DGCN论文阅读
1. 网络架构
本文的主要思想: 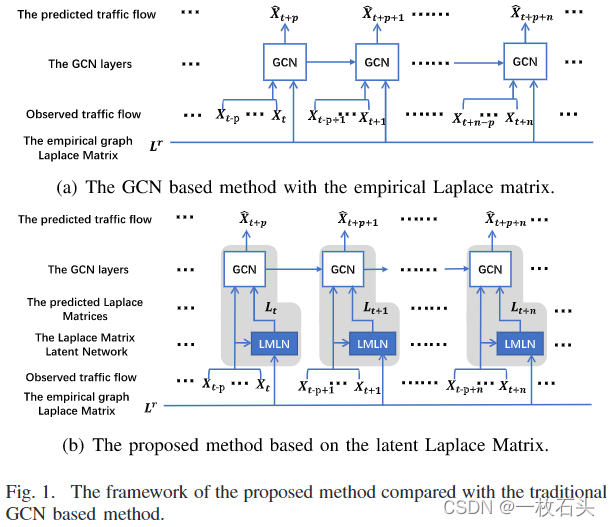
DGCN的网络框架: 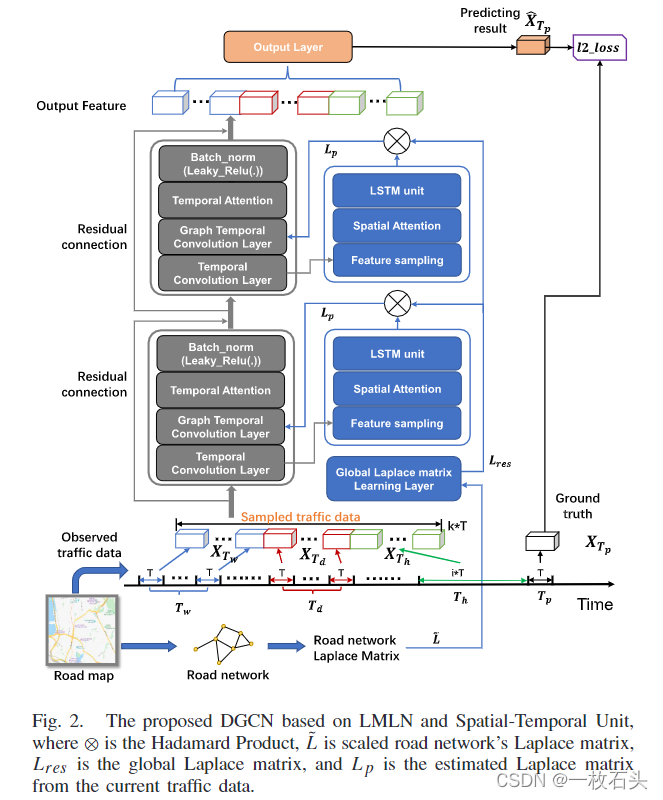
输入由三部分组成:1. the recent-period data X_Th 2. the daily-period data X_Td; 3. the weekly-period data X_Tw
网络主要由两个部分组成:1. LMLN(蓝色部分)和 2. GCN(灰色部分)
1.1 LMLN,Laplace Matrix Latent Network
看Fig. 2. 蓝色的部分,主要有一个Global Laplace matrix Learning Layer 和两个Laplace matrix prediction unit组成。
Laplace matrix prediction unit的结构如下,主要包括三个部分:1. the feature sampling; 2. the spatial attention; 3. LSTM unit : 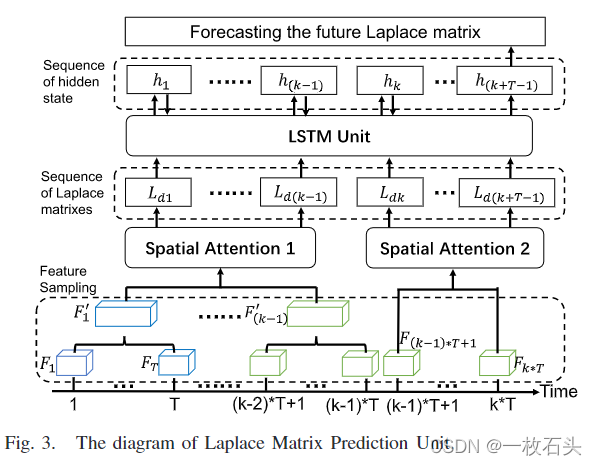
(1)Global Laplace Matrix Learning Layer*
(提到了参考文献17,maybe思路是从那里来的。)
这里进行了这样的一个数学运算: 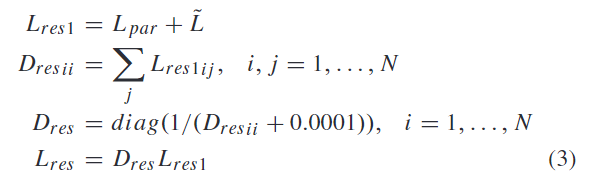
(2)Feature Sampling
这个模块的目的是:to reduce the data dimension of the traffic feature according to the importance of different time interval
把输入的数据按照时间维度分为k组,每组长度为T。除了最近的T个特征,其余的k-1组都将T步长的特征融合为1个。
