Zhang-Suen法
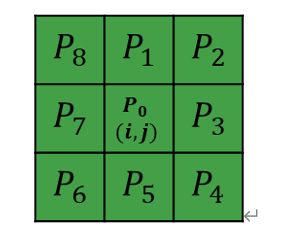 细化法(又称形态学骨架法)是通过对光条纹不断地进行腐蚀操作,剥离光条纹边界,得到单像素宽度的光条纹连通线(又称骨架)的形态学处理方法。该方法是通过细化技术,将光条纹区域的细化曲线作为光条纹中心线。 由于细化法是基于形态学的方法,只是对光条纹骨架进行提取,没有考虑到光条纹的横截面灰度分布特点。因此,细化法提取的光条纹中心线精度有限。另一方面,由于该方法需要大量时间来进行反复的细化操作,提取算法的运算速度被大大降低。 细化的方法有很多种,下面介绍一种常用的Zhang-Suen细化算法。细化算法的一般分为以下四个步骤, (1)标记将被删除的边界点; (2)删除做了标记的点; (3)继续标记将被删除的剩余的边界点; (4)删除标记过的点。反复应用这个基本过程,直到再也没有被删除的点为止,此时算法终止,生成了该区域的骨架。 假设目标像素点标记为1,背景像素点标记为0,在图上图中 的八个邻域如图所示,Zhang-Suen算法第一步循环所有前景像素点,对满足如下公式(1-5)的像素标记点进行删除。第二步和第一步类似,满足公式(1-6)的像素 标记点记为删除。循环上述两个步骤,直到两步中没有像素标记点记为删除为止,输出图像即为二值图像细化后的骨架。 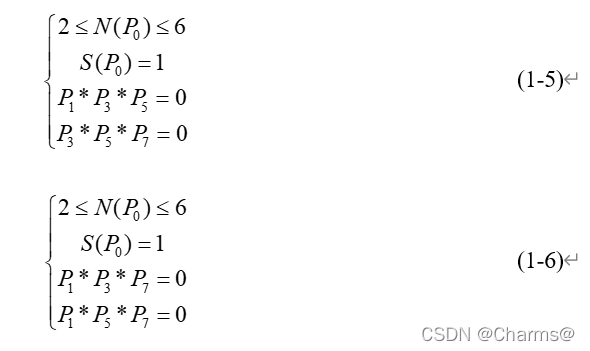 其中 N(P0)表示八个邻域中非零像素标记点的个数(二值图像只有0和1), S(P0)表示八个邻域中,按照顺时针方向,相邻两个像素出现0→1的次数,。 细化法由于需要做多次膨胀腐蚀操作,耗时较多,处理速度较慢,另外细化法对二值化的要求极高,往往因为噪声的干扰,将图像二值化会出现较大的误差。细化法属于形态学范畴,没有利用到灰度值之间的信息,因此对于复杂条件下的激光曲线提取效果较差。
python代码
import cv2
import numpy as np
import time
import matplotlib.pyplot as plt
def ROI(img):
indexXY = np.argwhere(img > 0)
minxy = np.min(indexXY, axis=0)
maxxy = np.max(indexXY, axis=0)
return minxy,maxxy
def neighbours(x,y,img):
i = img
x1,y1,x_1, y_1 = x+1, y-1, x-1, y+1
return [i[y1][x], i[y1][x1], i[y][x1], i[y_1][x1],
i[y_1][x], i[y_1][x_1], i[y][x_1], i[y1][x_1]]
def transitions(neighbours):
n = neighbours + neighbours[0:1]
return sum((n1, n2) == (0, 1) for n1, n2 in zip(n, n[1:]))
def ZhangSuenPlus(image):
"""
运行时间55s
:param image:
:return:
"""
changing1 = changing2 = [(-1, -1)]
while changing1 or changing2:
changing1 = []
for y in range(1, len(image) - 1):
for x in range(1, len(image[0]) - 1):
P2,P3,P4,P5,P6,P7,P8,P9 = n = neighbours(x, y, image)
if (image[y][x] == 1 and
P4 * P6 * P8 == 0 and
P2 * P4 * P6 == 0 and
transitions(n) == 1 and
2 <= sum(n) <= 6):
changing1.append((x,y))
for x, y in changing1: image[y][x] = 0
changing2 = []
for y in range(1, len(image) - 1):
for x in range(1, len(image[0]) - 1):
P2,P3,P4,P5,P6,P7,P8,P9 = n = neighbours(x, y, image)
if (image[y][x] == 1 and
P2 * P6 * P8 == 0 and
P2 * P4 * P8 == 0 and
transitions(n) == 1 and
2 <= sum(n) <= 6):
changing2.append((x,y))
for x, y in changing2: image[y][x] = 0
flags = image>0
image[flags] = 255
return image
def ZhangSuenPlus02(image):
"""
# 运行时间12.135秒
:param image:
:return:
"""
indexXY = np.argwhere(image>0)
minxy = np.min(indexXY,axis=0)
maxxy = np.max(indexXY,axis=0)
