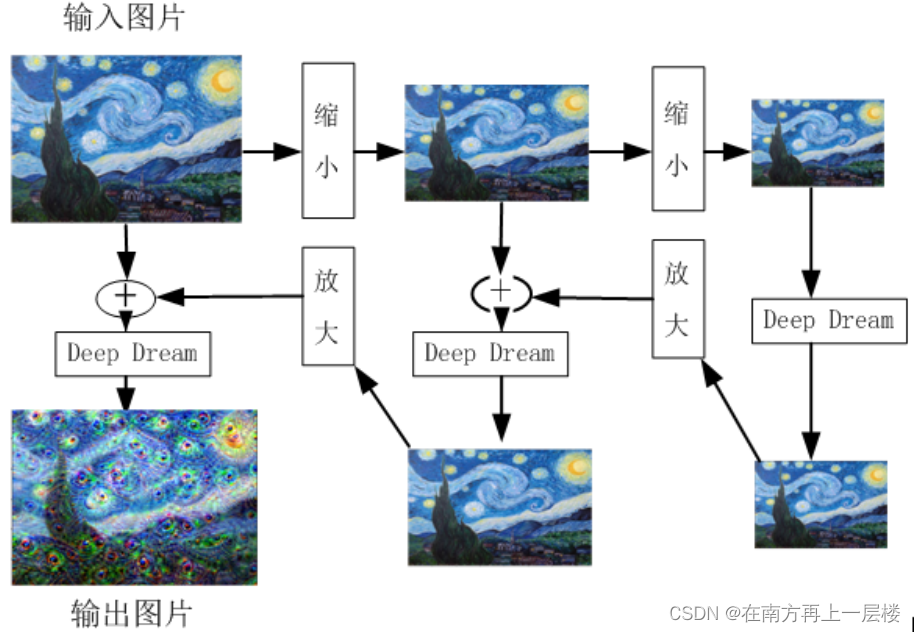
??先对图像连续做二次等比例缩小,该比例是1.5,之所以要缩小,图像缩小是为了让图像的像素点调整后所得结果图案能显示的更加平滑,过程主要是抑制了图像的高频成分,放大了低频成分。缩小二次后,把图像每个像素点当作参数,对它们求偏导,这样就可以知道如何调整图像像素点能够对给定网络层的输出产生最大化的刺激。
3 PyTorch实现DeepDream
??本次实现是取 VGG19 模型为预训练模型,将获取的特征最大化之后展示在一张普通的图像上,本次使用的是梵高的星空图。为了训练更加有效,还使用对图像进行不同大小的缩放处理。 1) 下载预训练模型。VGG19 模型包括了三种不同的模块,第一个是特征提取模块 (features) ,一共有36层,第二个是池化层 (avgpool) ,只有一层,第三个是分类层 (classifier) ,一共有6层。
vgg = models.vgg19(pretrained = True).to(device)
modulelist = list(vgg.features.modules())
2) 函数 prod 主要功能是传入输入图像,正 向传播到 VGG19 的指定层(如第8层或第32层等),然后,用梯度上升更新输入图像的特征值。
def prod(image, feature_layers, iterations, lr, transform, device, vgg, modulelist) :
input = transform(image).unsqueeze(0)
input = input.to(device).requires_grad_(True)
vgg.zero_grad()
for i in range(iterations) :
out = input
for j in range(feature_layers) :
out = modulelist[j + 1](out)
loss = out.norm()
loss.backward()
with torch.no_grad() :
input += lr * input.grad
input = input.squeeze()
input = input.permute(1, 2, 0)
input = np.clip(deprocess(input, device).detach().cpu().numpy(), 0, 1)
image = Image.fromarray(np.uint8(input * 255))
return image
3) 函数 deep_dream_vgg 是一个递归函数,多次缩小图像,然后调用函数 prod 。接着再放大结果,并与按一定比例图像混合在一起,最终得到与输入图像相同大小的输出图像。
def deep_dream_vgg(image, feature_layers, iterations, lr, transform, device, vgg, modulelist, octave_scale = 2, num_octaves = 100) :
if num_octaves > 0 :
image1 = image.filter(ImageFilter.GaussianBlur(2))
if (image1.size[0] / octave_scale < 1 or image1.size[1] / octave_scale < 1) :
size = image1.size
else :
size = (int(image1.size[0] / octave_scale), int(image1.size[1] / octave_scale))
image1 = image1.resize(size, Image.ANTIALIAS)
image1 = deep_dream_vgg(image1, feature_layers, iterations, lr, transform, device, vgg, modulelist, octave_scale, num_octaves - 1)
size = (image.size[0], image.size[1])
image1 = image1.resize(size, Image.ANTIALIAS)
image = ImageChops.blend(image, image1, 0.6)
img_result = prod(image, feature_layers, iterations, lr, transform, device, vgg, modulelist)
img_result = img_result.resize(image.size)
return img_result
4 全部代码(详细注释)
import torch
import matplotlib.pyplot as plt
import numpy as np
from PIL import Image, ImageFilter, ImageChops
from torchvision import models
from torchvision import transforms
def load_image(path) :
img = Image.open(path)
return img
def deprocess(image, device):
