import matplotlib.pyplot as plt
from mpl_toolkits.mplot3d import Axes3D
from sklearn import datasets
from sklearn.decomposition import PCA
iris = datasets.load_iris()
y = iris.target
X_reduced = PCA(n_components=3).fit_transform(iris.data)
fig = plt.figure(1, figsize=(8, 6))
ax = Axes3D(fig, elev=-150, azim=110)
ax.scatter(
X_reduced[:, 0],
X_reduced[:, 1],
X_reduced[:, 2],
c=y,
cmap=plt.cm.Set1,
edgecolor="k",
s=40,
)
ax.set_title("First three PCA directions")
ax.set_xlabel("1st eigenvector")
ax.w_xaxis.set_ticklabels([])
ax.set_ylabel("2nd eigenvector")
ax.w_yaxis.set_ticklabels([])
ax.set_zlabel("3rd eigenvector")
ax.w_zaxis.set_ticklabels([])
plt.show()
运行结果 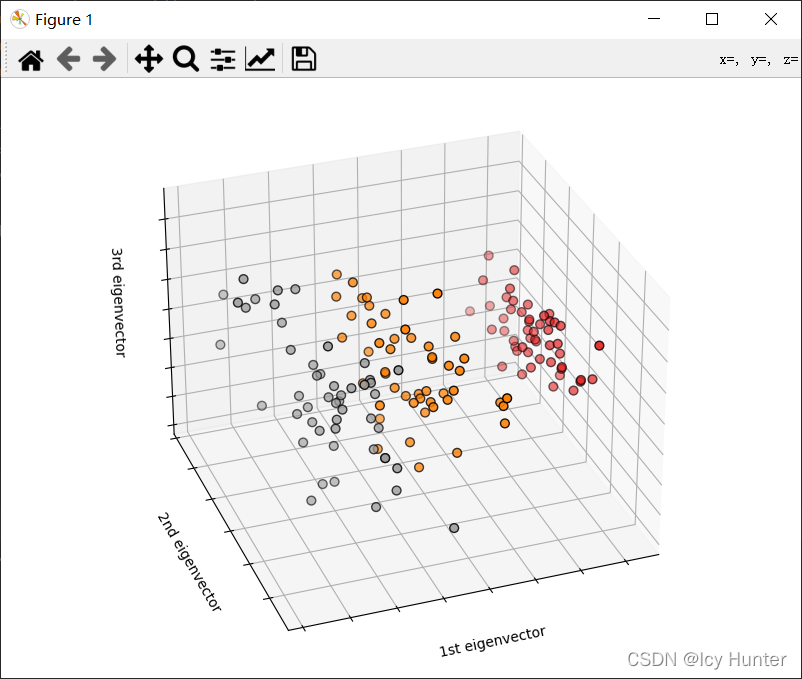 参考:https://scikit-learn.org/stable/auto_examples/datasets/plot_iris_dataset.html#sphx-glr-auto-examples-datasets-plot-iris-dataset-py
鸢尾花数据进行降维后使用DBSCAN聚类后三维可视化实例
import numpy as np
from sklearn.cluster import DBSCAN
from sklearn import metrics, datasets
from sklearn.decomposition import PCA
from sklearn.preprocessing import StandardScaler
from mpl_toolkits.mplot3d import Axes3D
import matplotlib.pyplot as plt
iris = datasets.load_iris()
labels_true = iris.target
X = PCA(n_components=3).fit_transform(iris.data)
X = StandardScaler().fit_transform(X)
db = DBSCAN(eps=0.6, min_samples=8).fit(X)
core_samples_mask = np.zeros_like(db.labels_, dtype=bool)
core_samples_mask[db.core_sample_indices_] = True
labels = db.labels_
n_clusters_ = len(set(labels)) - (1 if -1 in labels else 0)
n_noise_ = list(labels).count(-1)
print("Estimated number of clusters: %d" % n_clusters_)
print("Estimated number of noise points: %d" % n_noise_)
print("Homogeneity: %0.3f" % metrics.homogeneity_score(labels_true, labels))
print("Completeness: %0.3f" % metrics.completeness_score(labels_true, labels))
print("V-measure: %0.3f" % metrics.v_measure_score(labels_true, labels))
print("Adjusted Rand Index: %0.3f" % metrics.adjusted_rand_score(labels_true, labels))
print(
"Adjusted Mutual Information: %0.3f"
% metrics.adjusted_mutual_info_score(labels_true, labels)
)
print("Silhouette Coefficient: %0.3f" % metrics.silhouette_score(X, labels))
fig = plt.figure(1, figsize=(8, 6))
ax = Axes3D(fig, elev=-150, azim=110)
unique_labels = set(labels)
colors = ["r", "g", "b", "y"]
for k, c in zip(unique_labels, colors):
if k == -1:
c = "black"
class_member_mask = labels == k
xy = X[class_member_mask & core_samples_mask]
ax.scatter(
xy[:, 0],
xy[:, 1],
xy[:, 2],
c=c,
cmap=plt.cm.Set1,
edgecolor="k",
s=100,
label=str(c)
)
xy = X[class_member_mask & ~core_samples_mask]
ax.scatter(
xy[:, 0],
xy[:, 1],
xy[:, 2],
c=c,
cmap=plt.cm.Set1,
edgecolor="k",
s=40,
)
ax.legend(loc="upper left")
xlabel = ["1st eigenvector", "2nd eigenvector", "3rd eigenvector"]
ax.set_title("First three PCA directions")
ax.set_xlabel(xlabel[0])
ax.w_xaxis.set_ticklabels([])
ax.set_ylabel(xlabel[1])
ax.w_yaxis.set_ticklabels([])
ax.set_zlabel(xlabel[2])
ax.w_zaxis.set_ticklabels([])
plt.title("Estimated number of clusters: %d" % n_clusters_)
plt.show()
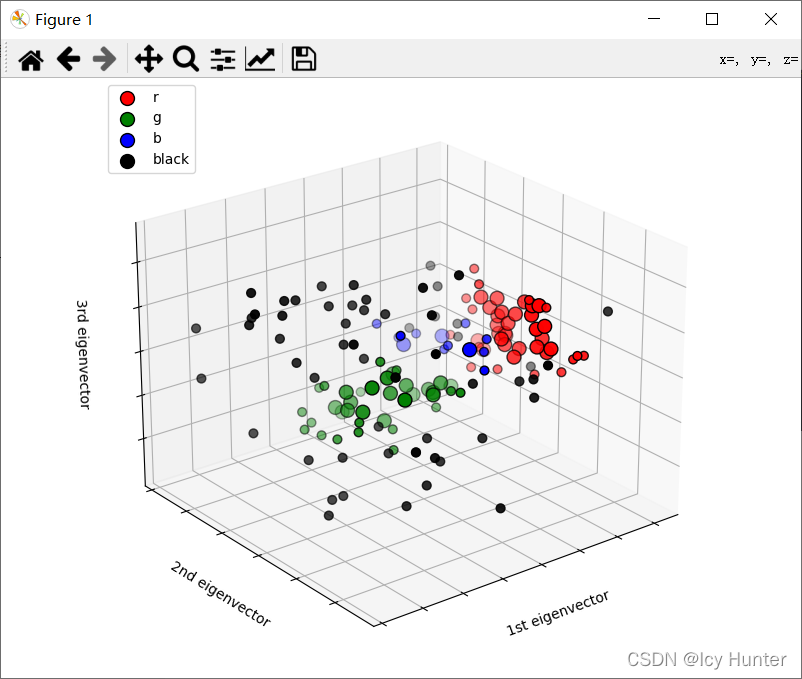
|