论文阅读
感谢P导 ShuffleNet: An Extremely Efficient Convolutional Neural Network for Mobile Devices
文章中提出了一个非常有效的Architecture——ShuffleNet,主要使用两种操作,分组PW卷积和通道重排,在保证准确率的情况下降低了计算代价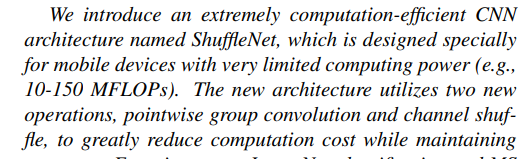 之前提出的模型,Xception和ResNeXt因为其中大量的Pw操作使得计算代价挺高,无法实现小型模型,因此,我们使用分组Pw COnv代替Pw Conv,为了减少分组Pw Conv带来的副作用(近亲繁殖?),提出使用通道重排(见论文 Fig 1)让信息在不同的组中的channel进行流通。因此ShuffleNet与其他网络相比,在相同的计算代价下,可以支持更多的Channel也就可以encode更多的信息  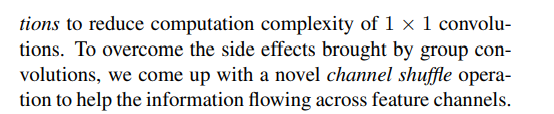 分组卷积第一次在AlexNet中提出,之后在ResNeXt中证明了他的又相信,Dw Conv在Xception中提出,Mobile结合pw和Dw使用depthwise separation,shufflenet用一种新方式来使用Conv 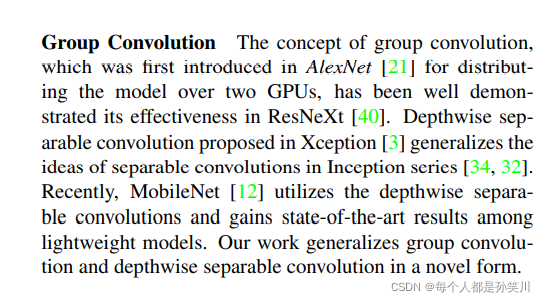 通道重排:首先将feature map转换成广义矩阵,之后进行transpose操作,重新flatten成feature map 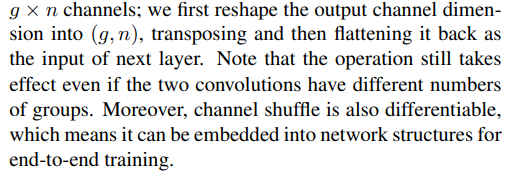 关于ShuffleNet的unit:residual block中33 Conv换成DwConv为(a),之后将其中的11 Conv换成 GConv并接一个Channel shuffle就是(b),stride为2的时候为?,在shortcut connection上,使用的是全局平均池化,而不是resnet中的Conv进行操作,在之后的addition操作中,使用的是concatenation而不是Add。 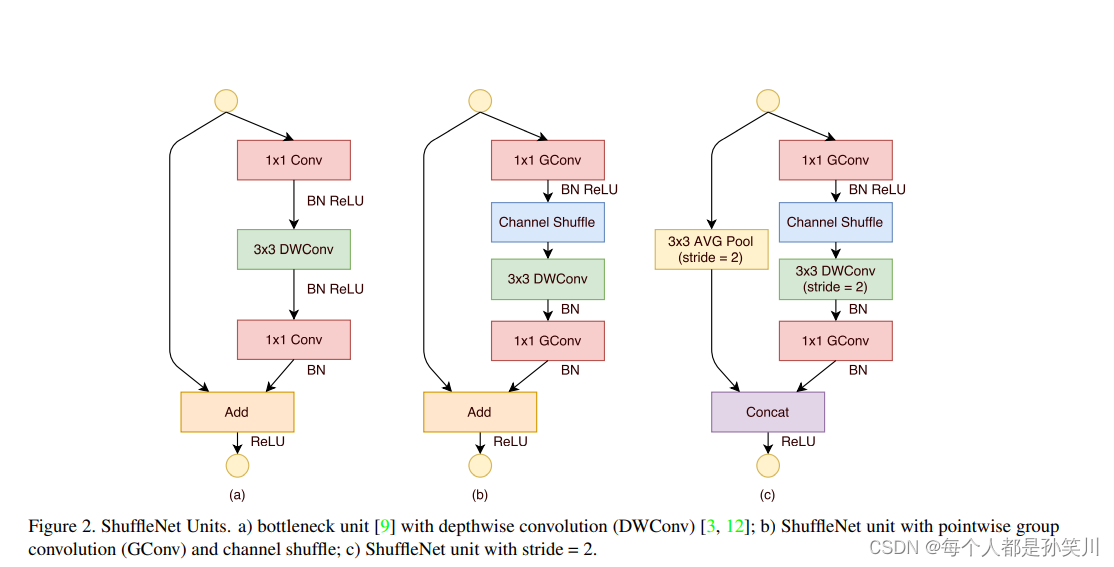 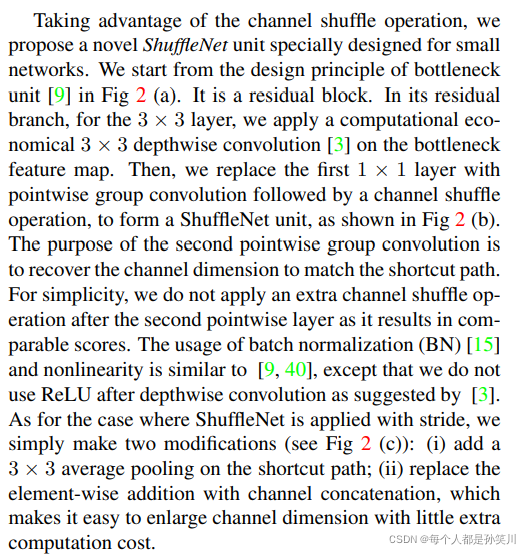 网络架构,在每个stage中的第一个block为stride=2,bottle neck中的channel为out feature map中的channel的1/4,添加两个超参数,g来控制分组的个数,来实现sparsity connection,factor s来控制每层的channel个数(类似于mobilenet中的α) 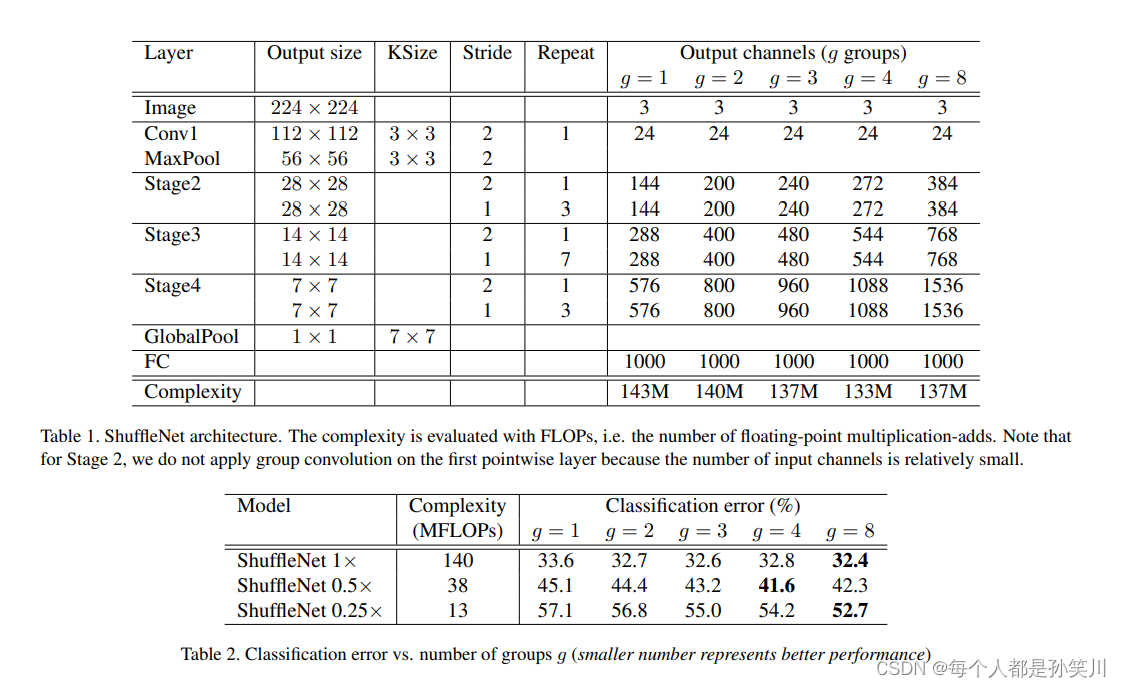 之后文章做了对比消融(Ablation Study)实验,分别在Gpw Conv和 channel shuffle上做了对比实验,也与其他的架构做了实验对比(在相同的计算代价下)
代码实现
model
构建1x1 3x3的基本模块 1x1要注意是否需要relu,3x3的要注意是否stride=2 之后搭建bottleneck模块,然后堆叠成shufflenet网络
import torch.nn.functional as F
import torch
import torch.nn as nn
from torch import Tensor
def channel_shuffle(x: Tensor, groups: int) -> Tensor:
batch_size, num_channels, height, width = x.size()
channels_per_group = num_channels // groups
x = x.view(batch_size, groups, channels_per_group, height, width)
x = torch.transpose(x, 1, 2).contiguous()
x = x.view(batch_size, -1, height, width)
return x
class conv1x1(nn.Module):
def __init__(self, in_channel, out_channel, group, relu=True, bias=False) -> None:
super(conv1x1, self).__init__()
self.relu = relu
self.group = group