前k-1组的操作可以表示为:  经过Feature Sampling,数据的维度变成这样:  然后融合后的k-1个特征和最后的这个T个特征会通过两个不同的Spatial Attention继续学习。
(3)Spatial Attention
Spatial Attention的计算借鉴了Transformer中self-attention的思想,但是具体的计算不同: 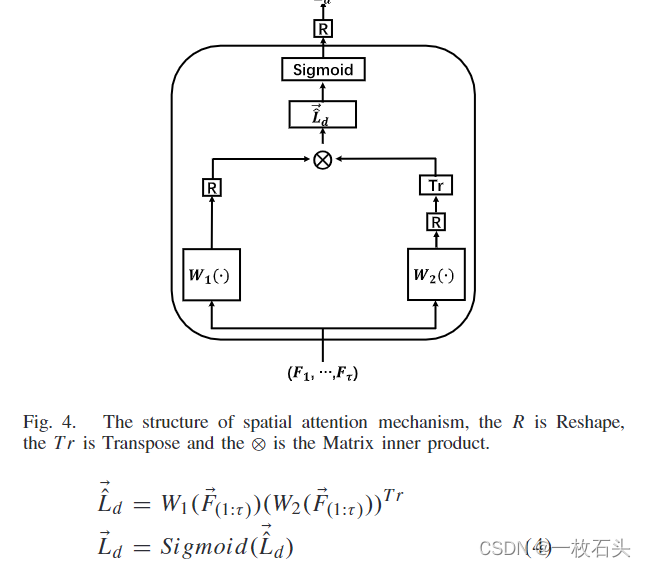 融合后的k-1个特征经过注意力机制产生k-1个邻接矩阵:  最后的T个特征经过注意力机制产生T个邻接矩阵:  最终该层的输出是k+T-1个邻接矩阵: ![[外链图片转存失败,源站可能有防盗链机制,建议将图片保存下来直接上传(img-Cxl8Voju-1646383434482)(C:\Users\admin\AppData\Roaming\Typora\typora-user-images\image-20220303161913550.png)]](https://img-blog.csdnimg.cn/9029eb833b11495781b3a3bdca37e6ae.png)
(4) LSTM Unit
将k+T-1个N×N的拉普拉斯矩阵沿着时间轴通过LSTM进行融合,LSTM就是一个普通的LSTM: 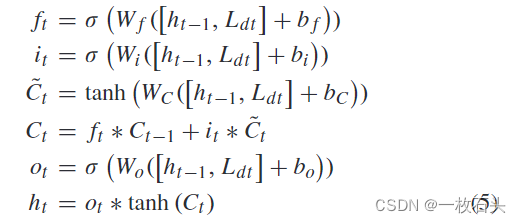
通过LSTM我们得到了Laplace matrix prediction unit的最终输出h_k+T-1
再与Global Laplace Matrix Learning Layer的输出 global Laplace matrix L_res结合,就得到了 Laplace matrix prediction network 的输出 L_p: 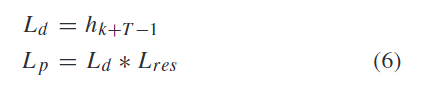 LMLN的算法可以总结为如下伪代码: 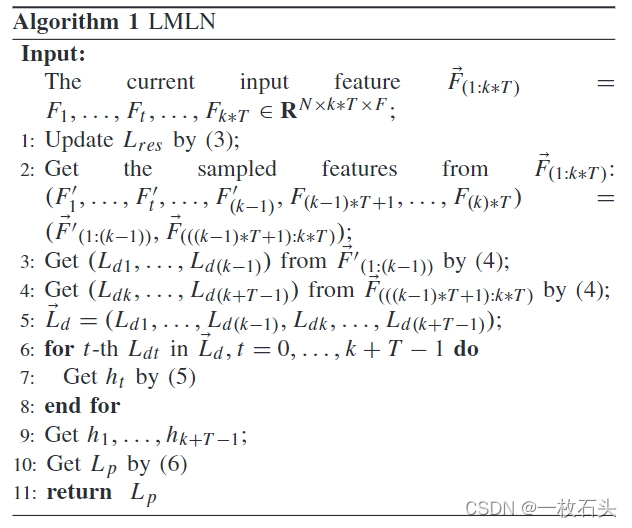