roi = image[minxy[0]-1:maxxy[0]+2,minxy[1]-1:maxxy[1]+2]
changing1 = changing2 = [(-1, -1)]
while changing1 or changing2:
changing1 = []
for y in range(1, len(roi) - 1):
for x in range(1, len(roi[0]) - 1):
if roi[y][x] == 1:
P2,P3,P4,P5,P6,P7,P8,P9 = n = neighbours(x, y, roi)
if (
P4 * P6 * P8 == 0 and
P2 * P4 * P6 == 0 and
transitions(n) == 1 and
2 <= sum(n) <= 6):
changing1.append((x,y))
for x, y in changing1: roi[y][x] = 0
changing2 = []
for y in range(1, len(roi) - 1):
for x in range(1, len(roi[0]) - 1):
if roi[y][x] == 1:
P2,P3,P4,P5,P6,P7,P8,P9 = n = neighbours(x, y, roi)
if (
P2 * P6 * P8 == 0 and
P2 * P4 * P8 == 0 and
transitions(n) == 1 and
2 <= sum(n) <= 6):
changing2.append((x,y))
for x, y in changing2: roi[y][x] = 0
flags = roi>0
roi[flags] = 255
return image
def ZhangSuenPlus03(image):
"""
# 运行时间9秒
:param image:
:return:
"""
indexXY = np.argwhere(image>0)
minxy = np.min(indexXY,axis=0)
maxxy = np.max(indexXY,axis=0)
roi = image[minxy[0]-1:maxxy[0]+2,minxy[1]-1:maxxy[1]+2]
changing1 = changing2 = [(-1, -1)]
while changing1 or changing2:
indexXY = np.argwhere(roi>0)
minxy = np.min(indexXY, axis=0)
maxxy = np.max(indexXY, axis=0)
roi = roi[minxy[0] - 1:maxxy[0] + 2, minxy[1] - 1:maxxy[1] + 2]
changing1 = []
for y in range(1, len(roi) - 1):
for x in range(1, len(roi[0]) - 1):
if roi[y][x] == 1:
P2,P3,P4,P5,P6,P7,P8,P9 = n = neighbours(x, y, roi)
if (
P4 * P6 * P8 == 0 and
P2 * P4 * P6 == 0 and
transitions(n) == 1 and
2 <= sum(n) <= 6):
changing1.append((x,y))
for x, y in changing1: roi[y][x] = 0
changing2 = []
for y in range(1, len(roi) - 1):
for x in range(1, len(roi[0]) - 1):
if roi[y][x] == 1:
P2,P3,P4,P5,P6,P7,P8,P9 = n = neighbours(x, y, roi)
if (
P2 * P6 * P8 == 0 and
P2 * P4 * P8 == 0 and
transitions(n) == 1 and
2 <= sum(n) <= 6):
changing2.append((x,y))
for x, y in changing2: roi[y][x] = 0
flags = roi>0
roi[flags] = 255
return image
def ZhangSuen_Bad(img):
"""
将灰度值转化为0和1,企图加速计算过程
运行时间21.28s
thresh计算失败
:param img:
:return:
"""
copyMat = img.copy()
k = 0
row,col= img.shape
row = row-1
col = col-1
while(True):
k= k+1
stop= False
for i in range(1,row):
for j in range(1,col):
if img[i,j]>0:
print(">0")
p1 = 1 if img[i,j]>0 else 0
p2 = 1 if img[i-1,j]>0 else 0
p3 = 1 if img[i-1,j+1]>0 else 0
p4 = 1 if img[i, j+1] > 0 else 0
p5 = 1 if img[i+1, j+1] > 0 else 0