if self.relu:
self.conv1x1 = nn.Sequential(
nn.Conv2d(in_channels=in_channel, out_channels=out_channel,
kernel_size=1, stride=1, groups=self.group, bias=bias),
nn.BatchNorm2d(out_channel),
nn.ReLU(inplace=True)
)
else:
self.conv1x1 = nn.Sequential(
nn.Conv2d(in_channels=in_channel, out_channels=out_channel,
kernel_size=1, stride=1, groups=self.group, bias=bias),
nn.BatchNorm2d(out_channel)
)
def forward(self, x):
if self.relu:
out = self.conv1x1(x)
return channel_shuffle(out, self.group)
return self.conv1x1(x)
class conv3x3(nn.Module):
def __init__(self, in_channel, stride, bias=False):
super(conv3x3, self).__init__()
self.conv3x3 = nn.Sequential(
nn.Conv2d(in_channels=in_channel, out_channels=in_channel,
kernel_size=3, stride=stride,padding=1, groups=in_channel, bias=bias),
nn.BatchNorm2d(in_channel)
)
def forward(self,x):
return self.conv3x3(x)
class bottleneck(nn.Module):
def __init__(self,in_channel,out_channel,stride,groups):
super(bottleneck,self).__init__()
self.stride=stride
channel=int(out_channel/4)
g=1 if in_channel==24 else groups
self.layer1=conv1x1(in_channel,channel,group=g,relu=True,bias=False)
self.layer2=conv3x3(channel,stride=stride,bias=False)
if self.stride==2:
self.layer3=conv1x1(channel,out_channel-in_channel,group=groups,relu=False,bias=False)
else:
self.layer3=conv1x1(channel,out_channel,group=groups,relu=False,bias=False)
self.shortcut=nn.Sequential(
nn.AvgPool2d(3,stride=2,padding=1)
)
def forward(self,x):
out=self.layer1(x)
out=self.layer2(out)
out=self.layer3(out)
if self.stride==2:
x=self.shortcut(x)
out=F.relu(torch.cat([out,x],1)) if self.stride==2 else F.relu(out+x)
return out
class ShuffleNet(nn.Module):
def __init__(self,stages_repeats,stages_out_channels,groups,num_classes=1000):
super(ShuffleNet,self).__init__()
self.conv1 = nn.Sequential(
nn.Conv2d(3, 24, kernel_size=3, stride=2, padding=1, bias=False),
nn.BatchNorm2d(24),
nn.ReLU(inplace=True)
)
self.maxpool = nn.MaxPool2d(kernel_size=3, stride=2, padding=1)