1.2 GCN
GCN是Fig. 2. 中的灰色部分,主要有4个部分。
(1)Temporal Convolution Layer
本模块的目的:to extract the high-dimensional local temporal information from the original traffic data.
对于输入 ,TCL的运算可以表示为: 
(2) Graph Temporal Convolution Layer*
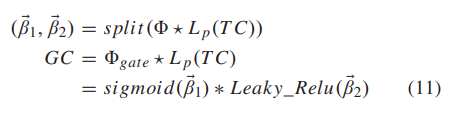
(3) Temporal Attention*
这里是借鉴了参考文献[19]中的Temporal Attention,目的是to explore the long-range time relation。
其运算公式为: 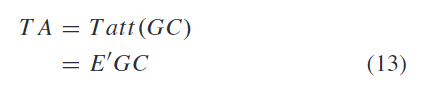 其中: 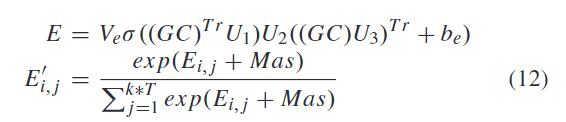
(4)Batch norm

GCN模块整体的运算过程如下: 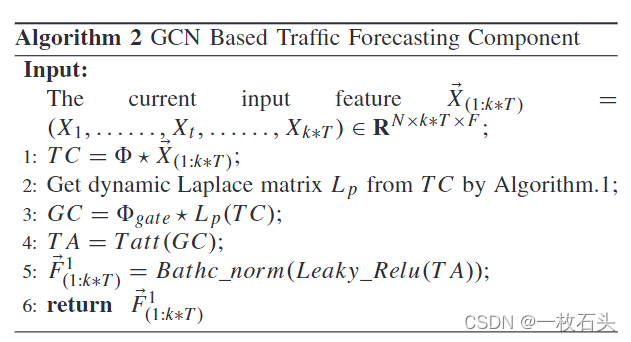
1.3 Loss Function
Output Layer 对 recent data、 daily-period data、 weekly-period data 分开处理。
主要是 recent data是连续的,而 daily-period data和weekly-period data经过了sample处理,被切分成k-i个单元,在时间轴上不连续。
所以使用: Conv_1×i to deal with the recent data, Conv_1×1 to reflect the k ? i feature unit dependently in the daily-period and weekly-period.