p6 = 1 if img[i+1, j] > 0 else 0
p7 = 1 if img[i+1, j-1] > 0 else 0
p8 = 1 if img[i,j-1] > 0 else 0
p9 = 1 if img[i-1, j-1] > 0 else 0
np1 = p2+p3+p4+p5+p6+p7+p8+p9
sp2 = 1 if (p2 == 0 and p3 == 1) else 0
sp3 = 1 if (p3 == 0 and p4 == 1) else 0
sp4 = 1 if (p4 == 0 and p5 == 1) else 0
sp5 = 1 if (p5 == 0 and p6 == 1) else 0
sp6 = 1 if (p6 == 0 and p7 == 1) else 0
sp7 = 1 if (p7 == 0 and p8 == 1) else 0
sp8 = 1 if (p8 == 0 and p9 == 1) else 0
sp9 = 1 if (p9 == 0 and p2 == 1) else 0
sp1 = sp2 + sp3 + sp4 + sp5 + sp6 + sp7 + sp8 + sp9
if np1>=2 and np1<=6 and sp1==1 and(p2*p4*p6)==0 and (p4*p6*p8)==0:
stop = True
copyMat[i,j] = 0
print("success")
img = copyMat.copy()
for i in range(1,row):
for j in range(1,col):
if img[i,j]>0:
print(">>")
p2 = 1 if img[i - 1, j] > 0 else 0
p3 = 1 if img[i - 1, j + 1] > 0 else 0
p4 = 1 if img[i, j + 1] > 0 else 0
p5 = 1 if img[i + 1, j + 1] > 0 else 0
p6 = 1 if img[i + 1, j] > 0 else 0
p7 = 1 if img[i + 1, j - 1] > 0 else 0
p8 = 1 if img[i, j - 1] > 0 else 0
p9 = 1 if img[i - 1, j - 1] > 0 else 0
np1 = p2 + p3 + p4 + p5 + p6 + p7 + p8 + p9
sp2 = 1 if (p2 == 0 and p3 == 1) else 0
sp3 = 1 if (p3 == 0 and p4 == 1) else 0
sp4 = 1 if (p4 == 0 and p5 == 1) else 0
sp5 = 1 if (p5 == 0 and p6 == 1) else 0
sp6 = 1 if (p6 == 0 and p7 == 1) else 0
sp7 = 1 if (p7 == 0 and p8 == 1) else 0
sp8 = 1 if (p8 == 0 and p9 == 1) else 0
sp9 = 1 if (p9 == 0 and p2 == 1) else 0
sp1 = sp2 + sp3 + sp4 + sp5 + sp6 + sp7 + sp8 + sp9
if np1 >= 2 and np1 <= 6 and sp1 == 1 and (p2*p4*p8) == 0 and (p2*p6*p8) == 0:
stop = True
copyMat[i,j] = 0
print("success")
img = copyMat.copy()
if(not stop):
break
resImg = copyMat.copy()
flags = resImg>0
resImg[flags] = 255
return resImg
def ZhangSuen(img):
"""
运行时间20.7s
:param img:
:return:
"""
roi = img
k = 0
row,col= roi.shape
changing1 = changing2 = [(-1, -1)]
while changing1 or changing2:
changing1 = []
for i in range(1,row-1):
for j in range(1,col-1):
if roi[i,j]==1:
p2 = roi[i-1,j]
p3 = roi[i-1,j+1]
p4 = roi[i, j+1]
p5 = roi[i+1, j+1]
p6 = roi[i+1, j]
p7 = roi[i+1, j-1]
p8 = roi[i,j-1]
p9 = roi[i-1, j-1]
np1 = p2+p3+p4+p5+p6+p7+p8+p9
sp2 = 1 if (p2,p3)==(0,1) else 0
sp3 = 1 if (p3,p4)==(0,1) else 0
sp4 = 1 if (p4,p5)==(0,1) else 0
sp5 = 1 if (p5,p6)==(0,1) else 0
sp6 = 1 if (p6,p7)==(0,1) else 0
sp7 = 1 if (p7,p8)==(0,1) else 0