self.in_channel=24
self.layer1 = self._make_layer(stages_out_channels[0], stages_repeats[0], groups)
self.layer2 = self._make_layer(stages_out_channels[1], stages_repeats[1], groups)
self.layer3 = self._make_layer(stages_out_channels[2], stages_repeats[2], groups)
self.avgpool = nn.AdaptiveAvgPool2d((1, 1))
self.fc = nn.Linear(stages_out_channels[2], num_classes)
def _make_layer(self, out_channel, num_blocks, groups):
layers = []
for i in range(num_blocks):
if i == 0:
layers.append(bottleneck(self.in_channel,
out_channel,
stride=2, groups=groups))
else:
layers.append(bottleneck(self.in_channel,
out_channel,
stride=1, groups=groups))
self.in_channel = out_channel
return nn.Sequential(*layers)
def forward(self,x):
out=self.conv1(x)
out=self.maxpool(out)
out=self.layer1(out)
out=self.layer2(out)
out=self.layer3(out)
out=self.avgpool(out)
out=out.view(out.size(0),-1)
out=self.fc(out)
return out
def ShuffleNetG2(num_classes=1000):
model = ShuffleNet(stages_repeats=[4, 8, 4],
stages_out_channels=[200, 400, 800],
groups=2,
num_classes=num_classes)
return model
def ShuffleNetG3(num_classes=1000):
model = ShuffleNet(stages_repeats=[4, 8, 4],
stages_out_channels=[240, 480,960],
groups=3,
num_classes=num_classes)
return model
def ShuffleNetG4(num_classes=1000):
model = ShuffleNet(stages_repeats=[4, 8, 4],
stages_out_channels=[272,544,1088],
groups=4,
num_classes=num_classes)
return model
train
import os
import sys
import json
import torch
import torch.nn as nn
import torch.optim as optim
from torchvision import transforms, datasets
from tqdm import tqdm
from model_v1 import ShuffleNetG4
def main():
device = torch.device("cuda:0" if torch.cuda.is_available() else "cpu")
print("using {} device.".format(device))
batch_size = 16
epochs = 20
data_transform = {
"train": transforms.Compose([transforms.RandomResizedCrop(224),
transforms.RandomHorizontalFlip(),
transforms.ToTensor(),
transforms.Normalize([0.485, 0.456, 0.406], [0.229, 0.224, 0.225])]),