image = image * torch.tensor([0.229, 0.224, 0.225], device = device) + torch.tensor([0.485, 0.456, 0.406], device = device)
return image
def prod(image, feature_layers, iterations, lr, transform, device, vgg, modulelist) :
input = transform(image).unsqueeze(0)
input = input.to(device).requires_grad_(True)
vgg.zero_grad()
for i in range(iterations) :
out = input
for j in range(feature_layers) :
out = modulelist[j + 1](out)
loss = out.norm()
loss.backward()
with torch.no_grad() :
input += lr * input.grad
input = input.squeeze()
input = input.permute(1, 2, 0)
input = np.clip(deprocess(input, device).detach().cpu().numpy(), 0, 1)
image = Image.fromarray(np.uint8(input * 255))
return image
def deep_dream_vgg(image, feature_layers, iterations, lr, transform, device, vgg, modulelist, octave_scale = 2, num_octaves = 100) :
if num_octaves > 0 :
image1 = image.filter(ImageFilter.GaussianBlur(2))
if (image1.size[0] / octave_scale < 1 or image1.size[1] / octave_scale < 1) :
size = image1.size
else :
size = (int(image1.size[0] / octave_scale), int(image1.size[1] / octave_scale))
image1 = image1.resize(size, Image.ANTIALIAS)
image1 = deep_dream_vgg(image1, feature_layers, iterations, lr, transform, device, vgg, modulelist, octave_scale, num_octaves - 1)
size = (image.size[0], image.size[1])
image1 = image1.resize(size, Image.ANTIALIAS)
image = ImageChops.blend(image, image1, 0.6)
img_result = prod(image, feature_layers, iterations, lr, transform, device, vgg, modulelist)
img_result = img_result.resize(image.size)
return img_result
if __name__ == '__main__':
tranform = transforms.Compose([
transforms.Resize((224, 224)),
transforms.ToTensor(),
transforms.Normalize(mean = [0.485, 0.456, 0.406],
std = [0.229, 0.224, 0.225])
])
device = torch.device("cuda:0" if torch.cuda.is_available() else "cpu")
vgg = models.vgg19(pretrained = True).to(device)
modulelist = list(vgg.features.modules())
night_sky = load_image('starry_night.jpg')
night_sky_30 = deep_dream_vgg(night_sky, 36, 6, 0.2, tranform, device, vgg, modulelist)
plt.imshow(night_sky_30)
plt.show()
运行结果: 输入图像: 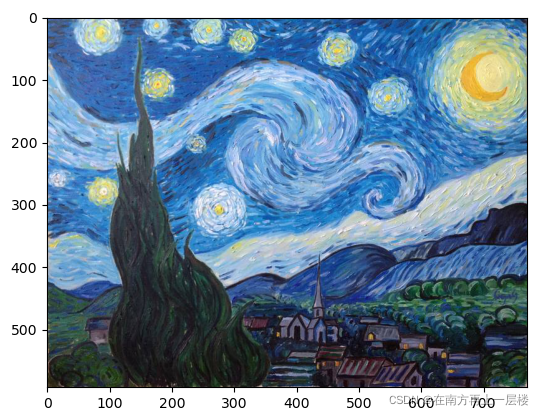
VGG19 的第10层学习的特征: 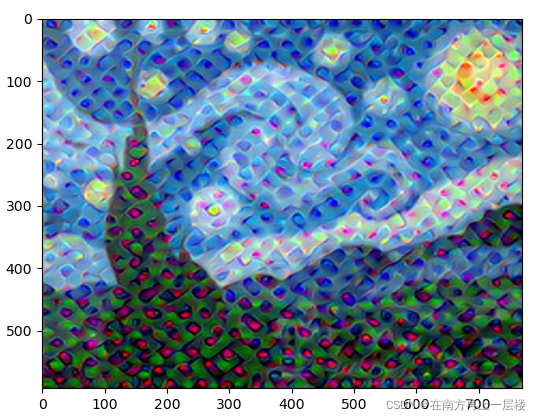 VGG19 的第20层学习的特征: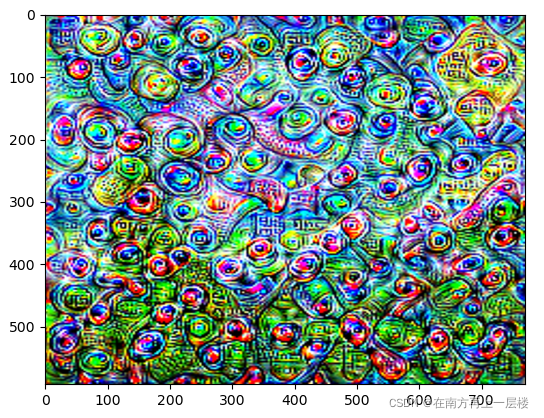 VGG19 的第30层学习的特征: 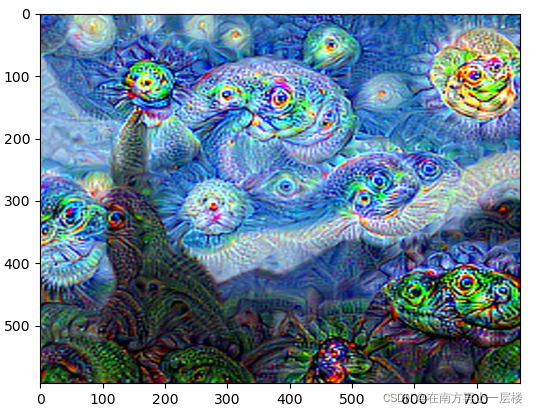 ??VGG19 预训练模型是基于ImageNet大数据集训练的模型,该数据集共有1000个类别。从上面的结果可以看出,越靠近顶部的层,其激活值表现就越全面或抽象,如像某些类别(比如狗)的图案。
|