sp8 = 1 if (p8,p9)==(0,1) else 0
sp9 = 1 if (p9,p2)==(0,1) else 0
sp1 = sp2 + sp3 + sp4 + sp5 + sp6 + sp7 + sp8 + sp9
if 2<=np1<=6 and sp1==1 and(p2*p4*p6)==0 and (p4*p6*p8)==0:
changing1.append([i,j])
for x,y in changing1:roi[x,y] = 0
changing2 = []
for i in range(1,row-1):
for j in range(1,col-1):
if roi[i,j]==1:
p2 = roi[i - 1, j]
p3 = roi[i - 1, j + 1]
p4 = roi[i, j + 1]
p5 = roi[i + 1, j + 1]
p6 = roi[i + 1, j]
p7 = roi[i + 1, j - 1]
p8 = roi[i, j - 1]
p9 = roi[i - 1, j - 1]
np1 = p2 + p3 + p4 + p5 + p6 + p7 + p8 + p9
sp2 = 1 if (p2, p3) == (0, 1) else 0
sp3 = 1 if (p3, p4) == (0, 1) else 0
sp4 = 1 if (p4, p5) == (0, 1) else 0
sp5 = 1 if (p5, p6) == (0, 1) else 0
sp6 = 1 if (p6, p7) == (0, 1) else 0
sp7 = 1 if (p7, p8) == (0, 1) else 0
sp8 = 1 if (p8, p9) == (0, 1) else 0
sp9 = 1 if (p9, p2) == (0, 1) else 0
sp1 = sp2 + sp3 + sp4 + sp5 + sp6 + sp7 + sp8 + sp9
if 2<=np1<= 6 and sp1 == 1 and (p2*p4*p8) == 0 and (p2*p6*p8) == 0:
changing2.append([i,j])
for x,y in changing2:roi[x,y] = 0
flags = roi>0
roi[flags] = 255
return roi
def Draw():
plt.figure()
plt.subplot(131)
plt.imshow(cv2.cvtColor(img,cv2.COLOR_BGR2RGB))
plt.title("origin image")
plt.axis("off")
plt.subplot(132)
plt.title("res image")
plt.axis("off")
plt.subplot(133)
plt.title("resP image")
plt.axis("off")
plt.show()
def XiHua(img):
kernel_d = cv2.getStructuringElement(cv2.MORPH_RECT, (5, 5))
image_d = cv2.dilate(image, kernel_d, iterations=8)
kernel_e = cv2.getStructuringElement(cv2.MORPH_RECT, (3, 3))
image_e = cv2.erode(image_d, kernel_e)
image_ske = morphology.skeletonize(image_e)
image_e = np.multiply(image_e, image_ske)
return image_e
if __name__ == "__main__":
import os
import time
from skimage import morphology
image_path = './image/'
save_path = './paper/zhang-suen/'
sum_time = 0
if not os.path.isdir(save_path): os.makedirs(save_path)
for img in os.listdir(image_path):
image = cv2.imread(os.path.join(image_path, img))
gray = cv2.cvtColor(image, cv2.COLOR_BGR2GRAY)
ret, thresh = cv2.threshold(gray, 100, 255, cv2.THRESH_BINARY)
time1 = time.time()
result = ZhangSuen(thresh)
time2 = time.time()
temp_time = time2 - time1
sum_time += temp_time
cv2.imwrite(os.path.join(save_path, img), result)
per_time = sum_time / len(os.listdir(image_path))
print("average image time:", per_time)
|