"val": transforms.Compose([transforms.Resize(256),
transforms.CenterCrop(224),
transforms.ToTensor(),
transforms.Normalize([0.485, 0.456, 0.406], [0.229, 0.224, 0.225])])}
data_root = os.path.abspath(os.path.join(os.getcwd(), "../dataset"))
image_path = os.path.join(data_root, "flower_data")
assert os.path.exists(image_path), "{} path does not exist.".format(image_path)
train_dataset = datasets.ImageFolder(root=os.path.join(image_path, "train"),
transform=data_transform["train"])
validate_dataset = datasets.ImageFolder(root=os.path.join(image_path, "val"),
transform=data_transform["val"])
val_num = len(validate_dataset)
train_num = len(train_dataset)
train_loader = torch.utils.data.DataLoader(train_dataset,
batch_size=batch_size, shuffle=True)
validate_loader = torch.utils.data.DataLoader(validate_dataset,
batch_size=batch_size, shuffle=False)
print("using {} images for training, {} images for validation.".format(train_num,
val_num))
flower_list = train_dataset.class_to_idx
cla_dict = dict((val, key) for key, val in flower_list.items())
json_str = json.dumps(cla_dict, indent=4)
with open('class_indices.json', 'w') as json_file:
json_file.write(json_str)
net = ShuffleNetG4(num_classes=5).to(device)
loss_function = nn.CrossEntropyLoss()
optimizer = optim.Adam(net.parameters(), lr=0.0001)
best_acc = 0.0
save_path = './ShuffleNetV1.pth'
train_steps = len(train_loader)
for epoch in range(epochs):
net.train()
running_loss = 0.0
train_bar = tqdm(train_loader)
for data in train_bar:
images, labels = data
optimizer.zero_grad()
logits = net(images.to(device))
loss = loss_function(logits, labels.to(device))
loss.backward()
optimizer.step()
running_loss += loss.item()
train_bar.desc = "train epoch[{}/{}] loss:{:.3f}".format(epoch + 1,
epochs,
loss)
net.eval()
acc = 0.0
with torch.no_grad():
val_bar = tqdm(validate_loader, file=sys.stdout)
for val_data in val_bar:
val_images, val_labels = val_data
outputs = net(val_images.to(device))