最后我们将所有convolution output看作我们模型的预测,采用l2_loss计算损失。 
2. 实验
2.1 实验结果
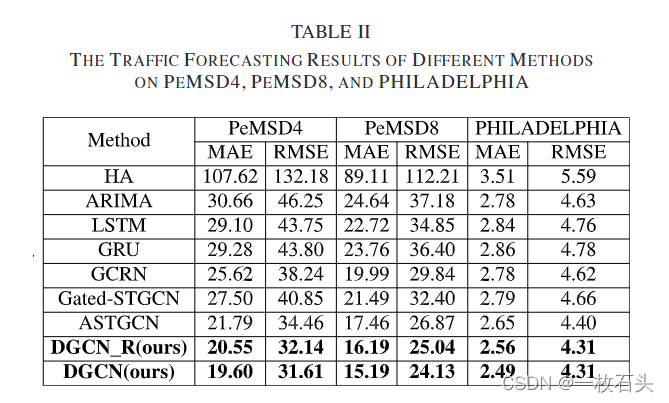 GCRN:Y. Seo, M. Defferrard, P. Vandergheynst, and X. Bresson, “Structured sequence modeling with graph convolutional recurrent networks,” in Proc. Int. Conf. Learn. Represent. (ICLR), 2017, pp. 362–373.
Gated-STGCN:B. Yu, H. Yin, and Z. Zhu, “Spatio-temporal graph convolutional networks: A deep learning framework for traffic forecasting,” in Proc. Int. Joint Conf. Artif. Intell., Jul. 2018, pp. 1–9.
ASTGCN: S. Guo, Y. Lin, N. Feng, C. Song, and H. Wan, “Attention based spatialtemporal graph convolutional networks for traffic flow forecasting,” in Proc. Assoc. Advance Artif. Intell. Conf.(AAAI), 2019, pp. 922–933.
2.2 消融实验
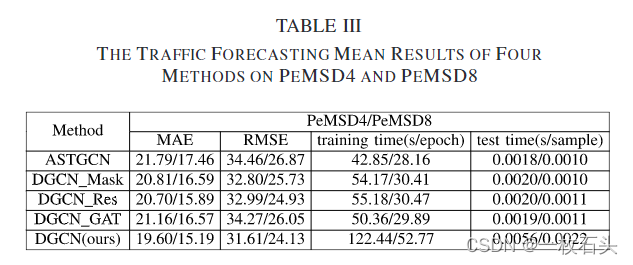
2.4
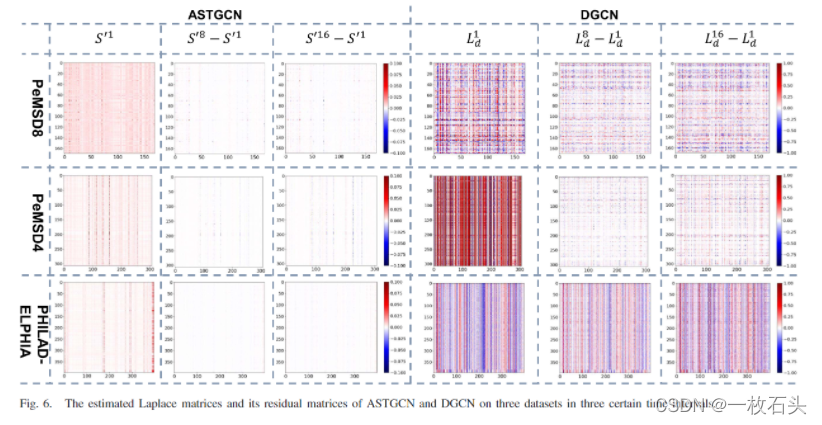
2.5

3. Introduction
本文的研究动机:Thus, a data-driven method [17] was proposed to optimize a parameterized global-temporal-sharing Laplace matrix in the network training phase, and it obtained richer space connections compared to the empirical one [15], [16]. But, this graph’s Laplace matrix is still fixed in the prediction phase, which cannot capture the dynamic information of the graph to improve the forecasting accuracy.
本文的一个比较重要的灵感提供文:AAAI19 ASTGCN:attention mechanism based Spatial-Temporal Graph Convolutional Network (ASTGCN) [18] was presented, in which a dynamic Laplace matrix of the graph was constructed at each input sequence data by the spatial attention mechanism [19].
ASTGCN的两个缺点:①it still utilized the empirical Laplace matrix [15], [16] as a mask;② ASTGCN ignored the inner temporal connection between Laplace matrices of the adjacent periods.
|