predict_y = torch.max(outputs, dim=1)[1]
acc += torch.eq(predict_y, val_labels.to(device)).sum().item()
val_bar.desc = "valid epoch[{}/{}]".format(epoch + 1,
epochs)
val_accurate = acc / val_num
print('[epoch %d] train_loss: %.3f val_accuracy: %.3f' %
(epoch + 1, running_loss / train_steps, val_accurate))
if val_accurate > best_acc:
best_acc = val_accurate
torch.save(net.state_dict(), save_path)
print('Finished Training')
if __name__ == '__main__':
main()
predict
**import os
import json
import torch
from PIL import Image
from torchvision import transforms
import matplotlib.pyplot as plt
from model_v1 import ShuffleNetG4
def main():
device = torch.device("cuda:0" if torch.cuda.is_available() else "cpu")
data_transform = transforms.Compose(
[transforms.Resize(256),
transforms.CenterCrop(224),
transforms.ToTensor(),
transforms.Normalize([0.485, 0.456, 0.406], [0.229, 0.224, 0.225])])
img_path = "../tulip.jpg"
print(img_path)
assert os.path.exists(img_path), "file: '{}' dose not exist.".format(img_path)
img = Image.open(img_path)
plt.imshow(img)
img = data_transform(img)
img = torch.unsqueeze(img, dim=0)
json_path = './class_indices.json'
assert os.path.exists(json_path), "file: '{}' dose not exist.".format(json_path)
json_file = open(json_path, "r")
class_indict = json.load(json_file)
model = ShuffleNetG4(num_classes=5).to(device)
model_weight_path = "./ShuffleNetV1.pth"
model.load_state_dict(torch.load(model_weight_path, map_location=device))
model.eval()
with torch.no_grad():
output = torch.squeeze(model(img.to(device))).cpu()
predict = torch.softmax(output, dim=0)
predict_cla = torch.argmax(predict).numpy()
print_res = "class: {} prob: {:.3}".format(class_indict[str(predict_cla)],
predict[predict_cla].numpy())
plt.title(print_res)
for i in range(len(predict)):
print("class: {:10} prob: {:.3}".format(class_indict[str(i)],
predict[i].numpy()))
plt.show()
if __name__ == '__main__':
main()
实验结果
都是使用group为4的模型 
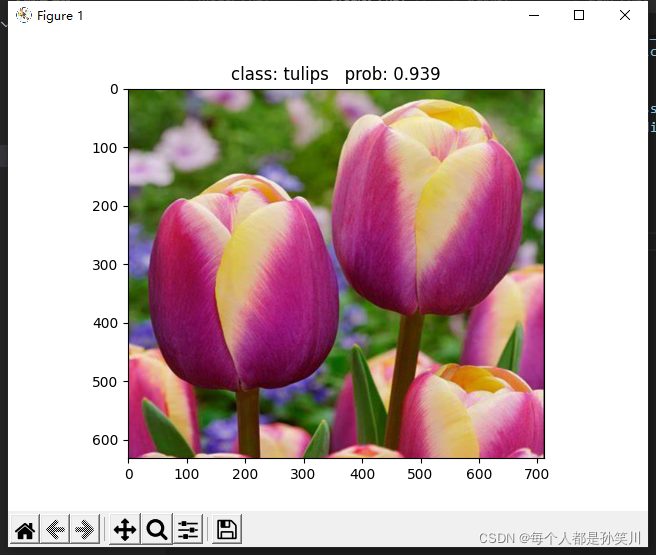
|